
Source: DeepHub IMBA
This article is approximately 1800 words and is suggested to be read in 6 minutes.
This article will introduce how to integrate and create a scalable and customizable agent RAG.
Building large language model applications can be quite challenging, especially when we have to choose between different frameworks like LangChain and LlamaIndex. LlamaIndex excels in intelligent search and data retrieval, while LangChain serves as a more general application framework, providing better compatibility with various platforms.
This article will introduce how to integrate LlamaIndex and LangChain to create a scalable and customizable agent RAG (Retrieval-Augmented Generation) application, leveraging the powerful capabilities of both technologies to develop efficient applications capable of handling complex queries and providing accurate answers.
Before we proceed with the implementation, we need to briefly introduce some knowledge about agent RAG:
Agent RAG is a proxy-based implementation of RAG. Compared to traditional general RAG methods, agent RAG has significantly improved autonomy and decision-making capabilities. We create a complex reasoning loop by authorizing large language models (LLMs) to access multiple RAG query engines. Each query engine acts as a tool that can be called by the LLM as needed. This structure not only makes it possible to execute complex decisions but also expands the system’s ability to answer various queries, providing users with the most appropriate responses.
In this way, agent RAG can consider the diversity and quality of information sources while providing answers, thereby achieving higher accuracy and relevance in responses. The implementation of this model provides more powerful tools for handling complex problems and delivering innovative solutions.
First, we define the basic LLM and embedding model:
# LLM
llm = ChatOpenAI(model_name="gpt-4-1106-preview", temperature=0, streaming=True)
# Embedding Model
embed_model = OpenAIEmbedding(
model="text-embedding-3-small", embed_batch_size=100
)
# Set LlamaIndex Configs
Settings.llm = llm
Settings.embed_model = embed_model
Then, we use LlamaIndex’s indexing and retrieval capabilities to define separate query engines for the documents.
# Building Indexes for each of the Documents
try:
storage_context = StorageContext.from_defaults(
persist_dir="./storage/lyft"
)
lyft_index = load_index_from_storage(storage_context)
storage_context = StorageContext.from_defaults(
persist_dir="./storage/uber"
)
uber_index = load_index_from_storage(storage_context)
index_loaded = True
print("Index was already created. We just loaded it from the local storage.")
except:
index_loaded = False
print("Index is not present. We need to create it again.")
if not index_loaded:
print("Creating Index..")
# load data
lyft_docs = SimpleDirectoryReader(
input_files=["./data/10k/lyft_2021.pdf"]
).load_data()
uber_docs = SimpleDirectoryReader(
input_files=["./data/10k/uber_2021.pdf"]
).load_data()
# build index
lyft_index = VectorStoreIndex.from_documents(lyft_docs)
uber_index = VectorStoreIndex.from_documents(uber_docs)
# persist index
lyft_index.storage_context.persist(persist_dir="./storage/lyft")
uber_index.storage_context.persist(persist_dir="./storage/uber")
index_loaded = True
# Creating Query engines on top of the indexes
lyft_engine = lyft_index.as_query_engine(similarity_top_k=3)
uber_engine = uber_index.as_query_engine(similarity_top_k=3)
print("LlamaIndex Query Engines created successfully.")
Then, we use LlamaIndex’s QueryEngineTool abstract class to convert query engines into tools that will later be provided to the LLM.
# creating tools for each of our query engines
query_engine_tools = [
QueryEngineTool(
query_engine=lyft_engine,
metadata=ToolMetadata(
name="lyft_10k",
description=(
"Provides information about Lyft financials for year 2021. "
"Use a detailed plain text question as input to the tool."
),
),
),
QueryEngineTool(
query_engine=uber_engine,
metadata=ToolMetadata(
name="uber_10k",
description=(
"Provides information about Uber financials for year 2021. "
"Use a detailed plain text question as input to the tool."
),
),
),
]
Then we convert the LlamaIndex tools into a format compatible with the LangChain agent, allowing for integration with LangChain.
llamaindex_to_langchain_converted_tools = [t.to_langchain_tool() for t in query_engine_tools]
In addition, we also define an additional LangChain tool with web search functionality. This allows for page searches:
search = DuckDuckGoSearchRun()
duckduckgo_tool = Tool(
name='DuckDuckGoSearch',
func=search.run,
description='Use for when you need to perform an internet search to find information that another tool cannot provide.'
