This article will introduce the LangChain framework and explain its functions with Python code examples, utilizing the OpenAI API, along with code and results. Interested readers can follow along with the code.
What is LangChain Framework
LangChain is a framework for building and deploying applications based on language models (such as large language models, LLMs). Imagine LangChain as a toolbox filled with various tools and guides to help you create and optimize language-related software projects more easily.
The practical functions of the LangChain framework mainly manifest in the following aspects:
1. Developing LLM Applications:
-
LangChain provides a set of tools and components that simplify the application development process based on large language models (LLMs).
-
Application Scenarios: Chatbots, virtual assistants, language translation tools, and sentiment analysis tools are all LLM-driven applications that can be created using LangChain.
2. Integrating Different Models and Data:
-
LangChain allows developers to connect different language models, data sources, and tools to create more powerful applications.
-
Application Scenarios: For instance, multiple LLMs can be combined, using the output of one as the input for the next, or integrating LLMs with external data sources for question-answering systems.
3. Providing Templates and Tools:
-
LangChain offers templates that allow developers to quickly start building specific applications, such as creating chatbots with custom data or extracting structured data from unstructured data.
-
Application Scenarios: Developers can use these templates to reduce development time and costs, rapidly deploying applications.
4. Debugging, Testing, and Monitoring:
-
LangSmith, as part of LangChain, allows developers to debug, test, evaluate, and monitor their LLM applications.
-
Application Scenarios: During development, developers can use LangSmith to optimize and deploy applications, ensuring application stability and performance.
5. Deploying as API:
-
LangServe is part of LangChain, which helps developers deploy their applications as REST APIs, making them widely accessible and interactive.
-
Application Scenarios: Developers can deploy their LLM applications as APIs for use by other developers or users.
6. Handling Documents and Data:
-
LangChain provides various tools for handling documents and data, such as document loaders, text splitters, and index/retrievers.
-
Application Scenarios: In scenarios requiring the processing of large volumes of documents or unstructured data, LangChain can assist in extracting, organizing, and retrieving information.
7. Building Complex Workflows:
-
LangChain allows developers to build workflows containing multiple steps, combining LLMs with other components, such as prompt templates and long-term memory.
-
Application Scenarios: In applications requiring complex logic and multi-step interactions, such as customer service automation, LangChain can provide robust support.
Key Modules
The key modules of the LangChain framework are as follows:
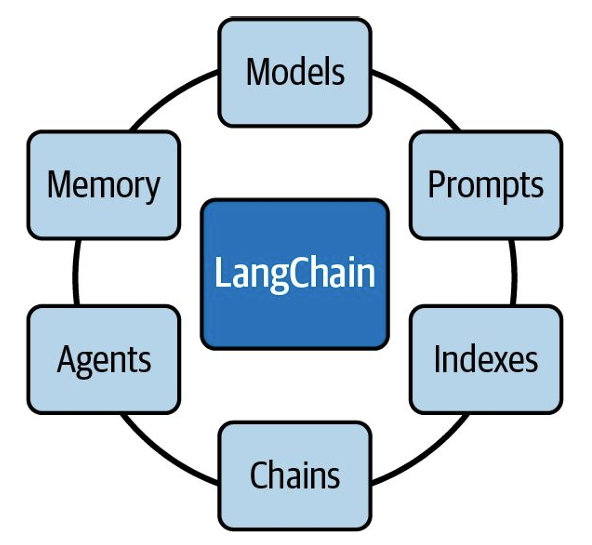
-
Models
-
This module is the standard interface provided by LangChain for interacting with various LLMs. LangChain supports integration with different types of models from providers like OpenAI, Hugging Face, Cohere, GPT4All, etc.
-
Prompts
-
Prompts have become the new standard in LLM programming. This module contains many tools for managing prompts.
-
Indexes or Retrieval Module
-
This module allows you to combine LLMs with your data.
-
Chains
-
This module provides the Chain interface. You can use this interface to create a sequence of calls, combining multiple models or prompts together.
