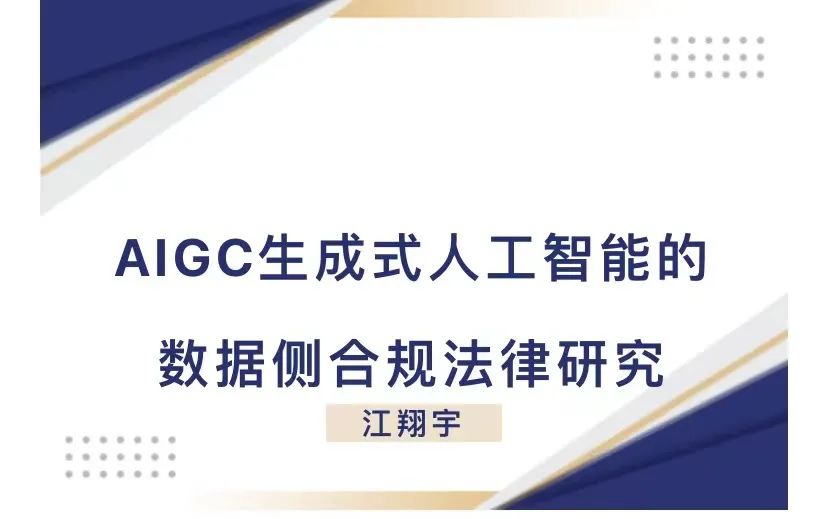
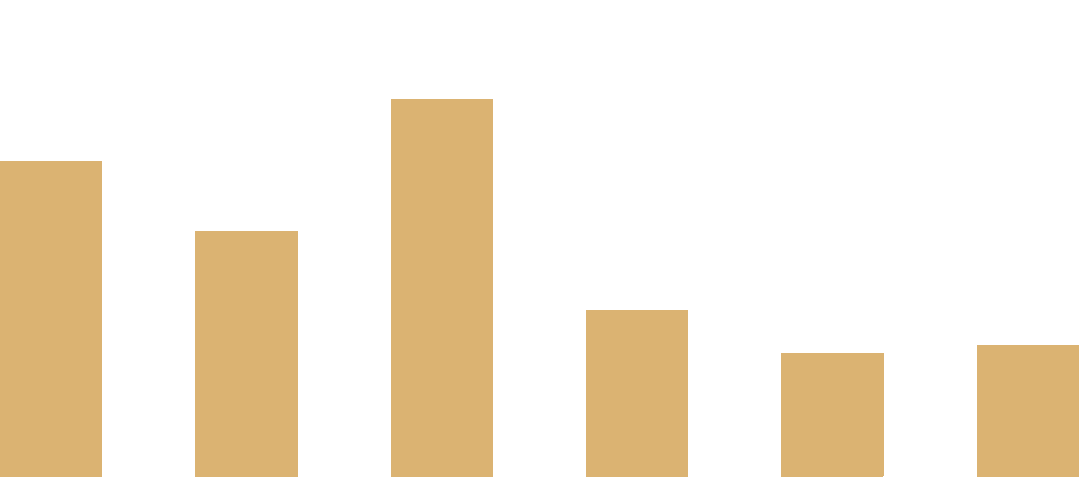
The “Interim Measures for the Management of Generative Artificial Intelligence Services” came into effect on August 15, 2023. AIGC generative artificial intelligence faces significant challenges regarding data compliance, personal privacy protection, and intellectual property protection based on massive amounts of data. This article focuses on a preliminary study of this issue.
01
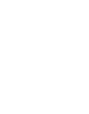
Definition of Generative Artificial Intelligence
Classification of Artificial Intelligence
Artificial intelligence can be classified into narrow artificial intelligence (Artificial Narrow Intelligence, ANI), general artificial intelligence (Artificial General Intelligence, AGI), and super artificial intelligence (Artificial Super Intelligence, ASI). Among them, ANI, also known as weak artificial intelligence, refers to machines exhibiting intelligence in specific domains, such as chess, language translation, and predictive development; AGI, also known as strong artificial intelligence, refers to machines achieving human-level performance capable of solving complex problems across various domains. It is generally believed that human technology has not yet reached the level of AGI, but general large models represented by GPT-4 are approaching so-called general artificial intelligence.
In the narrow artificial intelligence stage, the main technical directions can be divided into two categories: decision/analytical AI (Discriminant/Analytical AI) and generative AI (Generative AI). Decision AI refers to learning the conditional probability distribution from data, analyzing, judging, and predicting based on existing data, while generative AI is a type of machine learning algorithm that does not only analyze existing data but learns the joint probability distribution of data, synthesizing existing data to create and innovate autonomously, generally outputting text, images, language, video, code, and other content.
Currently, decision/analytical AI has been commercialized in recommendation systems, image recognition, content review, and autonomous driving, especially in the field of facial recognition in image recognition, which has fully integrated into the real economy, and in the field of autonomous driving, it has reached a semi-mature state; generative AI belongs to the production tools of Web 3.0 and has been involved in areas such as game development, literary creation, music composition, drug invention, and new material synthesis, more related to the field of innovation.
Thus, it can be inferred that the “generative artificial intelligence technology” mentioned in the “Interim Measures for the Management of Generative Artificial Intelligence Services” (hereinafter referred to as the “new regulations”) refers to the generative AI in the narrow artificial intelligence stage, and there is currently a trend toward the development of general artificial intelligence.
