When it comes to RAG or Agent, many people immediately think of LangChain or LlamaIndex, as they seem to believe these two are standard tools for developing applications with large models.
But for me, I particularly dislike these two. Because they are the typical representatives of over-encapsulation. Especially with the extensive use of dependency injection, it makes them very uncomfortable to use.
What is Dependency Injection
Suppose we want to simulate the sounds of various animals in Python, we can write it like this using dependency injection:
def make_sound(animal):
sound = animal.bark()
print(f'This animal goes {sound}')
class Duck:
def bark(self):
return 'quack'
class Dog:
def bark(self):
return 'woof'
class Cat:
def bark(self):
return 'meow'
small_cat = Cat()
make_sound(small_cat)
For the make_sound
function, you do not need to know how the bark
method of the animal object is implemented; you just need to call it and get its return value.
When you want to add a new animal, you only need to implement a class with a method called bark
. So when this animal needs to make a sound, you can just pass the animal instance to the make_sound
function.
It seems very convenient, right? Different animal classes do not affect each other, shielding the details.
Why I Dislike Dependency Injection
The above code looks good, following design patterns. If this code was written by you, it would indeed be very convenient. But if this code was written by someone else, and you do not know the details, then these dependency injections are a disaster. Let’s take a look at the code provided in the LlamaIndex documentation[1]:
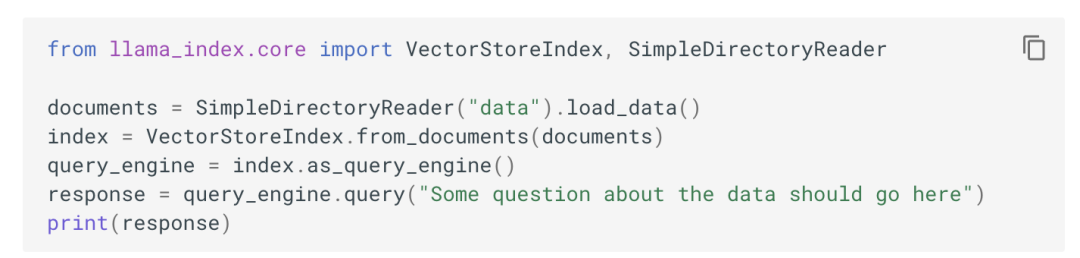
This code is a simplified version of RAG. It vectorizes text files and stores them in a vector database. After the user inputs a question, the program automatically queries the vector database for data. The code looks very clean, right? The logic of text-to-vector conversion is hidden, as is the logic for reading and writing to the vector database. Developers do not need to worry about these unimportant details; they can simply modify the documents in the data
folder to index the original documents. By modifying the parameters of query_engine.query
, they can implement a RAG. Developers focus on what is truly important, saving time and improving efficiency. It’s just perfect!
Perfect nonsense!
This kind of garbage code can only be used for a demo. When developers truly need to do secondary development, the above code is completely unusable.
