Due to the new feature launched by the WeChat public account platform, the name displayed on the left of “Language Resource Bulletin” is not the original author, but the account manager. The original author will be displayed in the main title and at the end of the article. Thank you for your understanding!
How Much Do You Know About Voice Recognition
Before introducing voice recognition, let me briefly explain what voice technology is.
In fact, most laymen’s understanding of voice technology is limited to voice recognition. In reality, voice technology encompasses many aspects, as shown in the figure below.
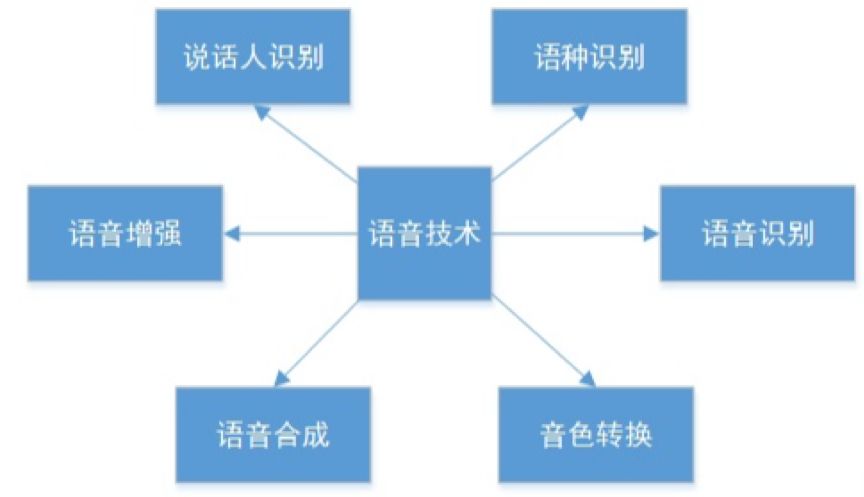
(Image source: Zhihu)
This means that in the field of voice technology, there are many directions: speaker recognition, language recognition, voice enhancement, voice synthesis, voice conversion, and voice recognition.
