Since the concept of “Attention is All You Need” was introduced in 2017, the Transformer model has quickly emerged in the field of Natural Language Processing (NLP), establishing its leading position. By 2021, the idea that “one image is equivalent to 16×16 words” successfully brought the Transformer model into computer vision tasks. Since then, numerous Transformer-based architectures have emerged and been applied in the field of computer vision.
This article will detail the Vision Transformer (ViT) described in “one image is equivalent to 16×16 words”, including its open-source code and conceptual explanations of various components. All code is implemented using the PyTorch Python package.
This article is one of a series of in-depth studies on the internal workings of Vision Transformers, providing an executable code version in Jupyter Notebook. Other articles in the series include: Analysis of Vision Transformers, Application of Attention Mechanism in Vision Transformers, Analysis of Position Encoding in Vision Transformers, Tokens-to-Token Vision Transformers Analysis, and the GitHub repository for the Vision Transformers analysis series.
So, what are Vision Transformers? As introduced in “Attention is All You Need”, Transformers are machine learning models that utilize attention mechanisms as their primary learning mechanism. They quickly became the leading technology for sequence-to-sequence tasks (such as language translation).
The concept of “one image is equivalent to 16×16 words” successfully improved the Transformer proposed in [1] to handle image classification tasks, giving rise to the Vision Transformer (ViT). Like the Transformer in [1], ViT is based on the attention mechanism. However, unlike the Transformer used for NLP tasks, which contains both encoder and decoder attention branches, ViT only uses the encoder. The output of the encoder is then passed to a neural network “head” for prediction.
However, the ViT implemented in “one image is equivalent to 16×16 words” has a drawback: its optimal performance requires pre-training on large datasets. The best model was pre-trained on the proprietary JFT-300M dataset. In contrast, models pre-trained on the smaller open-source ImageNet-21k dataset perform comparably to the state-of-the-art convolutional ResNet models.
Tokens-to-Token ViT: Training Vision Transformers from Scratch on ImageNet attempts to eliminate this pre-training requirement by introducing a novel preprocessing method that converts input images into a series of tokens. For more information on this method, please refer to the relevant literature. In this article, we will focus on the ViT implemented in “one image is equivalent to 16×16 words”.
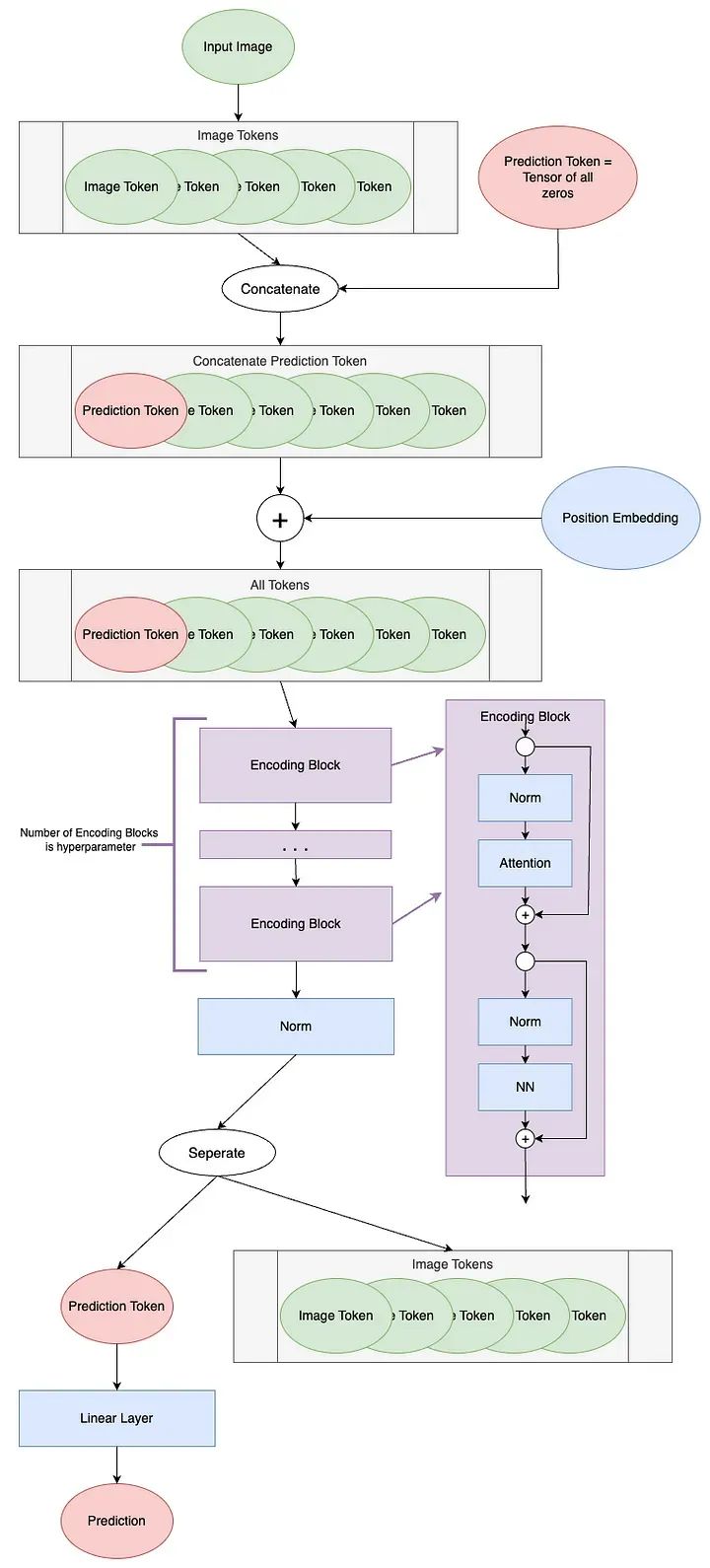
ViT model diagram
The first step of ViT is to create tokens from the input image. Transformers operate on a series of tokens; in NLP, this is typically the words of a sentence. For computer vision, how to segment the input into tokens is not very clear. ViT converts images into tokens such that each token represents a local area (or patch) of the image. They describe how to reshape an image with height H, width W, and C channels into N patches of size P:
Each token has a length of P²*C. Let’s take this pixel art “Mountain at Dusk” (by Luis Zuno) as an example for patch tokenization. The original artwork has been cropped and converted to a single-channel image. This means that the value of each pixel is between 0 and 1. Single-channel images are usually displayed in grayscale, but we will show it in a purple color scheme for better visibility. Note that the patch tokenization is not included in the code related to [3].
