Source: Xixiaoyao Technology
Author | Richard
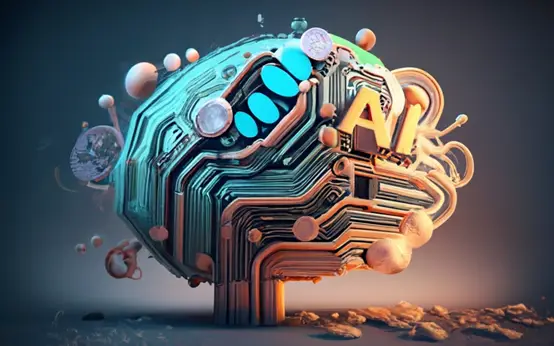
Since the advent of GPT-4, large models seem to have become increasingly intelligent, possessing an “encyclopedic” knowledge base. But are they really approaching human intelligence?
Not quite. Large models still have significant shortcomings in knowledge integration and long-term memory, which are precisely the strengths of the human brain. The human brain can continually integrate new knowledge, forming a robust long-term memory that supports our thinking and decision-making. So how can large models achieve efficient knowledge integration and long-term memory like the human brain?
A group of scientists from Ohio State University and Stanford University has proposed an interesting idea: to give artificial intelligence a “memory brain” similar to the human hippocampus. They designed a model named HippoRAG, which mimics the role of the hippocampus in long-term memory, enabling efficient integration and searching of knowledge like the human brain. Experiments show that this “memory brain” can significantly improve performance on tasks requiring knowledge integration, such as multi-hop question answering. This may indicate a new direction for endowing large models with “human-like” memory capabilities.
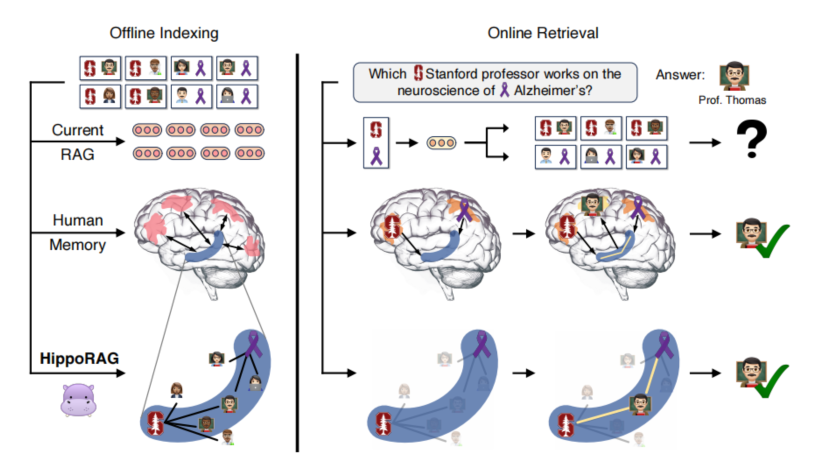
Paper Title: HippoRAG: Neurobiologically Inspired Long-Term Memory for Large Language Models
Paper Link: https://arxiv.org/pdf/2405.14831
In recent years, large AI models have demonstrated remarkable capabilities across various tasks, seemingly getting closer to the dream of “general artificial intelligence.” However, large models still have significant flaws in knowledge integration and long-term memory, making it difficult to compete with the efficiency of the human brain.
Recently, scientists from Ohio State University and Stanford University proposed an interesting idea: to give large models a “memory operating system” similar to the human hippocampus. They designed a new retrieval-augmented model named HippoRAG, inspired by the key role of the hippocampus in human memory. Experiments show that large models equipped with this “brain-like” memory system exhibit astonishing performance improvements on various tasks requiring knowledge integration.
The birth of HippoRAG opens up a new path for endowing large models with “brain-like” knowledge integration and long-term memory capabilities. This groundbreaking work is expected to help large models further tap their potential, moving closer to human intelligence.
Hippocampal Memory Techniques
The design inspiration for HippoRAG comes from the hippocampus in the human brain. The hippocampus is an important structure located in the medial temporal lobe of the brain, playing a key role in learning and memory processes. Scientists have found that the hippocampus seems to be responsible for indexing new memories during their formation and linking these memory indices together. This allows the human brain to efficiently store, integrate, and retrieve different knowledge, forming lasting long-term memories.
Inspired by this, researchers designed a “memory mechanism” similar to the hippocampus. They utilize the large language model to act as the neocortex, responsible for processing information; a knowledge graph serves as the “memory index,” and a retrieval model is introduced to connect the language model and knowledge graph, simulating the function of the entorhinal cortex. When the model receives a new query, it first extracts key concepts from the query, then applies the Personalized PageRank algorithm on the knowledge graph for concept expansion and retrieval, simulating the associative memory capabilities of the hippocampus. Finally, the model ranks and retrieves passages based on the importance of nodes, as if performing “pattern completion.”