In real life, apart from voice recognition, people are also familiar with voice synthesis (such as some apps that can add background music to spoken content) and language recognition. As the name suggests, language recognition allows your machine to accurately identify any language you speak.
For example, when asking Siri.
Chinese
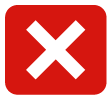
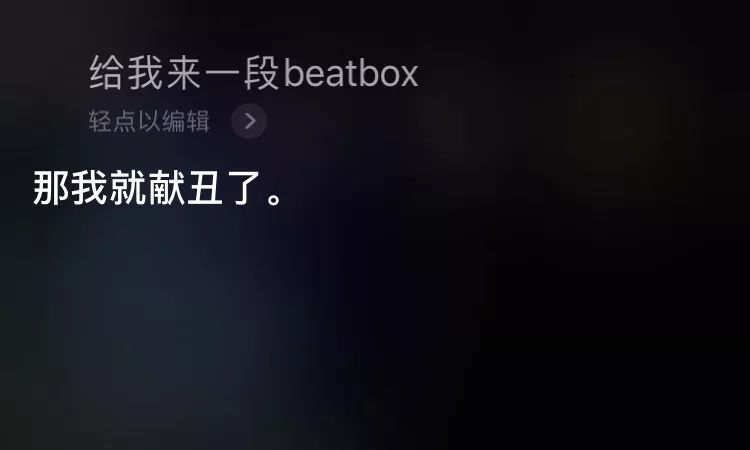
Cantonese
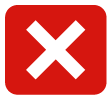
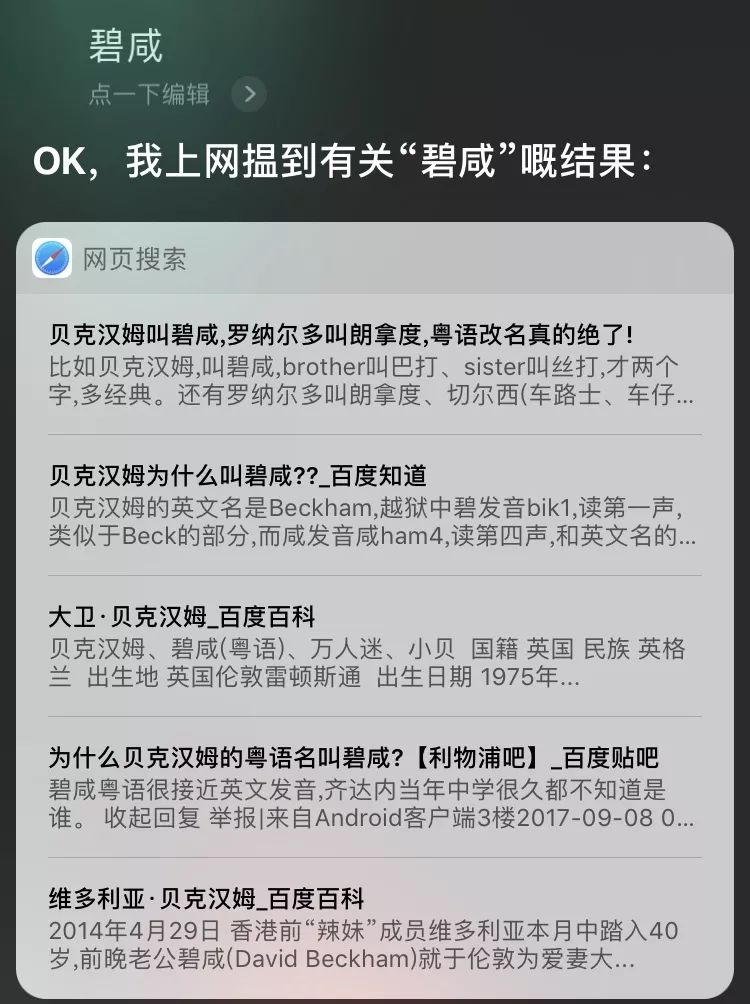
English
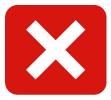
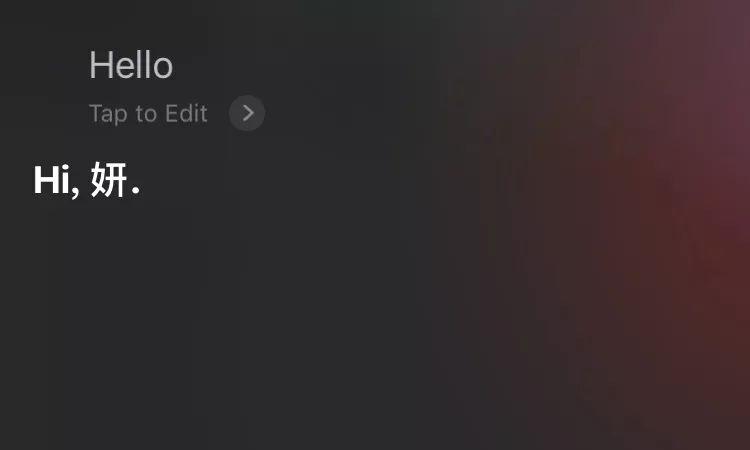
Japanese
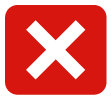
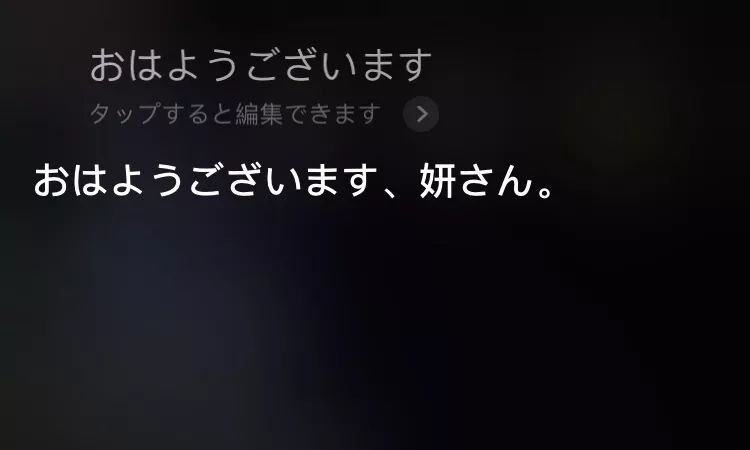
Korean
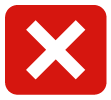
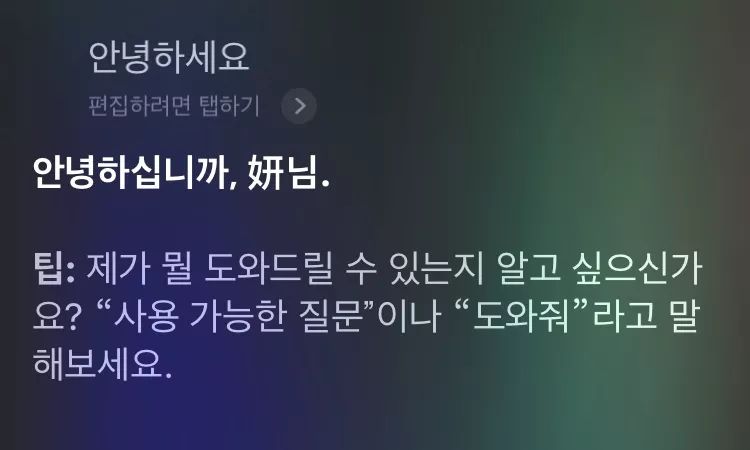
Thai
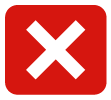
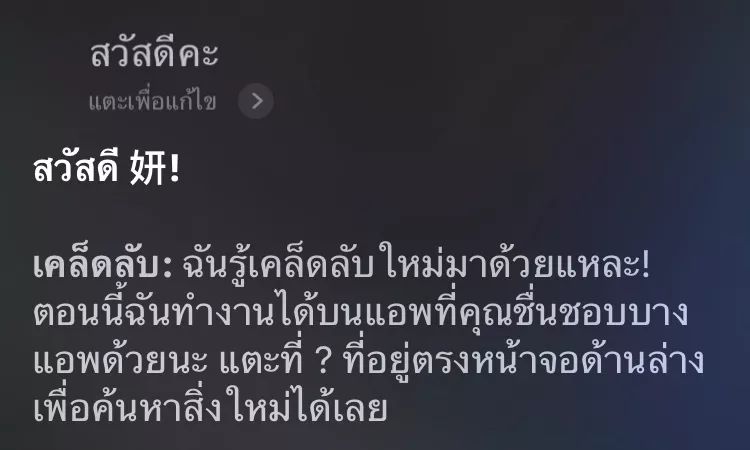
Without further ado, let me introduce to you the most familiar voice recognition.