)
langchain_tools = [duckduckgo_tool]
# Combine to create final list of tools
tools = llamaindex_to_langchain_converted_tools + langchain_tools
Next, we initialize the LangChain agent.
system_context = "You are a stock market expert.\ You will answer questions about Uber and Lyft companies as in the persona of a veteran stock market investor."
prompt = ChatPromptTemplate.from_messages(
[
(
"system",
system_context,
),
("placeholder", "{chat_history}"),
("human", "{input}"),
("placeholder", "{agent_scratchpad}"),
]
)
# Construct the Tools agent
agent = create_tool_calling_agent(llm, tools, prompt,)
# Create an agent executor by passing in the agent and tools
agent_executor = AgentExecutor(agent=agent, tools=tools, verbose=True, return_intermediate_steps=True, handle_parsing_errors=True, max_iterations=10)
Then we can proceed with testing.
Test 1:
question = "What was Lyft's revenue growth in 2021?"
response = agent_executor.invoke({"input": question})
print("\nFinal Response:", response['output'])
The agent correctly called the lyft_10k query engine tool.
Test 2:
question = "Is Uber profitable?"
response = agent_executor.invoke({"input": question})
print("\nFinal Response:", response['output'])
The agent correctly called the uber_10k query engine tool.
Test 3:
question = "List me the names of Uber's board of directors."
response = agent_executor.invoke({"input": question})
print("\nFinal Response:", response['output'])
This information exceeds the capabilities of any retrieval tool, so the agent decides to call the external search tool and then return the result.
As we can see, our example perfectly combines the strengths of both approaches. By introducing multiple agents, the efficiency and accuracy of the system can be further enhanced. Each agent can specialize in handling different subsets of documents within the same domain, making information retrieval more refined and professional.
We can designate one agent to act as the coordinator or supervisor of these agents. This agent is responsible for monitoring and regulating the activities of each agent, ensuring the coordination of information flow, and optimizing the overall query process. This hierarchical management structure not only optimizes the data processing workflow but also improves response speed and accuracy, making the entire system more efficient and reliable in handling complex queries.
Through this method, we can achieve a more dynamic and adaptable RAG system that can better meet the ever-changing user demands and tackle diverse information challenges. We hope this article helps you understand how to effectively integrate LlamaIndex and LangChain to build an efficient, scalable agent RAG application.
Editor: Huang Jiyan
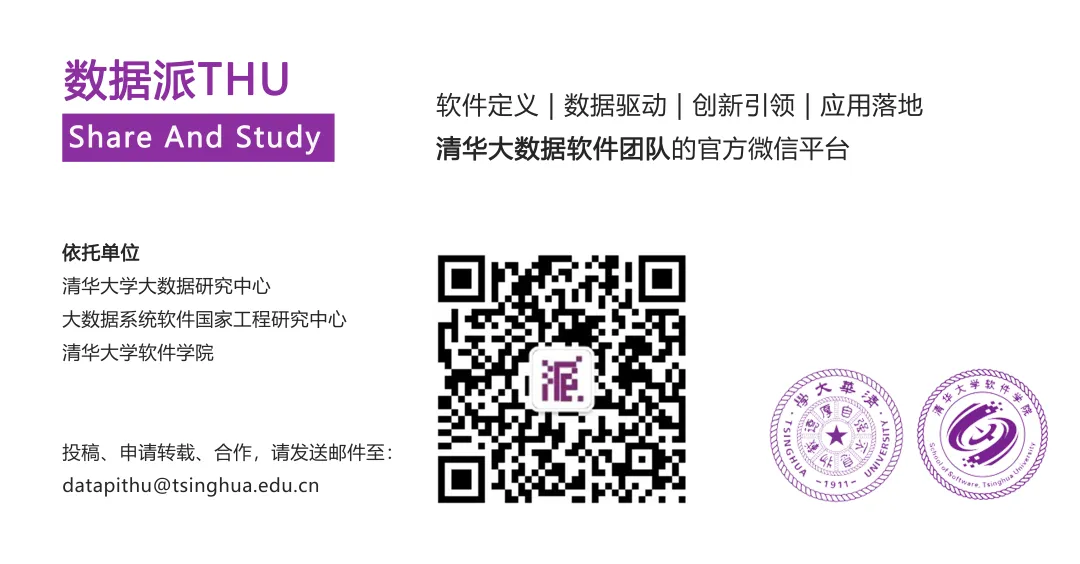