In February 2024, OpenAI released its first video generation model, Sora. Users can generate high-definition videos with smooth scene transitions and clear details by simply inputting a text segment. Compared to AI-generated videos from a year ago, Sora has achieved qualitative improvements across various dimensions. This breakthrough has once again brought AIGC into the public eye. AIGC refers to artificial intelligence systems trained on large amounts of data, capable of generating content such as text, audio, images, and code based on personalized user instructions. Since the launch of ChatGPT in 2022, generative AI has shown enormous potential and value across multiple application scenarios, including gaming, film, publishing, finance, and digital humans. According to incomplete statistics, global AIGC industry financing exceeded 190 billion yuan in 2023, with companies in this sector securing funding almost every month. For example, in June 2023, Runway raised a new round of $141 million from investors including Google, NVIDIA, and Salesforce; meanwhile, Runway’s strong competitor Pika completed three rounds of financing within just six months, totaling $55 million.
This article will analyze the direction of AIGC commercialization applications and industry development trends based on the current state of the AIGC industry ecosystem and technological development paths.
ONE
Overview of Industry Ecosystem
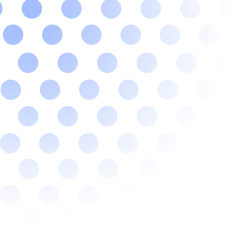
Industry Ecosystem Map: The foundational layer represented by the data sector needs breakthroughs, the model layer occupies a core position, and the application layer is flourishing
Overall, the current AIGC industry ecosystem can be divided into three parts: the upstream infrastructure layer, the midstream model layer, and the downstream application layer. The infrastructure layer includes algorithms foundational platforms such as data, computing power, and model development training platforms; the model layer includes foundational general large models, intermediate layer models, and open-source communities; the application layer has developed strategy generation and cross-modal generation based on four types of modalities: text, audio, images, and video, achieving commercial applications in various industries such as finance, data analysis, and design.
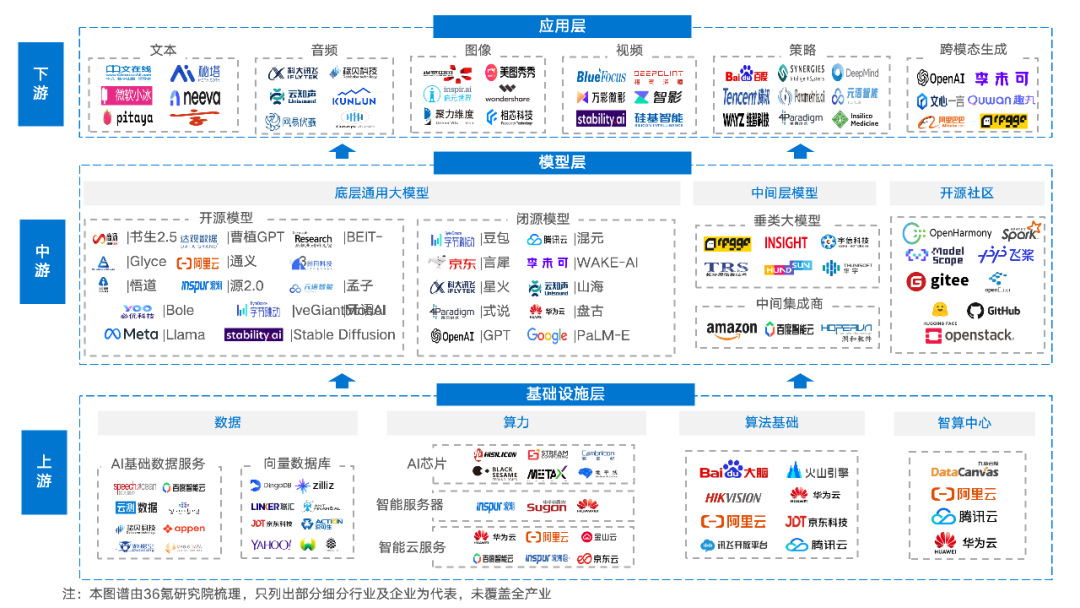
Illustration: AIGC Industry Ecosystem Map
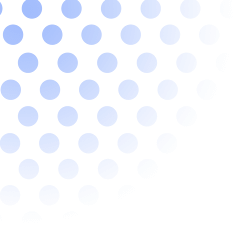
Infrastructure Layer: Data Service Sector Becomes New Growth Area, Computing Power and Algorithm Industry Ecosystem Structure Relatively Certain
AIGC has high requirements for the volume of training data, the industry field it belongs to, corresponding vertical businesses, and granularity. For pre-trained large models, multimodal datasets are crucial. Additionally, to ensure that training questions and outputs meet expectations, data providers need to ensure the immediacy and effectiveness of the data. Currently, the world’s largest open-source cross-modal database is LAION-5B, and the world’s first hundred million-level Chinese multimodal dataset, “Wukong,” was open-sourced by Huawei’s Noah’s Ark Lab.