Voice recognition technology, also known as automatic speech recognition (ASR), computer speech recognition, or speech-to-text recognition (STT), aims to automatically convert human speech into corresponding text using computers. Unlike speaker recognition, which attempts to identify or confirm the speaker of the voice, voice recognition focuses on the content of the words spoken.
The applications of voice recognition technology include voice dialing, voice navigation, indoor device control, voice document retrieval, simple dictation data entry, and more.
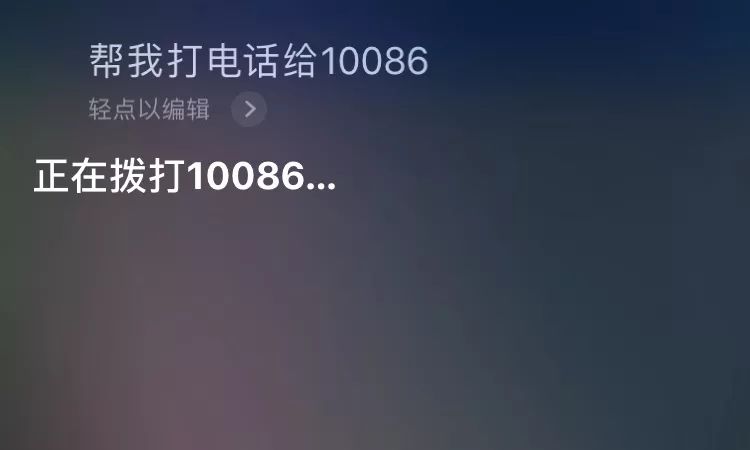
