In 2024, OCR technology is no longer a black technology! Today, let’s play with something interesting – PyTesseract, a Python tool that allows you to easily extract text from images. Whether it’s scanned documents, screenshots, or photos taken with a phone, it can help you convert them into editable text with just one click, making it incredibly convenient.
1.
Installation Steps
Installing PyTesseract is not just a simple pip command; you also need to install the Tesseract-OCR engine. Windows users, don’t worry, just follow my steps:
# First, install PyTesseract
pip install pytesseract
# Then install the image processing library Pillow
pip install Pillow
Friendly reminder: Windows users, remember to download the Tesseract-OCR installer from the official website, and add the installation path to the environment variable. Otherwise, if the code doesn’t run later, don’t blame me!
2.
Getting Started
Let’s take a look at the most basic usage, reading an image to recognize text:
from PIL import Image
import pytesseract
# Windows users may need to specify the path
# pytesseract.pytesseract.tesseract_cmd = r'C:\Program Files\Tesseract-OCR\tesseract.exe'
img = Image.open('test.png')
text = pytesseract.image_to_string(img)
print(text)
With just a few lines of code, the text in the image is extracted! However, real scenarios are not that simple. What if the image is tilted? What if there is background interference?
3.
Image Preprocessing Techniques
Sometimes the image quality is poor, and the recognition rate is very low, so we need to beautify the image first:
import cv2
import numpy as np
def preprocess_image(image_path):
img = cv2.imread(image_path)
# Convert to grayscale
gray = cv2.cvtColor(img, cv2.COLOR_BGR2GRAY)
# Binarization
_, binary = cv2.threshold(gray, 150, 255, cv2.THRESH_BINARY + cv2.THRESH_OTSU)
# Denoising
denoised = cv2.fastNlMeansDenoising(binary)
return denoised
This code converts the image to grayscale, then binarizes it, and finally denoises it. The effect is excellent!
4.
Advanced Features
PyTesseract can not only recognize text but also tell you where the text is and what language it is:
# Get text position
boxes = pytesseract.image_to_boxes(img)
# Get recognition confidence
data = pytesseract.image_to_data(img, output_type=pytesseract.Output.DICT)
# Supports Chinese!
text = pytesseract.image_to_string(img, lang='chi_sim')
Friendly reminder: Want to recognize Chinese? You need to download the Chinese data package separately, or it will be awkward…
5.
Practical Tips
In actual projects, I found these tips particularly useful:
-
Image resolution too high? Compress it first; it will speed things up a lot. -
Recognition effect not ideal? Try adjusting the contrast and brightness. -
Encountering tables? Use <span>image_to_data</span>
to get more detailed layout information.
# Compress image size
def resize_image(image, scale_percent=50):
width = int(image.shape[1] * scale_percent / 100)
height = int(image.shape[0] * scale_percent / 100)
return cv2.resize(image, (width, height))
PyTesseract is a pretty reliable tool, and combined with OpenCV’s image processing, it can handle most OCR needs. Remember to handle exceptions well when writing code, as OCR can sometimes fail, and you don’t want your program to crash.
No matter how well the code is written, it is essential to pay attention to real scenarios. Sometimes a simple image preprocessing technique can yield better results than piling up complex code. OCR technology is developing rapidly; new tools like paddleocr are also pretty good. If you’re interested, you can try them all.
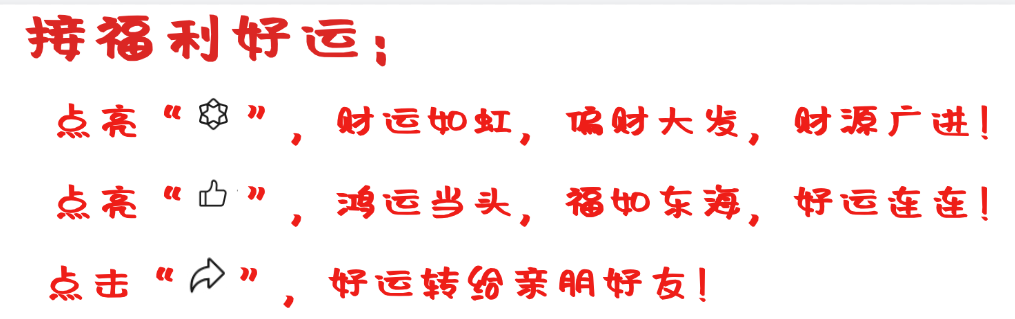