Since various large models entered the public eye, the token size limit has troubled many developers and users. For instance, with GPT, when a user sends a command, the program automatically combines the recent dialogue records (based on a dialogue word limit of 4096 tokens) into the final question and sends it to ChatGPT. Once the user’s dialogue memory exceeds 4096 tokens, it becomes difficult for the model to incorporate previous dialogue content into its logical reasoning, leading to AI hallucinations when facing more complex tasks.
In this context, developers are continuously seeking new solutions, and vector databases have become one of the popular solutions. The core concept of vector databases is to convert data into vectors stored in the database; when a user inputs a question, it also converts the question into a vector and searches for the most similar vectors and contexts in the database, ultimately returning text to the user. This not only significantly reduces the computational load on GPT, improving response speed but also lowers costs, supports multimodal data, and bypasses GPT’s token limits. As overseas vector databases such as Weaviate and MongoDB attract capital attention, domestic giants like Tencent and JD.com are also actively laying out in this field.
Compared to the data sector, the supply side of computing power and algorithm foundations in China is still dominated by leading enterprises, with relatively fewer opportunities for startups. However, intelligent computing centers that provide required computing power services, data services, and algorithm services for the application layer based on artificial intelligence computing architecture have become one of the new types of public computing power infrastructure.
For example, AIDC OS is a dedicated AI operating system developed by JiuZhang Cloud’s DataCanvas. It targets large-scale computing power in intelligent computing centers and mid-to-large-sized enterprises’ internal intelligent computing clusters, providing management and unified scheduling of intelligent computing resources, business operation support for intelligent computing services, and core capabilities for building, training, and inferring AI models. AIDC OS has upgraded the operational capabilities of computing power operators from bare computing power device operation to AI large model operation, and with its open compatibility for various heterogeneous computing power and AI applications, AIDC OS has successfully enhanced the added value of computing power assets.
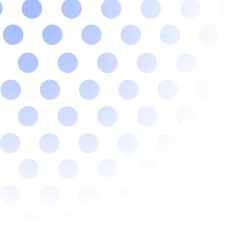
Model Layer: Domestic Market Players Mainly Focused on Foundational General Large Models, Few Intermediate Layer Players
AIGC foundational general large models can be divided into open-source and closed-source categories. Closed-source models are generally accessed through paid APIs or limited trial interfaces, with foreign closed-source models including OpenAI’s GPT model and Google’s PaLM-E model. Domestic closed-source model vendors started later but have rapidly improved in multimodal interaction capabilities and integration with smart hardware. For example, the WAKE-AI large model developed by Li Weike Technology has multimodal interaction capabilities such as text generation, language understanding, image recognition, and video generation, specifically optimized for future AI+ terminals. Currently, the WAKE-AI large model is temporarily used on Li Weike Technology’s smart terminal—AI glasses and XR glasses. In the future, Li Weike Technology will open this AI platform, allowing more developers to quickly and cost-effectively deploy or customize multimodal AI on various terminals using low-code or no-code methods.
Open-source models use publicly available model source codes and datasets, allowing anyone to view or modify the source code, such as Stability AI’s open-source Stable Diffusion, Meta’s open-source Llamax, xAI’s open-source Grok-1, and China’s Zhiyuan’s open-source Aquila. In comparison, closed-source models have the advantage of lower initial investment costs and stable operation; open-source models, however, offer higher data privacy security guarantees based on private deployment and faster iteration and updates. Currently, most domestic large model development enterprises or institutions are committed to developing cross-modal large models, such as Tencent’s Hunyuan AI and Baidu’s Wenxin large model, which can perform cross-modal generation, but an open-source ecosystem has not yet formed widely.
The intermediate layer model market players can be roughly divided into vertical large models and intermediate integrators. Vertical large models require a high understanding of the business of vertical industries and resource accumulation, while intermediate integrators are responsible for combining multiple model interfaces to form new overall models. For example, AI game engine company RPGGO can assist individual creators in simplifying development processes and maximizing creative output based on its self-developed game engine, Zagii Engine; for game studios, RPGGO can provide API linkage to enhance game development efficiency.
In terms of strategic cooperation or product layout, domestic foundational large model vendors are actively laying out intermediate and terminal application layers to provide capability exports and data entry for their foundational large model products, such as Li Weike Technology, which is preparing for multimodal AI platforms targeting future smart terminals.
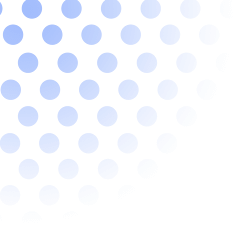
Application Layer: Text Generation Has a Longer Development History, Cross-Modal Generation Has the Highest Potential
The application layer of the AIGC industry is mostly based on model capabilities and insights into user demands, directly serving B-end or C-end customers, which can be simply understood as various tools in the mobile internet era, with significant potential for future growth, allowing many startups to participate.