Voice dialing
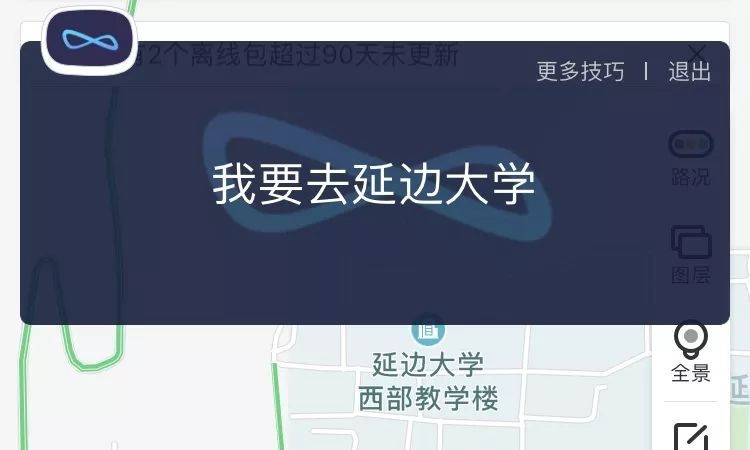
Voice navigation
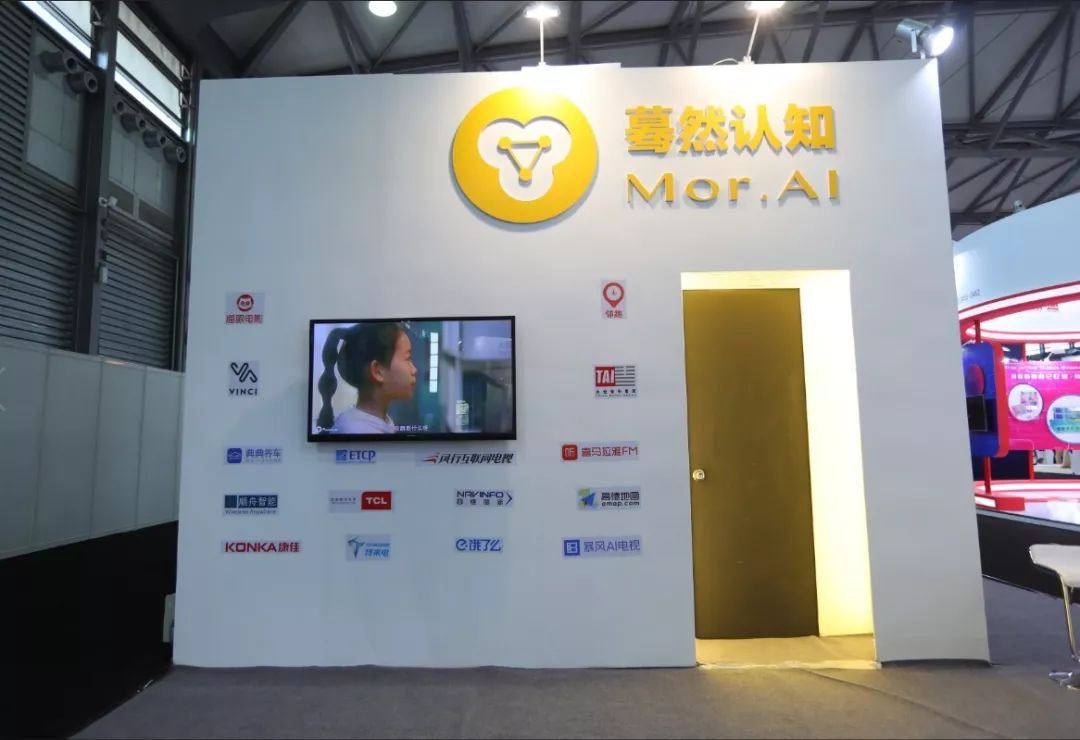
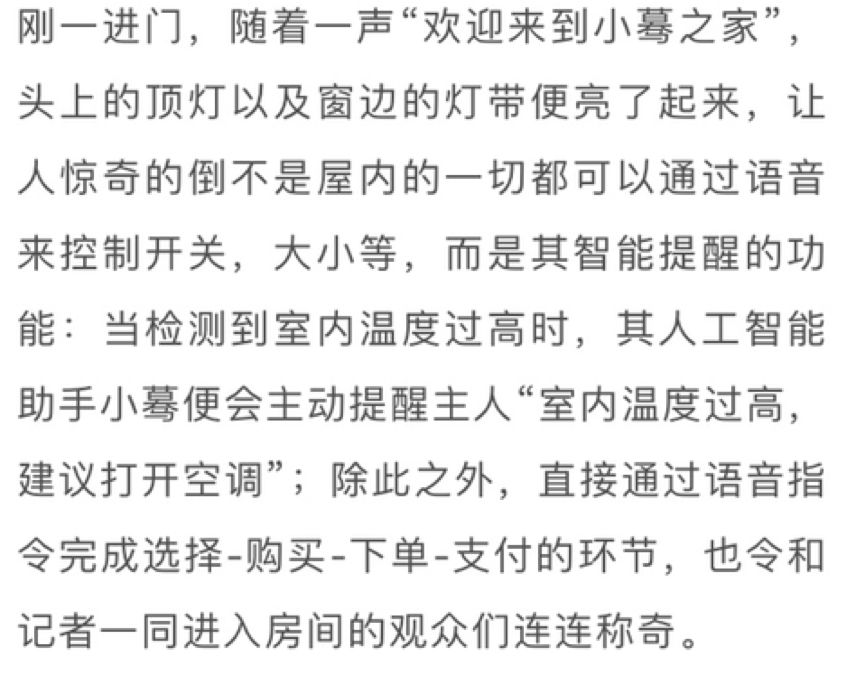
Indoor device control – suddenly realizing intelligent home
(Image source: Lei Feng Network)
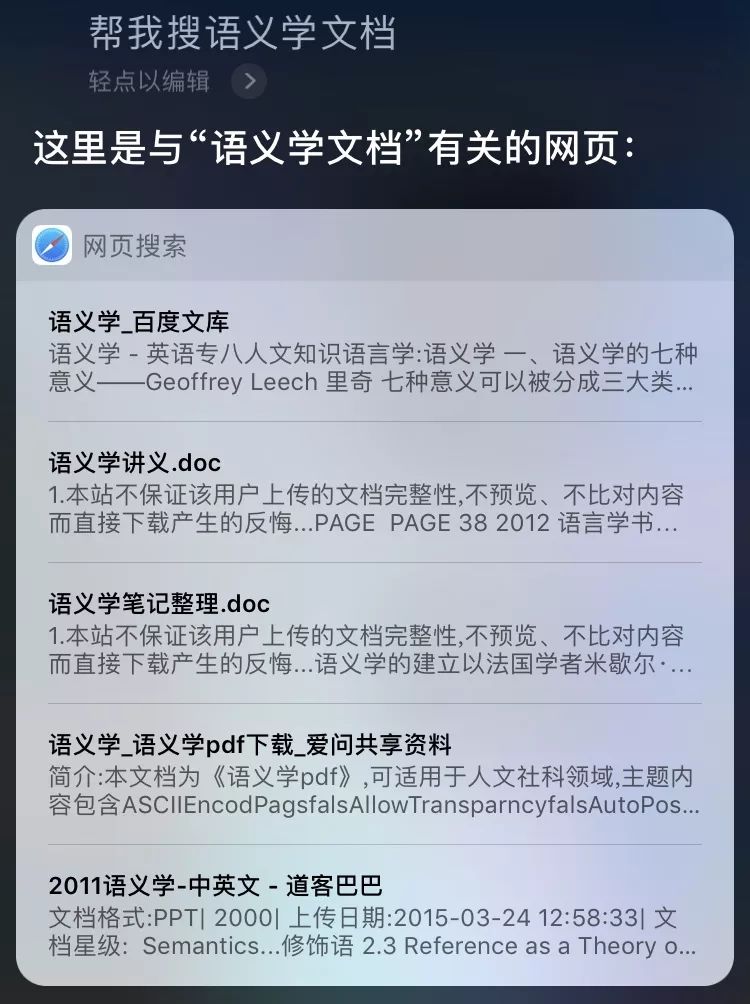
Voice document retrieval
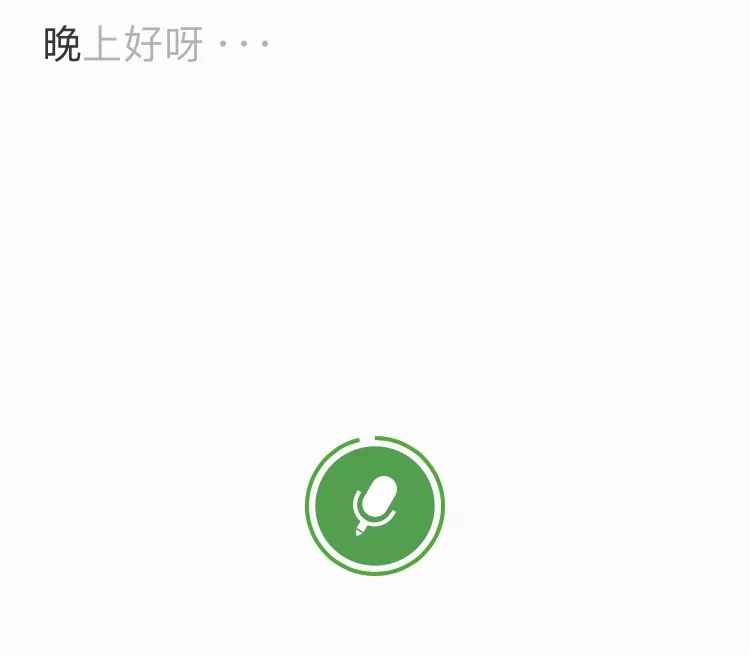
Simple dictation data entry
Voice recognition technology, combined with other natural language processing technologies such as machine translation and voice synthesis, can create more complex applications, such as voice-to-voice translation.