Distinction Between Generative Artificial Intelligence Technology and Deep Synthesis Technology
Deep synthesis technology originated from “deepfake”. The “Deep Synthesis Management Regulations” in China define deep synthesis technology as “the technology that uses deep learning, virtual reality, and other generative synthesis algorithms to produce text, images, audio, video, virtual scenes, and other network information.”
For generative artificial intelligence technology, the “Interim Measures for the Management of Generative Artificial Intelligence Services (Draft for Comments)” (hereinafter referred to as the “draft for comments”) defines it as “the technology that generates text, images, sounds, videos, codes, and other content based on algorithms, models, and rules”; the new regulations also define it as “generative artificial intelligence technology refers to models and related technologies that have the capability to generate text, images, audio, video, etc.” Compared to the draft for comments, the new regulations narrow down “algorithms, models, and rules” to “models and related technologies”, indicating that regulatory authorities do distinguish between the underlying technologies of regulatory objects.
From the legal definition of the concepts, deep synthesis technology is based on algorithms, while generative artificial intelligence technology emphasizes models more. Each model contains algorithms, which are designed, have low freedom, and strong interpretability; models are more complex and are the result of training. Algorithms refer to methods for specific problems, usually implemented by program code, such as handwritten recognition algorithms, sorting algorithms, etc.; models are a collection of multiple algorithms organized according to a certain architecture, which refers to a module that can solve specific problems obtained after data is trained by algorithms. For example, a handwritten recognition model can output the text in an image when a handwritten image is input. The so-called large model refers to a machine learning model with a large number of parameters and complex structures, applicable to processing large-scale data and complex problems. Furthermore, the definitions of relevant technologies in the above regulations are relatively vague, which somewhat expands the original scope of both technologies, especially deep synthesis technology. Therefore, there are also views that under the existing legal framework, generative artificial intelligence technology is a subset of deep synthesis technology, and it can be regulated under the “Deep Synthesis Management Regulations” through certain interpretations. However, I believe that although there is a connection between the two, they are indeed different, so this view needs to be debated.
From the perspective of the technologies themselves, although both involve generative synthesis algorithms and have certain similarities and intersections in the application of underlying technologies, they differ in specific application directions. Deep synthesis technology essentially combines and stitches existing data (images, text, etc.) based on certain requirements and cannot generate new content from scratch; whereas the logic of generative artificial intelligence technology is “understand-create”, and the generated content is novel, not merely a stitching of existing content; in other words, it has the ability to derive and innovate from existing data.
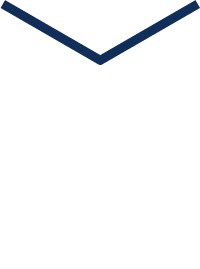
02
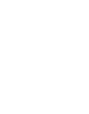
Scope of Application of the Interim Measures for the Management of Generative Artificial Intelligence Services
General Judgment Criteria

Article 2 of the new regulations states that “the provision of generative AI services to the public within the People’s Republic of China, which utilizes generative artificial intelligence technology to generate text, images, audio, video, etc. (hereinafter referred to as generative AI services), shall be governed by these measures. If the state has other regulations for activities such as news publishing, film and television production, and literary creation using generative AI services, those regulations shall prevail. Industry organizations, enterprises, educational and research institutions, public cultural institutions, and relevant professional institutions that develop and apply generative artificial intelligence technology without providing generative AI services to the public within the territory shall not be subject to the provisions of these measures.”
Thus, it can be concluded that when judging whether relevant enterprises are subject to the regulation of the new regulations, four criteria must be considered simultaneously: “within the territory”, “public”, “providing generative artificial intelligence technology services”, and the following “(2) situations excluded from the application of the new regulations”.
First, “within the territory” means that the service target is within the territory of the People’s Republic of China. Furthermore, according to Article 20 of the new regulations, if foreign service providers provide generative artificial intelligence technology services to the public within the territory, they are also subject to the regulation of the new regulations.
Second, “public” refers to an unspecified majority and does not necessarily only refer to natural persons. For instance, Article 8 of the Supreme People’s Court’s interpretation of several issues regarding the application of law in civil disputes over trademark cases clearly states that “the relevant public referred to in trademark law refers to consumers related to a certain type of goods or services identified by the trademark and other operators closely related to the marketing of the aforementioned goods or services”, without excluding non-natural organizations, legal persons, etc.
According to Item 3 of Article 22 of the new regulations, users of generative artificial intelligence services include both organizations and individuals. Thus, if generative artificial intelligence technology services are provided to unspecified enterprises (B2B), it also constitutes a service provider and should be regulated by the new regulations.
Third, “providing generative artificial intelligence technology services” means providing the “generative artificial intelligence technology” specified in the new regulations, the specific scope of which has already been defined and will not be elaborated here.
