Click on the above “Beginner Learning Vision” to choose to add a Star or “Top”
Important content delivered promptly
-
Vision Transformer -
Main Ideas -
Operations -
Hybrid Architecture -
Loss of Structure -
Results -
Self-Supervised Learning via Masked -
Masked Autoencoding Vision Transformer -
Main Ideas -
Architecture -
Final Comments and Examples
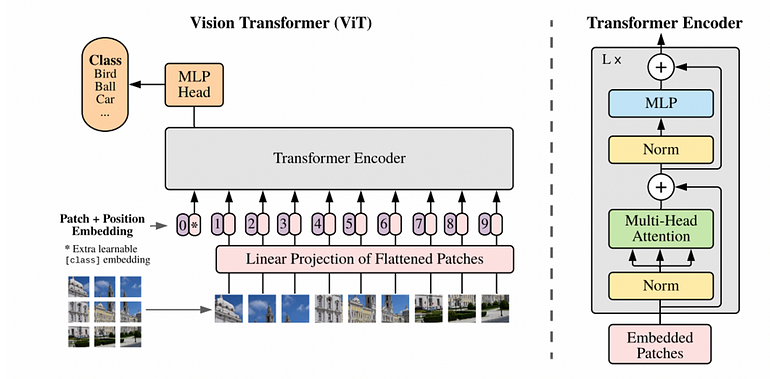
-
In NLP, the Transformer encoder takes a sequence of one-hot vectors (or equivalent token indices) representing input sentences/paragraphs and returns a sequence of context embedding vectors usable for further tasks (e.g., classification). -
To generalize to computer vision, the Vision Transformer takes a sequence of patch vectors representing the input image and returns a sequence of context embedding vectors usable for further tasks (e.g., classification).
-
Divide it into k² patches, where k is some value (e.g., k=3), as shown in the figure. -
Now each patch will be of size (n/k,n/k,3), and the next step is to flatten each patch into a vector.
-
These patch vectors are passed through a trainable embedding layer -
Add position embedding to each vector to preserve spatial information in the image -
The output is num_patches encoder representations (one for each patch), usable for classification at the patch or image level -
More commonly (as in the paper), a CLS token is added before the representations, corresponding to predictions for the entire image (similar to BERT)
The two-dimensional neighborhood structure is used very sparingly…position embeddings at initialization time carry no information about the 2D positions of the patches and all spatial relations between the patches have to be learned from scratch
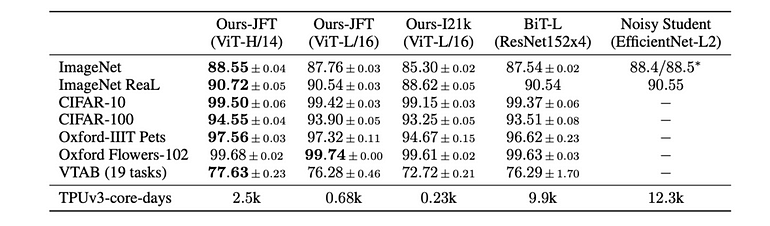
“We employ the masked patch prediction objective for preliminary self-supervision experiments. To do so, we corrupt 50% of patch embeddings by either replacing their embeddings with a learnable [mask] embedding (80%), a random other patch embedding (10%), or just keeping them as is (10%).”
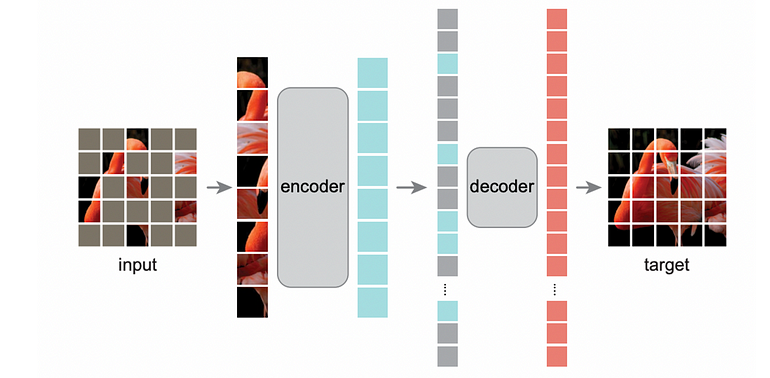
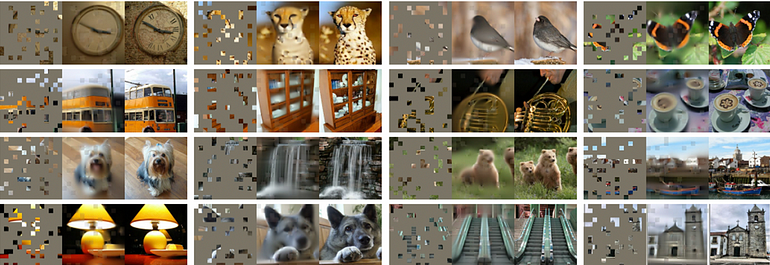
-
The masked token vector for missing patchs -
The encoder output vectors for known patchs
Download 1: OpenCV-Contrib Extension Module Chinese Version Tutorial
Reply "Extension Module Chinese Tutorial" in the backend of "Beginner Learning Vision" public account to download the first Chinese version of OpenCV extension module tutorial online, covering more than twenty chapters including extension module installation, SFM algorithm, stereo vision, object tracking, biological vision, super-resolution processing, etc.
Download 2: Python Vision Practical Project 52 Lectures
Reply "Python Vision Practical Project" in the backend of "Beginner Learning Vision" public account to download 31 vision practical projects including image segmentation, mask detection, lane line detection, vehicle counting, eyeliner addition, license plate recognition, character recognition, emotion detection, text content extraction, face recognition, etc., to help quickly learn computer vision.
Download 3: OpenCV Practical Project 20 Lectures
Reply "OpenCV Practical Project 20 Lectures" in the backend of "Beginner Learning Vision" public account to download 20 practical projects based on OpenCV for advanced OpenCV learning.
Discussion Group
Welcome to join the public account reader group to communicate with peers. Currently, there are WeChat groups for SLAM, 3D vision, sensors, autonomous driving, computational photography, detection, segmentation, recognition, medical imaging, GAN, algorithm competitions, etc. (will gradually be subdivided in the future). Please scan the WeChat number below to join the group, and note: "Nickname + School/Company + Research Direction", for example: "Zhang San + Shanghai Jiao Tong University + Vision SLAM". Please follow the format; otherwise, the request will not be approved. After successful addition, you will be invited to enter the relevant WeChat group based on research direction. Please do not send advertisements in the group; otherwise, you will be removed from the group. Thank you for your understanding~