In RAG, after reading the files, the main task is to split the data into smaller chunks and then embed these features to express their semantics. The location of this process in RAG is shown in the figure below.
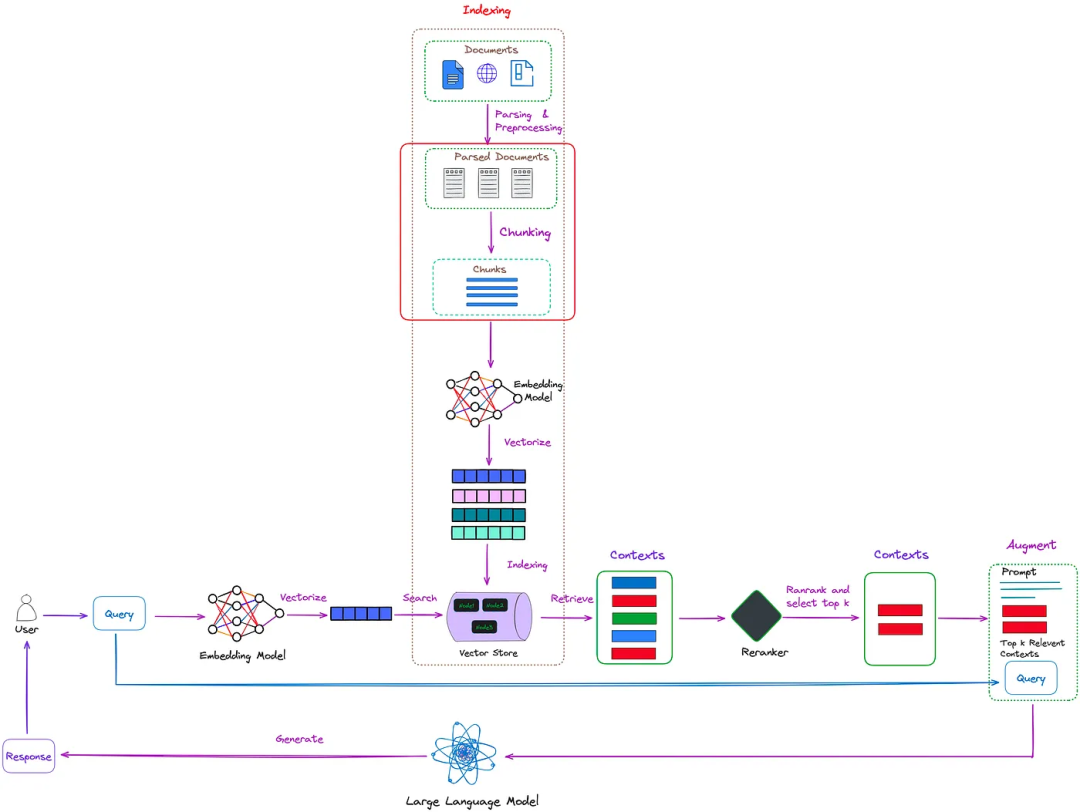
The most common chunking method is rule-based, using techniques such as fixed chunk sizes or overlapping adjacent chunks. For multi-level documents, we can use the RecursiveCharacterTextSplitter provided by Langchain. It allows us to define multi-level delimiters.
However, in practical applications, due to the rigidity of predefined rules (such as chunk size or the size of overlapping parts), rule-based chunking methods can easily lead to issues such as incomplete retrieval context or chunks that are too large and contain noise. Therefore, in terms of chunking, the most elegant method is obviously semantic chunking. The goal of semantic chunking is to ensure that each chunk contains semantically independent information as much as possible.
This article will explore methods for semantic chunking, and we will introduce three types of methods:
-
Embedding-based methods -
Model-based methods -
Large Language Model (LLM)-based methods
Embedding-based methods
Both LlamaIndex and Langchain provide embedding-based semantic chunkers. Taking LlamaIndex as an example, the core code flow is:
# load documents
dir_path = "YOUR_DIR_PATH"
documents = SimpleDirectoryReader(dir_path).load_data()
embed_model = OpenAIEmbedding()
splitter = SemanticSplitterNodeParser(
buffer_size=1, breakpoint_percentile_threshold=95, embed_model=embed_model
)
nodes = splitter.get_nodes_from_documents(documents)
By tracking the flow in the semantic_splitter.py file, we can find that its main process is as follows:
The “sentences” mentioned above is a Python list where each element is a dictionary containing four key-value pairs. The meanings of these keys are:
-
sentence
: The current sentence -
index
: The index of the current sentence -
combined_sentence
: A sliding window that by default contains[index - self.buffer_size, index, index + self.buffer_size]
(typically,self.buffer_size = 1
). This window is used to calculate the semantic relevance between sentences. The purpose of combining the previous and subsequent sentences is to reduce noise and better capture the relationships between consecutive sentences. -
combined_sentence_embedding
: The embedding vector of the sentence
From the above analysis, we can see that embedding-based semantic chunking essentially calculates similarity through a sliding window (combined_sentence
). Sentences that are adjacent and meet the threshold are grouped into one chunk.