Fields involved in voice recognition technology include signal processing, pattern recognition, probability theory and information theory, vocal mechanism and auditory mechanism, artificial intelligence, and more.
In fact, even before the invention of computers, the concept of automatic speech recognition was already on the agenda, and early vocoders can be seen as the prototype of speech recognition and synthesis.
The “Radio Rex” toy dog produced in the 1920s may be the earliest voice recognizer, as it could pop out from its base when its name was called. The earliest computer-based speech recognition system was the Audrey speech recognition system developed by AT&T Bell Labs, which could recognize 10 English digits. Its recognition method tracked the resonances in speech, achieving a 98% accuracy rate.
By the late 1950s, Denes at the College of London had incorporated grammatical probabilities into speech recognition.
In the 1960s, artificial neural networks were introduced into speech recognition. The two major breakthroughs of this era were Linear Predictive Coding (LPC) and Dynamic Time Warping (DTW) technology.
The most significant breakthrough in speech recognition technology was the application of Hidden Markov Models (HMM). From Baum’s mathematical reasoning to the research of Rabiner and others, Kai-Fu Lee at Carnegie Mellon University ultimately realized the first large vocabulary speech recognition system based on HMM called Sphinx. Since then, speech recognition technology has strictly not deviated from the HMM framework.
Although researchers have long tried to promote the “dictation machine”, speech recognition technology still cannot support dictation applications for unlimited domains and unlimited speakers.
Currently, mainstream large vocabulary speech recognition systems mostly use statistical pattern recognition technology. A typical speech recognition system based on statistical pattern recognition consists of the following basic modules:
Signal processing and feature extraction module
Acoustic model
Pronunciation dictionary
Language model
Decoder
The language model is trained using a large amount of text and can utilize the statistical laws of a language itself to help improve recognition accuracy. The language model is very important; without it, when the state network is large, the recognized results can be a complete mess.
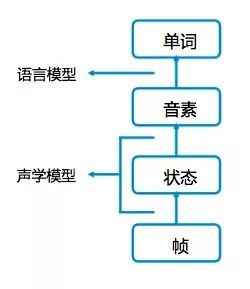
Now, let’s take a closer look at the Chinese acoustic features:
Taking Mandarin pronunciation as an example, we will segment the pronunciation of a character into two parts: initials and finals. During the pronunciation process, the transition from initials to finals is a gradual rather than instantaneous change. Therefore, I use the Right-Context-Dependent Initial Final (RCDIF) model as the analysis method to more accurately identify the correct syllables.
According to the different characteristics of initials, they can be divided into the following four categories:
Plosive:
When pronounced, the lips are tightly closed, and air is expelled to create a sound similar to an explosion. The amplitude of the sound will first drop to a minimum (representing closed lips) and then sharply rise, depending on whether there is sustained airflow. If there is sustained airflow (aspirated), there may be another peak in amplitude; if not (un-aspirated), the amplitude will decrease after the peak. For example, ㄆ and ㄅ have this relationship, where ㄆ has sustained airflow, while ㄅ does not. The left side of the right figure is ㄅ, and the right side is ㄆ.
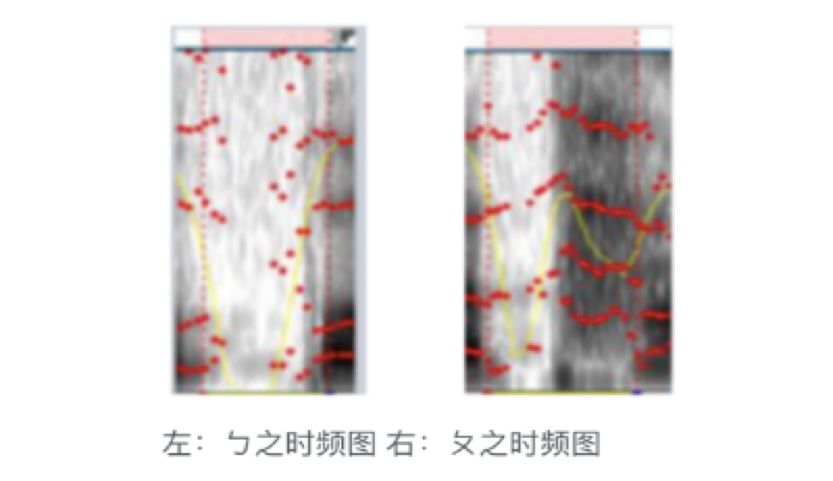
Fricative:
When pronounced, the tongue is pressed against the hard palate, forming a narrow channel, and turbulence occurs as the airflow passes, creating friction and thus sound. Since fricatives are produced by stable airflow, the amplitude changes are relatively small compared to plosives. Examples include ㄏ and ㄒ.