According to modality classification, the application layer can be divided into text generation, audio generation, image generation, video generation, cross-modal generation, and strategy generation. Due to the long development history of NLP technology, text generation is the longest-standing and most mature application track. In this wave of AIGC development, cross-modal generation is expected to bring the most new application scenarios. Among them, text-to-image generation, text-to-video generation, and image/video-to-text generation products have already emerged, especially text-to-image generation, such as Stability AI, which has proven its user base globally.
According to estimates by the Quantum Bit Think Tank on the technical maturity, application maturity, and future market size of different modalities and application scenarios, currently, among text generation, the text-assisted generation track has the largest scale potential; among cross-modal generation, the text-to-image/video track has the largest scale potential.
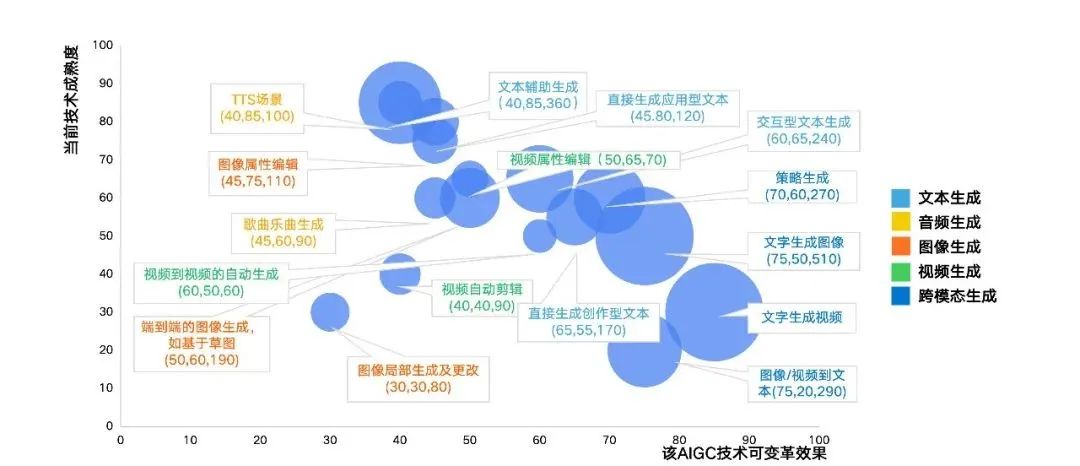
Illustration: AIGC Industry Application Layer Development Forecast for Different Tracks (Circle Size Represents Estimated Market Size for 2030)
Data Source: Quantum Bit Think Tank, Organized by 36Kr Research Institute
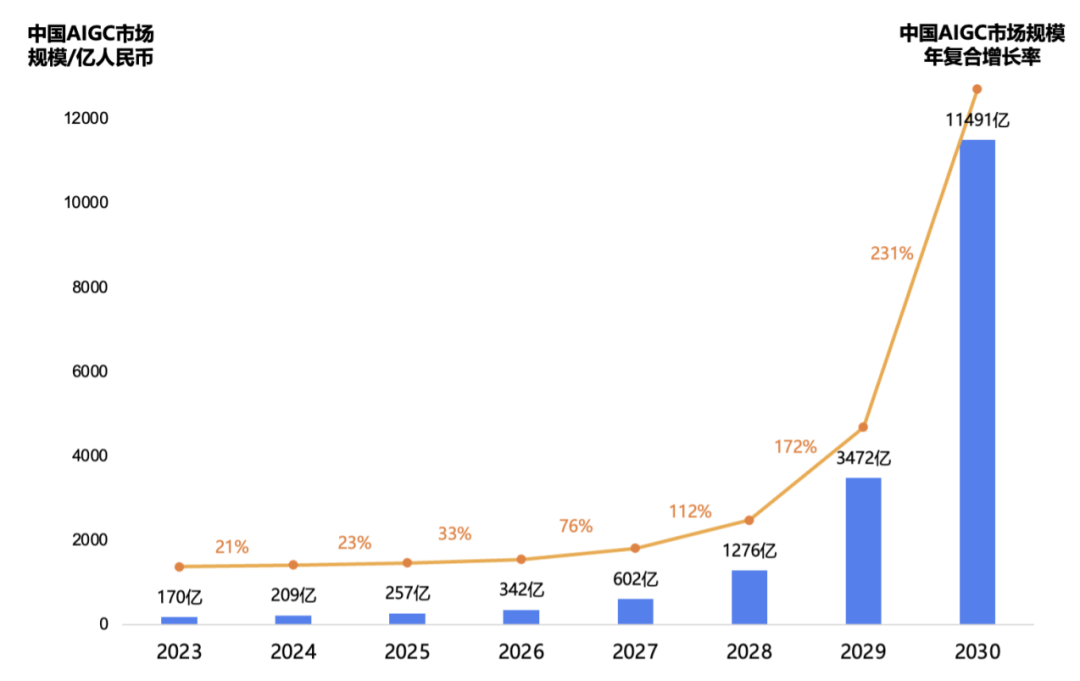
By 2030, China’s AIGC Market Size Will Reach Trillions
According to data from the Quantum Bit Think Tank, China’s AIGC market size in 2023 is about 17 billion yuan, and it is expected to maintain a growth rate of around 25% before 2025. By 2025, the market size will reach 25.7 billion yuan. Starting in 2025, as foundational large models gradually open up, the intermediate and application layers will experience explosive growth, driving rapid market size growth in the AIGC industry, with an average annual compound growth rate exceeding 70%. By 2027, China’s AIGC market size will exceed 60 billion yuan. Starting in 2028, the AIGC industry ecosystem will become more mature, achieving commercial applications across various industries. By 2030, the market size will exceed one trillion yuan.
