If you have used the ChatGPT API extensively or have heard of AutoGPT, you might know that it relies on the language framework LangChain[1]. LangChain allows large language models to access the internet, interact with various APIs, and even execute system commands.
The number of tokens supported by ChatGPT’s prompt is limited, but using LangChain makes it easy to achieve the effects of ChatPDF/ChatDoc. Even if a text has millions of words, LangChain can summarize its content and allow you to ask questions about it.
Question Answering over Docs[2] is an example provided in the official LangChain documentation. If you are using the official OpenAI API, you can simply copy and paste the code above to ask questions about large texts.
If you are using the Azure OpenAI interface, it can be a bit more complicated and requires additional setup. Let’s take a look at the pitfalls I encountered during the process.
First, we copy the following four lines of code:
from langchain.document_loaders import TextLoader
from langchain.indexes import VectorstoreIndexCreator
loader = TextLoader('article.txt')
index = VectorstoreIndexCreator().from_loaders([loader])
print(index)
Here, article.txt
is just a random article from my blog, as shown in the image below:
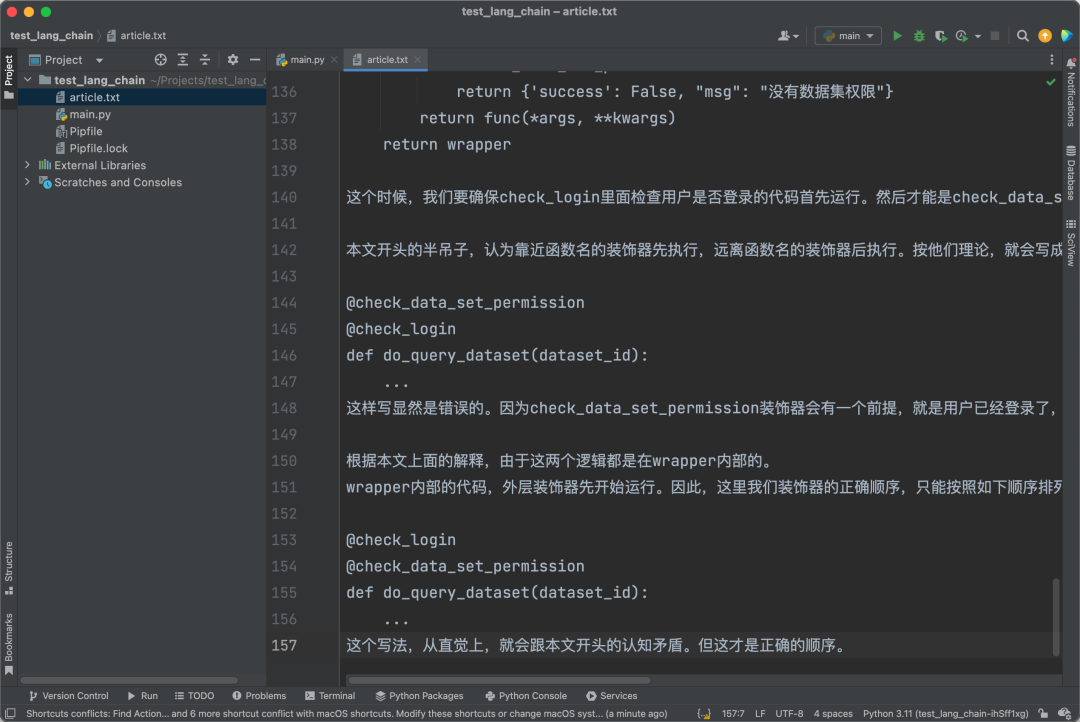
Running it directly will definitely result in an error because we haven’t configured the API-related information yet:
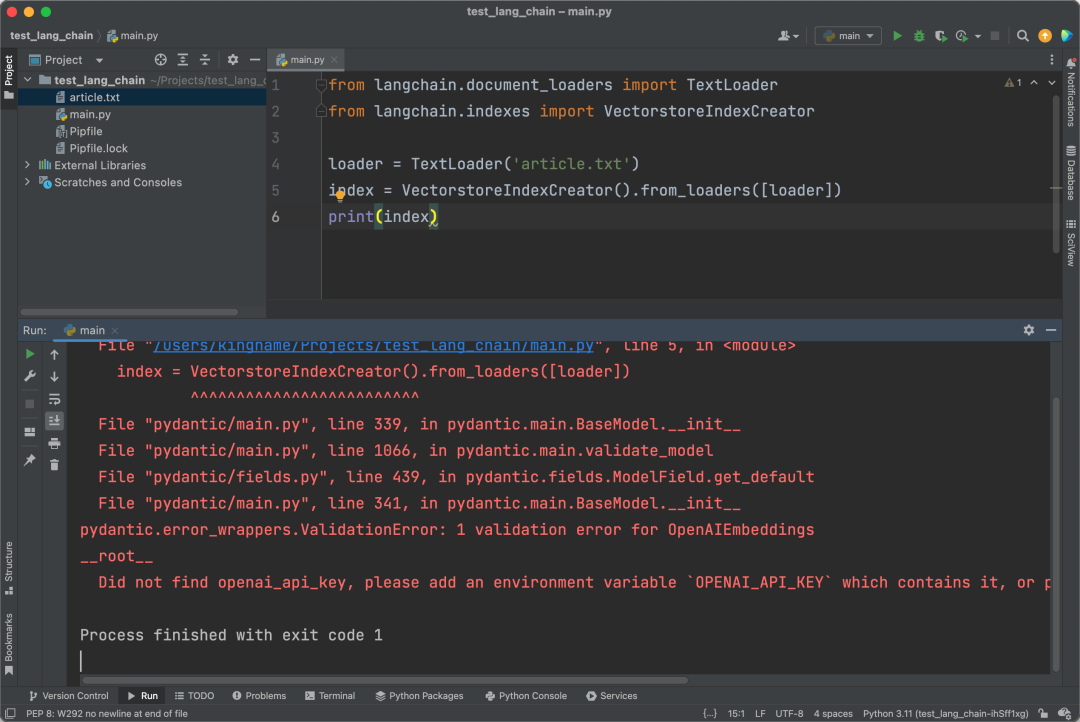
Since we are using the Azure OpenAI interface, we need to set some additional parameters through environment variables:
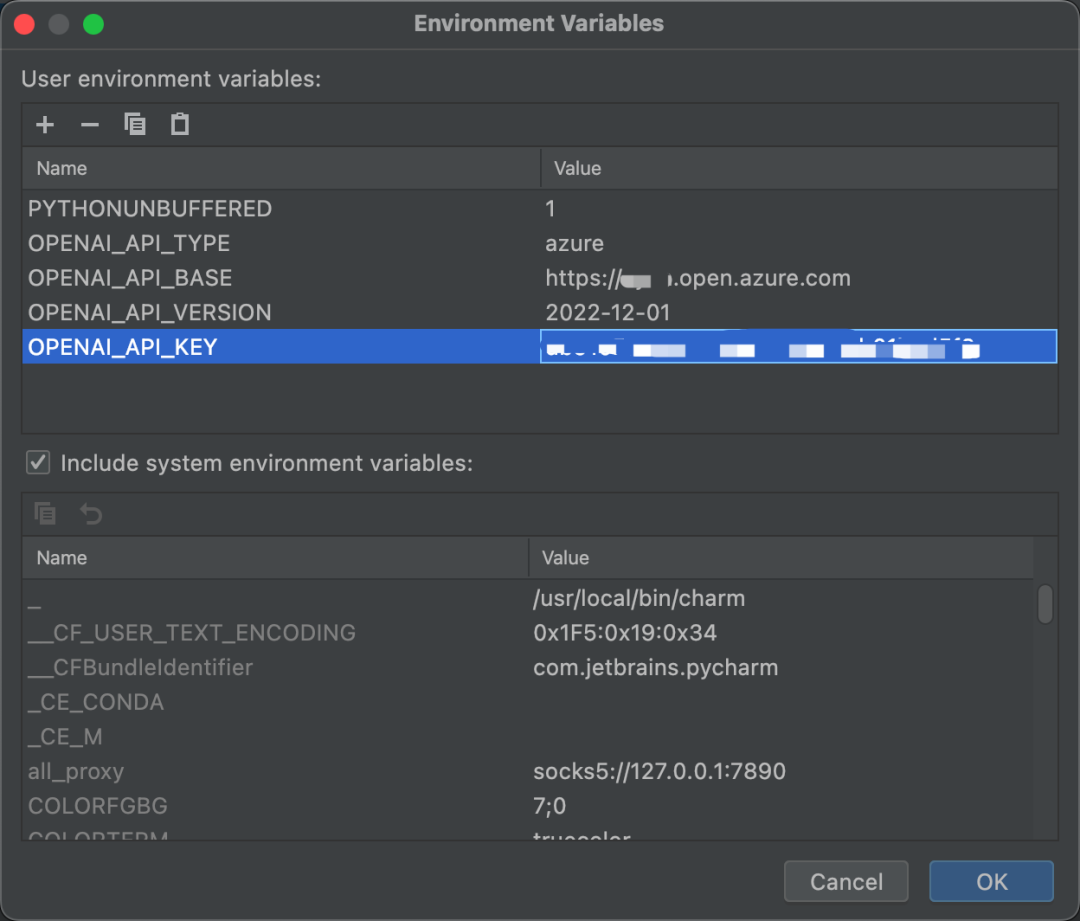
After completing the setup, running it again will still result in an error. This indicates that it automatically uses chromadb
as the vector database without giving me a choice.
