Click the “High Technology and Industrialization” above to subscribe!
Speech recognition technology enables computers to “understand” human speech through research in signal processing and pattern recognition. In recent years, with the rapid development of deep learning technology, the accuracy of speech recognition systems has significantly improved, reaching or approaching human levels in many fields. Speech recognition can convert unstructured speech or video data into structured text, and its accuracy and efficiency effectively promote industry applications, significantly enhancing the work efficiency of professionals across multiple fields.
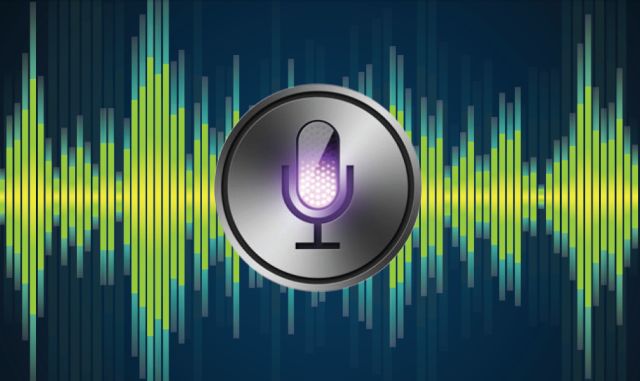
Evolution of Speech Recognition Technology
From the emergence of the prototype speech recognition system at Bell Labs in the 1950s to the industrial applications launched by companies like Google, Microsoft, IBM, and iFlytek, speech recognition has traversed a glorious path over the past sixty years. The last decade has been particularly significant in the history of speech recognition technology, as it transitioned from the mainstream HMM-GMM framework to deep learning frameworks represented by feedforward neural networks, recurrent neural networks, and convolutional neural networks, achieving excellent practical results.
Firstly, the speech recognition framework based on feedforward neural networks replaces the hybrid Gaussian model with feedforward neural networks. In this framework, all modeling units in speech recognition use the same model for modeling, which allows for a more comprehensive use of training data and easier utilization of context-related features, resulting in a revolutionary improvement in speech recognition performance. Secondly, the recurrent neural network-based speech recognition framework replaces the feedforward neural network. Given that speech inherently possesses contextual relevance, the recurrent neural network effectively remembers historical data and utilizes future information, further advancing speech recognition technology. Finally, the convolutional neural network-based speech recognition framework represents another direction for the development of speech recognition. The convolutional neural network adopts a local receptive field mechanism, providing greater robustness against variations in speaker, channel, and noise interference. Both convolutional and recurrent neural networks address different challenges in speech recognition, leading to significant advancements in the technology.
Speech recognition can be categorized into speech dictation for human-computer dialogue and speech transcription for human-to-human dialogue. Thanks to continuous breakthroughs in deep learning technology, speech dictation has been widely applied in products such as speech input, voice search, and virtual assistants, reaching maturity. However, in industry applications of speech recognition, the focus is more on human-to-human dialogue scenarios, such as meetings, interviews, and lectures, where challenges such as speaking styles, accents, and recording quality arise. Additionally, due to the unstructured nature of human dialogue, even with high accuracy in speech recognition, the readability of the transcribed text remains problematic, necessitating post-processing for sentence segmentation, paragraphing, and fluency to enhance readability.
In recent years, both academia and industry have conducted in-depth research on the transcription of human-to-human dialogue. In terms of recording quality, microphone array technology can form a pickup beam in the direction of the target person, enhancing the target speech while suppressing background noise, human voice interference, and echoes; moreover, combining microphone arrays with deep learning can further achieve noise reduction and dereverberation, making transcription in noisy, distant situations practical. Regarding speaking styles, researchers aim to bridge the modeling gap between spoken and written language by automatically introducing spoken “noise” phenomena such as repetitions, inversions, and filler words based on written language, thus generating a vast amount of spoken language corpus to address mismatches between spoken and written language. For post-processing, researchers utilize long short-term memory recurrent neural networks to segment, punctuate, and enhance the fluency of spoken text, further improving the readability of speech recognition results. Progress in addressing these technical challenges has enhanced the robustness and usability of speech recognition systems, paving the way for large-scale industry applications.