-
Agents
-
This module introduces the Agent interface. An agent is a component that can process user input, make decisions, and select appropriate tools to complete tasks. It works iteratively, taking a series of actions until the problem is solved.
-
Memory
-
This module allows you to maintain state between chain calls or agent calls. By default, chains and agents are stateless, meaning they process each incoming request independently, just like LLMs.
LangChain is a universal interface for different LLMs, and you can refer to its documentation for more information. The LangChain documentation includes a list of integrations involving OpenAI and many other LLM providers. Most integrations require an API key to establish a connection. For OpenAI models, the API key can be set in the environment variable OPENAI_API_KEY.
Installing LangChain Framework
pip install langchain
Dynamic Prompts
from langchain.chat_models import ChatOpenAI
from langchain import PromptTemplate, LLMChain
template = """
Question: {question}
Let's think step by step.
Answer: """
prompt = PromptTemplate(template=template, input_variables=["question"])
llm = ChatOpenAI(model_name="gpt-4")
llm_chain = LLMChain(prompt=prompt, llm=llm)
question = """
What is the population of the capital of the country where the Olympic Games were held in 2016?
"""
llm_chain.run(question)
PromptTemplate is responsible for constructing the model’s input. That is, it can generate prompts in a reproducible way. It contains an input text string called template, where the values can be specified through input_variables. In this case, the prompt we defined will automatically add the “Let’s think step by step” part to the question.
The LLM used in this example is gpt-4. The default model currently is gpt-3.5-turbo. The ChatOpenAI function assigns the model’s name to the variable llm. This function assumes that the user has set the API key in the environment variable OPENAI_API_KEY.
Prompts and models are combined together by the LLMChain function, forming a chain that contains these two elements. Finally, we need to call the run function to request the completion of the input question. When the run function is executed, the LLMChain formats the prompt template using the provided input key (as well as any available memory key), and then passes the formatted string to the LLM, returning the LLM output. We can see that the model utilizes the “step-by-step thinking” technique to automatically answer the question.
Agents and Tools
Agents and tools are key features provided by the LangChain framework: they can make applications very powerful, enabling LLMs to perform various operations and integrate with various functionalities to solve complex problems.
The “tools” referred to here are specific abstractions around functions that make it easier for language models to interact with. Agents can use tools to interact with the world. Specifically, the tool’s interface has a text input and a text output. LangChain has many predefined tools, including Google Search, Wikipedia Search, Python REPL, calculators, World Weather Forecast APIs, and more. To get the complete list of tools, please check the tools page in the LangChain documentation. In addition to using predefined tools, you can also build custom tools and load them into the agent, making the agent very flexible and powerful.
This section introduces an agent applicable to applications that require a series of intermediate steps. This agent organizes the execution of these steps and can efficiently use various tools to respond to user queries. In a sense, because of “step-by-step thinking”, the agent has more time to plan actions, thus completing more complex tasks.
The steps organized by the agent are described as follows.
-
The agent receives input from the user.
-
The agent decides which tools to use (if any) and what text to input.
-
Using that input text, it calls the corresponding tool and receives output text from the tool.
-
The output text is input into the agent’s context.
-
Repeat steps 2 to 4 until the agent decides no longer to use tools. At this point, it will respond directly to the user.
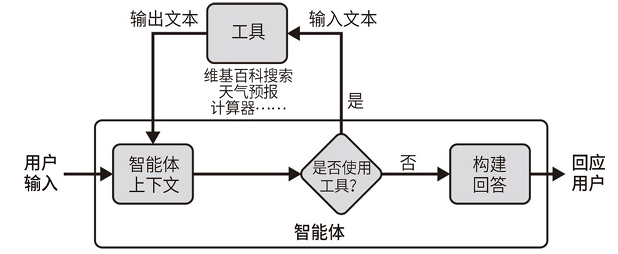
Benefits of Using Tools
In this section, we want the model to answer the following question: What is the square root of the population of the capital of the country where the Olympic Games were held in 2016? This question has no special meaning, but it nicely demonstrates how LangChain agents and tools can enhance the reasoning capabilities of LLMs.
