Part.1
OpenAI o1: The AI Model Beyond Human PhDs
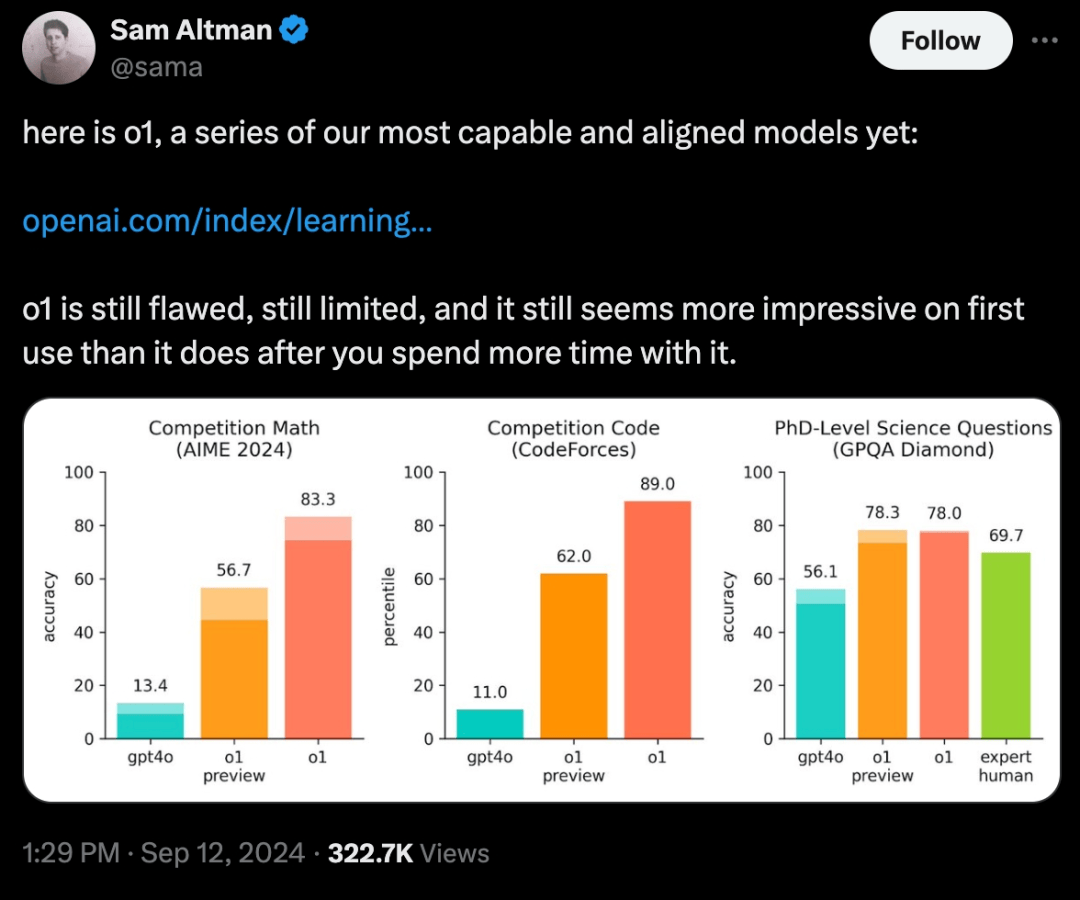
What Unique Techniques Did OpenAI Use in the o1 Model?