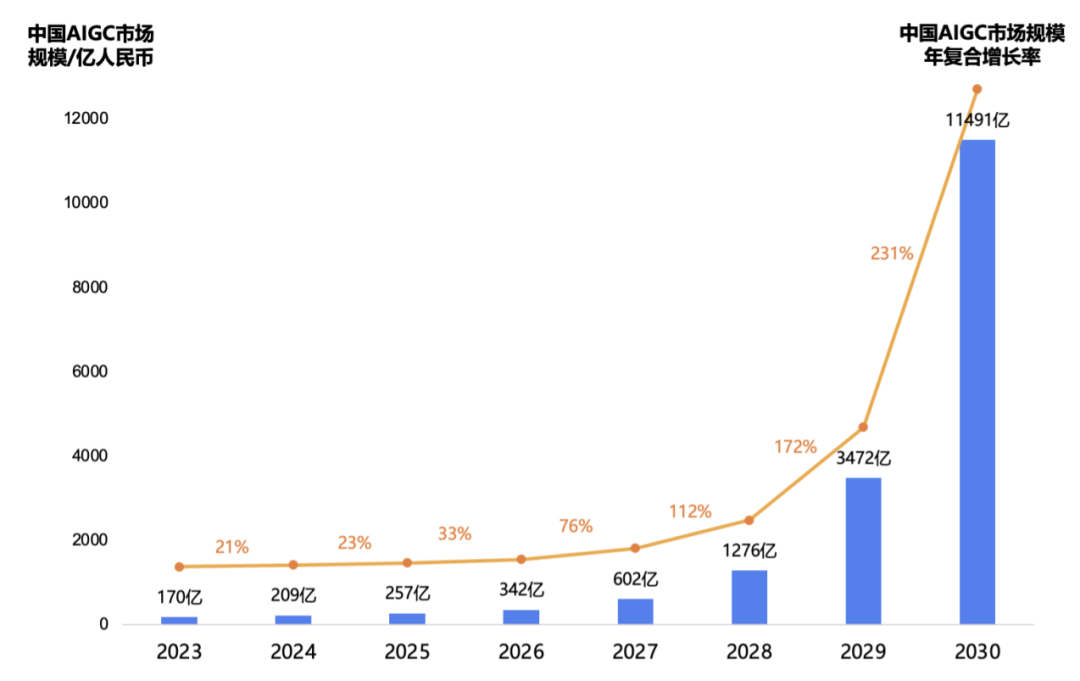
Illustration: Forecast Data for China’s AIGC Industry Market Size from 2023 to 2030. Data Source: Quantum Bit Think Tank, Organized by 36Kr Research Institute
TWO
Frontier Technology Analysis
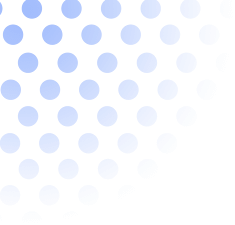
Multimodal Development Has Become an Industry Consensus, Text End Technology Path Has Converged to LLM
According to the number of data types processed, AI models can be divided into single-modal and multimodal categories: single-modal can only process one type of data, such as text, audio, or images; multimodal can process two or more types of data. Compared to single-modal, multimodal large models have significant advantages in input and output: the complementary nature of different modal input data helps rapidly expand the capabilities of general large models, multimodal data input has a lower threshold and less data loss, and it can greatly enhance user application experience; the output of multimodal data eliminates the need for integrating multiple models, making commercial implementation easier.
Currently, the development of AIGC large models from single-modal to multimodal has become an industry consensus. Under the influence of applications like ChatGPT (launched in November 2022) and the image generation representative application Midjourney V5 (launched in March 2023), text and image generation applications experienced explosive growth in 2023. On February 16, 2024, OpenAI released the text-to-video application Sora, making video generation a new industry hotspot, and it is expected that 2024 will see heightened technological and capital attention.
