Introduction:
This article aims to explore an innovative path by utilizing Google’s Gemini Flash instead of the widely known LangChain to achieve intelligent interaction with CSV files. We will build a simple CSV interpreter that generates code and parses data without LangChain, creating an intuitive user interface with Streamlit.©️【Deep Blue AI】
In today’s rapidly evolving AI tool landscape, tools like LangChain (LLM programming framework), LangGraph, LlamaIndex, and CrewAI are emerging one after another. Although each of these tools has its strengths, the learning curve can often be steep for developers, especially when they genuinely want to build projects rather than just follow trends in learning these libraries. While LangChain is powerful, its complex documentation can confuse users. For simple projects, LangChain may not be the first choice, and it is particularly important to adhere to the KISS (Keep it simple stupid) principle.
Although these tools may not be as difficult to handle as described, it is recommended to first try implementing some basic functions before transitioning to more advanced tools based on needs.
Since November 2022, the AI field has been evolving rapidly, especially with the continuous updates of GPT-4o and Gemini.
The “Sky” voice of GPT-4o has generated a huge response during demonstrations, sparking a new wave in the AI industry and even overshadowing the brilliance of Gemini at the Google I/O conference to some extent. Indeed, GPT has always been at the forefront of other LLMs, but for building minimum viable products (MVPs) and personal projects, Gemini brings good news to developers, allowing access to APIs and the latest models at its free tier, with a request limit of 15 times per minute, which is quite friendly.
Although the free version of ChatGPT allows users to upload files, it still cannot directly parse CSV or Excel data without a code interpreter. Therefore, to test the capabilities of Gemini Flash, this project constructs a CSV interpreter. There are many tutorials on the market that use LangChain to create CSV interpreters, but this project will build it from scratch in a more understandable way, especially suitable for beginners.
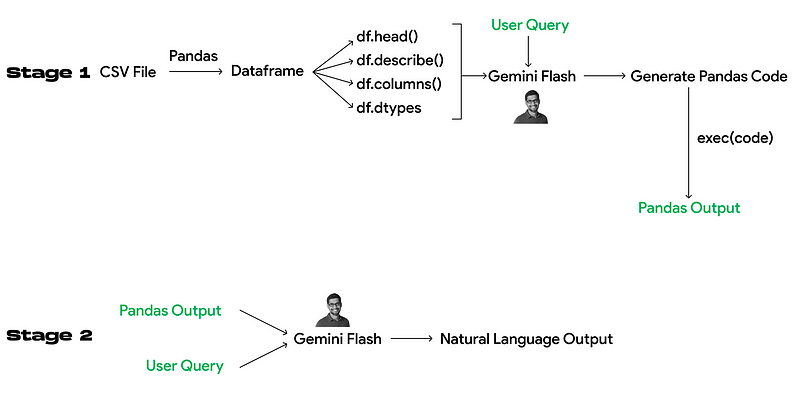
The core of this project is to leverage the capabilities of LLM to automatically generate code. First, provide key information about the dataset to the LLM, such as basic descriptions obtained through methods like head(), describe(), columns(), and dtypes, to serve as the basis for code generation. Then, the generated code will be combined with the user’s specific queries, aiming to enhance the interaction experience between the user and the system, making the results more aligned with the user’s actual needs.
This project is divided into two major phases:
●Code Generation Phase:This phase focuses on utilizing the generative capabilities of LLM to automatically generate corresponding pandas commands or code snippets based on the metadata information of the dataset.
●Result Integration and Dialogue Optimization Phase:After code generation, the outputs of these codes will be closely integrated with the user’s specific queries, improving the dialogue experience between the user and the system by transforming code outputs into natural language, making the results more intuitive and understandable.
When designing prompts, the following points need to be noted:
●Clear and Precise: Ensure that the instructions or questions in the prompt are clearly and accurately stated to avoid ambiguity. ●Concise and Direct: Try to convey core information using concise language, avoiding lengthy and complex descriptions. ●Avoid Instruction Overload: Do not pile too many instructions or questions into the same prompt, as it may lead to the LLM being unable to understand or execute them.
At the same time, techniques such as zero-shot, chain-of-thought, few-shot prompts, and role-based prompt design methods can be referenced to better guide the LLM and improve the quality and accuracy of the generated code.
A total of four prompts are needed: two system prompts and two main prompts (one system prompt + one main prompt for each phase). The system prompts serve as a framework to create conditions for the AI to operate within specific parameter ranges and generate coherent, relevant, and expected results.
By dynamically injecting metadata into this prompt, we can generate pandas commands.
Data Frame Metadata:
df = pd.read_csv(uploaded_file)
head = str(df.head().to_dict())
desc = str(df.describe().to_dict())
cols = str(df.columns.to_list())
dtype = str(df.dtypes.to_dict())
System Prompt:
model_pandas = genai.GenerativeModel('gemini-1.5-flash-latest', system_instruction="You are an expert python developer who works with pandas. You make sure to generate simple pandas 'command' for the user queries in JSON format. No need to add 'print' function. Analyse the datatypes of the columns before generating the command. If unfeasible, return 'None'. ")
model_response = genai.GenerativeModel('gemini-1.5-flash-latest', system_instruction="Your task is to comprehend. You must analyse the user query and response data to generate a response data in natural language.")
