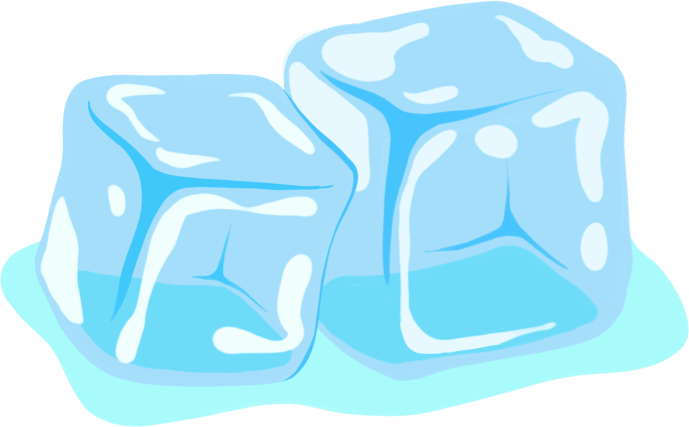
Click <天津大学学报> to follow us
Deng Bin, Zhang Nan, Wang Jiang, Ge Leijiao
Tianjin University, School of Electrical Automation and Information Engineering, Tianjin 300072
1 Citation Format
Deng Bin, Zhang Nan, Wang Jiang, et al. Medium- and Long-Term Power Load Forecasting Method Based on LTC-RNN Model [J]. Journal of Tianjin University (Science and Technology), 2022, 55(10): 1026-1133.
Deng Bin, Zhang Nan, Wang Jiang, et al. Medium- and Long-Term Power Load Forecasting Method Based on LTC-RNN Model [J]. Journal of Tianjin University (Science and Technology), 2022, 55(10): 1026-1133.
2 Abstract
In response to the challenge of low accuracy in medium- and long-term power load forecasting affected by multiple uncertain factors such as weather, temperature, and holidays, this paper proposes a method based on a liquid time constant recursive neural network to achieve accurate forecasting of medium- and long-term power loads, laying a solid foundation for power system planning and design. The liquid time constant recursive neural network improves the state equation of neurons using membrane integrators and employs a semi-implicit Euler algorithm to complete the iterative update of neuron states, addressing the weak self-correction ability and the tendency to fall into local optima in recursive neural networks. This paper uses external influencing factors such as weather, temperature, and holidays as input to construct a medium- and long-term power load forecasting model based on a recursive neural network, and obtains the input-output mapping relationship through extended calculations in the hidden layers, achieving model training through the backpropagation algorithm over time to optimize the parameters of the neural network. In experiments, using actual grid data from a northern region as an example, the accuracy and efficiency of this forecasting method are validated. The research results show that the prediction accuracy of the liquid time constant recursive neural network for medium- and long-term power loads can reach 95.3%. Compared to Long Short-Term Memory (LSTM) and continuous-time recursive neural networks, this model exhibits more stable training results and higher prediction accuracy.
[Keywords]: Medium- and Long-Term Power Load Forecasting; Recursive Neural Network; Power System
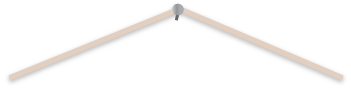

Statement
This article is an original work, and all text and images are copyrighted by the editorial department of the Journal of Tianjin University (Science and Technology). According to the latest regulations from the National Copyright Administration, for reprinting or excerpting our works in paper media, websites, Weibo, or WeChat public accounts, please contact our editorial department in advance. Individuals may share this article as it appears in WeChat.
Contact Us
Address: 412 East Annex Building, Tianjin University, 92 Weijin Road, Nankai District, Tianjin 300072
Phone: 022-27890392, 022-87402145
Website: http://xbzrb.tju.edu.cn/#/
Click the lower left corner “Read Original” to view the content of this issue