Industry Applications of Speech Recognition Technology
With the rapid development of speech recognition technology, its industrial applications are also accelerating. Depending on the demand scenarios, applications of speech recognition can be primarily categorized into real-time recording and audio-video content management.
In real-time recording, speech recognition technology plays a vital role. For instance, in the context of meeting minutes, government agencies typically require detailed records of speeches during large-scale meetings, which are often achieved by hiring stenographers. On average, large and medium-sized enterprises hold over 1,000 meetings each year, totaling more than 20 million hours, making manual meeting recording costly and demanding high standards for the recorders. In the judicial field, the total duration of various meetings, including court sessions and interrogations, exceeds 19 million hours annually. To ensure the traceability of judicial processes, there are higher demands for the completeness and accuracy of records in this field.
Through a combined hardware and software meeting recording system, it is possible to convert the speaker’s speech into text during meetings. This not only helps participants quickly understand meeting content but also employs a human-machine collaborative approach, where the meeting recorder or court staff edits, modifies, and refines the machine-generated speech recognition results. This method alleviates the workload of staff, enhances their efficiency, and further ensures the completeness and traceability of records.
In audio-video content management, such as in the media industry, millions of television programs and interview recordings are produced annually, requiring substantial human and material resources for editing subtitles or generating interview scripts. In the education sector, there are vast resources of excellent teacher micro-lectures, but effective resource management methods are lacking. In the customer service industry, the dialogue data between customer service representatives and users contain valuable information but lack effective mining methods.
The common challenge faced by these fields is that audio-video files are unstructured information sources; only by converting them into structured text can effective content management be achieved. Therefore, speech recognition plays a crucial role in these industries. For example, by providing an open speech recognition interface, users can upload audio-video files to quickly obtain relevant text content. Industry users can efficiently manage content, conduct information retrieval, and perform data mining based on the text corresponding to the audio-video files, thereby enhancing the value of audio-video files.
Development and Outlook
Reflecting on the history of speech recognition technology development, we find that breakthroughs are difficult and slow. However, by adhering to the spiral development pattern of technology, practitioners in speech recognition can identify many application breakthroughs. Nevertheless, there remains significant room for growth in both technology and application aspects of speech recognition.
In terms of technology, firstly, the overall framework of speech recognition still has considerable room for adjustment. Current solutions rely heavily on supervised data, which deviates significantly from the workings of the human brain. Therefore, many scholars are focusing on researching unsupervised methods, attempting to break free from traditional pattern recognition frameworks. This line of research is expected to yield breakthrough advancements in speech recognition in the future. Secondly, adapting speech recognition to more challenging environments, such as achieving good recognition performance in high noise, strong accents, and ultra-long distances, is a crucial direction for the practical development of speech recognition. Lastly, progress in research on personalized issues, such as mixed languages, names, place names, and technical terms, will also impact the end-user experience of speech recognition systems.
In terms of application, there are increasingly more cases of industry applications for speech transcription, but practitioners and developers still need to refine their work in various vertical application fields to address personalized issues and truly meet users’ rigid demands. For instance, in meeting minutes, while converting speech to text addresses some problems, international meetings still require the integration of translation technology to convert text from one language to another, breaking down language barriers and enhancing international communication efficiency. In audio-video content management, deep customization is still needed for different industries and recording channels to further improve the accuracy and usability of speech recognition systems. In personal applications, we speak far more words in our lives than we write. Imagine if we could convert all the words we’ve spoken into text, recording every important moment in life; this would be a meaningful endeavor, necessitating continuous exploration by speech recognition practitioners to develop more creative personal products.
Speech is the foundation of human communication and cultural transmission. In recent years, the development of industry applications for speech recognition has painted a bright picture for practitioners. China still needs to strengthen research and development efforts based on the evolution of speech recognition technology, cultivate talent, expand the market, and continue to lead industry applications of speech recognition, becoming a global leader in speech recognition technology.
This article is authored by: Liu Cong, Gao Jianqing, Wan Genshun, Chen Yimin
Author’s affiliation: iFlytek