In the past six months, I have been continuously engaged in development work related to LLM applications. However, as many may have noticed, there haven’t been many “cognitive-refreshing” technological or application changes lately, so I haven’t had enough enthusiasm to write new articles.
Model Progress
From the model perspective, since the release of GPT-4 in March last year, we haven’t seen a significant improvement in model capabilities that excites us. Although those working on large models often mention that we can expect a tenfold improvement in model capabilities soon, a closer examination shows that the direction of improvement may not align with our expectations.
If we categorize model capabilities into perception, reasoning, and generation, many developers focused on Agent applications are primarily concerned with reasoning capabilities. However, in the past year, the main advancements in model capabilities seem to be concentrated in perception and generation, particularly in various multimodal capabilities. For example, Sora’s video generation capability, GPT-4o’s voice capabilities, and Suno.ai’s music generation. The enhancement of multimodal capabilities may help enrich interaction methods and collect more interaction/feedback data, but it currently seems to have no direct effect on the enhancement of reasoning/planning capabilities that everyone is eagerly anticipating.
Another area of significant progress over the past year is the substantial improvement in long-context support, which could be described as a hundredfold improvement. However, if the inference cost and latency do not drop to a sufficiently low level, users will still have many concerns about using long-context. Moreover, in the case of long-context, it may further increase expectations for strong reasoning capabilities. A larger context represents a more complex and end-to-end application method; if reasoning capabilities do not keep pace, then a long context seems to have little use.
Returning to reasoning capabilities themselves, the improvement in this area indeed seems to be more challenging than previously anticipated. After the release of Opus, OpenAI has yet to announce GPT-5. There are two voices in the industry:
-
The optimistic faction believes that we have already found effective paths to enhance model reasoning capabilities (starting to enter the realm of science fiction), such as discovering methods to construct long-term planning/reasoning synthetic data, or finding new model architectures that combine planning capabilities, or proving the effectiveness of easy-to-hard generalization, etc. In interviews with OpenAI and Anthropic, they indicated that data should not be a bottleneck in the short term, scaling laws still hold, but training larger models requires longer preparation time. -
The pessimistic faction is more inclined to think of autonomous driving technology; with the exponential growth of data volume, the growth of model capabilities is merely linear, or even more subdued due to the scarcity of effective data in later stages. Of course, there are also viewpoints that do not agree with this route from the principle of autoregressive transformers in achieving strong reasoning capabilities.
Which side do you lean towards?
Application Progress
When first engaging in LLM application development, everyone is bound to find prompt engineering fascinating, as it seems there are various ingenious techniques emerging daily, from CoT to ToT, from ReAct to AutoGPT, from RAG[1] to MemGPT, etc. However, as the “intelligence” capabilities of models develop relatively steadily, developers may find themselves in a dilemma:
-
If we broadly understand scaling laws/intelligent Moore’s law, assuming that model capabilities will certainly improve by over 100% in the next two years, should we construct applications in an agent swarm manner, allowing various business logic connections to be planned by agents themselves? However, due to current model capabilities limitations, applications built along this path often fail to achieve satisfactory results. -
If we want to generate some deterministic application value in the short term, we must rely on humans to perform more task decomposition and workflow construction work. This entails a risk that when model capabilities genuinely improve later, many of the accumulated work results may become irrelevant. A typical example is that when long-context support significantly improves, many previously meticulously crafted tasks such as document segmentation, summarization, and multi-hop retrieval for RAG may be directly discarded.
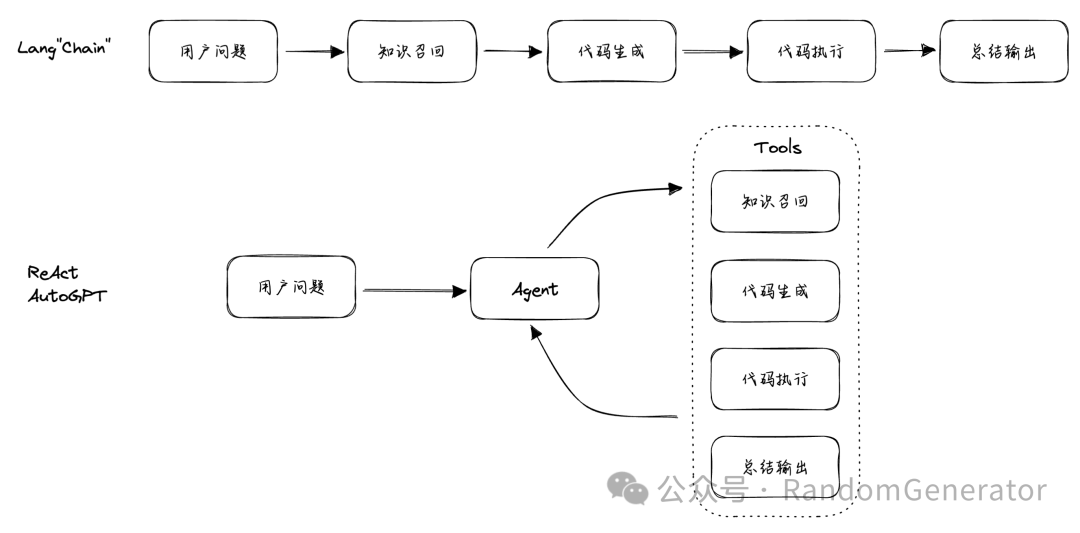
Thus, predicting the development of model capabilities and choosing the current application landing path is a matter that requires careful consideration. Where exactly will the much-discussed tenfold improvement in large models manifest? How can we bet on it ahead of time?
Opening a New Pit
We might also make an assumption: in the next three years, there will be no significant improvement in model reasoning capabilities, but there are still many relatively certain advancements, such as inference speeds possibly becoming faster, costs decreasing, contexts lengthening, and multimodal integration improving. If we treat LLM as system 1, relying on workflow construction to supplement system 2 thinking capabilities, it may also be a feasible path.