<span>mountains = np.load(os.path.join(figure_path, 'mountains.npy'))</span>
<span>H = mountains.shape[0]</span>
<span>W = mountains.shape[1]</span>
<span>print('Mountain at Dusk is H =', H, 'and W =', W, 'pixels.')</span>
<span>print('
')</span>
<span>fig = plt.figure(figsize=(10,6))</span>
<span>plt.imshow(mountains, cmap='Purples_r')</span>
<span>plt.xticks(np.arange(-0.5, W+1, 10), labels=np.arange(0, W+1, 10))</span>
<span>plt.yticks(np.arange(-0.5, H+1, 10), labels=np.arange(0, H+1, 10))</span>
<span>plt.clim([0,1])</span>
<span>cbar_ax = fig.add_axes([0.95, .11, 0.05, 0.77])</span>
<span>plt.clim([0, 1])</span>
<span>plt.colorbar(cax=cbar_ax);</span>
<span>#plt.savefig(os.path.join(figure_path, 'mountains.png'))</span>
<span>Mountain at Dusk <span>is</span> H = <span>60</span> <span>and</span> W = <span>100</span> pixels.</span>
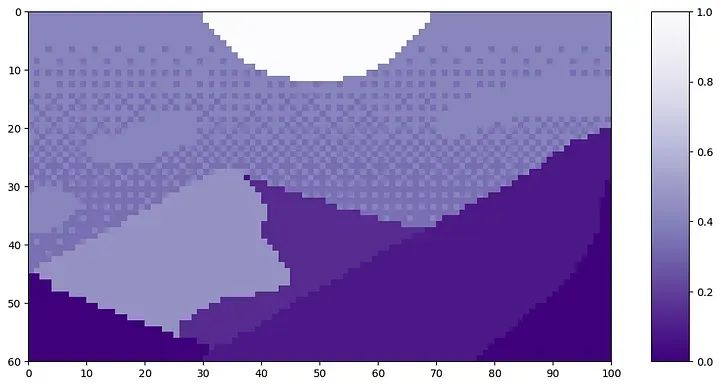
<span>P = 20</span>
<span>N = int((H*W)/(P**2))</span>
<span>print('There will be', N, 'patches, each', P, 'by', str(P)+'.')</span>
<span>print('
')</span>
<span>fig = plt.figure(figsize=(10,6))</span>
<span>plt.imshow(mountains, cmap='Purples_r')</span>
<span>plt.hlines(np.arange(P, H, P)-0.5, -0.5, W-0.5, color='w')</span>
<span>plt.vlines(np.arange(P, W, P)-0.5, -0.5, H-0.5, color='w')</span>
<span>plt.xticks(np.arange(-0.5, W+1, 10), labels=np.arange(0, W+1, 10))</span>
<span>plt.yticks(np.arange(-0.5, H+1, 10), labels=np.arange(0, H+1, 10))</span>
<span>x_text = np.tile(np.arange(9.5, W, P), 3)</span>
<span>y_text = np.repeat(np.arange(9.5, H, P), 5)</span>
<span>for i in range(1, N+1):</span>
<span> plt.text(x_text[i-1], y_text[i-1], str(i), color='w', fontsize='xx-large', ha='center')</span>
<span>plt.text(x_text[2], y_text[2], str(3), color='k', fontsize='xx-large', ha='center');</span>
<span>#plt.savefig(os.path.join(figure_path, 'mountain_patches.png'), bbox_inches='tight'</span>
<span>There will be <span>15</span> patches, each <span>20</span> <span>by</span> <span>20.</span></span>
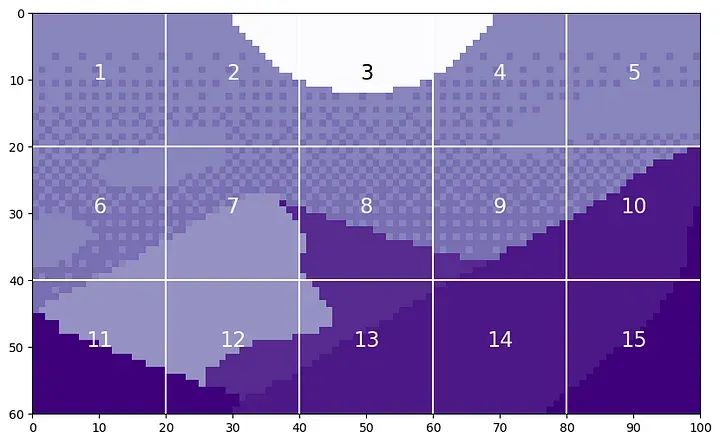
<span><span>print</span>(<span>'Each patch will make a token of length'</span>, str(P**<span>2</span>)+<span>'.'</span>)</span>
<span><span>print</span>(<span>'
'</span>)</span>
<span>patch12 = mountains[<span>40</span>:<span>60</span>, <span>20</span>:<span>40</span>]</span>
<span>token12 = patch12.reshape(<span>1</span>, P**<span>2</span>)</span>
<span>fig = plt.figure(figsize=(<span>10</span>,<span>1</span>))</span>
<span>plt.imshow(token12, aspect=<span>10</span>, cmap=<span>'Purples_r'</span>)</span>
<span>plt.clim([<span>0</span>,<span>1</span>])</span>
<span>plt.xticks(np.arange(<span>-0.5</span>, <span>401</span>, <span>50</span>), labels=np.arange(<span>0</span>, <span>401</span>, <span>50</span>))</span>
<span>plt.yticks([]);</span>
<span>#plt.savefig(os.path.join(figure_path, <span>'mountain_token12.png'</span>), bbox_inches=<span>'tight'</span>)</span>
<span>Each patch will make a token <span>of</span> length <span>400.</span></span>
After extracting tokens from the image, a linear projection is typically used to change the length of the tokens. This is achieved through a learnable linear layer. The new token length is referred to as the latent dimension, channel dimension, or token length. After projection, the tokens can no longer be visually recognized as patches of the original image. Now that we understand this concept, we can look at how patch tokenization is implemented in code.
<span><span><span>class</span> <span>Patch_Tokenization</span>(<span>nn</span>.<span>Module</span>):</span></span>
<span> <span><span>def</span> <span>__init__</span><span>(<span>self</span>,</span></span></span><code><span> <span>img_size:</span> tuple[int, int, int]=(<span>1</span>, <span>1</span>, <span>60</span>, <span>100</span>)</span>,