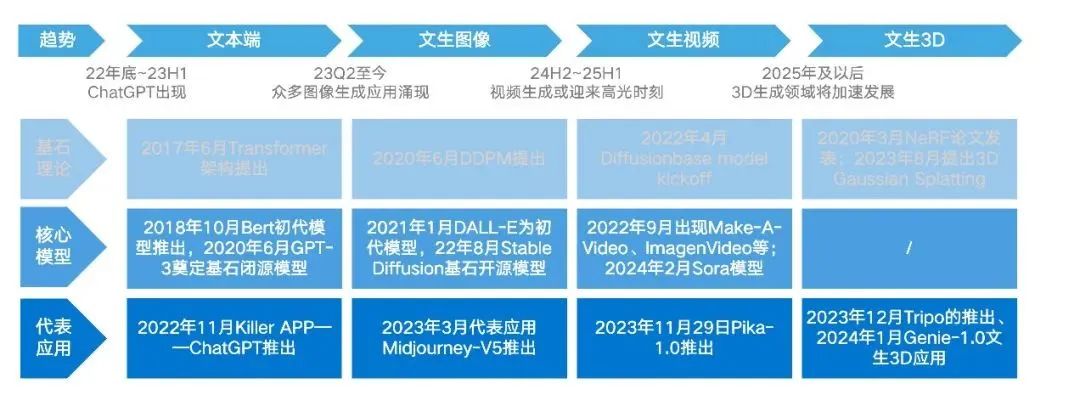
Illustration: Multimodal Large Model Technology Development Status
Data Source: Southwest Securities, Public Market Data, Organized by 36Kr Research Institute
Currently, pre-trained models based on the Transformer structure are the mainstream training method for multimodal large models. For example, Google’s GEMINI pre-trains on different modalities and fine-tunes using additional multimodal data to enhance its effectiveness. With the development of text generation large models, LLM has become a definitive technological path. Through extension, the performance of LLM can achieve significant improvements in quantitative indicators such as perplexity (the fluency of generated text), as long as it is exposed to diverse language patterns and structures during training, LLM can faithfully imitate and reproduce these patterns.
However, multimodal technology faces the dilemma of impending data depletion. The cost of annotating different types of data varies; for example, visual modal data collection costs are generally higher than text data, leading to a significant scarcity of multimodal datasets (especially high-quality datasets) compared to text datasets. Epochai data shows that, against the backdrop of rapid development of AIGC large models, high-quality language data may be exhausted before 2026, while low-quality language data may also face depletion within the next 20 years.
To address the data depletion issue, AI synthetic data has emerged, such as structured data companies like Mostly AI and unstructured data companies like DataGen. The former can generate anonymized datasets with characteristics comparable to real data predictions, while the latter provides a self-service platform for synthetic datasets for computer vision teams. AI synthetic data adapts to the data modal combinations of multimodal models, and data acquisition is faster, effectively increasing data volume.
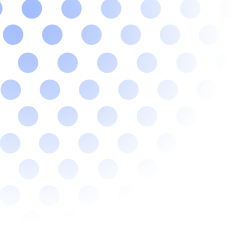
Path Comparison: Diffusion Models Dominate, Auto-regressive Models Still Have Potential
AI-generated videos and AI-generated images share a similar underlying technological framework, primarily including Generative Adversarial Networks (GAN), Auto-regressive Models, and Diffusion Models. Currently, diffusion models have become the mainstream model for AI-generated videos.
(1) Generative Adversarial Networks (GAN)
GAN is an early mainstream image generation model that improves the model’s image generation and discrimination capabilities through adversarial training between a generator and a discriminator, making the generated network’s data approach real data and thus images approach real images. Compared to other models, GAN has a smaller number of model parameters, making it more adept at modeling single or multiple object categories. However, the downside is that GAN’s training process lacks stability, leading to a lack of diversity in generated images, and it has gradually been replaced by auto-regressive models and diffusion models.
(2) Auto-regressive Models
Auto-regressive models use Transformers for auto-regressive image generation. The overall framework of Transformers is mainly divided into Encoder and Decoder, which can simulate spatial relationships between pixels and high-level attributes (texture, semantics, and scale) using multi-head self-attention mechanisms for encoding and decoding. Compared to GAN, auto-regressive models have clear density modeling and stable training advantages, generating more coherent and natural videos through frame-to-frame connections. However, due to the typically larger number of parameters in auto-regressive models compared to diffusion models, their requirements for computational resources and datasets are often higher than those of other models, thus limiting them due to resource and time constraints. Nevertheless, due to their potential for greater parameter expansion, image generation and video generation auto-regressive models are expected to draw on Transformers’ experiences in the text field of LLM, achieving “miracles” through cross-modal and large-scale training.
(3) Diffusion Models
In simple terms, diffusion models define a Markov chain of diffusion steps, continuously adding random noise to data until pure Gaussian noise is obtained, and then learning the reverse diffusion process to generate images through reverse denoising inference, optimizing the process by perturbing the distribution of data and restoring data distribution. For example, Sora consists of three main Transformer components: Visual Encoder, Diffusion Transformer, and Transformer Decoder. During training, given an original video X, the Visual Encoder compresses the video into a lower-dimensional latent space, which is then trained in the latent space using the Diffusion Transformer based on the diffusion model, adding noise and then denoising, gradually optimizing until the generated temporally and spatially compressed latent representation is mapped back to pixel space through the Transformer decoder, resulting in video X1. Due to its higher computational efficiency, lower costs, and ability to achieve high-quality images when processing data (compression/enlargement), diffusion models have gradually become the mainstream technological path in text-to-image and text-to-video fields.