Under this assumption, there are still many interesting projects that might become mainstream application forms in the near future. I will also look for some previously seen interesting projects to learn and share insights with everyone. Planned directions include:
-
Introduction to Agent frameworks, such as the well-known AutoGen, CrewAI, etc. -
Agent application projects, for example, the currently popular coding direction has a bunch of hot projects, MetaGPT, OpenDevin, SWE-Agent, etc. -
More broadly applicable LLM development frameworks, such as LangGraph, DSPy, various controllable generation frameworks, etc. -
Projects related to evaluation, which are also a very critical part of practical application implementation. -
Topics like serving, fine-tuning, etc.
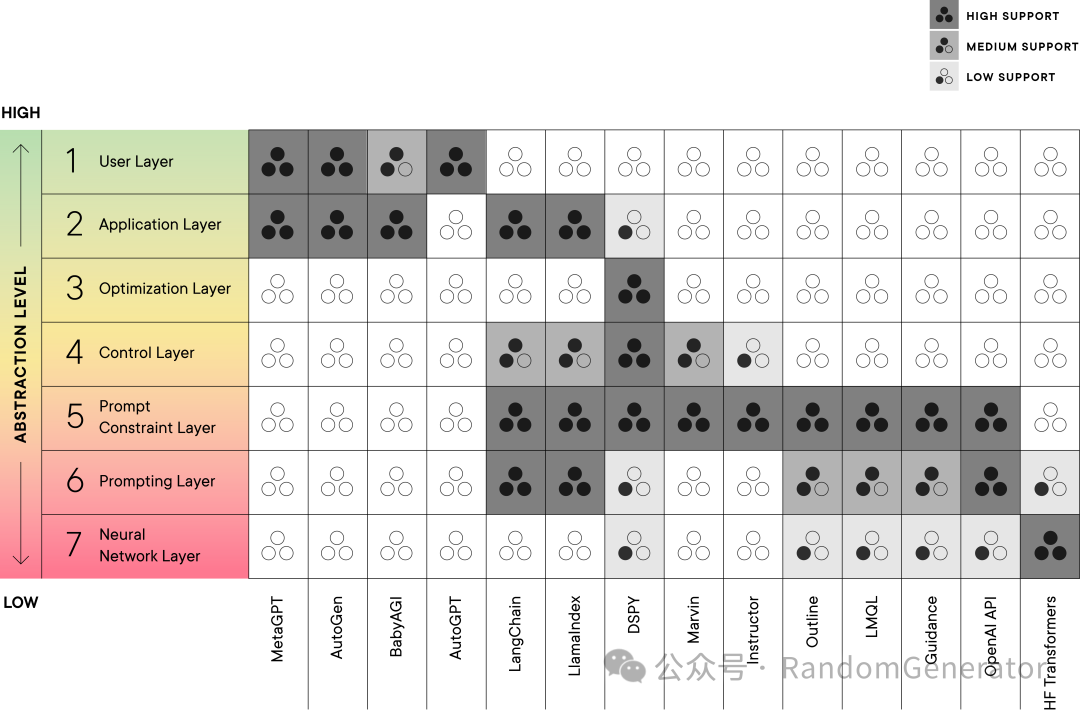
If anyone has any interesting products, frameworks, or projects, please feel free to mention them in the comments.
Prompt Optimization with DSPy
Today, as a start, let’s first discuss tools and frameworks related to automatic prompt optimization, focusing on the well-known Stanford project DSPy[2].
Problem
What does your daily LLM application development process look like? A common process usually involves:
-
Clarifying requirements, such as input and output content. -
Preparing several test cases. -
Writing a version of the prompt. -
Observing the results of the test cases and performing error analysis. -
Modifying the prompt based on relevant issues and repeating the experiment.
In scenarios that are not very complex, the number of prompts we need to maintain may not be large, and this method remains feasible. However, as the entire pipeline becomes increasingly complex, we may face a sharp increase in maintenance workload and complexity:
-
Decomposing sub-tasks as needed, each task corresponding to a set of prompts, leading to an explosion in quantity. -
Seeing new prompting techniques and wanting to try their effects. -
Modifying and optimizing the pipeline logic itself, such as integrating RAG, etc. -
Switching to new models or model versions. -
Business data experiencing “concept drift”.
At this point, manual experimentation and maintaining the prompt system become very fragile and costly.
DSPy’s Solution Approach
A close observation of the previous development process reveals that this process resembles parameter optimization in machine learning, albeit done manually. Therefore, the core idea proposed in DSPy is to automatically optimize the entire pipeline based on “training data”.