<span> <span>patch_size:</span> int=<span>50</span>,</span>
<span> <span>token_len:</span> int=<span>768</span>):</span>
<span> <span>"""</span><span> Patch Tokenization Module</span></span>
<span> Args:</span>
<span> img_size (tuple[int, int, int]): size of input (channels, height, width)</span>
<span> patch_size (int): the side length of a square patch</span>
<span> token_len (int): desired length of an output token</span>
<span><span> """</span><span>"""</span></span>
<span> <span>super</span>().__init_<span>_</span>()</span>
<span> <span>## Defining Parameters</span></span>
<span> <span>self</span>.img_size = img_size</span>
<span> C, H, W = <span>self</span>.img_size</span>
<span> <span>self</span>.patch_size = patch_size</span>
<span> <span>self</span>.token_len = token_len</span>
<span> assert H % <span>self</span>.patch_size == <span>0</span>, <span>'Height of image must be evenly divisible by patch size.'</span></span>
<span> assert W % <span>self</span>.patch_size == <span>0</span>, <span>'Width of image must be evenly divisible by patch size.'</span></span>
<span> <span>self</span>.num_tokens = (H / <span>self</span>.patch_size) * (W / <span>self</span>.patch_size)</span>
<span> <span>## Defining Layers</span></span>
<span> <span>self</span>.split = nn.Unfold(kernel_size=<span>self</span>.patch_size, stride=<span>self</span>.patch_size, padding=<span>0</span>)</span>
<span> <span>self</span>.project = nn.Linear((<span>self</span>.patch_size**<span>2</span>)*C, token_len)</span>
<span> <span><span>def</span> <span>forward</span><span>(<span>self</span>, x)</span></span>:</span>
<span> x = <span>self</span>.split(x).transpose(<span>1</span>,<span>0</span>)</span>
<span> x = <span>self</span>.project(x)</span>
<span> <span>return</span> x</span>
<span>x = torch.from_numpy(mountains).unsqueeze(0).unsqueeze(0).to(torch.float32)</span>
<span>token_len = 768</span>
<span>print('Input dimensions are
batchsize:', x.shape[0], '
number of input channels:', x.shape[1], '
image size:', (x.shape[2], x.shape[3]))</span>
<span># Define the Module</span>
<span>patch_tokens = Patch_Tokenization(img_size=(x.shape[1], x.shape[2], x.shape[3]),</span>
<span> patch_size = P,</span>
<span> token_len = token_len)</span>
<span>Input dimensions are</span>
<span> batchsize: 1 </span>
<span> number of input channels: 1 </span>
<span> image size: (60, 100)</span>
<span>x = patch_tokens.split(x).transpose(2,1)</span>
<span>print('After patch tokenization, dimensions are
batchsize:', x.shape[0], '
number of tokens:', x.shape[1], '
token length:', x.shape[2])</span>
<span><span>After</span> <span>patch tokenization, dimensions are</span></span>
<span> <span>batchsize</span>: <span>1 </span></span>
<span> <span>number</span> <span>of tokens: 15 </span></span>
<span> <span>token</span> <span>length: 400</span></span>
<span><span>x</span> = patch_tokens.<span>split</span>(<span>x</span>).transpose(<span>2</span>,<span>1</span>)</span>
<span><span>print</span>(<span>'After patch tokenization, dimensions are
batchsize:'</span>, x.shape[<span>0</span>], <span>'
number of tokens:'</span>, x.shape[<span>1</span>, <span>'
token length:'</span>, x.shape[<span>2</span>])</span>
<span><span>After</span> <span>patch tokenization, dimensions are</span></span>
<span> <span>batchsize</span>: <span>1 </span></span>
<span> <span>number</span> <span>of tokens: 15 </span></span>
<span> <span>token</span> <span>length: 400</span></span>
The first step is to add a blank Token before the image Token, called the Prediction Token. This Token will be used to output the encoding blocks for prediction. It is initially blank—equivalent to zero—so it can gather information from other image Tokens.