If we throw the question directly to GPT-3.5 Turbo, we would get the following response:
The capital of the country where the Olympic Games were held in 2016 is Rio de Janeiro, Brazil. The population of Rio de Janeiro is approximately 6.32 million people as of 2021. Taking the square root of this population, we get approximately 2,513.29. Therefore, the square root of the population of the capital of the country where the Olympic Games were held in 2016 is approximately 2,513.29
This answer has at least two errors: Brazil’s capital is Brasília, not Rio de Janeiro; the square root of 6,320,000 is approximately 2,513.96, not 2,513.29. We could achieve better results by employing “step-by-step thinking” or other prompt engineering techniques, but due to the model’s difficulties with reasoning and mathematical operations, it is hard to trust the accuracy of the result. Using LangChain can provide us with better accuracy guarantees.
As shown in the following code, the LangChain agent can use two tools: Wikipedia Search and Calculator. After creating the tools through the load_tools function, we use the initialize_agent function to create the agent. The agent’s reasoning capabilities require an LLM, and in this example, we use gpt-3.5-turbo. The parameter ZERO_SHOT_REACT_DESCRIPTION defines how the agent selects tools at each step. By setting the value of verbose to True, we can view the agent’s reasoning process and understand how it makes its final decision.
from langchain.chat_models import ChatOpenAI
from langchain.agents import load_tools, initialize_agent, AgentType
llm = ChatOpenAI(model_name="gpt-3.5-turbo", temperature=0)
tools = load_tools(["wikipedia", "llm-math"], llm=llm)
agent = initialize_agent(
tools, llm, agent=AgentType.ZERO_SHOT_REACT_DESCRIPTION,
verbose=True
)
question = """
What is the square root of the population of the capital of the Country where the Olympic Games were held in 2016?"""
agent.run(question)
Before using the Wikipedia Search tool, you need to install the corresponding Python package wikipedia. You can install this package using pip install wikipedia
.
As you can see, the agent decides to query Wikipedia for information about the 2016 Olympic Games:
> Entering new chain... I need to find the country where the Olympic Games were held in 2016 and then find the population of its capital city. Then I can take the square root of that population. Action: Wikipedia Action Input: "2016 Summer Olympics" Observation: Page: 2016 Summer Olympics [...]
The next line of output contains an excerpt from Wikipedia about the Olympics. Next, the agent uses the Wikipedia Search tool to perform two additional operations:
Thought:I need to search for the capital city of Brazil. Action: Wikipedia Action Input: "Capital of Brazil" Observation: Page: Capitals of Brazil Summary: The current capital of Brazil, since its construction in 1960, is Brasilia. [...] Thought: I have found the capital city of Brazil, which is Brasilia. Now I need to find the population of Brasilia. Action: Wikipedia Action Input: "Population of Brasilia" Observation: Page: Brasilia [...]
Next, the agent uses the Calculator tool:
Thought: I have found the population of Brasilia, but I need to calculate the square root of that population. Action: Calculator Action Input: Square root of the population of Brasilia (population: found in previous observation) Observation: Answer: 1587.051038876822
Finally, it reaches the final answer:
Thought: I now know the final answer Final Answer: The square root of the population of the capital of the country where the Olympic Games were held in 2016 is approximately 1587. > Finished chain.
As you can see, the agent demonstrates strong reasoning capabilities: it completed four steps before arriving at the final answer. The LangChain framework allows developers to achieve this reasoning capability with just a few lines of code.
Memory
In some applications, remembering previous interactions is crucial, whether for short-term or long-term memory. Using LangChain, you can easily add state to chains and agents to manage memory. Building chatbots is the most common use case for this capability. In LangChain, you can quickly accomplish this process using ConversationChain, transforming a language model into a chat tool with just a few lines of code.