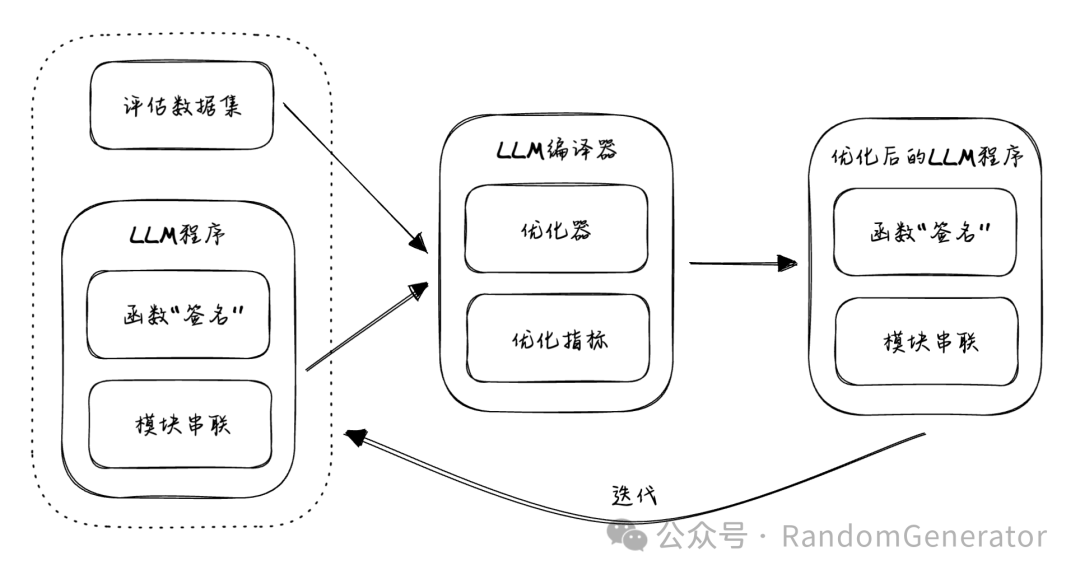
Some concepts in the figure will be elaborated on later. For clarity, we can draw an analogy with PyTorch:
-
The evaluation dataset can be considered as the training data in machine learning, providing expected input and output examples. -
The LLM program is similar to the neural network structure defined in PyTorch. -
The term “compile” is a bit nuanced; it resembles model training in PyTorch, but the LLM pipeline does not have a “backpropagation” learning algorithm. We will discuss the specific implementation later. -
The metrics used in compilation can correspond to loss functions, and optimizers can correspond to SGD, Adam, etc.
Example
Theoretically, the workflow in DSPy generally involves:
-
Collecting training data. -
Defining a program. -
Selecting evaluation methods and optimizers. -
Compile!
The simplest example from the official website is as follows:
import dspy
from dspy.datasets.gsm8k import GSM8K, gsm8k_metric
from dspy.teleprompt import BootstrapFewShot
from dspy.evaluate import Evaluate
# Set up the LM
turbo = dspy.OpenAI(model='gpt-3.5-turbo-instruct', max_tokens=250)
dspy.settings.configure(lm=turbo)
# Load training data
gsm8k = GSM8K()
gsm8k_trainset, gsm8k_devset = gsm8k.train[:10], gsm8k.dev[:10]
# Define program
class CoT(dspy.Module):
def __init__(self):
super().__init__()
self.prog = dspy.ChainOfThought("question -> answer")
def forward(self, question):
return self.prog(question=question)
# Define optimizer
config = dict(max_bootstrapped_demos=4, max_labeled_demos=4)
teleprompter = BootstrapFewShot(metric=gsm8k_metric, **config)
# Compile!
optimized_cot = teleprompter.compile(CoT(), trainset=gsm8k_trainset)
# Evaluate performance
evaluate = Evaluate(devset=gsm8k_devset, metric=gsm8k_metric, num_threads=4, display_progress=True, display_table=0)
evaluate(optimized_cot)
Isn’t it simple and clean? You can’t see where to manually write prompts…