<span><span># Define an Input</span></span>
<span>num_tokens = 175</span>
<span>token_len = 768</span>
<span>batch = 13</span>
<span>x = torch.rand(batch, num_tokens, token_len)</span>
<span><span>print</span>(<span>'Input dimensions are
batchsize:'</span>, x.shape[0], <span>'
number of tokens:'</span>, x.shape[1], <span>'
token length:'</span>, x.shape[2])</span>
<span><span># Append a Prediction Token</span></span>
<span>pred_token = torch.zeros(1, 1, token_len).expand(batch, -1, -1)</span>
<span><span>print</span>(<span>'Prediction Token dimensions are
batchsize:'</span>, pred_token.shape[0], <span>'
number of tokens:'</span>, pred_token.shape[1], <span>'
token length:'</span>, pred_token.shape[2])</span>
<span>x = torch.cat((pred_token, x), dim=1)</span>
<span><span>print</span>(<span>'Dimensions with Prediction Token are
batchsize:'</span>, x.shape[0], <span>'
number of tokens:'</span>, x.shape[1], <span>'
token length:'</span>, x.shape[2])</span>
<span><span>Input</span> <span>dimensions are</span></span>
<span> <span>batchsize</span>: <span>13 </span></span>
<span> <span>number</span> <span>of tokens: 175 </span></span>
<span> <span>token</span> <span>length: 768</span></span>
<span><span>Prediction</span> <span>Token dimensions are</span></span>
<span> <span>batchsize</span>: <span>13 </span></span>
<span> <span>number</span> <span>of tokens: 1 </span></span>
<span> <span>token</span> <span>length: 768</span></span>
<span><span>Dimensions</span> <span>with Prediction Token are</span></span>
<span> <span>batchsize</span>: <span>13 </span></span>
<span> <span>number</span> <span>of tokens: 176 </span></span>
<span> <span>token</span> <span>length: 768</span></span>
<span><span><span>def</span> <span>get_sinusoid_encoding</span><span>(num_tokens, token_len)</span>:</span></span>
<span> <span>""" Make Sinusoid Encoding Table</span></span>
<span> Args:</span>
<span> num_tokens (int): number of tokens</span>
<span> token_len (int): length of a token</span>
<span> Returns:</span>
<span> (torch.FloatTensor) sinusoidal position encoding table</span>
<span> """</span>
<span> <span><span>def</span> <span>get_position_angle_vec</span><span>(i)</span>:</span></span>
<span> <span>return</span> [i / np.power(<span>10000</span>, <span>2</span> * (j // <span>2</span>) / token_len) <span>for</span> j <span>in</span> range(token_len)]</span>
<span> sinusoid_table = np.array([get_position_angle_vec(i) <span>for</span> i <span>in</span> range(num_tokens)])</span>
<span> sinusoid_table[:, <span>0</span>::<span>2</span>] = np.sin(sinusoid_table[:, <span>0</span>::<span>2</span>])</span>
<span> sinusoid_table[:, <span>1</span>::<span>2</span>] = np.cos(sinusoid_table[:, <span>1</span>::<span>2</span>]) </span>
<span> <span>return</span> torch.FloatTensor(sinusoid_table).unsqueeze(<span>0</span>)</span>
<span>PE = get_sinusoid_encoding(num_tokens+<span>1</span>, token_len)</span>
<span>print(<span>'Position embedding dimensions are
number of tokens:'</span>, PE.shape[<span>1</span>, <span>'
token length:'</span>, PE.shape[<span>2</span>])</span>
<span>x = x + PE</span>
<span>print(<span>'Dimensions with Position Embedding are
batchsize:'</span>, x.shape[<span>0</span>, <span>'
number of tokens:'</span>, x.shape[<span>1</span>, <span>'
token length:'</span>, x.shape[<span>2</span>])</span>
<span><span>Position</span> <span>embedding dimensions are</span></span>
<span> <span>number</span> <span>of tokens: 176 </span></span>
<span> <span>token</span> <span>length: 768</span></span>
<span><span>Dimensions</span> <span>with Position Embedding are</span></span>
<span> <span>batchsize</span>: <span>13 </span></span>
<span> <span>number</span> <span>of tokens: 176 </span></span>
<span> <span>token</span> <span>length: 768</span></span>
<span><span><span>def</span> <span>get_sinusoid_encoding</span><span>(num_tokens, token_len)</span>:</span></span>
<span> <span>""" Make Sinusoid Encoding Table</span></span>
<span> Args:</span>
<span> num_tokens (int): number of tokens</span>
<span> token_len (int): length of a token</span>
<span> Returns:</span>
<span> (torch.FloatTensor) sinusoidal position encoding table</span>
<span> """</span>
<span> <span><span>def</span> <span>get_position_angle_vec</span><span>(i)</span>:</span></span>
<span> <span>return</span> [i / np.power(<span>10000</span>, <span>2</span> * (j // <span>2</span>) / token_len) <span>for</span> j <span>in</span> range(token_len)]</span>
