Is there a difference between LLM large models and embedding large models in the knowledge base domain? Why is it necessary to set up a separate embedding large model in the RAG field?
In the field of artificial intelligence, large language models (LLMs) and embedding models are two key technologies in natural language processing (NLP), especially playing an important role in knowledge base construction and information retrieval.
Although they both belong to the NLP category, there are significant differences in functionality, application scenarios, and resource requirements.
The main task of the embedding model is to convert text into numerical vector representations. These vectors can be used to calculate the similarity between texts, perform information retrieval, and conduct clustering analysis.
The output of the embedding model is numerical vectors. Computers lack the ability to understand the meaning of phrases; they can only see results like 01010111. However, humans perceive words as carrying intrinsic meanings and a wealth of universal knowledge.
Referring to a diagram by Professor Li Hongyi from National Taiwan University, let’s explain.
When we humans understand the differences between cat vs dog and dog vs flower, we naturally perceive that cats and dogs are closer species; whereas a dog and a flower are one animal and one plant, respectively, with greater divergence.
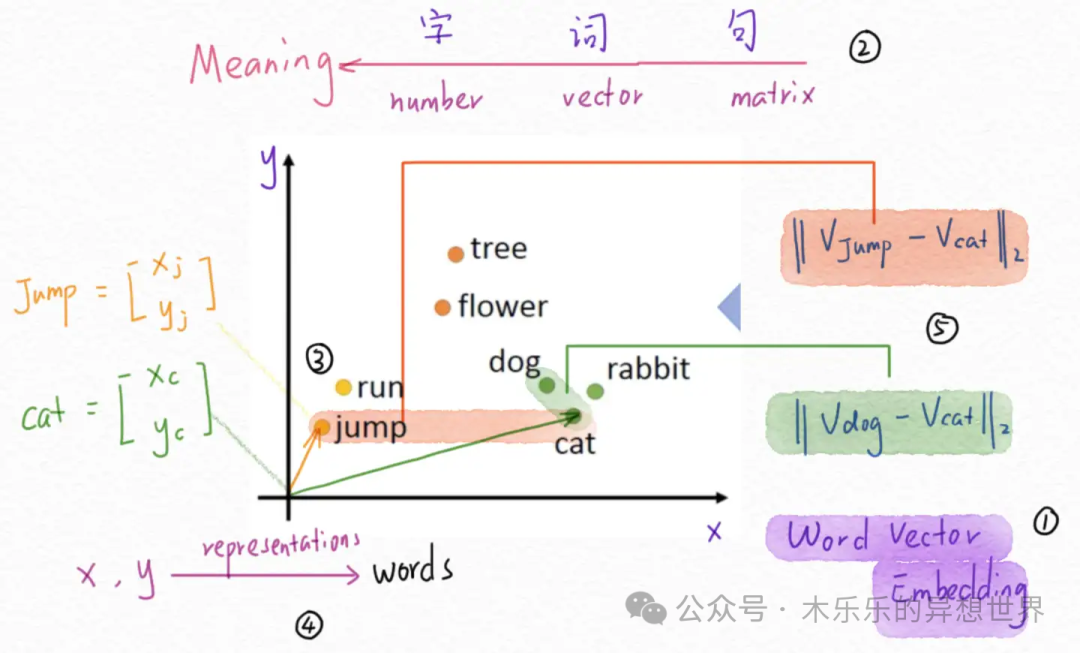
So, how can we enable computers to understand the meanings behind these words? The technology used for this is embedding. In simple terms, embedding is the process of converting words that computers cannot understand into a vector matrix.
The distances between similar or closely related word vectors are closer together. For example, the vectors for dog and cat, dog and rabbit are close; however, flower and dog are much further apart.
Embedding models are widely used in text similarity calculation, information retrieval, clustering, and recommendation systems.