Principle Analysis
Let’s delve into some core concepts in DSPy and their underlying working principles.
Prompt Structure Abstraction
The design behind DSPy actually abstracts the structure of prompts. It includes several components:
-
Instructions: Descriptions of the tasks that the LLM needs to complete. -
Structure description: Telling the LLM what the input and output structures are like. -
Example display: Providing the LLM with some concrete examples.
Of course, various prefixes and other elements can also be interspersed, but overall these three parts are core and correspond to the design of some concepts below.
Signatures
Formally, a signature is a function signature that defines the input and output structure of a module in a semi-structured natural language manner. For example, “sentence -> sentiment”, “document -> summary”, “context, question -> answer”, “question, choices -> reasoning, selection”, etc. Since it will later be converted into a prompt, this LLM-friendly description is quite suitable.
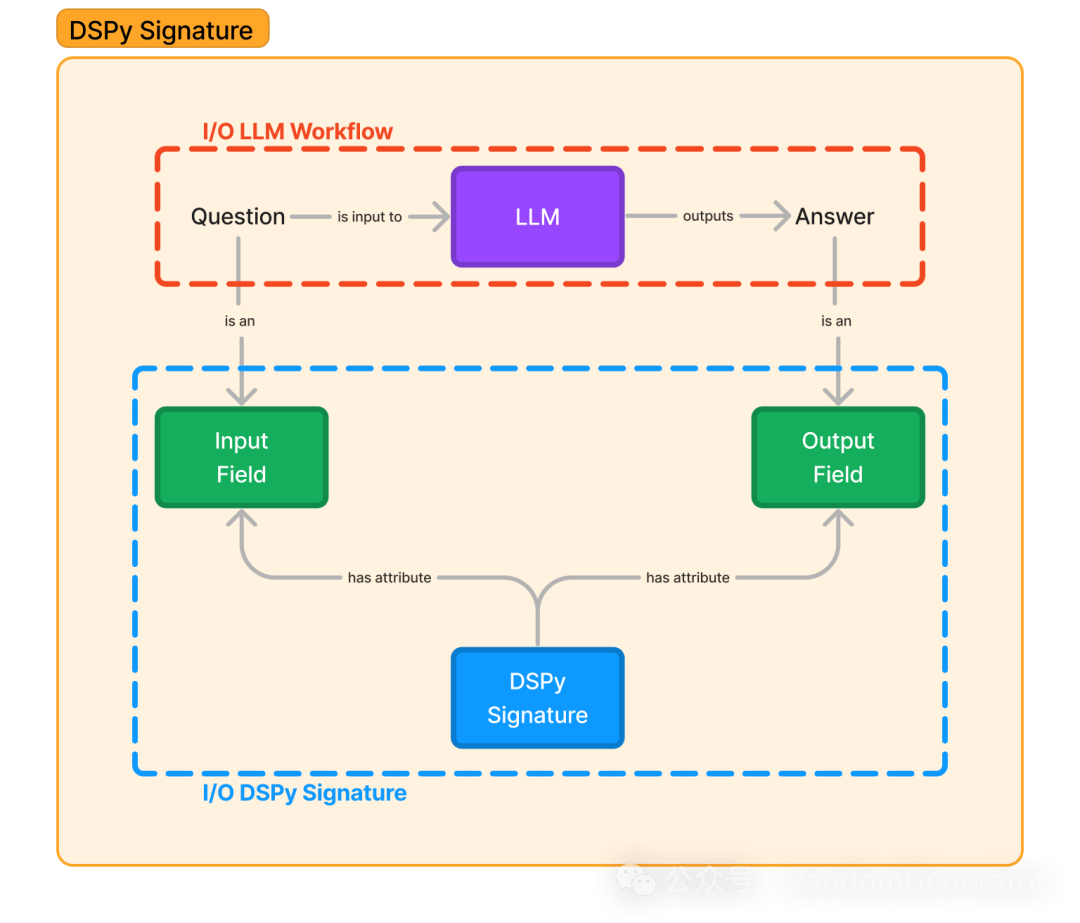
This signature will later transform into the core instructions and structure description in the prompt:
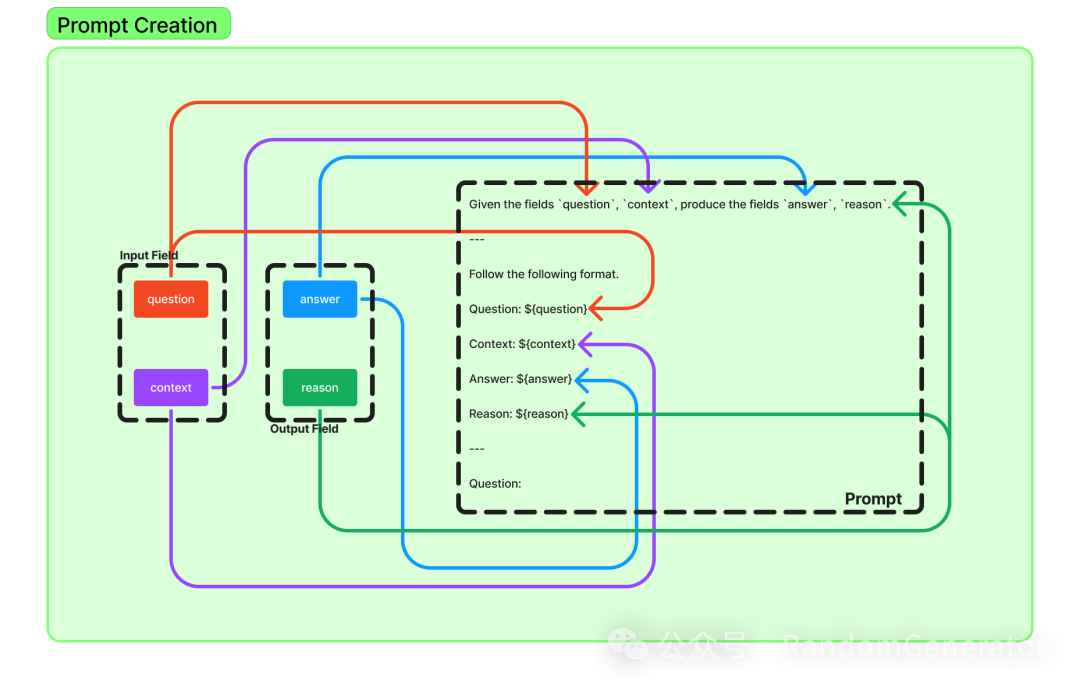
Interesting! Besides simple string-defined signatures, we can use Pydantic models to define them, making it easier to add extra instruction information, for example:
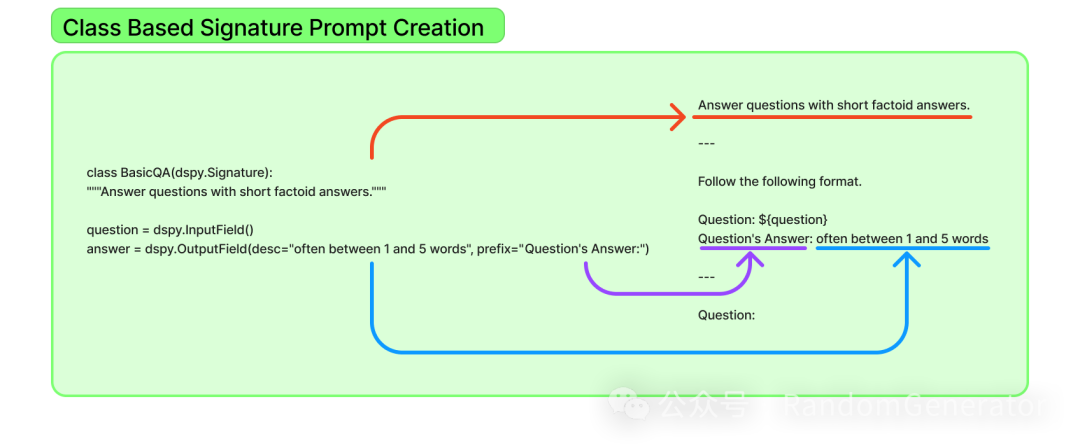
This method might remind you of Instructor[3]? DSPy indeed supports this, and we will mention it later.
Modules
DSPy modules follow specified signatures (analogous to how PyTorch handles different tensor shapes), accepting inputs and producing corresponding outputs. Similar to the concept in PyTorch, modules may contain some optimizable “parameters”; however, here the parameters may include signatures, demos, the LLM itself, etc. Moreover, modules can be chained and nested to form larger modules (ultimately a DAG at runtime).