<span> sinusoid_table = np.array([get_position_angle_vec(i) <span>for</span> i <span>in</span> range(num_tokens)])</span>
<span> sinusoid_table[:, <span>0</span>::<span>2</span>] = np.sin(sinusoid_table[:, <span>0</span>::<span>2</span>])</span>
<span> sinusoid_table[:, <span>1</span>::<span>2</span>] = np.cos(sinusoid_table[:, <span>1</span>::<span>2</span>]) </span>
<span> <span>return</span> torch.FloatTensor(sinusoid_table).unsqueeze(<span>0</span>)</span>
<span>PE = get_sinusoid_encoding(num_tokens+<span>1</span>, token_len)</span>
<span>print(<span>'Position embedding dimensions are
number of tokens:'</span>, PE.shape[<span>1</span>, <span>'
token length:'</span>, PE.shape[<span>2</span>])</span>
<span>x = x + PE</span>
<span>print(<span>'Dimensions with Position Embedding are
batchsize:'</span>, x.shape[<span>0</span>, <span>'
number of tokens:'</span>, x.shape[<span>1</span>, <span>'
token length:'</span>, x.shape[<span>2</span>])</span>
<span><span>Position</span> <span>embedding dimensions are</span></span>
<span> <span>number</span> <span>of tokens: 176 </span></span>
<span> <span>token</span> <span>length: 768</span></span>
<span><span>Dimensions</span> <span>with Position Embedding are</span></span>
<span> <span>batchsize</span>: <span>13 </span></span>
<span> <span>number</span> <span>of tokens: 176 </span></span>
<span> <span>token</span> <span>length: 768</span></span>
Encoding Blocks
The code for the encoding blocks is as follows.
<span><span>class</span> <span>Encoding(nn.Module): def __init__(self, dim: int, num_heads: int=1, hidden_chan_mul: float=4., qkv_bias: bool=False, qk_scale: NoneFloat=None, act_layer=nn.GELU, norm_layer=nn.LayerNorm): """ Encoding Block Args: dim (int): size of a single token num_heads(int): number of attention heads in MSA hidden_chan_mul (float): multiplier to determine the number of hidden channels (features) in the NeuralNet component qkv_bias (bool): determines if the qkv layer learns an addative bias qk_scale (NoneFloat): value to scale the queries and keys by; if None, queries and keys are scaled by ``head_dim ** -0.5`` act_layer(nn.modules.activation): torch neural network layer class to use as activation norm_layer(nn.modules.normalization): torch neural network layer class to use as normalization """ super().__init__() ## Define Layers self.norm1 = norm_layer(dim) self.attn = Attention(dim=dim, chan=dim, num_heads=num_heads, qkv_bias=qkv_bias, qk_scale=qk_scale) self.norm2 = norm_layer(dim) self.neuralnet = NeuralNet(in_chan=dim, hidden_chan=int(dim*hidden_chan_mul), out_chan=dim, act_layer=act_layer) def forward(self, x): x = x + self.attn(self.norm1(x)) x = x + self.neuralnet(self.norm2(x)) return x</span></span>
<span><span># Define an Inputnum_tokens = 176token_len = 768batch = 13heads = 4x = torch.rand(batch, num_tokens, token_len)print('Input dimensions are
batchsize:', x.shape[0], '
number of tokens:', x.shape[1], '
token length:', x.shape[2])# Define the ModuleE = Encoding(dim=token_len, num_heads=heads, hidden_chan_mul=1.5, qkv_bias=False, qk_scale=None, act_layer=nn.GELU, norm_layer=nn.LayerNorm)E.eval();</span></span>
<span><span>Input</span> <span>dimensions are batchsize: 13 number of tokens: 176 token length: 768</span></span>
<span>y = E.norm1(x)<span>print</span>(<span>'After norm, dimensions are
batchsize:'</span>, y.shape[0], <span>'
number of tokens:'</span>, y.shape[1], <span>'
token size:'</span>, y.shape[2])y = E.attn(y)<span>print</span>(<span>'After attention, dimensions are
batchsize:'</span>, y.shape[0], <span>'
number of tokens:'</span>, y.shape[1], <span>'
token size:'</span>, y.shape[2])y = y + xprint(<span>'After split connection, dimensions are
batchsize:'</span>, y.shape[0], <span>'
number of tokens:'</span>, y.shape[1], <span>'
token size:'</span>, y.shape[2])</span>
<span><span>After</span> <span>norm, dimensions are batchsize: 13 number of tokens: 176 token size: 768After attention, dimensions are batchsize: 13 number of tokens: 176 token size: 768After split connection, dimensions are batchsize: 13 number of tokens: 176 token size: 768</span></span>
<span>z = E.norm2(y)</span>
<span><span>print</span>(<span>'After norm, dimensions are
batchsize:'</span>, z.shape[0], <span>'
number of tokens:'</span>, z.shape[1], <span>'
token size:'</span>, z.shape[2])</span>
<span>z = E.neuralnet(z)</span>
<span><span>print</span>(<span>'After neural net, dimensions are