Experiments show that the results produced by the embedding-based chunking method seem somewhat “rough”. Moreover, an analysis of the source code indicates that this method is based on page-level content, and it does not directly address the issue of chunking content that spans multiple pages. To a large extent, the effectiveness of embedding methods is closely related to the selected embedding model. As for its actual performance, it still needs to be tested over time and further evaluated.
Model-based methods
▶Naive BERT
The pre-training process of BERT includes a binary classification task—Next Sentence Prediction (NSP)—aimed at allowing the model to learn the relationship between two sentences. In this process, BERT receives two sentences as input and predicts whether the second sentence follows the first.
We can design a simple chunking method based on this principle. For a document, we first split it into independent sentences. Then, using a sliding window approach, we send adjacent pairs of sentences into the BERT model for NSP judgment, as shown in the figure below:If the predicted score is below a preset threshold, it indicates a weak semantic relationship between the two sentences. We can then take this point as a text segmentation point, such as the split between sentence 2 and sentence 3 in the figure above.
One advantage of this method is that it can be used directly without additional training or fine-tuning. However, this method only considers adjacent sentences when determining text segmentation points, ignoring information from farther segments. Additionally, the prediction efficiency of this method is relatively low.
▶Cross Segment Attention
The paper “Text Segmentation by Cross Segment Attention”[1] proposes three models based on cross-segment attention, as shown in the figure below:Figure (a) shows the cross-segment BERT model, which defines text segmentation as a sentence classification task. The context on both sides of the potential segmentation point (each marked on the left and right) is input into the model. The hidden state corresponding to
[CLS]
is passed to the softmax classifier to determine whether to segment at the potential segmentation point. The paper also introduces two other models. One uses the BERT model to obtain the vector representation of each sentence. These vector representations of consecutive sentences are then input into a bidirectional LSTM (or another BERT) to predict whether each sentence is a text segmentation boundary.
These three models achieve state-of-the-art results, as shown in the figure below:
However, so far, only the training implementation[2] of this paper has been found. No publicly available models for inference have been discovered yet. Therefore, to apply them to practical text segmentation tasks, one needs to train the model manually.
▶SeqModel
The cross-segment model independently vectorizes each sentence without considering broader context information. The paper “Sequence Model with Self-Adaptive Sliding Window for Efficient Spoken Document Segmentation”[3] proposes further improvements, namely SeqModel. SeqModel leverages BERT to encode multiple sentences simultaneously, modeling dependencies within a longer context before calculating sentence vectors. It then predicts whether a text segmentation occurs after each sentence. Additionally, this model employs an adaptive sliding window method to enhance inference speed without sacrificing accuracy. A diagram of SeqModel is shown in the figure below:Currently, SeqModel can be used through the ModelScope framework[4]. For example, the following test code:
from modelscope.outputs import OutputKeys
from modelscope.pipelines import pipeline
from modelscope.utils.constant import Tasks
p = pipeline(
task = Tasks.document_segmentation,
model = 'damo/nlp_bert_document-segmentation_english-base'
)
result = p(documents='We demonstrate the importance of bidirectional pre-training for language representations. Unlike Radford et al. (2018), which uses unidirectional language models for pre-training, BERT uses masked language models to enable pretrained deep bidirectional representations. This is also in contrast to Peters et al. (2018a), which uses a shallow concatenation of independently trained left-to-right and right-to-left LMs. • We show that pre-trained representations reduce the need for many heavily-engineered taskspecific architectures. BERT is the first finetuning based representation model that achieves state-of-the-art performance on a large suite of sentence-level and token-level tasks, outperforming many task-specific architectures. Today is a good day')
print(result[OutputKeys.TEXT])
In summary, model-based semantic chunking methods still have significant room for improvement. One suggested improvement method by the authors is to create training data tailored for specific projects for domain fine-tuning.
LLM-based methods
The paper “Dense X Retrieval: What Retrieval Granularity Should We Use?”[5] introduces a new retrieval unit called proposition. A proposition is defined as an atomic expression in the text, each encapsulating a unique fact and presented in a concise, self-contained natural language format. This can be achieved by constructing prompts and combining them with LLM. Both LlamaIndex and Langchain have implemented related algorithms, and LlamaIndex’s implementation idea is to use the prompts provided in the paper to generate propositions:
Decompose the "Content" into clear and simple propositions, ensuring they are interpretable out of
context.
1. Split compound sentence into simple sentences. Maintain the original phrasing from the input
whenever possible.
2. For any named entity that is accompanied by additional descriptive information, separate this
information into its own distinct proposition.
3. Decontextualize the proposition by adding necessary modifier to nouns or entire sentences
and replacing pronouns (e.g., "it", "he", "she", "they", "this", "that") with the full name of the
entities they refer to.