Exclusions from the Application of the New Regulations

After clarifying the scope of application of the new regulations, it is not difficult to conclude that the new regulations exclude the following situations:
First, providing generative artificial intelligence services to foreign public from within China;
Second, providing generative artificial intelligence services only to specific objects within China, i.e., the objects are specific and do not constitute the “public” of “unspecified subjects”;
Third, Article 2, paragraph 2 of the new regulations states that “if the state has other regulations for activities such as news publishing, film and television production, and literary creation using generative artificial intelligence services, those regulations shall prevail”, meaning that in the aforementioned fields, specialized regulations should be prioritized;
Fourth, Article 2, paragraph 3 of the new regulations states that “industry organizations, enterprises, educational and research institutions, public cultural institutions, and relevant professional institutions that develop and apply generative artificial intelligence technology without providing generative artificial intelligence services to the public within the territory shall not be subject to the provisions of these measures”. Therefore, internal use of generative artificial intelligence services based on internal enterprise needs is not subject to these measures.
Discussion of Special Circumstances

In practice, there are some special circumstances, such as enterprises only using generative artificial intelligence technology to assist decision-making to improve their service capabilities, or the enterprises themselves do not control the large model, merely acting as a “megaphone” between the user and the large model (the aforementioned two scenarios will be elaborated later). At this point, it is necessary to make case-by-case judgments on whether relevant enterprises constitute “generative artificial intelligence service providers” (hereinafter referred to as service providers) to clarify whether they should be subject to the new regulations.
1. Judgment Criteria
Article 17 of the new regulations states that “generative artificial intelligence services with public opinion attributes or social mobilization capabilities” need to undergo algorithm filing, etc. Taking ChatGPT as an example, it is generally believed that since it only provides content to users in a “point-to-point” manner, it does not possess “public opinion attributes”. However, the content generated based on user needs is highly likely to influence users’ thoughts and behaviors, hence similar large models should be identified as having “social mobilization capabilities”. This provision can be understood as requiring enhanced regulation of generative artificial intelligence services that can influence public thought, to prevent the generated content from adversely affecting the public and disrupting normal social discourse and order.
Based on this, it can be concluded that the regulatory logic of the new regulations regarding generative artificial intelligence technology lies in whether it will have adverse effects on the “public”. In other words, if the enterprise itself has no illegal purpose, if generative artificial intelligence generates incorrect, immoral, or even illegal content, whether the “public” can access that content. That is, if the enterprise has actual control over the content, modifying, deleting, or adding it, and after a substantive review of the content generated by the generative artificial intelligence, it is then handed over to users directly (sending the generated content unchanged to users) or indirectly (summarized and paraphrased by enterprise staff), and this process can to some extent avoid users being affected by erroneous content, then the relevant enterprise should not be recognized as a service provider and thus not be subject to the new regulations.
2. Enterprises Introducing Generative Artificial Intelligence to Assist Decision-Making
In cases where enterprises introduce generative artificial intelligence to assist decision-making to enhance their service capabilities, for example, if the customer service department of an enterprise introduces generative artificial intelligence technology and uses the generated content as assistance and reference for staff in answering customer inquiries. At this point, when enterprise staff provide services to the “public”, they do not directly pass on the generated content from the generative artificial intelligence to the users; rather, the content serves only as assistance and reference for the staff to provide services, with the actual service being provided by the enterprise staff themselves, who are responsible for the content expressed. In other words, even if generative artificial intelligence generates erroneous content based on user circumstances, enterprise staff will screen it to avoid users encountering erroneous generated content within their capabilities.
In such cases, the relevant enterprise should not be recognized as a service provider, meaning that the enterprise is not subject to the new regulations.
3. Enterprises as “Megaphones” for Generative Artificial Intelligence Technology
Specifically, this situation can be understood as “megaphones”, where enterprises act on behalf of users to use generative artificial intelligence services, meaning that the enterprise nominally “provides generative artificial intelligence services” externally, but the enterprise does not actually control the relevant large model. After the user issues a request to the enterprise, the enterprise sends the same request to the large model accessible to it as a user, and after receiving the generated content, the enterprise directly conveys this generated content to the user without conducting substantial review (as illustrated below).
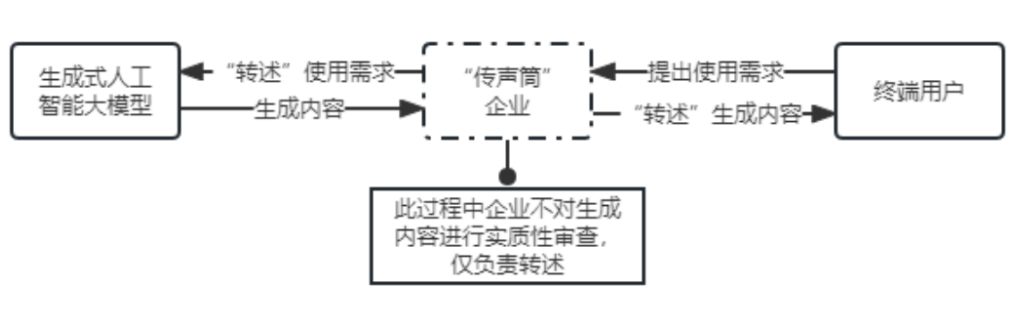
In this situation, if the generative artificial intelligence generates erroneous content, the end user may have access to that content. According to the aforementioned judgment criteria, the “megaphone” enterprise should be recognized as a service provider and thus subject to the new regulations. According to the provisions of the new regulations, service providers need to be responsible for the entire stage of research and operation of the generative artificial intelligence large model. Therefore, recognizing the “megaphone” enterprise as a service provider, making it bear the compliance risks of the entire stage related to the large model, does not violate the spirit of the new regulations.