batchsize:'</span>, z.shape[0], <span>'
number of tokens:'</span>, z.shape[1], <span>'
token size:'</span>, z.shape[2])</span>
<span>z = z + y</span>
<span><span>print</span>(<span>'After split connection, dimensions are
batchsize:'</span>, z.shape[0], <span>'
number of tokens:'</span>, z.shape[1], <span>'
token size:'</span>, z.shape[2])</span>
<span><span>After</span> <span>norm, dimensions are</span></span>
<span> <span>batchsize</span>: <span>13 </span></span>
<span> <span>number</span> <span>of tokens: 176 </span></span>
<span> <span>token</span> <span>size: 768</span></span>
<span><span>After</span> <span>neural net, dimensions are</span></span>
<span> <span>batchsize</span>: <span>13 </span></span>
<span> <span>number</span> <span>of tokens: 176 </span></span>
<span> <span>token</span> <span>size: 768</span></span>
<span><span>After</span> <span>split connection, dimensions are</span></span>
<span> <span>batchsize</span>: <span>13 </span></span><code><span> <span>number</span> <span>of tokens: 176 </span></span>
<span> <span>token</span> <span>size: 768</span></span>
<span><span><span>class</span> <span>NeuralNet</span>(<span>nn</span>.<span>Module</span>):</span></span>
<span> <span><span>def</span> <span>__init__</span><span>(<span>self</span>,</span></span></span><code><span> <span>in_chan:</span> int,</span>
<span> <span>hidden_chan:</span> NoneFloat=None,</span>
<span> <span>out_chan:</span> NoneFloat=None,</span>
<span><span> act_layer = nn.GELU)</span>:</span>
<span> <span>"""</span><span> Neural Network Module</span></span>
<span> Args:</span>
<span> in_chan (int): number of channels (features) at input</span>
<span> hidden_chan (NoneFloat): number of channels (features) in the hidden layer;</span>
<span> if None, number of channels in hidden layer is the same as the number of input channels</span>
<span> out_chan (NoneFloat): number of channels (features) at output;</span>
<span> if None, number of output channels is same as the number of input channels</span>
<span> act_layer(nn.modules.activation): torch neural network layer class to use as activation</span>
<span><span> """</span><span>"""</span></span>
<span> <span>super</span>().__init_<span>_</span>()</span>
<span> <span>## Define Number of Channels</span></span>
<span> hidden_chan = hidden_chan <span>or</span> in_chan</span>
<span> out_chan = out_chan <span>or</span> in_chan</span>
<span> <span>## Define Layers</span></span>
<span> <span>self</span>.fc1 = nn.Linear(in_chan, hidden_chan)</span>
<span> <span>self</span>.act = act_layer()</span>
<span> <span>self</span>.fc2 = nn.Linear(hidden_chan, out_chan)</span>
<span> <span><span>def</span> <span>forward</span><span>(<span>self</span>, x)</span></span>:</span>
<span> x = <span>self</span>.fc1(x)</span>
<span> x = <span>self</span>.act(x)</span>
<span> x = <span>self</span>.fc2(x)</span>
<span> <span>return</span> x</span>
<span><span># Define an Input</span></span>
<span>num_tokens = 176</span>
<span>token_len = 768</span>
<span>batch = 1</span>
<span>x = torch.rand(batch, num_tokens, token_len)</span>
<span>print('Input dimensions are
batchsize:', x.shape[0], '
number of tokens:', x.shape[1], '
token length:', x.shape[2])</span>
<span><span>Input</span> <span>dimensions are</span></span>
<span> <span>batchsize</span>: <span>1 </span></span>
<span> <span>number</span> <span>of tokens: 176 </span></span>
<span> <span>token</span> <span>length: 768</span></span>
<span>norm = nn.LayerNorm(token_len)</span>
<span>x = norm(x)</span>
<span><span>print</span>(<span>'After norm, dimensions are
batchsize:'</span>, x.shape[0], <span>'
number of tokens:'</span>, x.shape[1], <span>'
token size:'</span>, x.shape[2])</span>
<span><span>After</span> <span>norm, dimensions are</span></span>
<span> <span>batchsize</span>: <span>1 </span></span>
<span> <span>number</span> <span>of tokens: 1001 </span></span>
<span> <span>token</span> <span>size: 768</span></span>
<span>norm = nn.LayerNorm(token_len)</span>
<span>pred_token = x[:, 0]</span>
<span><span>print</span>(<span>'Length of prediction token:'</span>, pred_token.shape[-1])</span>
<span>Length <span>of</span> prediction token: <span>768</span></span>
<span>head = nn.Linear(token_len, 1)</span>
<span>pred = head(pred_token)</span>
<span><span>print</span>(<span>'Length of prediction:'</span>, (pred.shape[0], pred.shape[1]))</span>
<span><span>print</span>(<span>'Prediction:'</span>, <span>float</span>(pred))</span>