Affricate:
This type of sound model combines the characteristics of both plosives and fricatives. Its primary sound structure is similar to that of fricatives, where the tongue is pressed against the hard palate to create friction. However, the channel is tighter, causing the airflow to burst out suddenly, producing characteristics similar to plosives. For example, ㄑ and ㄔ.
Nasal:
When pronounced, the soft palate is lowered, blocking the airflow from the trachea into the oral cavity, directing it to the nasal cavity. This results in resonance between the nasal and oral cavities, which can be clearly observed in the time-frequency spectrum on the right, where the zero points (formants) exhibit resonance. This phenomenon is particularly evident in the RCDIF model. Therefore, this phenomenon can serve as one of the important bases for identifying nasals. The right figure shows the characteristics of the nasal ㄋ, where the red dot represents the zero point (formants).
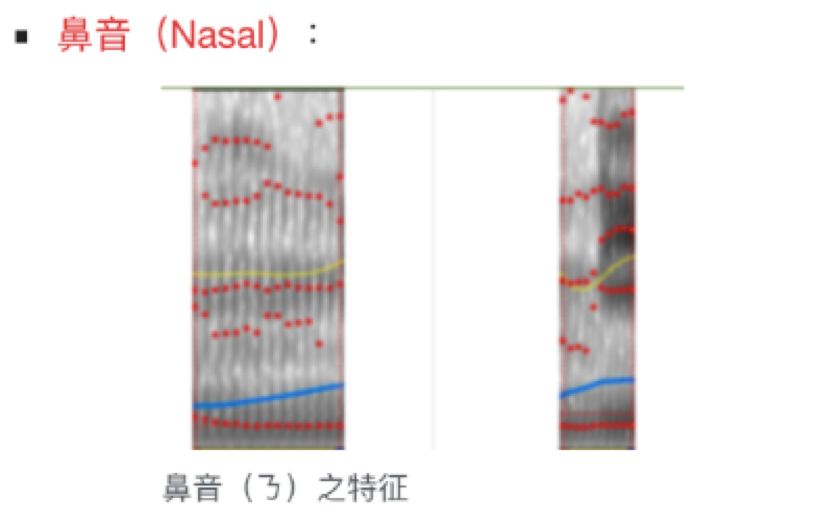
(Image source: Wikipedia)
Finals can also be divided into diphthongs and monophthongs, depending on whether there is a change in pitch during pronunciation. Based on whether the vocal cords vibrate, they can also be classified as voiceless (unvoiced: vocal cords do not vibrate) and other differences. The various pronunciation methods can generally be identified in the time-frequency graph, and by processing the two-dimensional time-frequency graph through traditional video processing methods, the goal of speech recognition can be achieved.
Related Concept: Voice Input Method
The voice input method allows users to convert spoken words into text input on digital devices, including smartphones, tablets, desktop computers, and laptops. Some devices may require additional input devices to use the voice input function.
The advantage of the voice input method is that users can convert their spoken words into text input. However, due to individual differences in tone, volume, and pronunciation, it may misrecognize similar sounds or homophones, which may not convey the user’s intended meaning. Users need to review and correct the output, although some systems have learning capabilities that allow them to understand the user’s preferences, thus improving accuracy over time for repeated users.
Currently, on Android phones, users can use Google Voice Input Method to achieve voice input by pressing the microphone button in the typing area.
The speed of the voice input method can be very fast; excluding the time needed for subsequent checking or correction, it can reach about 200 to 300 words per minute, depending on the speaker’s speed.
In situations requiring quick communication, such as using LINE or other messaging apps, the voice input method can save users a lot of typing time and avoid issues with slow typing speed or incorrect word selection, or quickly use voice input to search for information. Combined with natural language technology, the voice input method can simulate conversations with humans and achieve effects similar to chatbots.
Related Concept: Voice Processing
Voice processing, also known as speech signal processing or human voice processing, aims to produce desired signals for further speech recognition, applicable to mobile interfaces and everyday life, enabling communication between humans and computers.
The source of speech signals:
Human voice is produced by the vibration of vocal cords. When muscle movements compress the lungs, air flows through the vocal cords, causing periodic vibrations that generate sound. The air, carrying kinetic energy, exits the trachea into the oral or nasal cavity, vibrating in the chamber before leaving through the lips to reach human ears as sound.
Adjusting the position of the tongue in the oral cavity can produce different types of sounds. If the tongue remains relatively still, the air resonates only in the oral cavity and exits through the lips, producing vowels. If the tongue is raised, connecting the oral and nasal cavities, nasal sounds are produced.
Classification of speech signals:
From the perspective of Chinese pronunciation, sounds can still be divided into consonants and vowels. Vowels and consonants can be distinguished in two ways:
Articulation method: Generally speaking, vowels are related to the shape of the lips and do not resonate with the nasal cavity. In contrast, consonants utilize the nasal cavity in their articulation.
Frequency spectrum analysis: Observing the frequency spectrum reveals that consonant signal frequencies are higher, shorter in duration, and appear before vowels. Vowels have lower frequencies, longer durations, and can appear after consonants or independently, with vowels generally having greater energy than consonants.
Below is a list of vowels and consonants in Chinese phonetic symbols along with their pinyin.