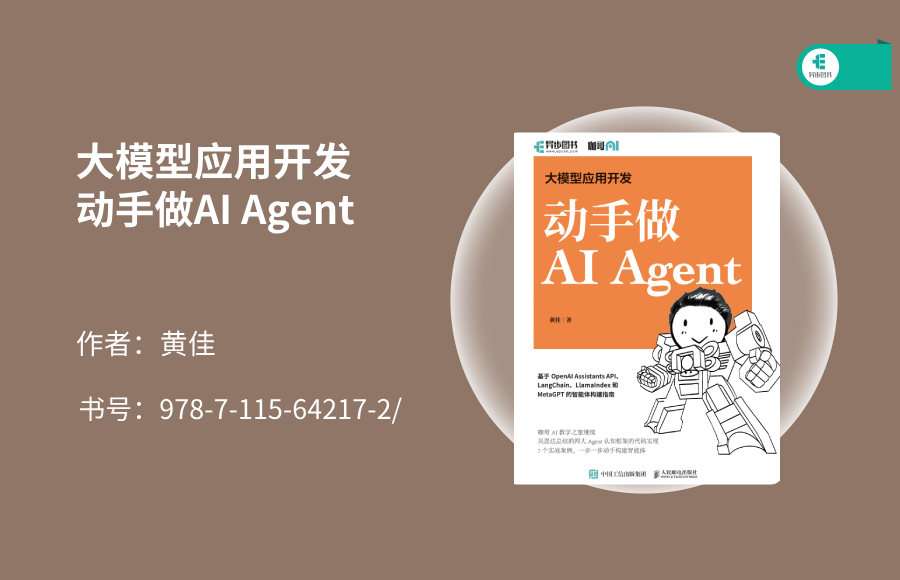
Part.2
The Secrets to Effectively Utilizing Powerful Models
1. Knowledgeable: Agents need to be trained on vast amounts of data to acquire extensive knowledge and skills.
2. Inquiry: Agents should receive clear and precise instructions, i.e., effective prompt engineering, to ensure correct understanding of task requirements.
3. Thoughtful: Agents should engage in cognition under carefully designed patterns, configuring Chain of Thought, Tree of Thought (ToT), ReAct framework, etc.
4. Discerning: Agents need to explicitly follow human ethical standards, ensuring AI safety and harmlessness through instruction fine-tuning and value alignment.
5. Diligent: Agents need to interact with the external world using technical tools (such as ToolCalls and Function Calling) to perform specific actions.
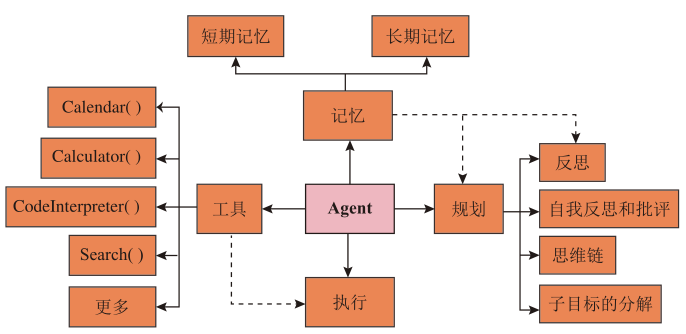
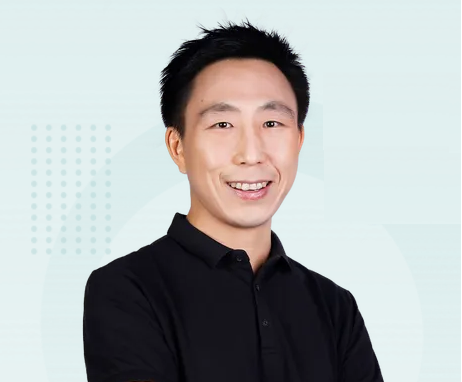
Part.3
Seven Examples to Master AI Agents
· Agent 1: Achieving Office Automation
Creating PPT with Assistants API and DALL·E 3 model. This project demonstrates how to utilize OpenAI’s API to achieve automated office tasks, such as creating presentations.
· Agent 2: Multifunctional Selection Engine
Calling functions through Function Calling. This Agent project explores how to implement Function Calling via Assistants API and Tool Calls via ChatCompletion API.
· Agent 3: Synergy of Reasoning and Action
This example demonstrates how to use LangChain’s ReAct framework to implement an automated pricing system.
· Agent 4: Decoupling Planning and Execution
Intelligent inventory scheduling through Plan-and-Execute in LangChain. It first introduces the Plan-and-Solve strategy, then implements logistics management through the Plan-and-Execute Agent.
· Agent 5: Knowledge Extraction and Integration
This Agent project utilizes LlamaIndex’s ReAct RAG Agent to implement flower language secret report retrieval, showcasing the capabilities of retrieval-augmented generation (RAG) through LlamaIndex.
· Agent 6: GitHub’s Influencer Community
This project introduces several popular AI Agent projects on GitHub, including AutoGPT, BabyAGI, and CAMEL, which have received widespread attention and discussion in the community.
· Agent 7: Multi-Agent Framework
This project explores the concept and implementation of multi-Agent frameworks, including the use of tools like AutoGen and MetaGPT.