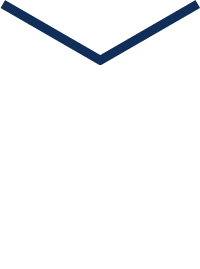
03
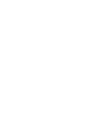
Ownership Issues of AIGC
Can AIGC Constitute Copyright Objects?
As for whether AIGC (AI Generated Content, referring to content generated by artificial intelligence, hereinafter referred to as “AIGC”) can constitute copyright objects and be recognized for rights distribution and ownership under the copyright system, there are currently no clear legal provisions, and opinions in the practical community on this issue are also not uniform.
In case number (2019) Jing 73 Min Zhong 2030, the Beijing Intellectual Property Court held that “works should be created and completed by natural persons, and content generated by computer software does not constitute works”. For related generated products, the developers (owners) of the computer software and the users of the software cannot sign as authors and should indicate that the related content is generated by the software intelligence. The users of the computer software can reasonably indicate their rights on the content generated by the software.
In case number (2019) Yue 0305 Min Chu 14010, the Nanshan District People’s Court recognized that Tencent Company holds copyright over the AI-generated works involved in the case, stating that “analyzing the external presentation form and generation process of the article involved in the case, the specific presentation form of the article and its origin from the creator’s personalized choices and arrangements, along with the technical ‘generation’ process by Dreamwriter software, meet the copyright law’s protection conditions for written works, this court recognizes that the article involved in the case belongs to the written works protected by China’s copyright law”.
In this regard, I believe that based on the encouragement of the development of generative artificial intelligence technology, it is appropriate to recognize that there are rights holders who enjoy copyright over AI-generated works that meet copyright standards. Additionally, one of the purposes of copyright law is to encourage innovation; recognizing that AIGC can constitute copyright objects and clarifying the criteria for determining its rights holders does not contradict the essence of copyright law and aligns with the social requirement to encourage the development of new technologies.
Criteria for Determining AIGC as Copyright Objects
On the premise of recognizing that AIGC can become copyright objects, it is necessary to clarify the corresponding judgment criteria, namely, what kind of AIGC can be recognized as copyright objects. In this regard, we can refer to the judgment criteria of the Nanshan District People’s Court, which states: first, whether AIGC has originality. This should be analyzed from whether it is independently created and whether its external presentation differs to some extent from existing works, or whether it possesses a minimum degree of creativity; secondly, the generation process of AIGC should be analyzed to see if it reflects the creator’s personalized choices, judgments, and skills, that is, whether the creator has invested sufficient contributions that can influence the generated content and style when using generative artificial intelligence to generate relevant content.
If the above two points can be satisfied, then AIGC should be considered to meet the recognition criteria for works under copyright law, and AIGC can constitute copyright objects.
For example, taking ChatGPT as an example, assuming its generated products already possess originality, it is necessary to determine whether the service provider/user has invested sufficient contributions in its creation. From the user’s perspective, if they have contributed sufficiently to the creation of the relevant generated product, collecting a large amount of information and continually adjusting the generated content (language, logic, style, etc.) within the system, ultimately creating content with originality, then it should be recognized that the content meets the recognition criteria for works under copyright law. The content generated by the generative artificial intelligence under the guidance and contribution of the service provider should also be included.
Determining Ownership of AIGC
Based on the above analysis, AIGC has the potential to constitute copyright objects, thus when determining the ownership of AIGC, it is essential to first judge whether it can constitute a work. In the case of constituting a work, the relevant copyright subject should be clarified; in the case of not constituting a work, it involves property rights related to AIGC such as “holding, using, and benefiting”, at which point it is appropriate to clarify the distribution of interests between the service provider and the user. However, due to the lack of clear legal provisions at present, this article only proposes general judgment criteria from the perspective of balancing the interests of various parties.
First, when determining the ownership of AIGC, it should first clarify whether there is a clear agreement between the service provider and the user regarding this; if so, the agreement should be followed under the premise of valid agreements between both parties.
Second, in the absence of prior agreements, it should be judged whether AIGC can constitute a work. If it can constitute a work, the copyright subject can be determined based on the extent of contribution by the service provider and the user to the generation of related content. When it is difficult to compare the extent of creative intellectual labor contributed by both parties, consideration can be given to attributing copyright to the user. This is because service providers often charge a fee for providing services, and they have already profited from providing generative artificial intelligence services; therefore, attributing copyright to the user can avoid the service provider from “multiple profits” to a certain extent and balance the interests between the service provider and the user.
Third, in cases where AIGC does not constitute a work, it no longer involves the determination of copyright ownership, and the corresponding AIGC should belong to the user’s “usage records”. According to Article 11 of the new regulations, “the provider shall fulfill its protection obligations regarding the user’s input information and usage records in accordance with the law, shall not collect unnecessary personal information, shall not illegally retain input information and usage records that can identify the user’s identity, and shall not illegally provide the user’s input information and usage records to others”. Therefore, it can be understood that when utilizing artificial intelligence generated products, service providers should obtain the user’s authorization, especially not illegally using identifiable user identity usage records. In this case, if the service provider utilizes the generated content without authorization, it would pose significant infringement and compliance risks.