In the large model knowledge base field, setting up a separate embedding model can reduce system resource usage and response latency, especially in large-scale knowledge base construction and information retrieval, significantly enhancing cost-effectiveness and efficiency. Typical applications include the following parts:
Typical Application Process
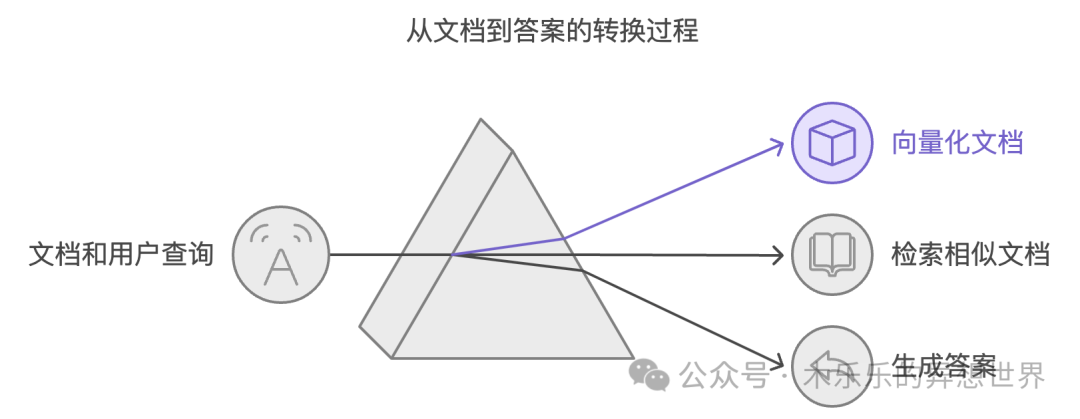
1. Knowledge Base Construction Phase
- Use the embedding model to convert documents into vectors, storing these vectors in a vector database (such as FAISS, Milvus)
2. Retrieval Phase
- Convert user queries into vectors using the same embedding model and quickly retrieve similar documents from the vector database
3. Answer Generation Phase
- Use the retrieved relevant documents as context to generate the final answer using LLM
Recommended Embedding Models
The embedding models supported by Crew.ai official components include:
- openai: OpenAI’s embedding model
- google: Google’s text embedding model
- azure: Azure OpenAI embedding
- ollama: Local embedding using Ollama
- vertexai: Google Cloud VertexAI embedding
- cohere: Cohere’s embedding model
- bedrock: AWS Bedrock embedding
- huggingface: Hugging Face model
- watson: IBM Watson embedding
Next, let’s take a look at how to install the embedding model provided by Ollama locally. The case we are using is nomic-embed-text.
Project reference link: https://ollama.com/search?c=embedding
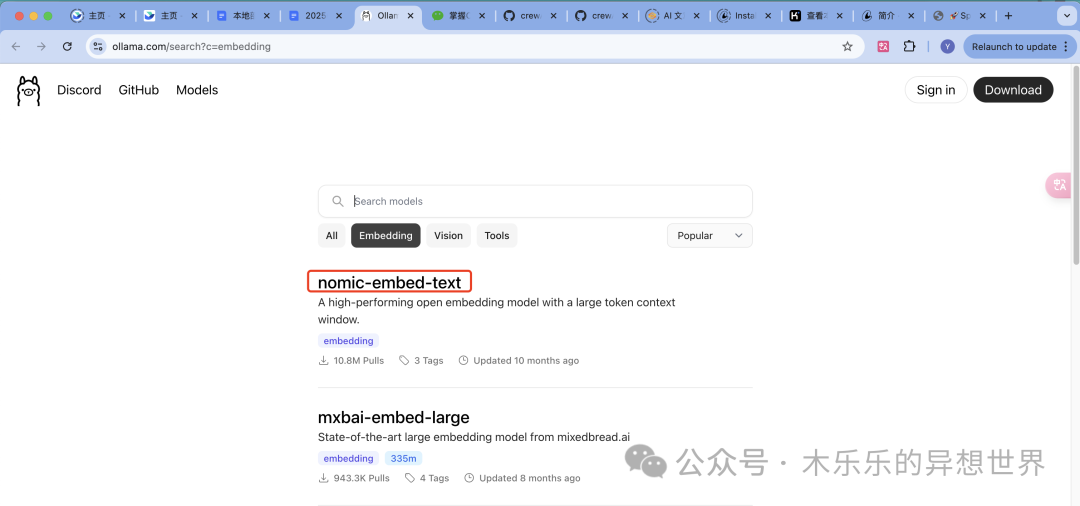
First, activate the corresponding working environment.
source crewai-env/bin/activate
Every time you start a new terminal session, you need to reactivate the virtual environment. If you see a label like (crewai) before the command prompt, it indicates that the environment has been activated correctly.