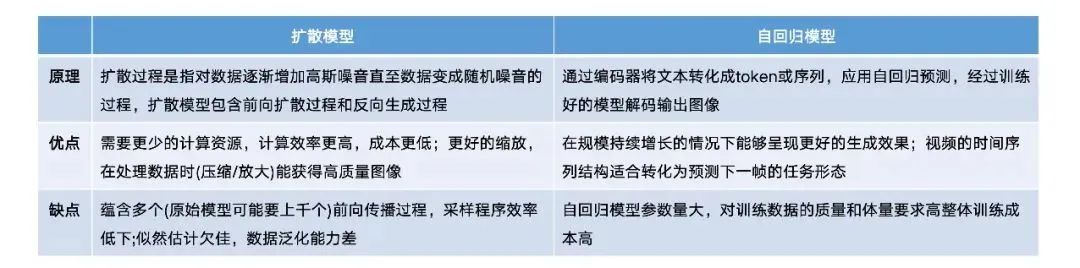
Illustration: Diffusion Models vs. Auto-regressive Models
Data Source: Public Market Data, Organized by 36Kr Research Institute
THREE
Application Overview
With the release of products like ChatGPT, Wenxin Yiyan, and Sora, the AIGC coverage scenarios are becoming increasingly rich, and performance effects are gradually maturing. Opportunities and challenges coexist; AIGC brings development opportunities to industries while creating more new application scenarios and business models, but it also comes with challenges that need to be addressed.
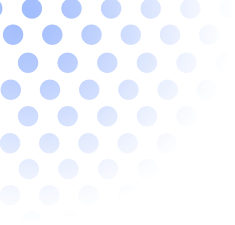
For ToB enterprises, AIGC can be organically integrated with their existing businesses to achieve cost reduction and efficiency improvement, bringing new opportunities to industries such as digital humans, SaaS, digital design, and finance
Digital Humans. The development of virtual digital humans is closely related to breakthroughs in foundational technologies across multiple fields such as AI, CG, and virtual reality. The integration of AIGC with digital humans endows virtual beings with more “agility” and “vitality,” enabling their deployment in more application scenarios. On one hand, AIGC technology can transform static photos into dynamic videos and achieve video effects such as face swapping and expression changes, making virtual beings more vivid and realistic; on the other hand, AI technology enhances the multimodal interaction capabilities of virtual beings, allowing for automatic interaction without human intervention, giving virtual beings an inherent “thinking” ability, thus accelerating their application in more fields. Moreover, AI technology is expected to achieve full-process automation from creation and driving to content generation, reducing development costs for enterprises. For example, Quwan Technology has initially built a high-naturalness virtual digital human generation technology platform, which can generate virtual digital humans with over 90% facial similarity in about 10 seconds using one or several photos, with short time consumption, low costs, and multimodal interaction capabilities, lowering the technical threshold and economic burden for ordinary users, enabling applications in popular science education, live retail, gaming, and animation.
SaaS. In the face of a continuously evolving market environment, maintaining digital operations on the business side and smooth upstream and downstream connections has become an inevitable choice for more enterprises, meaning the SaaS industry needs to enhance its intelligence level to provide enterprises with services that can quickly respond, interact, and analyze decision-making value. In customer management scenarios, AIGC’s text generation model can serve as a chatbot, quickly providing feedback based on customer communication content, offering personalized interactions, and proactively providing other related services beyond queries, making SaaS software more accessible and user-friendly. In business process automation scenarios, AIGC can comprehensively manage enterprise business processes through simple instructions, enhancing work efficiency. For example, in financial management, it can integrate and analyze financial data, providing comprehensive financial reports and analyses; in marketing, it can dynamically generate personalized customer emails and advertisements; in supply chain management, it can automatically process upstream and downstream documents and data entry; in human resources, it can realize intelligent interview and salary assessment automation.
Digital Design. With the gradual maturity of foundational technologies such as multimodal pre-trained large models, AIGC has shown stronger capabilities in audio, image, and video generation, with applications becoming increasingly widespread. On one hand, image generation is rapidly applied in digital design fields such as industrial design, graphic design, illustration design, and game animation production. In the early stages of work, AIGC can assist in collecting materials and quickly generating drafts; in later stages, users can achieve functions such as color adjustment, composition adjustment, image editing, and style adjustment through text instructions, lowering the creative threshold while reducing basic mechanical labor. On the other hand, video generation can provide more intuitive demonstration effects in industries such as architectural design, industrial design, and game design, significantly shortening work hours.