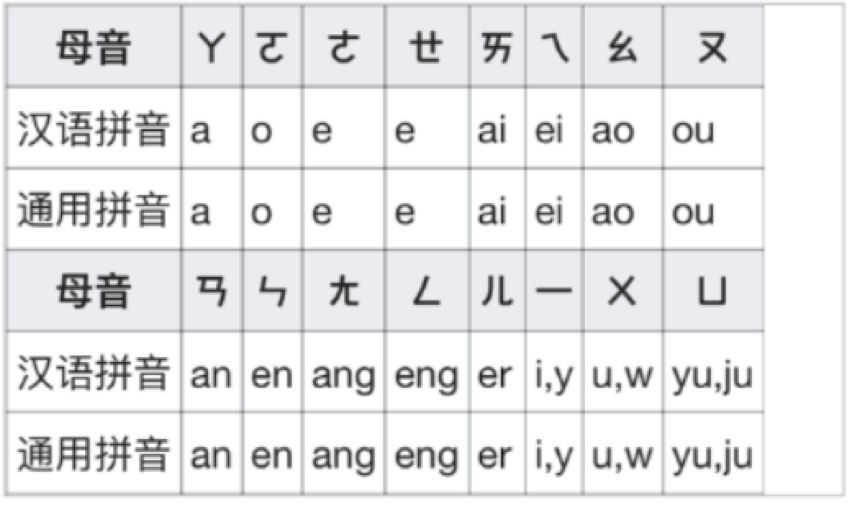
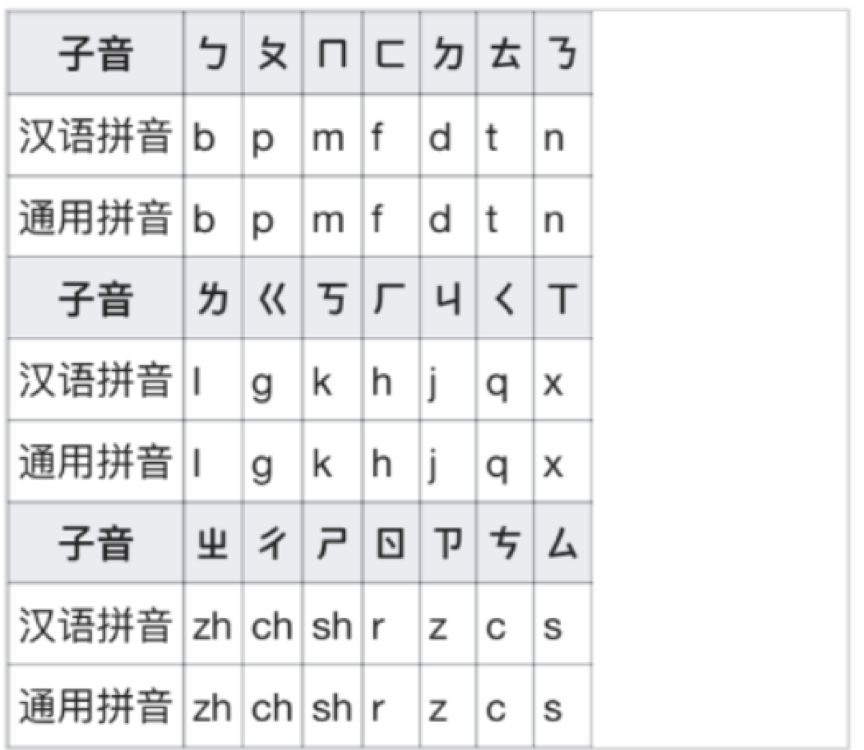
(Image source: Wikipedia)
The architecture of speech:
To analyze speech signals, one must first understand its architecture. The elements of speech, from smallest to largest, are: phoneme → syllable → word → sentence → paragraph.
A phoneme is the smallest unit of sound; for example, the phoneme of the character “呵” is “ㄏ” and “ㄜ”. However, phonemes and phonetic symbols are not equivalent. For example, “鸥” has only “ㄡ” as its vowel, but since it is a diphthong, it will be split into two phonemes. In Chinese, a syllable corresponds to a single character; for instance, “天天开心” has four syllables. Words are meaningful segments composed of characters, and various words combine to form sentences, ultimately creating paragraphs, which is the architecture of speech.
Voice processing has two main goals:
To reduce signal noise and produce desired signal modules;
To perform speech recognition, enabling communication between humans and computers.

Hofstadter said: “For a machine to understand what humans say, it must have legs, be able to walk, observe the world, and gain the experiences it needs. It must be able to live with people and experience their lives and stories…“

IBM’s speech recognition expert Frederick Jelinek once joked: “Every time I fire a linguist, the recognition rate goes up.” The reason is that speech recognition is equivalent to a lexer, while linguists study parsers and interpreters.
In the era of deep learning, we rely less on linguistic knowledge for speech recognition.
For example, for a frame of speech, after extracting features, we directly use it as an input vector for DNN (Deep Neural Network), with its output representing the predicted probabilities for each phoneme. We expect the algorithm to automatically learn probability predictions through backpropagation, marking the maturity of “intelligent” speech recognition.
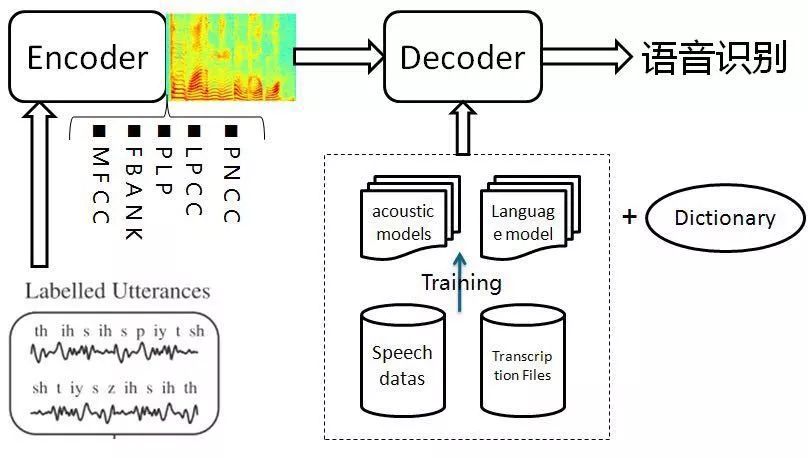
The general architecture of a speech recognition system is shown on the left, divided into training and decoding stages.