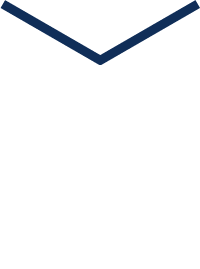
04
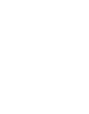
Can Models Trained on Illegal Training Data and Parameters Continue to Be Used?
Compared to the draft for comments, the new regulations have significantly relaxed the requirements for model developers/service providers regarding the training data used when training large models, but they have also made provisions regarding training data. Article 7, items 1 and 4 of the new regulations state that service providers should comply with “using data and foundational models with legal sources” and “taking effective measures to improve the quality of training data, enhancing the authenticity, accuracy, objectivity, and diversity of training data”.
Illegal Training Data Itself
Since the parameters of large models depend on the training data input, if the training data used in the development and training of large models is illegal, such as data obtained in violation of laws and regulations, public order, national interests, or human morality, the large model itself has a high possibility of generating illegal content. Of course, there is also a possibility that a large model needs to use data containing illegal content to train the model to identify “bad actors”. In this regard, it should be understood that, except for the aforementioned special circumstances, in cases where illegal data is used to train large models, neither the parameters obtained from the training of the large model nor the large model itself can continue to be used.
Illegal Source of Training Data
Regarding the training data content itself being legal but the source of the training data being illegal, whether the relevant parameters and large model obtained from training can continue to be used is currently not clearly regulated by law. In this regard, to promote and encourage the development of relevant technologies in the field of artificial intelligence, it should not be a “one-size-fits-all” approach, and case-by-case judgments should be made.
1. Service Providers Obtain Data from Legal Channels but the Source is Illegal
This situation occurs when service providers/model developers legally purchase training data from data holders A through legal means for training large models, but the training data acquired is obtained illegally by data holder A (e.g., through illegal scraping, illegal purchase, etc.). In this case, it should be recognized that service providers/model developers, through substantial labor and wisdom invested in the aforementioned data, are allowed to continue using the large models and related parameters obtained from the illegal source of training data (similar to the good faith acquisition system).
However, at the same time, service providers/model developers should provide proof that they have conducted reasonable examination of the relevant training data sources; otherwise, it is difficult to demonstrate that they have exercised reasonable due diligence in examining the sources of training data. In this case, if the service provider/model developer cannot provide corresponding evidence, it cannot be considered that they have conducted reasonable and necessary examination of the training data sources, and they should bear relevant infringement and other responsibilities; in serious cases, they should not be allowed to continue using the large model and related parameters trained based on the aforementioned training data.
2. Service Providers Illegally Obtain Training Data
If service providers/model developers obtain relevant data through illegal means or channels for training large models, they clearly violate the provisions of Article 7 of the new regulations regarding “using data and foundational models with legal sources”. In this case, the subjective maliciousness of the service providers/model developers is evident, and they should not be allowed to continue using the large models and related parameters obtained from the training of the aforementioned data, and they may also bear corresponding legal responsibilities due to their illegal actions.
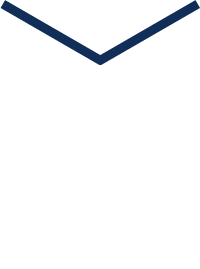
05
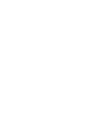
Compliance of Pre-Training Data for Model Developers and Service Providers
Article 7 of the new regulations states that “generative artificial intelligence service providers (hereinafter referred to as providers) must lawfully carry out pre-training, optimization training, and other training data processing activities, complying with the following provisions: (1) using data and foundational models with legal sources; (2) not infringing on the intellectual property rights legally enjoyed by others; (3) regarding personal information, obtaining personal consent or complying with other circumstances prescribed by laws and administrative regulations; (4) taking effective measures to improve the quality of training data, enhancing the authenticity, accuracy, objectivity, and diversity of training data; and (5) complying with other relevant laws and administrative regulations such as the “Cybersecurity Law of the People’s Republic of China”, “Data Security Law of the People’s Republic of China”, and “Personal Information Protection Law of the People’s Republic of China” as well as relevant regulatory requirements of competent authorities.”
Article 8 of the new regulations states that “when conducting data labeling during the research and development of generative artificial intelligence technology, providers should formulate clear, specific, and operable labeling rules that comply with the requirements of these measures; conduct data labeling quality assessments, and sample check the accuracy of labeling content; provide necessary training for labeling personnel to enhance their awareness of compliance with laws, and supervise and guide labeling personnel to carry out labeling work in a standardized manner.”
Based on this, it can be concluded that service providers/model developers need to focus on the following compliance risk points when using training data for pre-training large models:
Legality of Data Sources

Currently, model developers/service providers typically obtain training data through direct collection (e.g., through web scraping software, RPA technologies), indirect collection (e.g., purchasing from data providers, data sharing), or using synthetic data. In this regard, it is essential to focus on whether the behavior and channels of data collection by model developers/service providers are compliant.
For instance, when collecting data using web scraping software and other technologies, they must comply with the agreements with the scraped websites and must not damage or bypass their anti-scraping technical measures. The scraped data should be public data and must not involve personal information, important data, sensitive data, etc., and must not violate the relevant provisions of the “Anti-Unfair Competition Law”. When obtaining training data through purchasing or data sharing, model developers/service providers should conduct reasonable examinations of the sources of the relevant data to avoid compliance risks arising from illegal acquisition methods upstream.