The most basic module is dspy.Predict
, whose operational logic is consistent with the transformation of the signature into the prompt, followed by generation by the LLM. Complex pipelines can be easily constructed by chaining various modules, and similar to PyTorch, various loops, conditional control statements, etc., are also directly supported. For instance, a basic RAG example:
class RAG(dspy.Module):
def __init__(self, num_passages=3):
super().__init__()
self.retrieve = dspy.Retrieve(k=num_passages)
self.generate_answer = dspy.ChainOfThought(GenerateAnswer)
def forward(self, question):
context = self.retrieve(question).passages
prediction = self.generate_answer(context=context, question=question)
return dspy.Prediction(context=context, answer=prediction.answer)
DSPy comes with some built-in modules that encapsulate common prompting techniques, such as CoT, ReAct, etc. Let’s look at how CoT is implemented at a lower level:
class ChainOfThought(Predict):
def __init__(self, signature, **config):
super().__init__(signature, **config)
*_keys, last_key = signature.output_fields.keys()
# Here, the signature is modified to include additional CoT guidance
rationale_type = dspy.OutputField(
prefix="Reasoning: Let's think step by step in order to",
desc="${produce the " + last_key + "}. We ...",
)
self.extended_signature = signature.prepend("rationale", rationale_type, type_=str)
def forward(self, **kwargs):
return super().forward(signature=self.extended_signature, **kwargs)
Overall, it is quite understandable; it merely modifies the original signature so that additional CoT guidance is included when generating the prompt. However, the code here is simplified, and in practice, constructing a new module based on a new idea still has a certain threshold of difficulty. For example, modifying the signature in this manner may require some understanding of the signature structure and supported methods.
The core base class Predict
of the module hides quite a few intricacies in its forward
method. The code is relatively complex, but here’s a brief description:
-
It has its own lm
configuration, which will be seen later when discussing optimizers; if fine-tuning is selected in compile, thislm
will be updated. -
Various LLM parameters are also recorded, but so far, no optimizer has modified them. -
It also carries the configuration of demos
, which will save various examples. These examples will be used in prompt assembly. -
The signature will be transformed into a Template, which will then generate the prompt; this process is also quite complex. -
Inputs will be converted into dsp.Example
, and the final LLM outputs will also be wrapped intodsp.Example
, facilitating module chaining, etc.
If you need to customize certain capabilities deeply, there are many details to explore here.
As mentioned earlier, signatures can be defined using Pydantic models. If you want the module’s output results to also be Pydantic models, you can use TypedPredictor
. The documentation and examples in the code are also very intuitive.
Optimizers
Having laid the groundwork, we finally arrive at the legendary automatic optimization part. The premise of optimization is the ability to evaluate, so all the methods introduced here require pre-constructed evaluation datasets and related evaluation methods (evaluation metrics). Compared to neural network optimization, we can use any evaluation method here since it does not involve gradient calculations or backpropagation. DSPy has built-in evaluation methods, such as dspy.evaluate.metrics.answer_exact_math
, dspy.evaluate.metrics.answer_passage_match
, etc. Of course, we can also write our own, including using LLM for evaluation, which is quite flexible.
From the perspective of optimizable parameters, any LLM pipeline program we write has many forms of “knobs”, not just model weights in a relatively uniform form. For example:
-
Fixed instruction descriptions in prompt templates. -
Demonstration examples provided in the prompt. -
Choices of LLMs, different parameter settings. -
Fine-tuning of LLMs and corresponding parameters. -
Parameters of relevant modules in the pipeline, such as the configuration of retrieval modules. -
The structure of the pipeline itself, such as whether to decompose tasks, whether to use specific loop control processes, etc.
Currently, DSPy has implemented the aforementioned points 1, 2, and 4. Borrowing an image from Twitter, the effect is approximately:
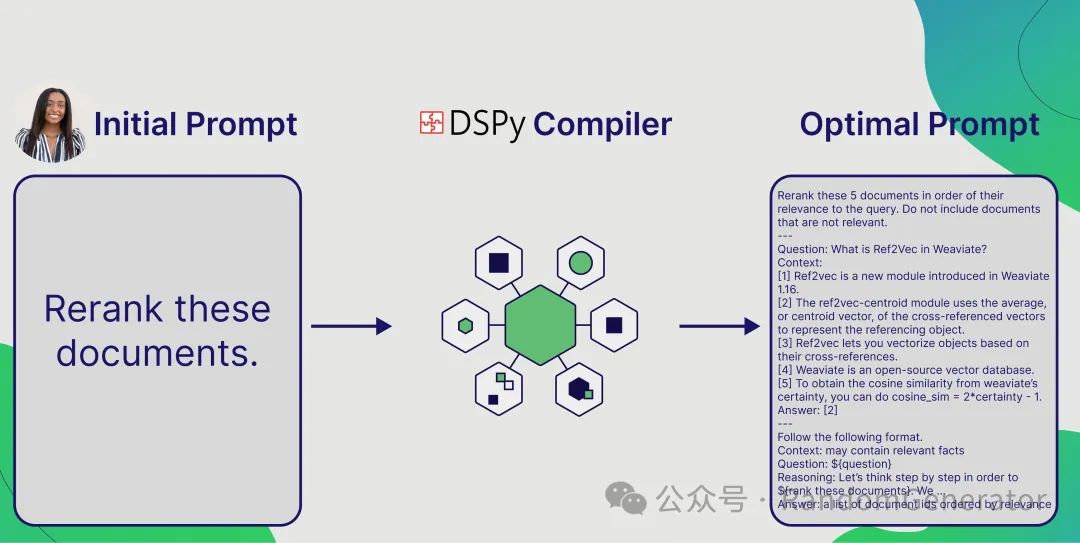
This effect is quite magical; recalling the previous example, invoking this optimization process only requires two simple lines of code:
teleprompter = BootstrapFewShot(metric=gsm8k_metric, **config)
optimized_cot = teleprompter.compile(CoT(), trainset=gsm8k_trainset)
Let’s see how this compilation process is specifically implemented, and we will select a few of the most representative optimizers to explain.