<span><span>Length</span> <span>of prediction: (1, 1)</span></span>
<span><span>Prediction</span>: <span>-0.5474240779876709</span></span>
<span><span><span>class</span> <span>ViT_Backbone</span>(<span>nn</span>.<span>Module</span>):</span></span>
<span> <span><span>def</span> <span>__init__</span><span>(<span>self</span>,</span></span></span><code><span> <span>preds:</span> int=<span>1</span>,</span>
<span> <span>token_len:</span> int=<span>768</span>,</span>
<span> <span>num_heads:</span> int=<span>1</span>,</span>
<span> <span>Encoding_hidden_chan_mul:</span> float=<span>4</span>.,</span>
<span> <span>depth:</span> int=<span>12</span>,</span>
<span> qkv_bias=False,</span>
<span> qk_scale=None,</span>
<span> act_layer=nn.GELU,</span>
<span><span> norm_layer=nn.LayerNorm)</span>:</span>
<span> <span>""" VisTransformer Backbone</span></span>
<span> Args:</span>
<span> preds (int): number of predictions to output</span>
<span> token_len (int): length of a token</span>
<span> num_heads(int): number of attention heads in MSA</span>
<span> Encoding_hidden_chan_mul (float): multiplier to determine the number of hidden channels (features) in the NeuralNet component of the Encoding Module</span>
<span> depth (int): number of encoding blocks in the model</span>
<span> qkv_bias (bool): determines if the qkv layer learns an addative bias</span>
<span> qk_scale (NoneFloat): value to scale the queries and keys by; </span>
<span> if None, queries and keys are scaled by ``head_dim ** -0.5``</span>
<span> act_layer(nn.modules.activation): torch neural network layer class to use as activation</span>
<span> norm_layer(nn.modules.normalization): torch neural network layer class to use as normalization</span>
<span><span> """</span><span>"""</span></span>
<span> <span>super</span>().__init_<span>_</span>()</span>
<span> <span>## Defining Parameters</span></span>
<span> <span>self</span>.num_heads = num_heads</span>
<span> <span>self</span>.Encoding_hidden_chan_mul = Encoding_hidden_chan_mul</span>
<span> <span>self</span>.depth = depth</span>
<span> <span>## Defining Token Processing Components</span></span>
<span> <span>self</span>.cls_token = nn.Parameter(torch.zeros(<span>1</span>, <span>1</span>, <span>self</span>.token_len))</span>
<span> <span>self</span>.pos_embed = nn.Parameter(data=get_sinusoid_encoding(num_tokens=<span>self</span>.num_tokens+<span>1</span>, token_len=<span>self</span>.token_len), requires_grad=False)</span>
<span> <span>## Defining Encoding blocks</span></span>
<span> <span>self</span>.blocks = nn.ModuleList([Encoding(dim = <span>self</span>.token_len, </span>
<span> num_heads = <span>self</span>.num_heads,</span>
<span> hidden_chan_mul = <span>self</span>.Encoding_hidden_chan_mul,</span>
<span> qkv_bias = qkv_bias,</span>
<span> qk_scale = qk_scale,</span>
<span> act_layer = act_layer,</span>
<span> norm_layer = norm_layer)</span>
<span> <span>for</span> i <span>in</span> range(<span>self</span>.depth)])</span>
<span> <span>## Defining Prediction Processing</span></span>
<span> <span>self</span>.norm = norm_layer(<span>self</span>.token_len)</span>
<span> <span>self</span>.head = nn.Linear(<span>self</span>.token_len, preds)</span>
<span> <span>## Make the class token sampled from a truncated normal distrobution </span></span>
<span> timm.layers.trunc_normal<span>_</span>(<span>self</span>.cls_token, std=.<span>02</span>)</span>
<span> <span><span>def</span> <span>forward</span><span>(<span>self</span>, x)</span></span>:</span>
<span> <span>## Assumes x is already tokenized</span></span>
<span> <span>## Get Batch Size</span></span>
<span> B = x.shape[<span>0</span>]</span>
<span> <span>## Concatenate Class Token</span></span>
<span> x = torch.cat((<span>self</span>.cls_token.expand(B, -<span>1</span>, -<span>1</span>), x), dim=<span>1</span>)</span>
<span> <span>## Add Positional Embedding</span></span>
<span> x = x + <span>self</span>.pos_embed</span>
<span> <span>## Run Through Encoding Blocks</span></span>
<span> <span>for</span> blk <span>in</span> <span>self</span>.<span>blocks:</span></span>
<span> x = blk(x)</span>
<span> <span>## Take Norm</span></span>
<span> x = <span>self</span>.norm(x)</span>
<span> <span>## Make Prediction on Class Token</span></span>
<span> x = <span>self</span>.head(x[<span>:</span>, <span>0</span>])</span>
<span> <span>return</span> x</span>
Through the ViT Backbone module, we can define the complete ViT model.