Data Training Process and Infringement of Others’ Intellectual Property Rights

The training of large models requires a substantial amount of data input, such as text and images, including many works protected by copyright. Therefore, training large models using publicly obtained data without the authorization of relevant copyright holders may constitute infringement of the copyright enjoyed by the respective copyright holders. At this stage, requiring service providers/model developers to obtain authorization from all relevant copyright holders before training large models is evidently unrealistic, and imposing excessively heavy compliance obligations would undoubtedly compress the development space for the generative artificial intelligence industry, which contradicts the spirit of the new regulations encouraging relevant technological development. Thus, achieving a balance of interests between service providers/model developers and copyright holders in the field of intellectual property is particularly important for promoting the development of the entire artificial intelligence sector. However, no consensus has been reached in both theoretical and practical circles on this issue.
In early August 2023, The New York Times officially announced its updated service terms, stating that “without its written permission, all photos, images, designs, and video clips published or provided by The New York Times, as well as other materials or data, shall not be used for training generative artificial intelligence, otherwise, civil or criminal liability will be pursued.” Regarding Open AI’s actions of training ChatGPT using works for which it holds copyright without authorization from The New York Times, The New York Times recently indicated that it may sue Open AI to defend its hard-earned intellectual property. Conversely, the “proponents” represented by the Associated Press hold an opposing view, having signed a cooperation agreement with Open AI in July, agreeing to provide past reports to Open AI for data training. The Wall Street Journal is also considering providing training content to AI developers for a fee, viewing generative artificial intelligence as support for future performance.
Regarding this issue, some scholars suggest that the scope of “fair use” in copyright law could be expanded to exempt model developers/service providers from the obligation to obtain copyright holder authorization for works used in training large models, allowing them to use relevant works for training without authorization or only requiring a fee for such use.
However, it should be noted that current legislation in China has not clearly defined such situations as falling within the scope of “fair use”. Thus, model developers/service providers are generally required to obtain authorization from relevant copyright holders when using others’ works as training data, otherwise, they will face significant compliance risks.
Whether Training Data Involves Personal Information

In practice, large models rarely use direct personal information (such as individual names, ID numbers, etc.), but such possibilities do exist. According to Item 3 of Article 7 of the new regulations, if the training data involves personal information, personal consent should be obtained, or it should comply with other circumstances prescribed by laws and administrative regulations.
Whether Effective Measures are Taken to Improve Training Data Quality

Compared to the “ability to ensure the authenticity, accuracy, objectivity, and diversity of data” required in the draft for comments, the new regulations have significantly relaxed the quality requirements for training data, only making “suggestive” requirements. However, for model developers/service providers, providing quality training data should not be overlooked. They should actively take effective measures and keep records of this to demonstrate to relevant departments that they have fulfilled reasonable obligations to improve the quality of training data during regulatory reviews.
Whether Data Labeling Activities Comply with Regulations

Service providers/model developers should formulate labeling rules, conduct data labeling quality assessments, and provide necessary training for labeling personnel in accordance with Article 8 of the new regulations.
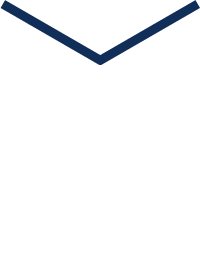
06
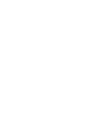
Compliance Issues Regarding Service Providers’ Storage and Use of User Input Information
The training of large models is not accomplished overnight. After service providers/model developers complete the pre-training of large models, the models are only in a preliminary form and require continuous training during subsequent service provision. It can be said that as users receive generative artificial intelligence services based on large models, they are indirectly assisting service providers in continuing to train large models. At this point, whether service providers can directly utilize the relevant information and usage records input by users during their use for upgrading and iterating the large models remains to be discussed. The new regulations do not provide clear provisions on this issue, but both the draft for comments and the new regulations stipulate the obligations of service providers regarding the input information and usage records involving users’ personal information. Based on this, it can be inferred that the regulatory authorities have a regulatory stance on this issue.
The draft for comments stipulates that “providers shall bear protection obligations for users’ input information and usage records during the provision of services. They shall not illegally retain input information that can infer the identity of users, nor create profiles based on users’ input information and usage conditions, nor provide users’ input information to others. If laws and regulations have other provisions, those shall prevail.” This requirement is a strict one, as it is presented in a prohibitive manner without specifying exceptions. Conversely, the new regulations somewhat relax the restrictions on service providers, stating that “providers shall fulfill their protection obligations regarding users’ input information and usage records in accordance with the law, shall not collect unnecessary personal information, shall not illegally retain input information and usage records that can identify users’ identities, and shall not illegally provide users’ input information and usage records to others.” By setting prerequisites of “unnecessary” and “illegal” for prohibitive provisions, the new regulations acknowledge that service providers can collect, store, and utilize such data under legal circumstances to optimize models.
Therefore, it can be concluded that the new regulations do not explicitly prohibit service providers from storing and utilizing users’ input information and usage records during use; rather, they recognize that service providers may utilize this data to optimize models. However, to avoid compliance risks, service providers should pre-emptively and explicitly obtain users’ authorization in the service agreement based on Article 9 of the new regulations, “signing service agreements with users to clarify the rights and obligations of both parties”, to enhance the legality and compliance of their collection, storage, and utilization of relevant data.
