Special Column: Machine Learning and Computational Applications
Host: Zhang Zhen: Deputy Director of the National Engineering Laboratory for Collaborative Security Technology of Big Data
Preface: Machine learning is an important branch of artificial intelligence, involving multiple disciplines such as probability theory, statistics, and algorithm complexity theory. By allowing computers to automatically learn and extract patterns from large amounts of data, it enables them to possess learning and decision-making capabilities similar to those of humans. In recent years, the widespread application of technologies such as the Internet of Things, cloud computing, and big data has provided strong support for research in machine learning. The “Notice of the State Council on Printing and Distributing the New Generation Artificial Intelligence Development Plan” points out that artificial intelligence has become a new focus of international competition and a new engine for economic development. The Ministry of Education’s “Action Plan for Innovation in Artificial Intelligence in Higher Education” advocates that in the face of opportunities for the development of the new generation of artificial intelligence, universities should further strengthen applied basic research and breakthroughs in common key technologies, continuously promote the deep integration of artificial intelligence and the real economy, and cultivate new functions for economic development. The Ministry of Science and Technology has specifically strengthened the top-level design of artificial intelligence and initiated major scientific and technological projects for the new generation of artificial intelligence. Against this background, research on machine learning and computational applications has important theoretical significance and practical application value. In the paper “Recent Advances in Low-Resource Few-Shot Continuous Speech Recognition”, the authors systematically summarize and analyze the latest technologies, research difficulties, and future research directions in low-resource few-shot speech recognition technology; in the paper “An Algorithm for Color Image Steganalysis Based on Adversarial Mechanisms”, the authors propose a deep convolutional network based on adversarial mechanisms, effectively addressing the problem of steganalysis of color graphics; in the paper “Application of Improved YOLOv5 Algorithm in Parking Lot Fire Detection”, the authors propose an improved YOLOv5 algorithm tailored for the application scenario of parking lot fire detection, which can effectively detect small flame targets; in the paper “Classification of Imbalanced Data Evidence Under Composite Reliability Analysis”, the authors address the issue where traditional classification models focus on large classes while neglecting small classes when dealing with imbalanced data, proposing a classification method for imbalanced data evidence under composite reliability analysis, enhancing the model’s classification capability for imbalanced data.
Recent Advances in Low-Resource Few-Shot Continuous Speech Recognition
Qu Dan, Yang Xukui, Yan Honggang, Chen Yaqi, Niu Tong
Information System Engineering College, Strategic Support Force Information Engineering University
Click “Read Original” at the end of the article to view the literature!
Table of Contents
01
Abstract
02
Chart Appreciation
03
Cite This Article
04
Author Introduction
Abstract
Automatic speech recognition technology is a very active area in artificial intelligence, and the latest speech recognition technologies have already surpassed human capabilities on certain specific datasets, but these breakthroughs heavily rely on large amounts of speech samples and labeled data. In most industry applications, the actual conditions faced are insufficient to establish effective speech recognition systems. Therefore, low-resource few-shot speech recognition is an urgent technical demand faced by the speech recognition industry. Low-resource minority language speech recognition refers to a lack of relevant data resources for training, including labeled speech, pronunciation dictionaries, and texts, among which the impact of pronunciation dictionaries and labeled speech is particularly significant. The difficulty in obtaining minority language speech data is reflected not only in the speech itself but also in the corpus, pronunciation dictionaries, and labeling resources. Compared with traditional speech recognition, few-shot low-resource speech recognition has many targeted technologies. Therefore, this article first focuses on introducing several key technological research advances in low-resource speech in terms of feature extraction, acoustic models, and resource expansion. Based on the development of continuous speech recognition framework technologies, advanced modeling techniques in deep learning have also brought new vitality to low-resource speech recognition technologies in recent years. This article emphasizes the latest developments of advanced deep learning technologies such as Generative Adversarial Networks, Self-supervised Representation Learning, Deep Reinforcement Learning, and Meta-learning in solving few-shot speech recognition. The core goal is to overcome the impacts of low-resource harsh environments from several aspects: first, data expansion at the data level, for instance, both Generative Adversarial Networks and Deep Reinforcement Learning can perform data augmentation; second, utilizing existing data to obtain more generalized and representative feature extraction algorithms, such as autoencoders and self-supervised representation learning technologies; third, referencing and utilizing existing relevant knowledge, such as transfer learning and meta-learning techniques; fourth, seeking better learning mechanisms, such as meta-learning, adversarial learning, and deep reinforcement learning mechanisms. On this basis, the article analyzes the current challenges faced by this technology, such as limited complementarity, task imbalance, and model deployment issues, providing new ideas and measures for subsequent development, and finally summarizes and prospects the few-shot continuous speech recognition.