The following diagram illustrates the retrieval process of HippoRAG. It first extracts key concepts from the query, such as “Stanford” and “Alzheimer’s,” and then uses the retriever to find corresponding nodes in the knowledge graph. It then explores the graph using the Personalized PageRank algorithm to find the most relevant nodes, such as “Thomas Sudhof,” and finally ranks the retrieved information based on the importance of the nodes, successfully retrieving the most relevant content.
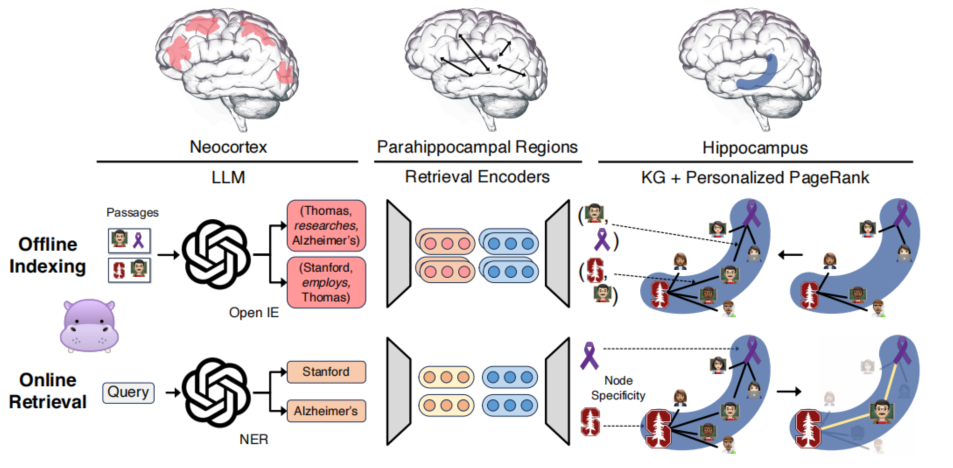
Researchers also introduced the concept of “node specificity” to aid retrieval by leveraging the uniqueness of nodes in the knowledge graph, which can be seen as a neuroscientifically reasonable “inverse document frequency” signal, allowing HippoRAG to weigh the importance of concepts.
Performance Evaluation of HippoRAG
To investigate HippoRAG’s knowledge integration capabilities, researchers selected three challenging multi-hop question answering datasets: MuSiQue, 2WikiMultiHopQA, and HotpotQA. These datasets require integrating information from multiple supporting paragraphs to answer questions, placing high demands on knowledge integration capabilities.
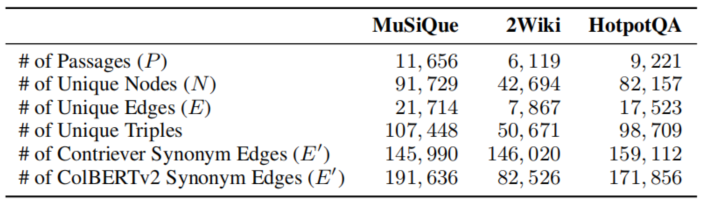
The table below shows the performance comparison of various models on the three datasets. It can be seen that:
-
In single-step retrieval experiments, HippoRAG significantly outperformed existing retrieval models on MuSiQue and 2WikiMultiHopQA, with F1 scores improving by 3-20 percentage points; -
It also achieved comparable results to the current best model on HotpotQA.
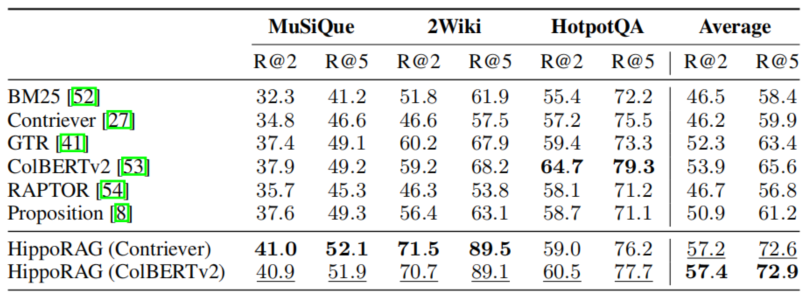
Notably, in multi-step retrieval experiments, when HippoRAG is combined with the iterative retrieval method IRCoT, the improvements are even more significant, with F1 scores increasing by 3-19 percentage points across the three datasets.