Moreover, both the draft for comments and the new regulations explicitly stipulate that service providers have the obligation to protect users’ input information and usage records. The temporary ban on ChatGPT by the Italian government and the leak of confidential codes from Samsung highlight the seriousness and severity of the leakage of users’ input information. The China Payment and Clearing Association has also pointed out that generative artificial intelligence tools have exposed risks such as cross-border data leakage, prompting them to issue a proposal on “caution for payment industry practitioners in using tools like ChatGPT” due to data security concerns. Therefore, service providers should prioritize their information security obligations and take effective measures to prevent user data leakage.
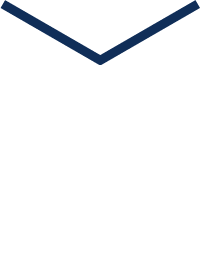
07
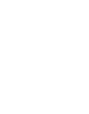
Establishment of a Data Corpus for Generative Artificial Intelligence General Large Models
Article 6, paragraph 2 of the new regulations states, “Promote the construction of generative artificial intelligence infrastructure and public training data resource platforms. Facilitate the collaborative sharing of computing power resources, enhancing the efficiency of computing resource utilization. Promote the orderly opening of public data by classification and grading, expanding high-quality public training data resources. Encourage the use of safe and trustworthy chips, software, tools, computing power, and data resources.” This highlights the state’s support for the development of generative artificial intelligence technology and emphasizes the importance of building a data corpus for general large models.
Currently, one significant pain point restricting the development of general large models for generative artificial intelligence in China is the scarcity of available Chinese training data. Statistics show that in the globally common training dataset of 5 billion large models, the proportion of Chinese data is only 1.3%, with even fewer high-quality Chinese data. Therefore, constructing a general large model Chinese data corpus, improving the quality of training data, and expanding the scale of data provision are particularly important. To address the current dilemma, the following approaches can be considered:
First, the government should take the lead in establishing large model and big data alliances. The government should play a sufficient role in coordinating data resources within its jurisdiction, accelerating the classification and opening of public data, and facilitating data sharing among enterprises. Additionally, since the government controls a significant amount of public data resources, it can establish a public data opening mechanism based on the level of data sharing among enterprises, allowing enterprises with high data sharing levels to enjoy more convenient and high-quality public data opening services within legal and reasonable limits.
Second, enhance the willingness of data holders to provide data. Standards for evaluating the quality and scale of training data can be developed, and a corpus certification mechanism can be established, along with a data pricing mechanism to promote data flow. Furthermore, data holders can be granted privileges for preferential access or use of related large models based on their data provision situation to enhance their willingness to provide data.
Third, create a safe harbor for data providers. This measure aims to reduce concerns among data providers, thereby encouraging them to provide data externally. Specifically, a mechanism for accountability for infringement by generative artificial intelligence large models should be clarified, ensuring that data providers do not bear legal responsibilities arising from infringements caused by large models when they legally collect, sell, or share training data, thus partially alleviating the responsibilities of data providers.
Fourth, support the listing and trading of corpus data products on data exchanges. Taking the Shanghai Data Exchange as an example, it has established a comprehensive data compliance assessment mechanism. Regarding the supply of corpus for large models, data providers can be encouraged to list their corpus data products on the data exchange, receiving policy support and economic subsidies for assessment fees and listing fees. This not only provides a degree of endorsement for data providers’ compliance issues but also has positive significance for data demanders.
Fifth, increase the supply of public data resources. With the intensification of data monopoly effects, it is not easy for enterprises to legally obtain high-quality training datasets. Establishing and developing public training datasets can effectively alleviate this contradiction by reducing the cost for enterprises to obtain legally sourced data.
Sixth, build an open-source community in the field of generative artificial intelligence. For example, Hugging Face Hub, one of the most influential open-source communities in the AIGC field abroad, offers over 120,000 models, 20,000 datasets, and 50,000 demo applications, all of which are open-source, public, and free, significantly lowering the technical and cost barriers in the large model industry and profoundly changing the development model of the AIGC industry. The success of this case can serve as a reference, with the government leading or encouraging enterprises to spontaneously create an open-source community in China, establishing a positive feedback mechanism within the community where users can score shared models, data, etc., allowing high-quality content sharers to profit from it, thus promoting the spontaneous circulation and sharing of data in the AIGC field.