Why is it unusable? Because I do not know how query_engine.query
queries the index
behind the scenes. I also do not know how VectorStoreIndex
operates when indexing documents. LlamaIndex seems to be smugly anticipating several questions users might ask in this documentation:
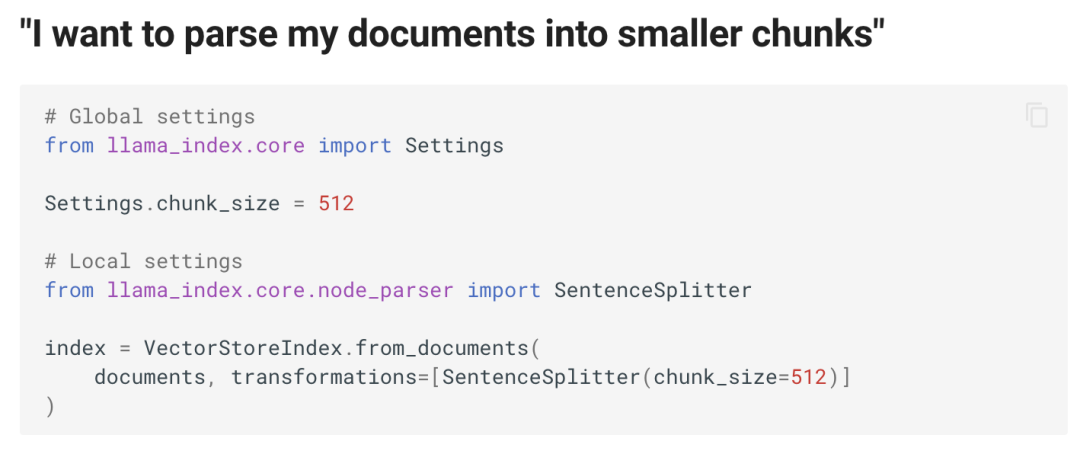
It thinks that when users want to split documents into different paragraphs, they can use SentenceSplitter
. Below are instructions on how to use other vector databases, query more documents, use different large models, and stream responses…
It looks like they have thought it through, right? They believe they have anticipated all potential user needs through different classes, methods, and parameters. Nonsense!
It is impossible to exhaustively list all user needs. For example:
-
I want the program to execute my own logic to filter out obviously problematic questions after querying multiple chunks from the vector database, and then perform ReRank -
After querying data from the vector database, I need to insert a few fixed chunks before asking the large model
These needs are completely unanticipated! And as a developer, I need them. But how should I insert them into its process?
In the above image, the instance of SentenceSplitter
is passed as a parameter to VectorStoreIndex.from_documents
. So if I have some specific requirements for the logic of splitting documents, how do I add that in? Should I write a class called MyCustomSentenceSplitter
? Now the question arises, what methods should this class have, and how should they be written? The from_documents
method calls which method? The reason the make_sound
function looks so simple is that I wrote it myself, and I know it will call animal.bark
. But now with LlamaIndex, it is written by someone else, and I do not even know how it will use SentenceSplitter
. Do I really have to read its syntax documentation or even look at its source code to implement a very simple logic for document tokenization? To implement the code I want, I would have to read through all its documentation and the source code before I can start working.
LangChain and LlamaIndex use a lot of dependency injection, drawing a box for developers and controlling all processes internally. Developers do not understand this process; they can only fill in the blanks in the code and get a barely working program.
But as a developer, what I need is to control this process, not to fill in the blanks.
Some might say, well, you can look at the source code of LlamaIndex to see how it queries the vector database and then write your own class to insert your code.
If someone thinks like this, I believe you are being abused and still thinking about how to lie down comfortably to make it less tiring for others to hit you.
What Do I Want
When developing applications with large models, what I need is to control the flow of the program. The simplifications I need are in the calling methods of each node in the process, not simplifying the process itself. I control the process; I know whether it should be simplified!
Let’s take a look at the new work by Requests author Kenneth Reitz: SimpleMind[2]. This is what I believe aligns with AI for Human
projects. Kenneth truly knows what users of this library need. Let’s look at how to use SimpleMind
:
Basic Usage
# First set the parameters for the large model through environment variables
import simplemind as sm
conv = sm.create_conversation()
conv.add_message("user", "Hi there, how are you?")
resp = conv.send()
Context Memory
class SimpleMemoryPlugin(sm.BasePlugin):
def __init__(self):
self.memories = [
"the earth has fictionally been destroyed.",
"the moon is made of cheese.",
]
def yield_memories(self):
return (m for m in self.memories)
def pre_send_hook(self, conversation: sm.Conversation):
for m in self.yield_memories():
conversation.add_message(role="system", text=m)
conversation = sm.create_conversation()
conversation.add_plugin(SimpleMemoryPlugin())
conversation.add_message(
role="user",
text="Please write a poem about the moon",
)
Tool Invocation
def get_weather(
location: Annotated[
str, Field(description="The city and state, e.g. San Francisco, CA")
],
unit: Annotated[
Literal["celsius", "fahrenheit"],
Field(
description="The unit of temperature, either 'celsius' or 'fahrenheit'"
),
] = "celsius",
):
"""
Get the current weather in a given location
"""
return f"42 {unit}"
# Add your function as a tool
conversation = sm.create_conversation()
conversation.add_message("user", "What's the weather in San Francisco?")