Chart Appreciation
Figure 1 Low-Resource Few-Shot Continuous Speech Recognition Technology
Figure 1 Low-resource few-shot continuous speech recognition
Figure 2 Advanced Deep Learning Framework in Few-Shot Speech Recognition
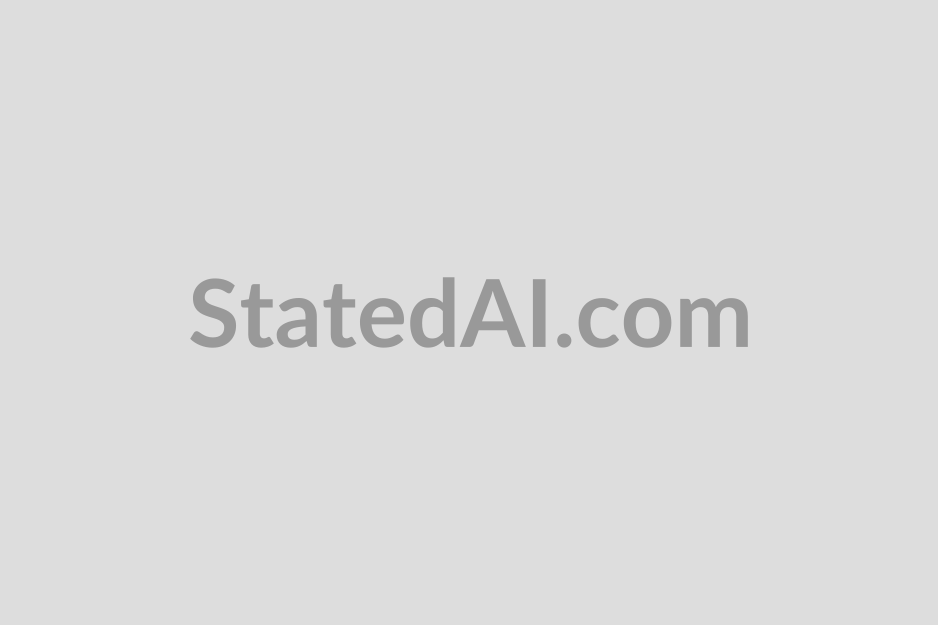
Cite This Article
Qu Dan, Yang Xukui, Yan Honggang, et al. Overview of recent progress in low-resource few-shot continuous speech recognition [J]. Journal of Zhengzhou University (Engineering Science), 2023, 44(4): 1-9.
QU D, YANG X K, YAN H G, et al. Overview of recent progress in low-resource few-shot continuous speech recognition [J]. Journal of Zhengzhou University (Engineering Science), 2023, 44(4): 1-9.
Author Introduction
1 Qu Dan
Professor, Doctor, PhD Supervisor, Information System Engineering College, Strategic Support Force Information Engineering University.
Main Research and Teaching Experience:Obtained a doctorate from Information Engineering University in 2005, a leading talent in scientific innovation in the Central Plains of Henan Province. Long engaged in scientific research and teaching work in the basic theory of artificial intelligence and intelligent information processing, reviewer for the National Natural Science Foundation and the Ministry of Science and Technology.
Research Achievements:Published over 100 academic papers in international journals, conferences, and domestic core journals, representative papers include: “Improving Speech Translation by Cross-Modal Multi-Grained Contrastive Learning”, “DropDim: A Regularization Method for Transformer Networks”, “An adapted data selection for deep learning-based audio segmentation in multi-genre broadcast channel”, etc.; published 6 monographs; applied for nearly 20 patents and software copyrights; received the second prize for provincial scientific and technological progress 4 times. Led and completed 5 national 863 projects, National Natural Science Foundation projects, and National Social Science Foundation projects. Ongoing projects include: National Natural Science Foundation project (No.62171470), Henan Province Central Plains Scientific Innovation Leading Talent Project (234200510019), and 14th Five-Year Plan Pre-research Project (30601010203), among others, with multiple achievements applied to frontline departments.
Recruitment Directions and Application Requirements:Master’s degree candidates are recruited in speech processing and recognition, digital image processing, text processing, etc.; Doctoral candidates are recruited in multimedia information processing, pattern recognition, and artificial intelligence, welcome to apply!
Contact Information:Email: [email protected].
✦
Contact Information for Journal of Zhengzhou University (Engineering Science):
Submission website: http://gxb.zzu.edu.cn
WeChat public account: zdxbgxb
Contact email: [email protected]
Contact number: 0371-67781276
Contact number: 0371-67781277
For more exciting content, please follow us
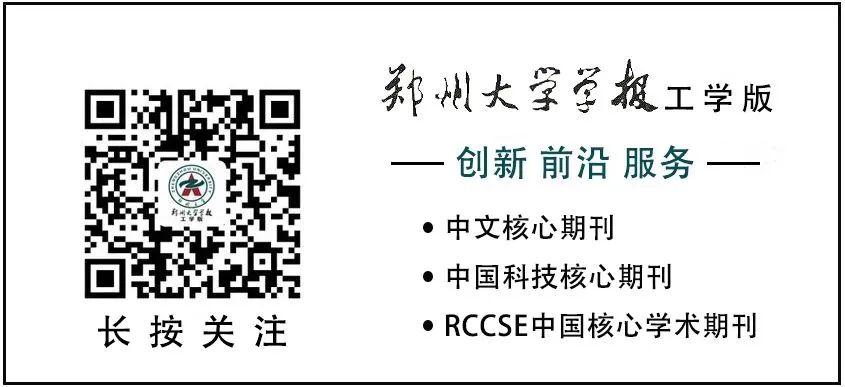
Copyright Statement:
This article is original content from the Editorial Department of the Journal of Zhengzhou University (Engineering Science), reprints are welcome!