1
“GPT Illustrated: How Large Models Are Built”
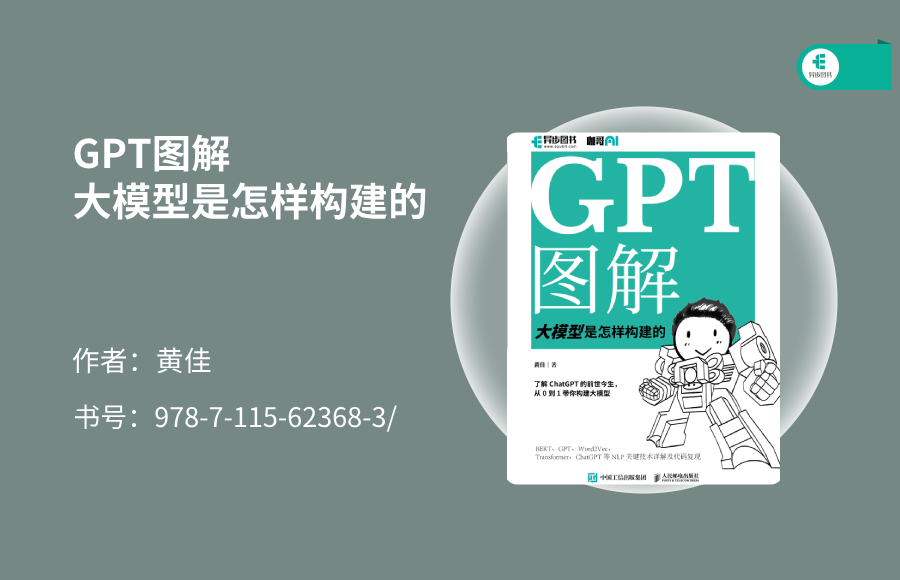
This is also a masterpiece by Brother Ka, maintaining his lively writing style, leading readers through the technical space with light-hearted stories and colorful illustrations, thoroughly understanding the core ideas of GPT technology, and building language models from scratch.
2
“Hands-on Reinforcement Learning”
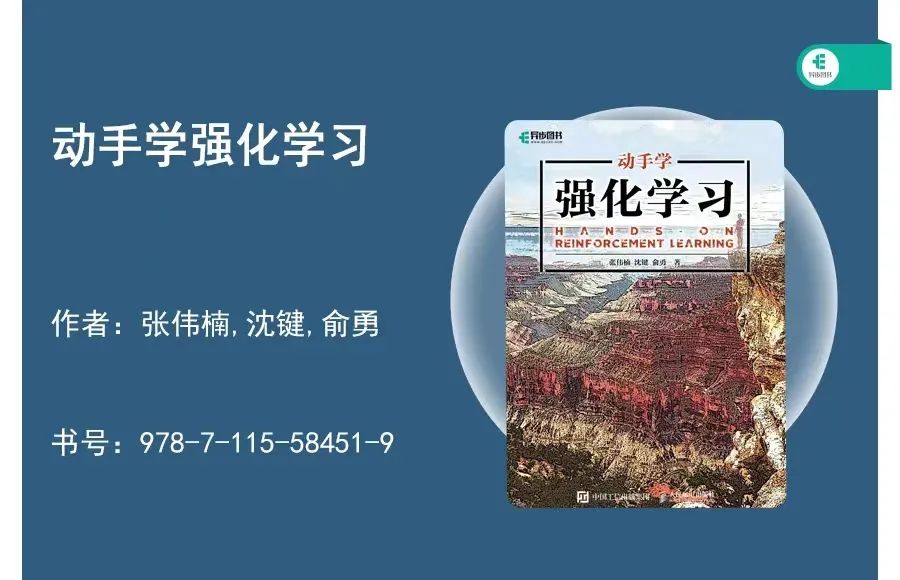
This is a classic introductory book on reinforcement learning launched by Professor Yu Yong’s team from Shanghai Jiao Tong University ACM class. The book comprehensively and systematically introduces the basic techniques of reinforcement learning, helping readers learn the basic concepts and representative methods of reinforcement learning, and covers cutting-edge techniques such as imitation learning and multi-agent reinforcement learning.
The book also provides executable code for each algorithm, helping readers quickly get started and build a theoretical and engineering system for reinforcement learning from scratch.