response = conversation.send(tools=[get_weather])
Control Flow
SimpleMind simplifies the invocation of the large model at this node. So if I can control the program’s logic myself, it would look like this for RAG:
def rag_ask(question):
question_embedding = text2embedding(question)
chunks = query_vector_db(question_embedding)
clean_chunks = my_logic_to_clean_chunks(chunks)
sorted_chunks = rerank(clean_chunks)
prompt = 'Use sorted_chunks and question to construct the RAG prompt'
answer = ask_llm(prompt)
In this code, I can implement the functions text2embedding
/query_vector_db
/rerank
/ask_llm
with just a few simple lines of code, and I can freely add my own logic between any two nodes in this process. This is what I want.
Conclusion
To be honest, seeing how LangChain is used, I feel that this thing is a patchwork created by people who write Java or C# trying to write Python. In the entire code, I see no Python coding philosophy; all I see is over-encapsulation, abstraction for the sake of abstraction. The authors of LangChain have clearly not considered the perspective of Python developers when designing its usage.
Code provided in the LlamaIndex documentation: https://docs.llamaindex.ai/en/stable/#getting-started
[2]
SimpleMind: https://github.com/kennethreitz/simplemind
END
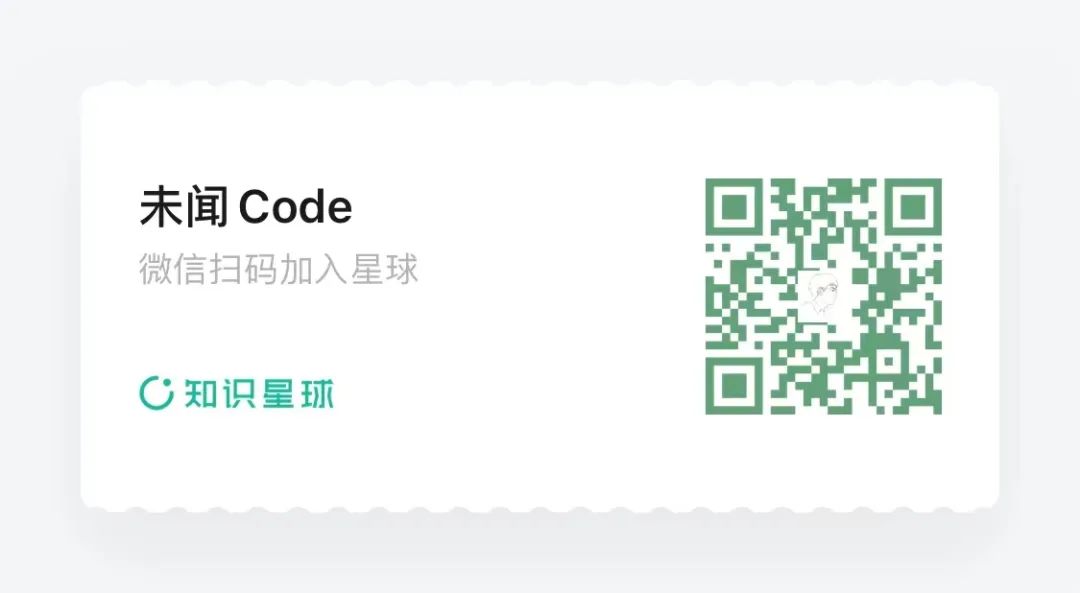
Unheard Code · Knowledge Planet is now open!
One-on-one Q&A on web scraping related issues
Career consulting
Interview experience sharing
Weekly live sharing
……
Unheard Code · Knowledge Planet looks forward to meeting you~