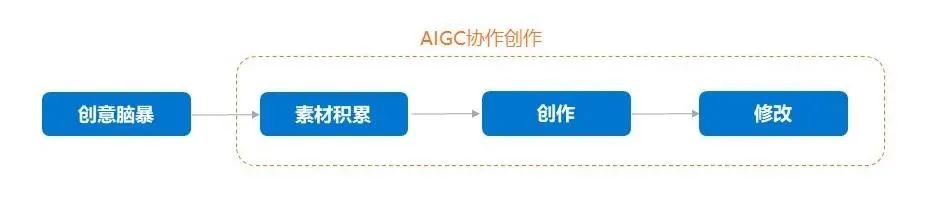
Illustration: AIGC Integrated into Digital Design Workflow
Finance. In the face of fierce market competition, the traditional financial industry has struggled to meet consumers’ personalized needs. The financial industry is resource-intensive, and leveraging AIGC’s analytical and generative capabilities can enhance service efficiency, optimize business processes, and provide more convenient customer-centric products and services. Specifically, AIGC is mainly applied in risk assessment, quantitative trading, and counter service handling. In the risk assessment phase, AIGC can quickly analyze scattered, multidimensional trading data and behavior patterns, accurately monitor and identify potential risks and detect fraud, thus improving risk control accuracy. In the counter service phase, AIGC can recommend more suitable products and customized financial services based on customer needs and profiles, thereby improving customer satisfaction.
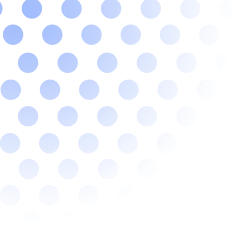
For ToC enterprises, AIGC will help industries such as gaming, film, and publishing improve content production efficiency and enhance consumer experience
Publishing. For the content-centric publishing industry, AIGC will trigger a paradigm shift in content production. On one hand, AIGC replaces users as content producers, rapidly increasing content production efficiency; on the other hand, AIGC can assist in completing editing tasks, saving editing time and freeing up human resources. Specifically, in the content production phase, AIGC’s text output capabilities assist authors in completing content creation; as technology develops, it may even directly create content with unique writing styles. Currently, some novel websites have launched AIGC-assisted creation features, allowing authors to automatically generate content by inputting special keywords and providing inspiration. In the editing phase, AIGC can quickly complete article proofreading by capturing trending news and events, selecting topics based on automatic analysis while using text recognition and deep learning models, thus improving editing efficiency.
Games. In the context of increasingly fierce competition and more segmented player preferences, the integration of AIGC with games optimizes the player experience comprehensively in terms of content, graphics, and gameplay. In terms of content and gameplay, AIGC enhances NPC dialogue logic, refines tone, expressions, and body language, and builds emotional connections between the environment and NPCs, enhancing player interaction and providing a high degree of immersive experience. On the other hand, by inputting goals, scenes, characters, and other information, AIGC can generate gameplay copy and provide suggestions on mechanisms and storylines, balancing and enriching gameplay to enhance its fun. Additionally, AIGC can assist in generating more exquisite graphics, allowing staff to produce images and animations through text descriptions, improving drawing efficiency while enhancing player experience.
Film and Television. The workflow in the film and television industry is generally lengthy, involving significant manpower and time costs. AIGC will empower the entire filmmaking process, from strategy, shooting, production to promotion, significantly lowering the entry barriers for the film and television industry while providing content creative references and achieving cost reduction and efficiency improvement in the industry. In the planning phase, deep learning algorithms can provide script creative references for screenwriters by quickly reading a large number of published films and combining keywords; after the script is completed, it can also assist screenwriters in polishing and translating. In the shooting phase, directors can utilize AIGC to assist in completing storyboarding, shot language design, and other tasks; meanwhile, producers can save time needed for scheduling, production coordination, and crew budgeting, simplifying work and saving time costs. In the post-production phase, AIGC can complete basic tasks such as adding subtitles, video editing, and color grading; as technology matures, it can gradually handle more complex tasks such as special effects production and animation production. For instance, the Oscar-winning film “Everything Everywhere All at Once” in 2023 had a visual effects team of only five people, who collaborated with Runway to use its AI tools to complete background work, slow down videos, and create infinitely extending images, greatly enhancing visual effects production efficiency.