3
“Easy RL: Reinforcement Learning Tutorial”
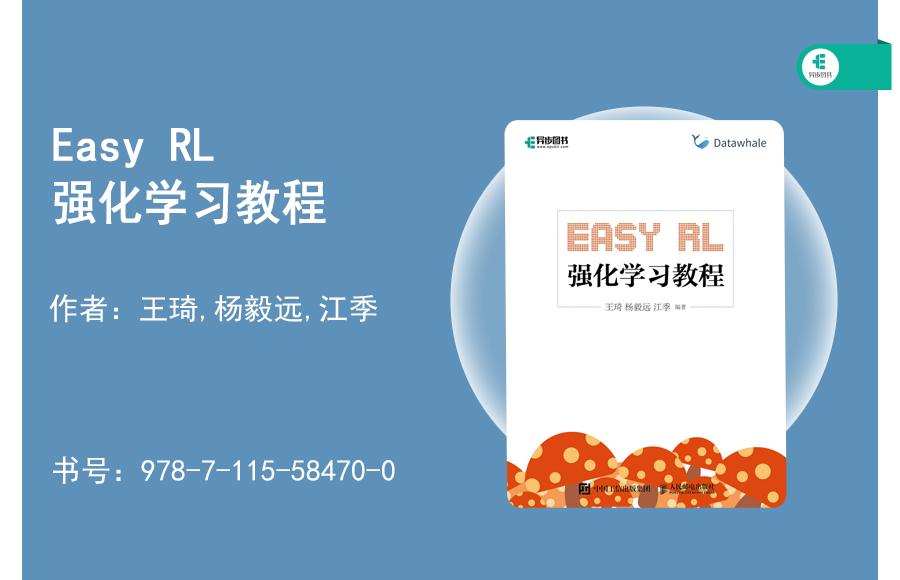
This book was created by the Datawhale technical team, incorporating the essence of Professor Li Hongyi’s “Deep Reinforcement Learning,” Professor Zhou Bolei’s “Reinforcement Learning Outline,” and Professor Li Kejiao’s “World Champion Takes You to Practice Reinforcement Learning from Scratch” open courses, introducing reinforcement learning knowledge in an accessible manner.
Main knowledge points include Markov decision processes, Monte Carlo methods, temporal difference methods, Sarsa, Q-learning, and traditional reinforcement learning algorithms, as well as policy gradients, proximal policy optimization, deep Q networks, and deep deterministic policy gradients.
The book also provides comprehensive exercise solutions and Python code implementations, helping readers fully grasp the principles of reinforcement learning algorithms and be able to practice.
4
“Everyone is a Prompt Engineer”
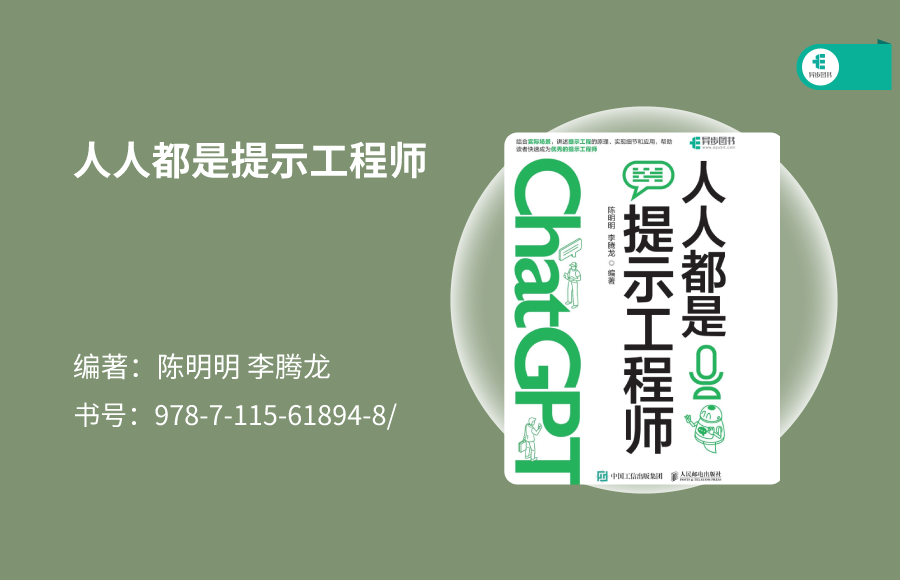
This book discusses the basic working principles of prompt technology, commonly used tools for prompt engineers, foundational patterns of prompt technology, and advanced knowledge of prompt technology, including zero-shot prompts, few-shot prompts, and chain-of-thought prompts.
It also explains the basics of NLP and the principles of the ChatGPT large model, as well as the characteristics and application scenarios of NLP models. It showcases the applications of prompt engineering in office tasks, image processing, code development, and e-commerce.