Install dependencies
pip install crewai ollama
If you see this, it indicates that you have activated the environment
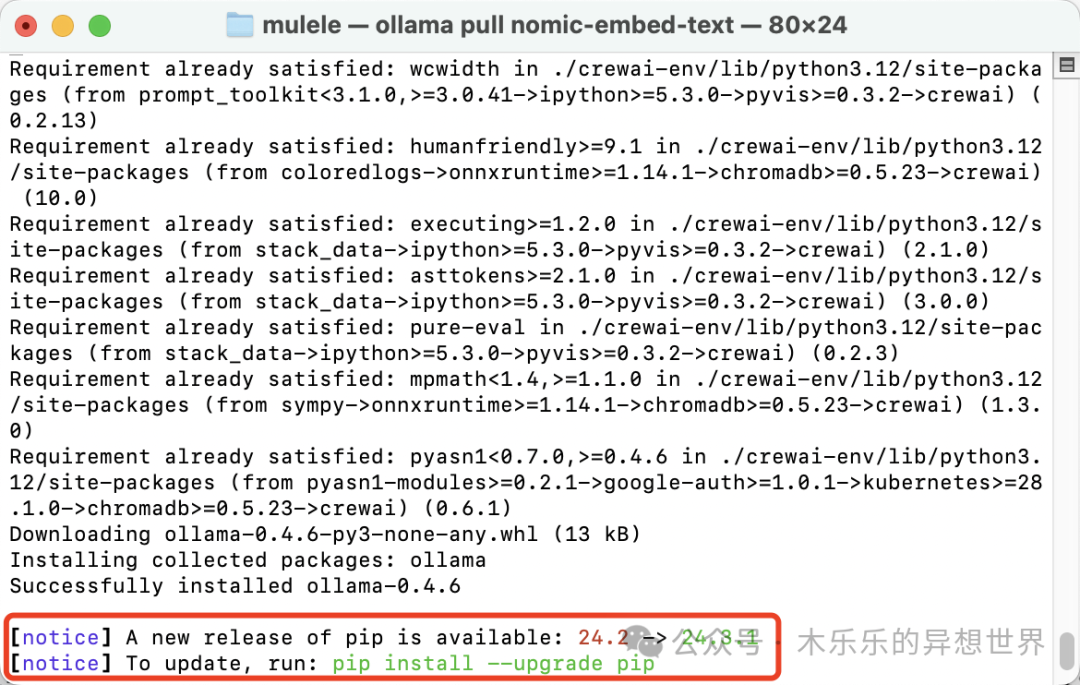
Start Ollama
ollama serve
Install the embedding model
ollama pull nomic-embed-text
Since it needs to be configured for other services, we need to solve the issue of obtaining Ollama’s embedding host.
To obtain Ollama’s embedding host, there are several methods:
Default address:
Ollama runs by default at http://localhost:11434. If you installed Ollama on your local machine, you typically do not need to change this address.
If you are unsure about this port, you can verify whether the Ollama service is running and its address by executing this method.
# Check Ollama service status
curl http://localhost:11434/api/version
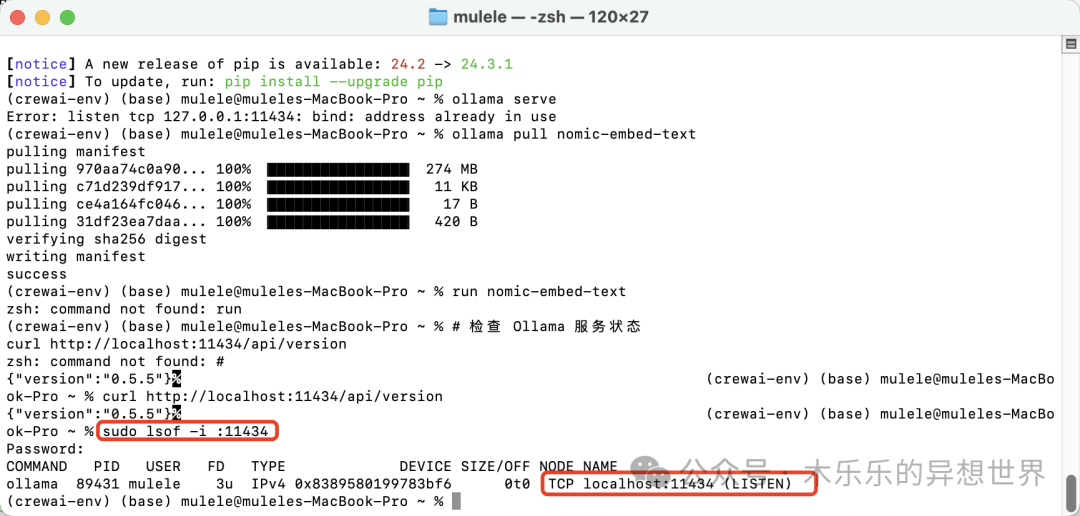
If you see the listening result for 11434, it means this port is the one that Ollama’s embedding model is open on.
At this point, it indicates that the embedding model of Ollama has been successfully installed. It can now be integrated with other applications and services.