<span><span><span>class</span> <span>ViT_Model</span><span>(nn.Module)</span>:</span></span>
<span> <span><span>def</span> <span>__init__</span><span>(self,</span></span></span><code><span> img_size: tuple[int, int, int]=<span>(<span>1</span>, <span>400</span>, <span>100</span>)</span>,</span>
<span> patch_size: int=<span>50</span>,</span>
<span> token_len: int=<span>768</span>,</span>
<span> preds: int=<span>1</span>,</span>
<span> num_heads: int=<span>1</span>,</span>
<span> Encoding_hidden_chan_mul: float=<span>4.</span>,</span>
<span> depth: int=<span>12</span>,</span>
<span> qkv_bias=False,</span>
<span> qk_scale=None,</span>
<span> act_layer=nn.GELU,</span>
<span><span> norm_layer=nn.LayerNorm)</span>:</span>
<span> <span>""" VisTransformer Model</span></span>
<span> Args:</span>
<span> img_size (tuple[int, int, int]): size of input (channels, height, width)</span>
<span> patch_size (int): the side length of a square patch</span>
<span> token_len (int): desired length of an output token</span>
<span> preds (int): number of predictions to output</span>
<span> num_heads(int): number of attention heads in MSA</span>
<span> Encoding_hidden_chan_mul (float): multiplier to determine the number of hidden channels (features) in the NeuralNet component of the Encoding Module</span>
<span> depth (int): number of encoding blocks in the model</span>
<span> qkv_bias (bool): determines if the qkv layer learns an addative bias</span>
<span> qk_scale (NoneFloat): value to scale the queries and keys by; </span>
<span> if None, queries and keys are scaled by ``head_dim ** -0.5``</span>
<span> act_layer(nn.modules.activation): torch neural network layer class to use as activation</span>
<span> norm_layer(nn.modules.normalization): torch neural network layer class to use as normalization</span>
<span> """</span>
<span> super().__init__()</span>
<span> <span>## Defining Parameters</span></span>
<span> self.img_size = img_size</span>
<span> C, H, W = self.img_size</span>
<span> self.patch_size = patch_size</span>
<span> self.token_len = token_len</span>
<span> self.num_heads = num_heads</span>
<span> self.Encoding_hidden_chan_mul = Encoding_hidden_chan_mul</span>
<span> self.depth = depth</span>
<span> <span>## Defining Patch Embedding Module</span></span>
<span> self.patch_tokens = Patch_Tokenization(img_size,</span>
<span> patch_size,</span>
<span> token_len)</span>
<span> <span>## Defining ViT Backbone</span></span>
<span> self.backbone = ViT_Backbone(preds,</span>
<span> self.token_len,</span>
<span> self.num_heads,</span>
<span> self.Encoding_hidden_chan_mul,</span>
<span> self.depth,</span>
<span> qkv_bias,</span>
<span> qk_scale,</span>
<span> act_layer,</span>
<span> norm_layer)</span>
<span> <span>## Initialize the Weights</span></span>
<span> self.apply(self._init_weights)</span>
<span> <span><span>def</span> <span>_init_weights</span><span>(self, m)</span>:</span></span>
<span> <span>""" Initialize the weights of the linear layers & the layernorms</span></span>
<span> """</span>
<span> <span>## For Linear Layers</span></span>
<span> <span>if</span> isinstance(m, nn.Linear):</span>
<span> <span>## Weights are initialized from a truncated normal distrobution</span></span>
<span> timm.layers.trunc_normal_(m.weight, std=<span>.02</span>)</span>
<span> <span>if</span> isinstance(m, nn.Linear) <span>and</span> m.bias <span>is</span> <span>not</span> <span>None</span>:</span>
<span> <span>## If bias is present, bias is initialized at zero</span></span>
<span> nn.init.constant_(m.bias, <span>0</span>)</span>
<span> <span>## For Layernorm Layers</span></span>
<span> <span>elif</span> isinstance(m, nn.LayerNorm):</span>
<span> <span>## Weights are initialized at one</span></span>
<span> nn.init.constant_(m.weight, <span>1.0</span>)</span>
<span> <span>## Bias is initialized at zero</span></span>
<span> nn.init.constant_(m.bias, <span>0</span>)</span>
<span><span> @torch.jit.ignore ##Tell pytorch to not compile as TorchScript</span></span>
<span> <span><span>def</span> <span>no_weight_decay</span><span>(self)</span>:</span></span>
<span> <span>""" Used in Optimizer to ignore weight decay in the class token</span></span>
<span> """</span>
<span> <span>return</span> {<span>'cls_token'</span>}</span>
<span> <span><span>def</span> <span>forward</span><span>(self, x)</span>:</span></span>
<span> x = self.patch_tokens(x)</span>
<span> x = self.backbone(x)</span>
<span> <span>return</span> x</span>
In the ViT model, img_size, patch_size, and token_len define the Patch Tokenization module. They represent the size of the input image, the size of the patches into which it is divided, and the length of the token sequence generated from it. It is through this module that ViT transforms the image into a sequence of tokens that the model can process. num_heads determines the number of “heads” in the multi-head attention mechanism; Encoding_hidden_channel_mul is used to adjust the number of hidden layer channels in the encoding blocks; qkv_bias and qk_scale control the biases and scaling of the query, key, and value vectors, respectively; and act_layer represents the activation function layer, from which we can choose any activation function in torch.nn.modules.activation. Additionally, the depth parameter determines how many such encoding blocks are included in the model.
Editor / Garvey
Review / Fan Ruiqiang
Verification / Garvey
Click below
Follow us