Main Prompt:
final_query = f"The dataframe name is 'df'. df has the columns {cols} and their datatypes are {dtype}. df is in the following format: {desc}. The head of df is: {head}. You cannot use df.info() or any command that cannot be printed. Write a pandas command for this query on the dataframe df: {user_query}"
natural_response = f"The user query is {final_query}. The output of the command is {str(data)}. If the data is 'None', you can say 'Please ask a query to get started'. Do not mention the command used. Generate a response in natural language for the output."
Response Generation:
# Stage 1
response = model_pandas.generate_content(
final_query,
generation_config=genai.GenerationConfig(
response_mime_type="application/json",
response_schema=Command,
temperature=0.3
)
)
# Stage 2
bot_response = model_response.generate_content(
natural_response,
generation_config=genai.GenerationConfig(temperature=0.7)
)
←Swipe left and right to view the complete code→
The temperature parameter controls the randomness of the response. A high temperature increases creativity and diversity but may deviate from the query; a low temperature ensures consistent and focused responses but with less creativity. Therefore, the project uses a low temperature in the first stage to ensure accurate commands, and a high temperature in the second stage to avoid monotony.
When executing pandas commands, the exec() function of Python is used. Although there are security risks, it is a necessary tool for achieving dynamic code execution. Future plans include optimizing the architecture and increasing validation to improve security.
User Interface Construction Reference:https://omkamath.medium.com/how-i-built-a-beautiful-web-app-purely-in-python-with-zero-experience-874731df6bc1
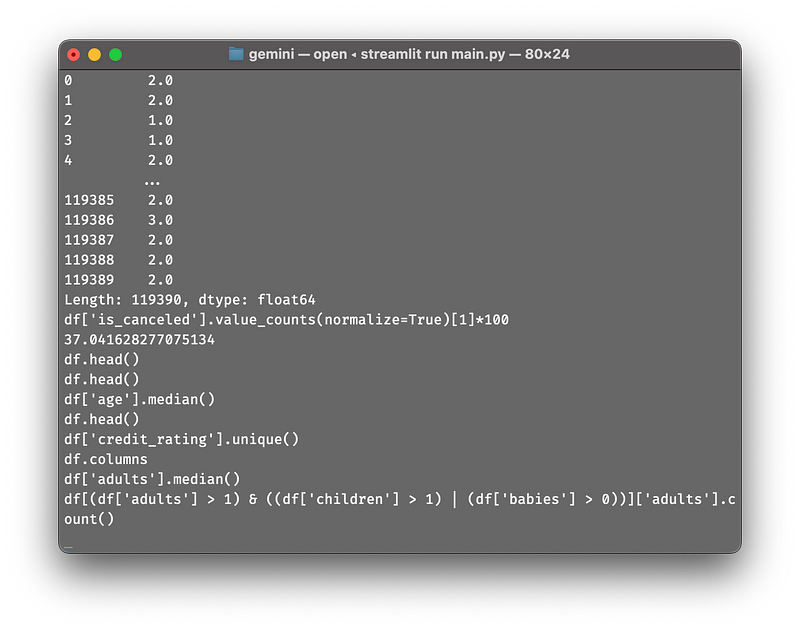
Through carefully designed prompts, LLM can easily transition and handle various tasks, which is undoubtedly the core driving force for future project innovation. Indeed, this project does not pursue groundbreaking technological innovation; its architecture aligns with the code interpreter concept in ChatGPT, showcasing high flexibility and universality. In my view, this highly abstract design philosophy is particularly suitable for beginners, opening a door to a vast technical world for them.
*For all the code related to Streamlit UI, please check the gist.
©️【Deep Blue AI】
References:
https://gist.github.com/Om-Kamath/90f1ae351bea470e72d0e6a567527eea
https://medium.com/google-cloud/did-google-just-kill-streamlit-76f719d9e275
https://levelup.gitconnected.com/chat-with-csv-files-using-googles-gemini-flash-no-langchain-0e8f79d63348
https://omkamath.medium.com/how-i-built-a-beautiful-web-app-purely-in-python-with-zero-experience-874731df6bc1
Written by|Sienna
Reviewed by|Los
Deep Blue Academy “Long-term Exchange Group” is under construction… including 14 subfields such as intelligent driving, robotics, vision, drones, and large models. The latest industry news, laboratory dynamics, latest papers, job opportunities… everything you need is in the group.
In September, we will also launch Universal Knowledge Planet 🌍: Daily sharing of industry, enterprise, laboratory dynamics, latest papers, and columns on cutting-edge reports, datasets/open-source projects, interview recruitment, AI intelligent driving corporate news, etc.(Internal group friends enjoy the first 200 members at 【26 yuan/year】, averaging 50 cents per week.)
👇👇 Scan the QR code in the image below to add the consultant and seize the opportunity 👇👇

Original content of 【Deep Blue AI】 is created with the personal effort of the author team. We hope everyone adheres to the original rules and cherishes the authors’ hard work. For reprints, please contact the backstage for authorization, and be sure to indicate that it comes from【Deep Blue AI】 WeChat official account, otherwise, legal action will be taken ⚠️⚠️
*Click like + view and save this article*