Sample Optimization in Prompts
The most typical optimization method in DSPy is BootstrapFewShot
. Its working steps are as follows:
-
First, initialize “teacher” and “student” LLM programs. From the code perspective, both must have the same pipeline structure, so the possible difference is that different LLMs can be used? It is not possible to implement distilling a more complex pipeline into a simpler one… -
Pass the initial training data to the “teacher” to form raw_demos
. -
For each training sample, the “teacher” will attempt to generate predictions. Note that this prediction can be a complex process, such as generating reasoning first and then generating the answer. -
Check the predictions generated by the “teacher”; if correct, the entire prediction trace will be added to augmented_demos
. This can achieve an effect whereby if the original sample only contains the question and answer, while we are optimizing a CoT module, this bootstrap process will save those samples that ultimately provide the correct answer while also supplementing the reasoning content! Similarly, if it is a RAG module, it can automatically add the recalled context content as part of the samples, although this may lead to larger samples… -
Finally, pass raw_demos
andaugmented_demos
to the “student” according to the configuration, completing the optimization.
In subsequent LLM program executions, this part of the demos will be included in the prompts sent to the LLM.
On this basis, we can further optimize bootstrap samples, such as:
-
BootstrapFewShotWithRandomSearch
: Randomly shuffling the initial training set, performing multiple bootstraps, and selecting the best results. -
BootstrapFewShotWithOptuna
: After completing the bootstrap, using Optuna (Bayesian optimization) to help search for the best demo. However, this only searches the index numbers of the demos, making it feel similar to random…
Instruction Optimization in Prompts
Besides samples, optimizing task descriptions and instructions is also crucial; you may often see some tricks in this area, such as structured descriptions, “Let’s think step by step”, “This is very important to my career”, and so on. Many other projects focusing on automatic prompt optimization also emphasize this aspect.
In DSPy, two main optimizers are provided.
COPRO
:
First, use BasicGenerateInstruction
to generate a series of “candidate prompts”. This meta-prompt looks something like:
class BasicGenerateInstruction(Signature):
"""You are an instruction optimizer for large language models. I will give you a ``signature`` of fields (inputs and outputs) in English. Your task is to propose an instruction that will lead a good language model to perform the task well. Don't be afraid to be creative."""
basic_instruction = dspy.InputField(desc="The initial instructions before optimization")
proposed_instruction = dspy.OutputField(desc="The improved instructions for the language model")
proposed_prefix_for_output_field = dspy.OutputField(desc="The string at the end of the prompt, which will help the model start solving the task")
Next, each “candidate prompt” undergoes multiple rounds of iteration. First, it generates and evaluates, then passes the previous prompt and evaluation results to GenerateInstructionGivenAttempts
to generate the next round of “candidate prompts”. This optimization meta-prompt looks like:
class GenerateInstructionGivenAttempts(dspy.Signature):
"""You are an instruction optimizer for large language models. I will give some task instructions I've tried, along with their corresponding validation scores. The instructions are arranged in increasing order based on their scores, where higher scores indicate better quality.
Your task is to propose a new instruction that will lead a good language model to perform the task even better. Don't be afraid to be creative."""
attempted_instructions = dspy.InputField(format=dsp.passages2text)
proposed_instruction = dspy.OutputField(desc="The improved instructions for the language model")
proposed_prefix_for_output_field = dspy.OutputField(
desc="The string at the end of the prompt, which will help the model start solving the task",
)
Finally, select the best results from all the “candidate prompts”. The entire process is illustrated in the diagram:
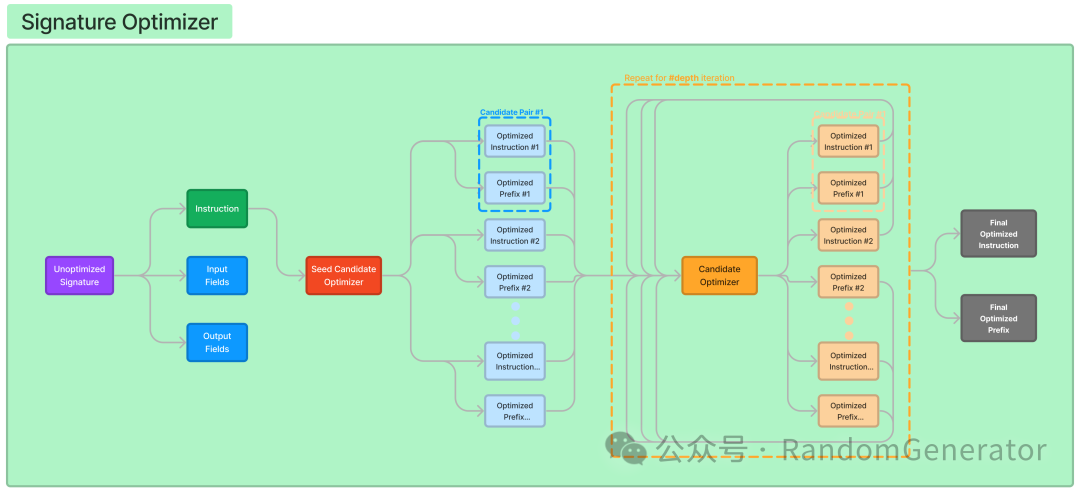
MIPRO
:
This process is even more refined.