4. Present the results as a list of strings, formatted in JSON.
Input: Title: ¯Eostre. Section: Theories and interpretations, Connection to Easter Hares. Content:
The earliest evidence for the Easter Hare (Osterhase) was recorded in south-west Germany in
1678 by the professor of medicine Georg Franck von Franckenau, but it remained unknown in
other parts of Germany until the 18th century. Scholar Richard Sermon writes that "hares were
frequently seen in gardens in spring, and thus may have served as a convenient explanation for the
origin of the colored eggs hidden there for children. Alternatively, there is a European tradition
that hares laid eggs, since a hare’s scratch or form and a lapwing’s nest look very similar, and
both occur on grassland and are first seen in the spring. In the nineteenth century the influence
of Easter cards, toys, and books was to make the Easter Hare/Rabbit popular throughout Europe.
German immigrants then exported the custom to Britain and America where it evolved into the
Easter Bunny."
Output: [ "The earliest evidence for the Easter Hare was recorded in south-west Germany in
1678 by Georg Franck von Franckenau.", "Georg Franck von Franckenau was a professor of
medicine.", "The evidence for the Easter Hare remained unknown in other parts of Germany until
the 18th century.", "Richard Sermon was a scholar.", "Richard Sermon writes a hypothesis about
the possible explanation for the connection between hares and the tradition during Easter", "Hares
were frequently seen in gardens in spring.", "Hares may have served as a convenient explanation
for the origin of the colored eggs hidden in gardens for children.", "There is a European tradition
that hares laid eggs.", "A hare’s scratch or form and a lapwing’s nest look very similar.", "Both
hares and lapwing’s nests occur on grassland and are first seen in the spring.", "In the nineteenth
century the influence of Easter cards, toys, and books was to make the Easter Hare/Rabbit popular
throughout Europe.", "German immigrants exported the custom of the Easter Hare/Rabbit to
Britain and America.", "The custom of the Easter Hare/Rabbit evolved into the Easter Bunny in
Britain and America." ]
Input: {node_text}
Output:
The corresponding Chinese is:
Decompose the "Content" into clear and simple propositions, ensuring they are interpretable out of
context.
1. Split compound sentence into simple sentences. Maintain the original phrasing from the input
whenever possible.
2. For any named entity that is accompanied by additional descriptive information, separate this
information into its own distinct proposition.
3. Decontextualize the proposition by adding necessary modifier to nouns or entire sentences
and replacing pronouns (e.g., "it", "he", "she", "they", "this", "that") with the full name of the
entities they refer to.
4. Present the results as a list of strings, formatted in JSON.
Input: Title: ¯Eostre. Section: Theories and interpretations, Connection to Easter Hares. Content:
The earliest evidence for the Easter Hare (Osterhase) was recorded in south-west Germany in
1678 by the professor of medicine Georg Franck von Franckenau, but it remained unknown in
other parts of Germany until the 18th century. Scholar Richard Sermon writes that "hares were
frequently seen in gardens in spring, and thus may have served as a convenient explanation for the
origin of the colored eggs hidden there for children. Alternatively, there is a European tradition
that hares laid eggs, since a hare’s scratch or form and a lapwing’s nest look very similar, and
both occur on grassland and are first seen in the spring. In the nineteenth century the influence
of Easter cards, toys, and books was to make the Easter Hare/Rabbit popular throughout Europe.
German immigrants then exported the custom to Britain and America where it evolved into the
Easter Bunny."
Output: [ "The earliest evidence for the Easter Hare was recorded in south-west Germany in
1678 by Georg Franck von Franckenau.", "Georg Franck von Franckenau was a professor of
medicine.", "The evidence for the Easter Hare remained unknown in other parts of Germany until
the 18th century.", "Richard Sermon was a scholar.", "Richard Sermon writes a hypothesis about
the possible explanation for the connection between hares and the tradition during Easter", "Hares
were frequently seen in gardens in spring.", "Hares may have served as a convenient explanation
for the origin of the colored eggs hidden in gardens for children.", "There is a European tradition
that hares laid eggs.", "A hare’s scratch or form and a lapwing’s nest look very similar.", "Both
hares and lapwing’s nests occur on grassland and are first seen in the spring.", "In the nineteenth
century the influence of Easter cards, toys, and books was to make the Easter Hare/Rabbit popular
throughout Europe.", "German immigrants exported the custom of the Easter Hare/Rabbit to
Britain and America.", "The custom of the Easter Hare/Rabbit evolved into the Easter Bunny in
Britain and America." ]
Input: {node_text}
Output:
Overall, this method of building propositions using LLM has achieved more refined text chunks. It forms an indexing structure from small to large with the original nodes, providing a novel approach to semantic chunking. However, this method relies on LLM, which is relatively costly.
Conclusion
Compared to using specific rules for chunk segmentation, semantic segmentation can retain contextual relationships to a certain extent. Therefore, the recalled content is also relatively more comprehensive, which helps achieve high-quality answers.
References
“Text Segmentation by Cross Segment Attention”: https://arxiv.org/abs/2004.14535
[2]Training implementation: https://github.com/aakash222/text-segmentation-NLP/
[3]“Sequence Model with Self-Adaptive Sliding Window for Efficient Spoken Document Segmentation”: https://arxiv.org/abs/2107.09278
[4]ModelScope framework: https://github.com/modelscope/modelscope/
[5]“Dense X Retrieval: What Retrieval Granularity Should We Use?”: https://arxiv.org/pdf/2312.06648.pdf