Part.4
Conclusion
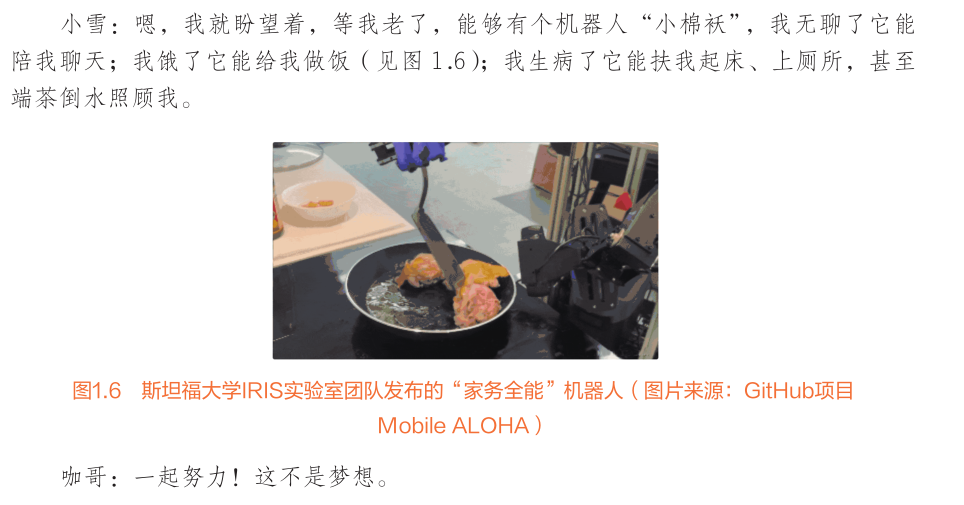
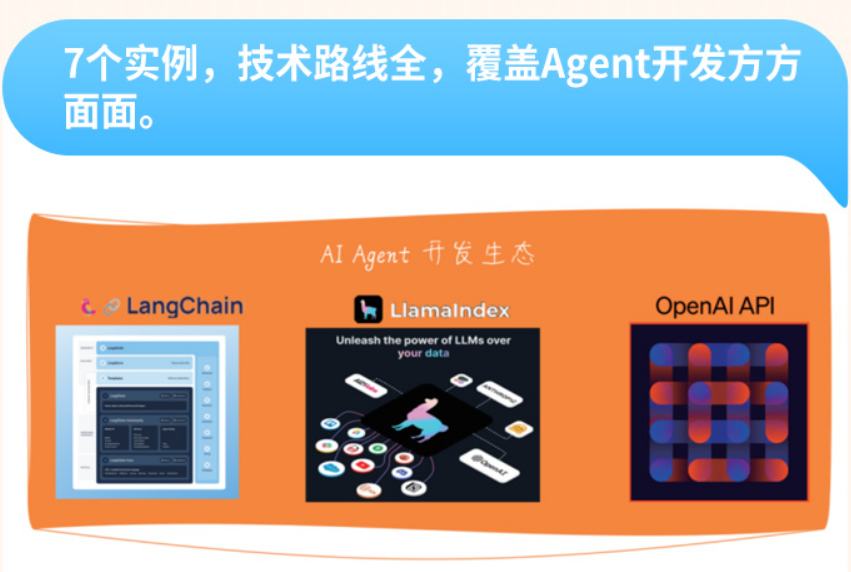
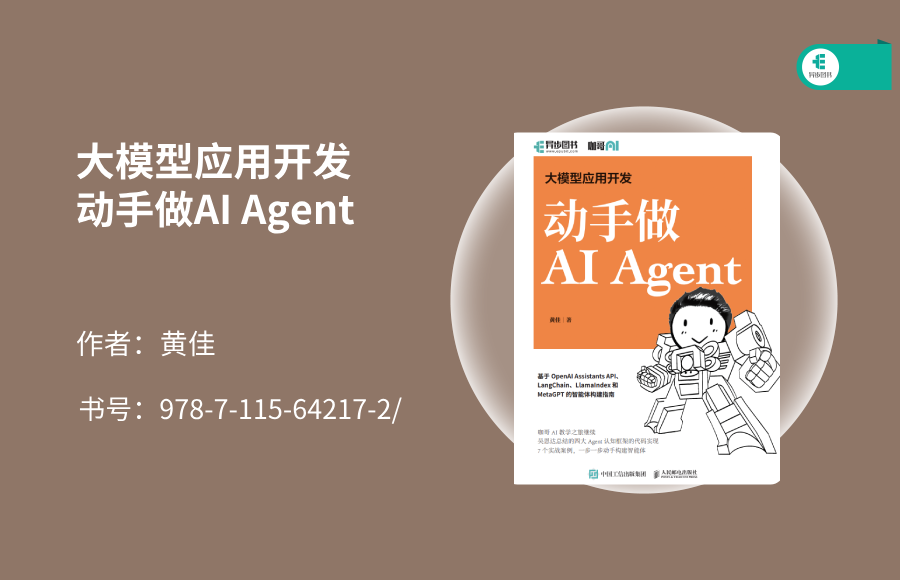

Share Your Thoughts on RAG
Participate in the interaction in the comment area, and click “Read” and share the activity to your friend circle. We will select 1 reader to receive 1 e-book version for free, deadline October 31.