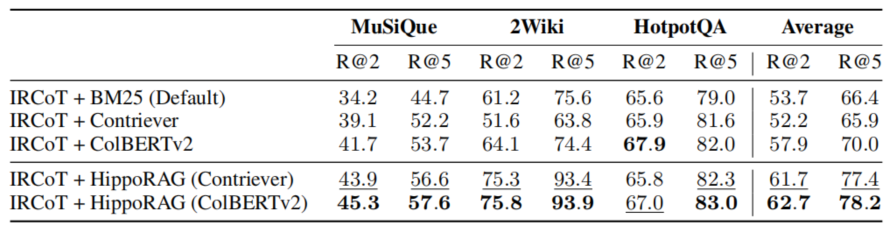
Even more surprisingly, the performance of HippoRAG in single-step retrieval is already close to or exceeds that of IRCoT’s multi-step iterative retrieval, while the computational cost is significantly lower. As shown in the figure below, the cost of calling the GPT-3.5 Turbo API for online retrieval with HippoRAG is only one-tenth to one-thirtieth of that of IRCoT, and the retrieval speed is improved by 6-13 times. This means that HippoRAG can effectively tackle complex knowledge integration challenges at a lower computational cost.
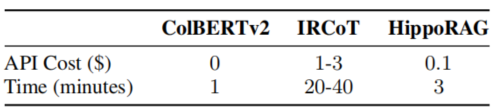
Overall experimental results indicate that the brain-like memory mechanism employed by HippoRAG has achieved significant success in endowing large models with knowledge integration and long-term memory capabilities. It not only reached new performance heights on existing multi-hop question answering tasks but also demonstrated the potential to handle more complex problems.
So, what gives HippoRAG such powerful capabilities? To gain a deeper understanding of its working mechanism, researchers conducted a series of ablation experiments and analyses. As shown in the figure below, they examined the impact of different OpenIE tools, graph traversal algorithms, and key design components on HippoRAG’s performance.
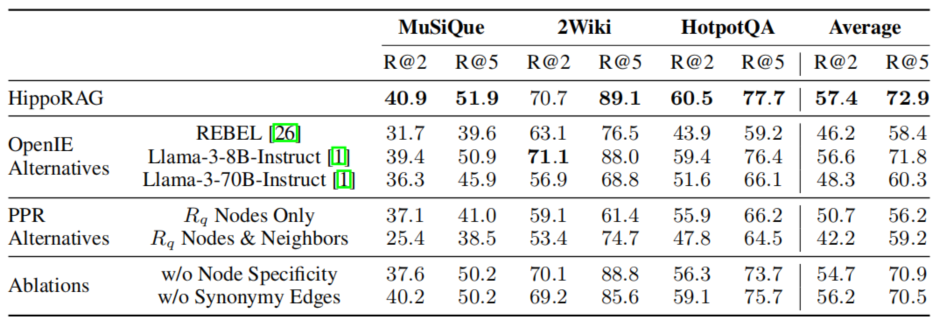
The experiments found that replacing GPT-3.5 with other OpenIE tools like REBEL would lead to a significant drop in HippoRAG’s performance. This reveals the unique advantage of GPT-3.5 in flexibly constructing knowledge graphs. When replacing GPT-3.5 with open-source language models like Llama-3, especially its 8B version, HippoRAG’s performance was very close to that of GPT-3.5. This finding suggests that we can use more economical open-source models to train HippoRAG, potentially expanding its application scenarios.
In terms of the choice of graph traversal algorithm, Personalized PageRank showed significant advantages. When using other simple query-node-based traversal methods, HippoRAG’s performance would drop significantly. This confirms the unique role of Personalized PageRank in capturing complex associations between queries and knowledge graphs.
Additionally, ablation experiments confirmed the value of two key designs: node specificity and synonym connections. Removing node specificity led to performance declines on MuSiQue and HotpotQA, while removing synonym connections significantly affected HippoRAG’s performance on 2WikiMultiHopQA. This indicates that node specificity helps HippoRAG weigh the importance of different concepts, while synonym connections facilitate entity alignment and knowledge integration.
Conclusion and Outlook
The HippoRAG method opens a new door to introducing neuroscience knowledge into the optimization of large models. By mimicking the memory mechanism of the human hippocampus, HippoRAG endows large language models with efficient knowledge integration and long-term memory capabilities, achieving significant performance improvements on complex tasks such as multi-hop question answering, and showing potential for solving new types of problems.
This new brain-like paradigm of HippoRAG has many questions and application prospects worth exploring further, such as optimizing model components, expanding application task domains, and integrating domain knowledge to build vertical intelligent assistants. However, truly achieving “human-like” AI systems still faces many technical and theoretical challenges, requiring deeper interdisciplinary research with neuroscience, cognitive science, and other fields.