-
First, it defaults to executing BootstrapFewShot
on all modules to generate samples. -
Next, using a prompt, let the LLM observe the training data and provide a summary. This is also an incremental summary, requiring multiple LLM calls. -
Combine the observations about the data with the examples generated during bootstrap to throw them into a meta-prompt to craft multiple “candidate prompts”. -
Finally, treat the demo selection and candidate prompt selection as an optimization problem and throw it into Optuna for optimization, which is quite advanced.
The content of the meta-prompt for generating candidate prompts is as follows:
class BasicGenerateInstructionWithExamplesAndDataObservations(dspy.Signature):
"""You are an instruction optimizer for large language models. I will give you a ``signature`` of fields (inputs and outputs) in English. Specifically, I will give you some ``observations`` I have made about the dataset and task, along with some ``examples`` of the expected inputs and outputs. I will also provide you with the current ``basic instruction`` that is being used for this task.
Your task is to propose a new improved instruction and prefix for the output field that will lead a good language model to perform the task well. Don't be afraid to be creative."""
observations = dspy.InputField(desc="Observations about the dataset and task")
examples = dspy.InputField(format=dsp.passages2text, desc="Example(s) of the task")
basic_instruction = dspy.InputField(desc="The initial instructions before optimization")
proposed_instruction = dspy.OutputField(desc="The improved instructions for the language model")
proposed_prefix_for_output_field = dspy.OutputField(
desc="The string at the end of the prompt, which will help the model start solving the task",
)
If you have previously worked with AutoML[4], you should be familiar with using Optuna for optimization. This implementation is also quite detailed, including batch processing during evaluation, allowing for early pruning. After all, each evaluation is a real LLM pipeline call, which can be quite costly.
Searching logic:
# Suggest the index of the instruction candidate to use in our trial
instruction_idx = trial.suggest_categorical(
f"{id(p_old)}_predictor_instruction",
range(len(p_instruction_candidates)),
)
demos_idx = trial.suggest_categorical(
f"{id(p_old)}_predictor_demos",
range(len(p_demo_candidates)),
)
Pruning logic:
for i in range(num_batches):
start_index = i * batch_size
end_index = min((i + 1) * batch_size, len(trainset))
split_trainset = trainset[start_index:end_index]
split_score = evaluate(candidate_program, devset=split_trainset, display_table=0)
total_score += split_score * len(split_trainset)
curr_weighted_avg_score = total_score / min((i + 1) * 100, len(trainset))
trial.report(curr_weighted_avg_score, i)
# Handle pruning based on the intermediate value.
if trial.should_prune():
print("Trial pruned.")
trial_logs[trial_num]["score"] = curr_weighted_avg_score
trial_logs[trial_num]["pruned"] = True
trial_num += 1
raise optuna.TrialPruned()
Even with such implementation, there are still many areas for potential improvement. For instance, when we conduct evaluations, we do not only look at a single score but also perform bad case analysis. Is it possible to utilize a meta-prompt to generate this error analysis and summary, incorporating it into the subsequent prompt optimization process?
LLM Fine-Tune Optimization
Besides optimizing prompts themselves, fine-tune optimization is also a common method in our daily work. DSPy implements this in BootstrapFinetune
, which is quite straightforward:
-
First, use the teacher to bootstrap a series of demos as training samples. -
Use the transformers
library to fine-tune a small model, with options for different models and training parameters. Here, there is a choice to fine-tune different modules separately or to fine-tune a “multi-task” model. -
Finally, replace the lm
in the module with the fine-tuned model, completing the optimization.
Pipeline Structure Optimization
Currently, there is only one Ensemble
that allows for manually defining various logics and integrating them together, deciding the final result at runtime through a voting-like method.
import dspy
from dspy.teleprompt import Ensemble
# Assume a list of programs
programs = [program1, program2, program3, ...]
# Define Ensemble teleprompter
teleprompter = Ensemble(reduce_fn=dspy.majority, size=2)
# Compile to get the EnsembledProgram
ensembled_program = teleprompter.compile(programs)
Of course, this compile itself incurs no overhead, but the runtime will amplify the costs. If it could automatically search for the optimal pipeline structure like NAS, that would be advanced. For instance, could a module be decomposed into subtasks, or could several modules be combined and fine-tuned into a single model to reduce overhead?
How to Choose an Optimizer
The official recommendations are:
-
By default, use BootstrapFewShotWithRandomSearch
. -
If there are fewer than 10 pieces of data, use BootstrapFewShot
. -
If there are about 50 pieces of data, use BootstrapFewShotWithRandomSearch
. -
If there are around 300 pieces of data, use MIPRO
. -
If using a larger model (greater than 7B) and hoping to improve efficiency, use BootstrapFinetune
.
Other Modules
Data, Metric, etc., are relatively easy to understand. One module worth mentioning in DSPy is Assertions. The goal and implementation of this module are quite similar to the guardrails discussed in LLM controllable generation[5]. For example, if we want the generated content from the large model to only be “yes or no”, or the generated length not to exceed 100 characters, it indeed resembles various assertions we write in code. However, in DSPy programs, assertions not only check and throw failures but also attempt to help us auto-fix.