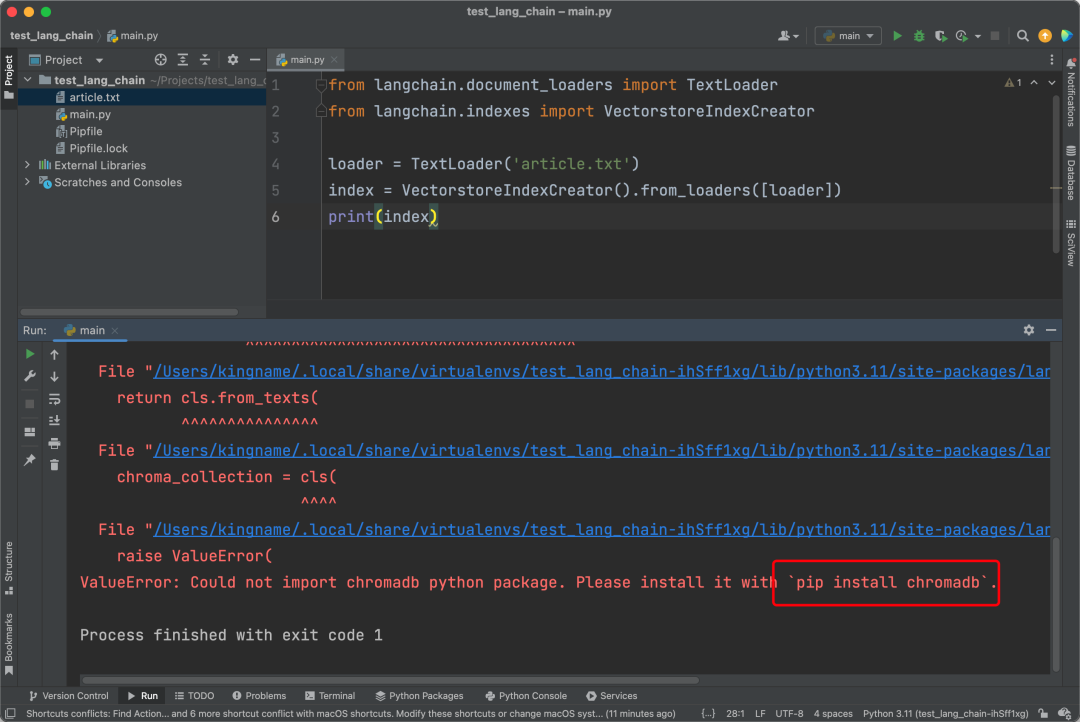
As required, I installed chromadb
. Running it again still resulted in an error: openai.error.InvalidRequestError: Resource not found
. This situation arises because the source code of LangChain reaches the position langchain.embeddings.openai.OpenAIEmbeddings._get_len_safe_embeddings
at the location shown in the image below:
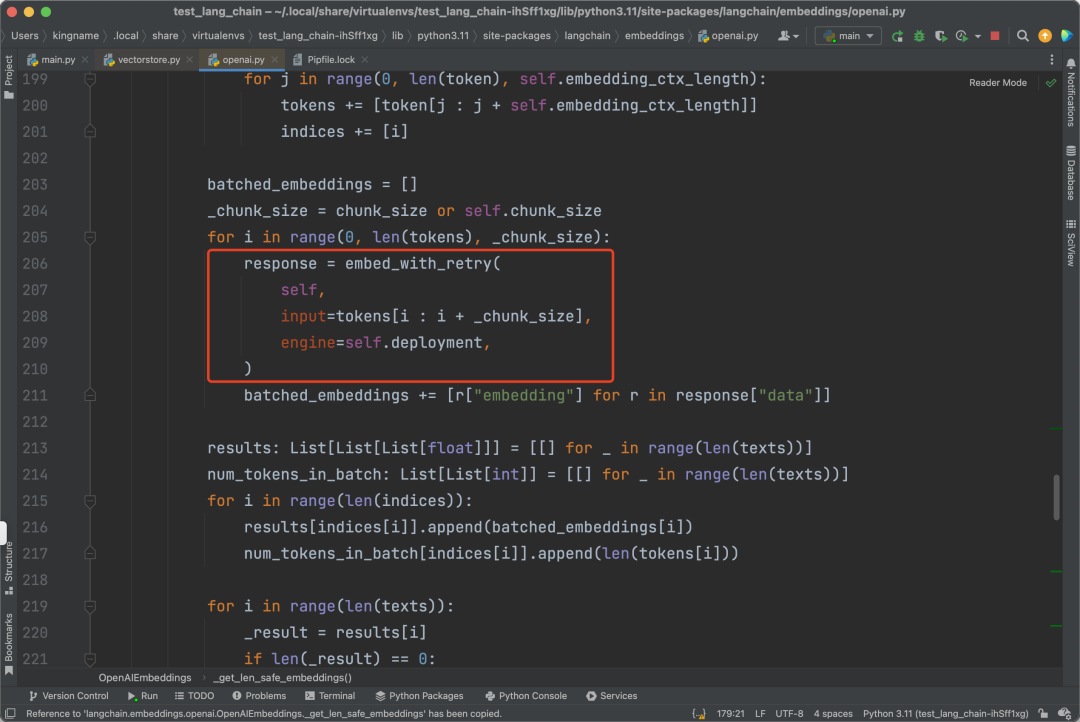
Parameters deployment
, api_type
, and api_version
should be passed in here, but they are missing. This causes the code to always request the URL using the official OpenAI interface, leading to a ‘not found’ error.