The following code creates a chatbot using the text-ada-001 model. This is a small model that can only perform basic tasks. However, it is the fastest and cheapest model in the GPT-3 series. This model has never been fine-tuned for chat tasks, but we can see that with just a few lines of LangChain code, we can start chatting using this simple text completion model:
from langchain import OpenAI, ConversationChain
chatbot_llm = OpenAI(model_name='text-ada-001')
chatbot = ConversationChain(llm=chatbot_llm , verbose=True)
chatbot.predict(input='Hello')
In the last line of the above code, we executed predict(input='Hello')
. This asks the chatbot to respond to our ‘Hello’ message. The model’s response is as follows:
> Entering new ConversationChain chain... Prompt after formatting: The following is a friendly conversation between a human and an AI. The AI is talkative and provides lots of specific details from its context. If the AI does not know the answer to a question, it truthfully says it does not know. Current conversation: Human: Hello AI: > Finished chain. ' Hello! How can I help you?'
Since we set verbose in the ConversationChain to True, we can see the complete prompt used by LangChain. When we execute predict(input='Hello')
, the text-ada-001 model receives not just the ‘Hello’ message but the complete prompt. This prompt is located between the tags > Entering new ConversationChain chain… and > Finished chain.
If we continue the conversation, we will find that the function retains the conversation history in the prompt. If we then ask the model if it is AI, that question will also be included in the prompt:
> Entering new ConversationChain chain... Prompt after formatting: The following [...] does not know. Current conversation: Human: Hello AI: Hello! How can I help you? Human: Can I ask you a question? Are you an AI? AI: > Finished chain. '
Yes, I am an AI.'
The ConversationChain object uses prompt engineering techniques and memory techniques to transform a text completion LLM into a chat tool.
Although LangChain gives all language models chat capabilities, this solution is not as powerful as GPT-3.5 Turbo and GPT-4, which have been specifically optimized for chat tasks. Additionally, OpenAI has announced the discontinuation of the text-ada-001 model.
Embeddings
Combining language models with your own text data helps personalize the model knowledge used by the application.
First, retrieve information by obtaining the user’s query and returning the most relevant documents; then send these documents to the model’s input context so it can respond to the query. This section demonstrates how simple it is to achieve this using LangChain and embedding techniques.
document_loaders is an important module in LangChain. Through this module, you can quickly load text data from various sources into your application. For instance, the application can load CSV files, emails, PowerPoint documents, Evernote notes, Facebook chat records, HTML pages, PDF files, and many other formats. To see the complete list of loaders, refer to the LangChain documentation. Each loader is very simple to set up.
If the PDF file is in the current working directory, the following code will load the content of the file and split it by page.
from langchain.document_loaders import PyPDFLoader
loader = PyPDFLoader("ExplorersGuide.pdf")
pages = loader.load_and_split()
Before using the PDF loader, you need to install the pypdf package. This can be done with pip install pypdf
.
When retrieving information, each loaded page needs to be embedded. As discussed in Chapter 2, in information retrieval, embeddings are a technique used to convert non-numeric concepts (such as words, tokens, and sentences) into numerical vectors. These embeddings enable the model to efficiently handle the relationships between these concepts. With OpenAI’s embedding endpoint, developers can obtain numerical vector representations of input text. Additionally, LangChain provides a wrapper to call these embeddings, as shown below.
from langchain.embeddings import OpenAIEmbeddings
embeddings = OpenAIEmbeddings()
To use OpenAIEmbeddings, you first need to install the tiktoken package using pip install tiktoken
.
Indexes save page embeddings and make searching easy. LangChain is centered around vector databases. There are many vector databases available, see the LangChain documentation for details. The following code snippet uses the Faiss vector database, a similarity search library primarily developed by the Facebook AI team.
from langchain.vectorstores import FAISS
db = FAISS.from_documents(pages, embeddings)
Before using the Faiss vector database, you need to install the faiss-cpu package using pip install faiss-cpu
.
The following image shows how the content of the PDF file is converted into embedding vectors and stored in the Faiss vector database.