Training involves training the acoustic model using a large amount of labeled speech data;
Decoding involves recognizing speech data outside the training set into text using the acoustic and language models.
In simple terms, the work of language recognition is merely to recognize frames as states (the difficult point); to combine states into phonemes; and to combine phonemes into words.
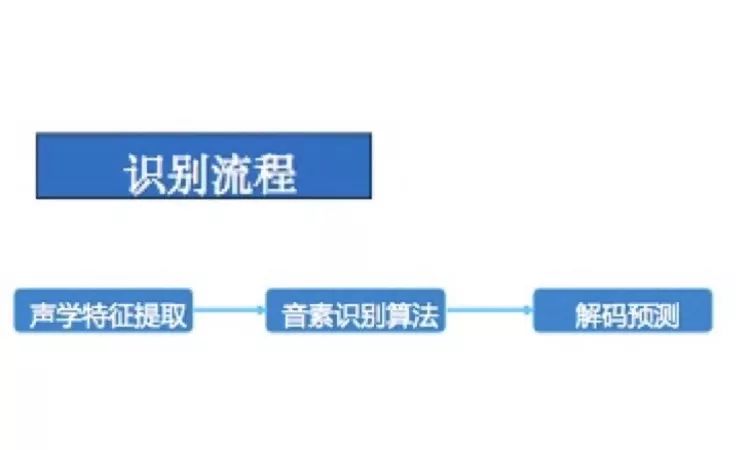
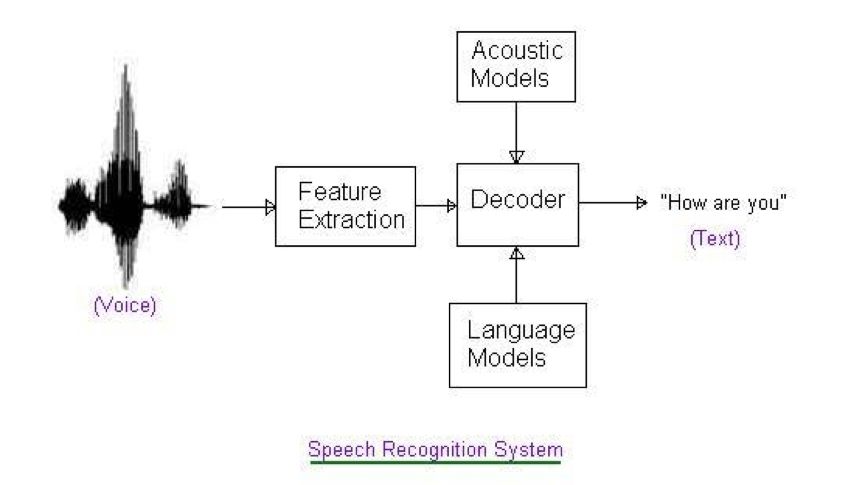
From data processing and feature extraction to model initialization, from monophoneme to triphoneme training and decoding, from maximum likelihood training to discriminative training based on maximum mutual information, and a large number of configuration files, it requires a high learning time cost; to achieve a relatively good recognition accuracy, alignment operations are also needed.
(References: Zhihu; Wikipedia)
Special thanks
Thanks to Zhao Yufan, a student from the Sino-French Engineering School of Beihang University (Natural Language Processing Major), for the valuable suggestions for this article.

Written by: Li Yan
(Studying at Yanbian University)
Image and text editor: Li Yan
Proofreader: Yi Ran
Editor: Lao Gan
Submission email: [email protected]
Previous reviews:
Special Report | Fang Fang: Using AI to Preserve Dialects and Build a Dialect Protection Platform
Language Technology | Li Dadong: Worried About Dialect Extinction? Artificial Intelligence May Help 【Forward】
Language Technology | iFly Input Method: Now, You Can Chat with the World in Suzhou Dialect! 【Forward】
Scan to Follow: Language Resource Bulletin
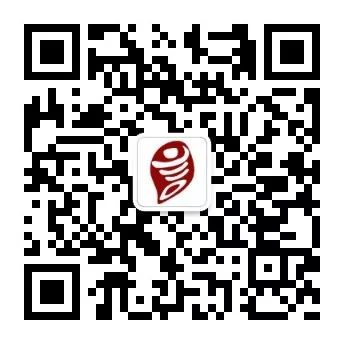
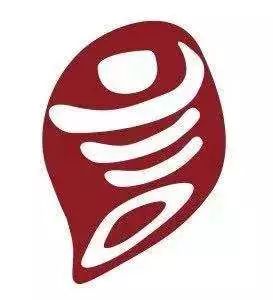
Dear Readers:
Running a language resource protection public account is not easy, and updating it daily is even harder. Providing new language data every day is especially challenging. Many readers have not yet developed the habit of giving rewards. We advocate a monthly reward of one yuan; little by little, our cause will have hope. Thank you all!