Even if you modify the source code to add these three parameters, you will still encounter issues, receiving the following error:
openai.error.InvalidRequestError: Too many inputs. The max number of inputs is 1. We hope to increase the number of inputs per request soon. Please contact us through an Azure support request at: https://go.microsoft.com/fwlink/?linkid=2213926 for further questions.
This is because the embedding model provided by Azure OpenAI allows only one concurrent request, while LangChain sends requests at a higher concurrency, resulting in this error.
Do not modify the source code further. Let’s return to the initial code:
index = VectorstoreIndexCreator().from_loaders([loader])
Let’s take a look at how the VectorstoreIndexCreator
class is implemented:
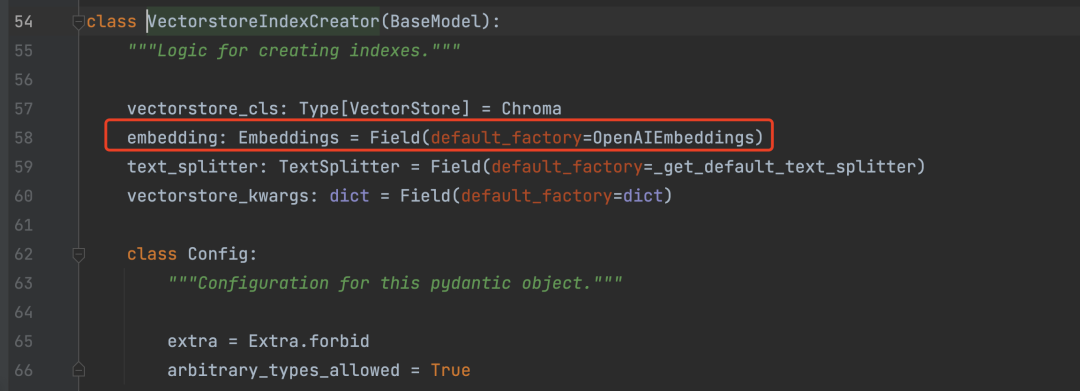
As you can see, this class inherits from pydantic.BaseModel
, which simplifies things. We can directly pass the embedding
parameter when initializing VectorstoreIndexCreator
. As shown in the image below:
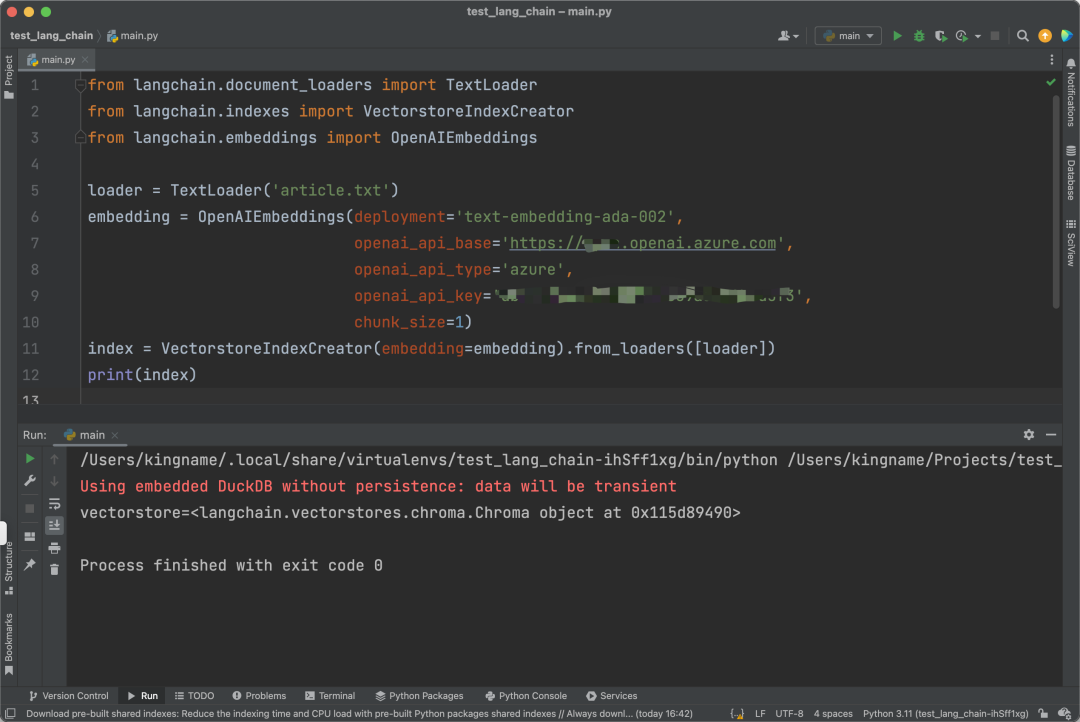
Now the code finally runs without errors. The chunk_size=1
in the code limits the concurrency to one. Let’s continue writing the code. The running effect is as shown in the image below:
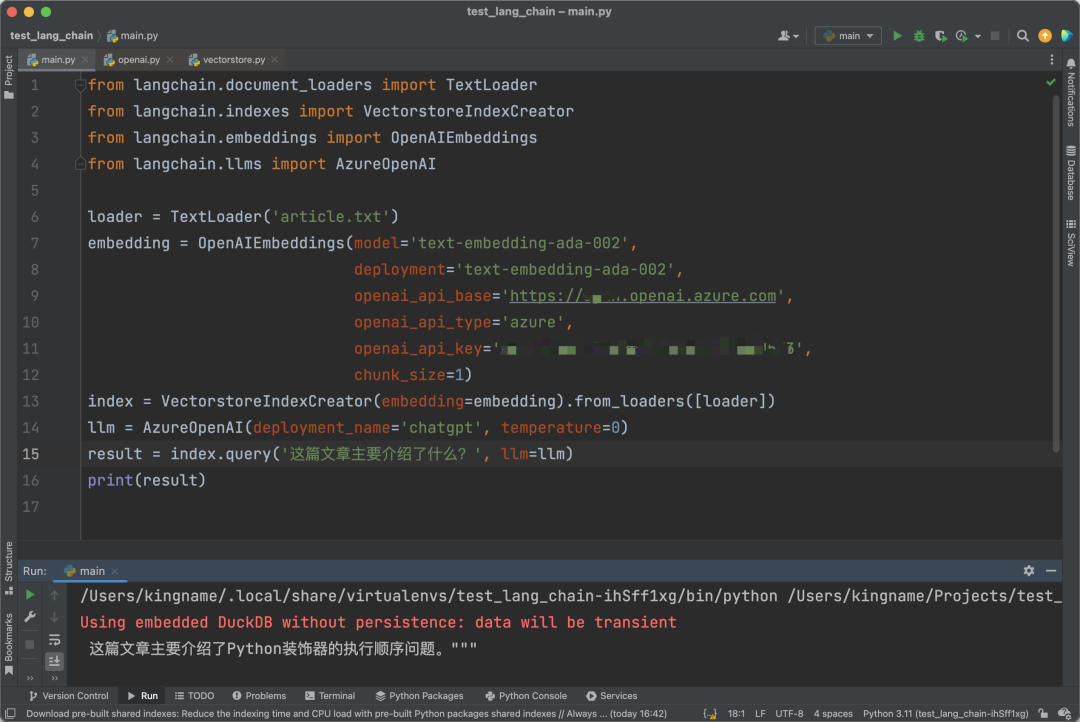
We can also actively pass parameters to use a different database instead of Chroma. Here, we use Redis as an example:
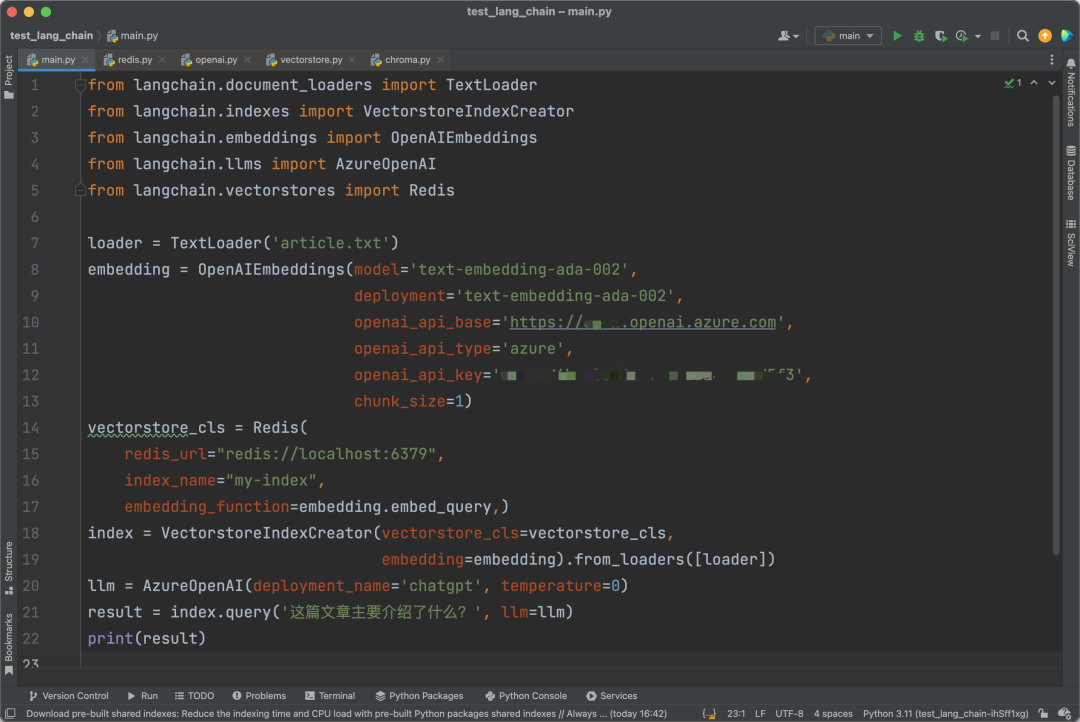
However, to use Redis as a vector database, you need to install the Redis Stack module in Redis. Installation methods can be found in the official Redis documentation[3].
LangChain: https://python.langchain.com/en/latest/index.html
[2]
Question Answering over Docs: https://python.langchain.com/en/latest/use_cases/question_answering.html
[3]
Official Redis Documentation: https://redis.io/docs/stack/