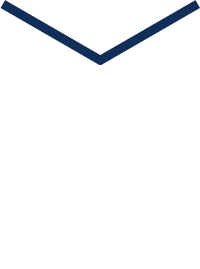
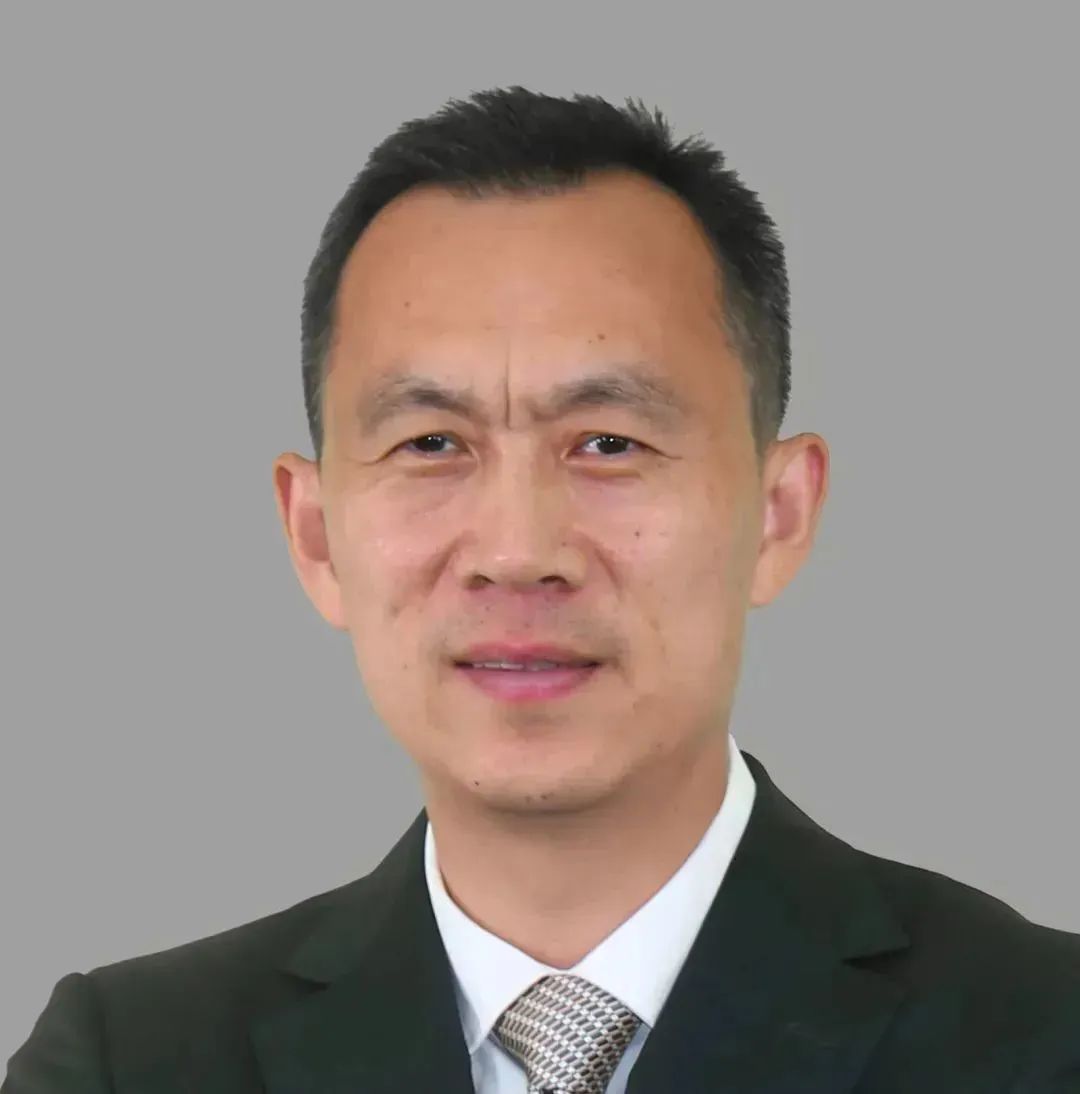
Senior Partner, Shanghai Xieli Law Firm
Doctor of Law, East China University of Political Science and Law
Focusing on legal services in asset management, securities futures, data protection, etc., has worked for Shanghai Law Society, Shanghai Financial Services Office (now Shanghai Local Financial Regulatory Bureau), UBS Securities, government industrial funds, leading Internet companies, and has been seconded to Shanghai Agricultural and Industrial Group (now Shanghai Bright Group) arranged by the Shanghai State-owned Assets Supervision and Administration Commission.
Has long served as a part-time lawyer and public lawyer, coordinating and handling numerous disputes involving financial institutions in financial regulatory departments, later responsible for legal compliance in foreign securities, equity investment funds, and the Internet industry, possessing rich legal practice and cross-border work experience in asset management, securities trading, algorithmic trading, futures and derivatives, and financial data protection. Currently provides ongoing or specialized legal services for several institutions including Guotai Junan, Eastern Airlines Jin Control, Shanghai Bank, Shanghai Yin Fund, Haitong Securities, UnionPay Smart Strategy, and Shanghai United Credit Investigation, while undertaking multiple legal research projects in the financial and data fields for the China Securities Investment Fund Industry Association, Shanghai Financial Industry Association, Shanghai Municipal Economic and Information Commission, and the Pudong Financial Bureau.
Dr. Jiang Xiangyu has been awarded the “Third-Class Merit of the Shanghai Judicial Administrative System” and “Commendation”, and concurrently serves as the Deputy Secretary-General of the Financial Law Research Association of Shanghai Law Society, Deputy Secretary-General of the Financial Legal System Professional Committee of the Shanghai Financial Industry Association, Head of the ITL Research and Development Department of the Asset Management Technology Research Alliance (volunteer), Arbitrator of the Shanghai Arbitration Commission, Arbitrator of the Shanghai International Arbitration Center, Public Mediator of the China Securities Capital Market Legal Service Center, Independent Director of Hehe Information, etc. He has published dozens of professional articles in the fields of funds, securities, trusts, and data protection in journals and newspapers such as “Legal Studies”, “East Asian Legal Studies”, “Politics and Law”, and “Shanghai Securities Journal”, and is the author of the first chapter on “Financial Data” in the first textbook on “Data Law” in China.