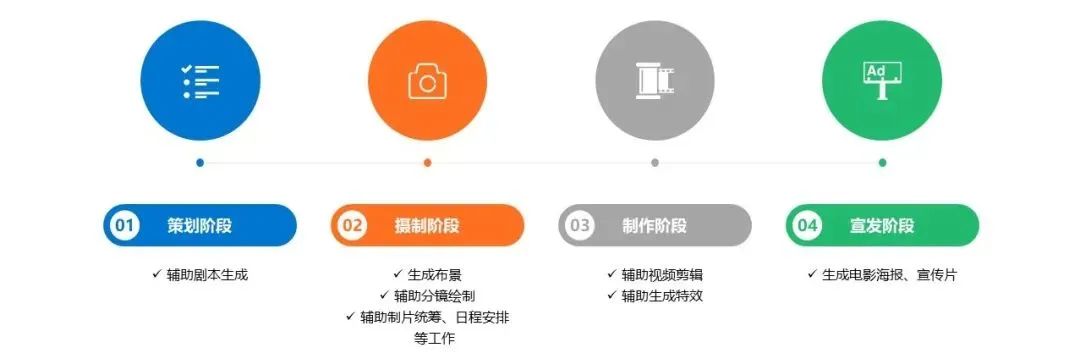
Illustration: AIGC Empowering Various Stages of Film Production
Although AIGC can significantly enhance the intelligence level and operational efficiency across industries, its development still faces certain limitations, and there are many challenges at the application end
SaaS. The application of AIGC in the SaaS industry has raised issues such as data privacy and information security. In providing personalized services and support, AIGC needs to input sensitive information data such as internal operations, finances, and personal transactions of enterprises. AIGC models have potential memory capabilities, which may inadvertently extract private data from other users during content generation, leading to serious privacy breach risks.
Digital Design. The design industry places particular importance on copyright requirements. When AIGC is trained using large-scale data from the internet and third-party datasets, it may include unauthorized data obtained through web scraping or other methods, resulting in the generation of derivative works that mix existing content and new creative elements, potentially causing confusion regarding intellectual property ownership and leading to potential legal risks and copyright disputes. Especially in the digital design field, the application of AIGC may involve significant use and transformation of original data, leading to considerable disputes over copyright ownership of generated works.
Finance. The financial industry often requires reference to information from multiple parties, with high demands for accuracy. However, the accuracy of analyses made by AIGC based on historical and real-time information still needs improvement and cannot predict unexpected events. In recent years, financial institutions have launched generative AI tools such as intelligent advisors; if investors overly rely on the predictions and suggestions provided by these tools, it may lead to irrational investment behaviors, exacerbate herd effects, and increase risk concentration. Additionally, AIGC can easily generate false news or misleading information, leading investors to make erroneous decisions and potentially causing abnormal market price fluctuations.
Games. As a form of entertainment that emphasizes real-time human-computer interaction, the emergence of AIGC has provided players with a better immersive experience in virtual worlds. However, the uncontrolled and limitless human-computer dialogues may pose significant uncertainties regarding compliance of interactive content, and if AIGC fails to manage filtering words effectively, players may be offended or harmed.
Film and Television. In the film and television industry, which requires emotional resonance, AIGC can only rely on existing data and algorithms to generate relatively rigid and cold content compared to human creation based on rich emotions and deep experiences. The anthropomorphized emotional expression still needs improvement.
Publishing. In the literary field, there are strict requirements for the ethical and moral issues involved in content. Currently, AIGC cannot ensure the compliance of generated content, and the training data used to develop AIGC models may contain discriminatory, violent, and other content, resulting in the generation of harmful content such as racial or gender discrimination.
Overall, AIGC empowers various industries such as finance, gaming, and publishing through multimodal large models and deep learning algorithms, but the ethical, copyright, and data security issues and challenges it brings cannot be ignored.
FOUR
Development Outlook
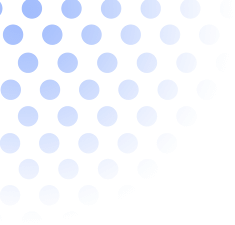
The Cross-Modal Generation Capabilities Demonstrated by Software like Sora Indicate the Acceleration of the AGI Era
Artificial General Intelligence (AGI) is an artificial intelligence system that can think, learn, correct, and perform intellectual tasks like humans in any professional field, requiring AI systems to possess common sense, shared action norms, and values understood by humans. Its most significant feature is responding to the rules of the real world, such as physical states, natural laws, and chemical changes, making it one of the highest goals of AI development. The release of applications like Sora and ChatGPT signifies a breakthrough in the AI technology field, possessing stronger spatiotemporal modeling capabilities and higher computational complexity, capable of simulating a real physical world that conforms to physical laws in three-dimensional space, laying the technical foundation for understanding and simulating the real world, which will also accelerate the development of multimodal AI and further expedite the progress of AGI.
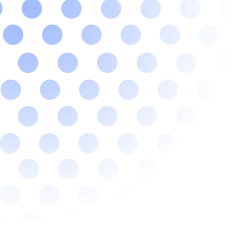
Technological Innovations and Integrations Will Continuously Enhance AIGC’s Generation and Application Capabilities
In the future, on one hand, as deep learning, computer vision, and other technologies continue to mature, along with ongoing innovations in new technologies such as knowledge distillation, AIGC’s capabilities in generation quality, speed, and efficiency will further improve; on the other hand, multimodal large models will integrate with richer technologies such as natural language processing, virtual reality, augmented reality, and digital twins, expanding more application scenarios such as autonomous driving, drug research and development, and security, while providing users with richer solutions to meet the increasing demands of users. For instance, in the field of autonomous driving, AIGC technology can create more synthetic data to compensate for the lack of real data, accelerating the construction of simulation scenarios and improving simulation testing efficiency.
Source: | 36Kr Research Institute