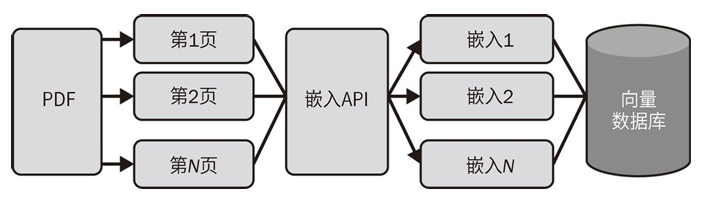
Now it is easy to search for similar content:
q = "What is Link's traditional outfit color?"
db.similarity_search(q)[0]
We get the following content:
Document(page_content='While Link’s traditional green tunic is certainly an iconic look, his wardrobe has expanded [...] Dress for Success', metadata={'source': 'ExplorersGuide.pdf', 'page': 35})
The answer to this question is that Link’s outfit color is green. We can see that the answer is within the selected content. The output indicates that the answer is on page 35 of ExplorersGuide.pdf. Remember, Python counts from 0, so if you check the original PDF file, you will find the answer on page 36, not page 35.
The following image shows how the information retrieval process uses the query’s embeddings and the vector database to identify the pages most similar to the query.
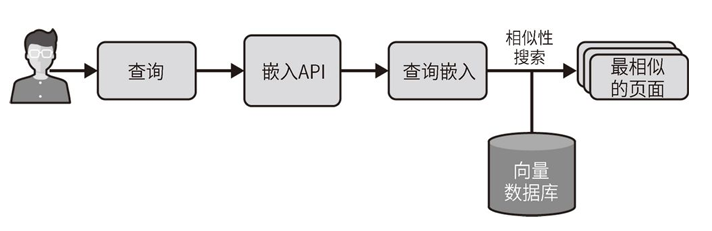
You might want to integrate embeddings into chatbots to use the retrieved information when answering questions. Again, using LangChain, this can be easily achieved with just a few lines of code. We use RetrievalQA, which takes LLM and vector databases as inputs. Then, we can ask the obtained object as usual:
from langchain.chains import RetrievalQA
from langchain import OpenAI
llm = OpenAI()
chain = RetrievalQA.from_llm(llm=llm, retriever=db.as_retriever())
q = "What is Link's traditional outfit color?"
chain(q, return_only_outputs=True)
We get the following answer:
{'result': " Link's traditional outfit color is green."}
The following image illustrates how RetrievalQA uses information retrieval to answer user questions. As we can see from the image, “Providing Context” groups the pages found by the information retrieval system with the user’s initial query. Then, the context is sent to the LLM. The LLM can utilize the additional information in the context to accurately answer the user’s question.
You might ask: why is it necessary to perform information retrieval before adding information to the LLM’s context? In fact, existing language models cannot handle large documents containing hundreds of pages. Therefore, if the input document is too large, we will pre-filter it. That is the task of the information retrieval process. We can compare the RAG flowchart, perhaps we can find some connections.
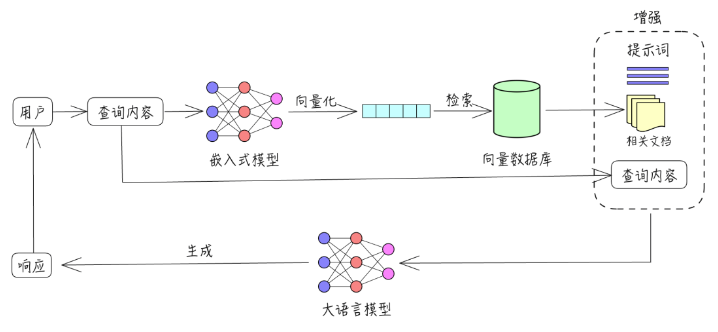
In the near future, as the input context continues to increase, it may no longer be necessary to use information retrieval techniques.
Recruitment Requirements
Complete qualified robot-related video production
Total duration needs to reach over 3 hours
Video content must be high-quality courses, ensuring quality and professionalism
Instructor Rewards
Enjoy course revenue sharing
Gift 2 courses from GuYue Academy’s premium courses (excluding training camps)
Contact Us
Add staff WeChat: GYH-xiaogu