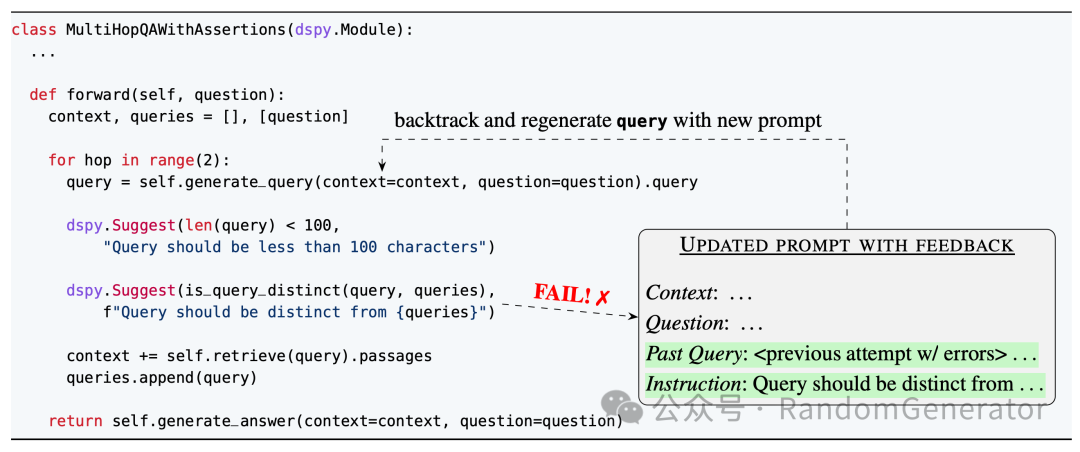
From the image above, it is also relatively easy to understand; we can define various assertions in the program, which will automatically trigger checks during code execution. When a check fails, it will concatenate the previous generation results and the attempted fix instructions, sending them to the LLM for self-refinement. DSPy has two types of assertions: Assertions
can be understood as “hard assertions”; if the fix fails, the program will terminate. Suggest
is a “soft assertion” that continues execution even if the attempt to fix is unsuccessful. Additionally, the previously mentioned TypedPredictor
also uses a similar auto-fix scheme.
There are various scenarios for using such assertions:
-
The most basic capability is to perform various checks during runtime, dynamically modifying the pipeline execution logic when issues arise, such as returning to a previous module to attempt re-execution. -
After enabling assertions, they can help us generate higher-quality samples during prompt sample optimization by removing those that, while correct in result, do not meet our expectations in intermediate steps. -
“Failure cases” that occur during the bootstrap process can also be preserved and later sent to the LLM as part of the prompt to reduce the recurrence of similar errors.
The implementation of the last point is also quite clever. Remember we mentioned earlier that during the bootstrap process, the reasoning process generated by the LLM would also be included in the samples? Because the CoT module altered the signature, the newly added fields will also be automatically saved in the output samples (here is the reasoning). Similarly, during the self-refine process, the signature is dynamically altered, so the inputs and outputs of the fix process will also be preserved in the new samples (previous erroneous attempts, instructions, new outputs, etc.).
Original example:
question: At My Window was released by which American singer-songwriter?
rationale: produce the query. We know that the song "At My Window" was released by an American singer-songwriter, so we need to find the name of the artist.
query: "At My Window" singer-songwriter American artist
Automatically fixed example:
question: At My Window was released by which American singer-songwriter?
past_rationale: produce the query. We know that the song "At My Window" was released by an American singer-songwriter, so we need to find the name of the artist.
past_query: "At My Window" singer-songwriter American artist
rationale: produce the query. We can search for "At My Window singer-songwriter" to find the artist's name.
feedback: Query should be short and less than 40 characters
query: At My Window singer-songwriter
Additionally, DSPy provides some configurations to choose whether to enable assertions during compile and runtime.
Summary of DSPy
Overall, DSPy effectively abstracts various operational elements and optimization methods in the LLM pipeline and has a systematic design. We have drawn multiple analogies with PyTorch in the text; if we were to make a comparison:
-
DSPy encourages us to define the “LLM network” through programming languages, introducing “inductive bias” through complex module combinations to better adapt to different problems. -
Just as in DNN definitions, we do not lay out all model parameters in a single layer FFN but instead make the network “deep”. Similarly, in LLM applications, we cannot solve problems end-to-end with a flat large prompt; we also need meticulous decomposition to deepen the “LLM network”. -
Training data remains a core element; garbage in, garbage out still applies in the LLM era.
In terms of optimization methods, while some basic parameters, such as the temperature of the LLM, are not yet supported, it is not difficult to extend support from an architectural perspective. For example:
-
Modules come with LLM configurations, and there are many parts that can be optimized, from model selection to invocation parameters, even advanced adjustments like logit_bias
. -
Since custom metrics can be defined, in theory, we can optimize not just accuracy but also metrics like speed. Methods like Optuna and evolutionary algorithms can fully support black-box optimization. -
Optimization for non-LLM modules should also be possible; for instance, the compile method currently targets all Predictor
modules in the pipeline, but it could be broader to include modules likeRetrieve
.
However, from my experience reading the code and trying it out, there are still some issues if you want to use DSPy in-depth in projects:
-
Executing compile incurs a significant amount of LLM calls, which can be quite costly. -
If you want to implement some flexible customizations, such as the aforementioned self-refine implementation, the threshold is quite high. -
Overall, it leans more towards an academic project, and the code quality is somewhat average.
Other Prompt Optimization Projects
I originally intended to write a short article within 5000 words, but unexpectedly generated so many tokens… Finally, I will briefly mention other prompt optimization projects:
-
gpt-prompt-engineer[6]: Automatically generates prompts, and after running the LLM, generates results. Then conducts pairwise competitions among the prompt generation cases, with the referee also being LLM. Ultimately, it derives ELO scores for ranking. The overall logic is quite simple and leans towards random search. -
AutoPrompt[7]: Users input tasks and initial prompts. The LLM generates test samples, which are manually labeled or model-generated expected outputs. Next, using the current prompt for predictions, conducts result analysis, and optimizes prompts in a continuous loop to improve. -
SAMMO[8]: A prompt optimization research project launched by Microsoft; from a cursory glance at the paper and code, it does quite detailed work on prompt modification, including rewriting, condensing, deleting, and format conversion, as well as generating instructions from samples. However, its popularity and documentation completeness are not as good as DSPy.
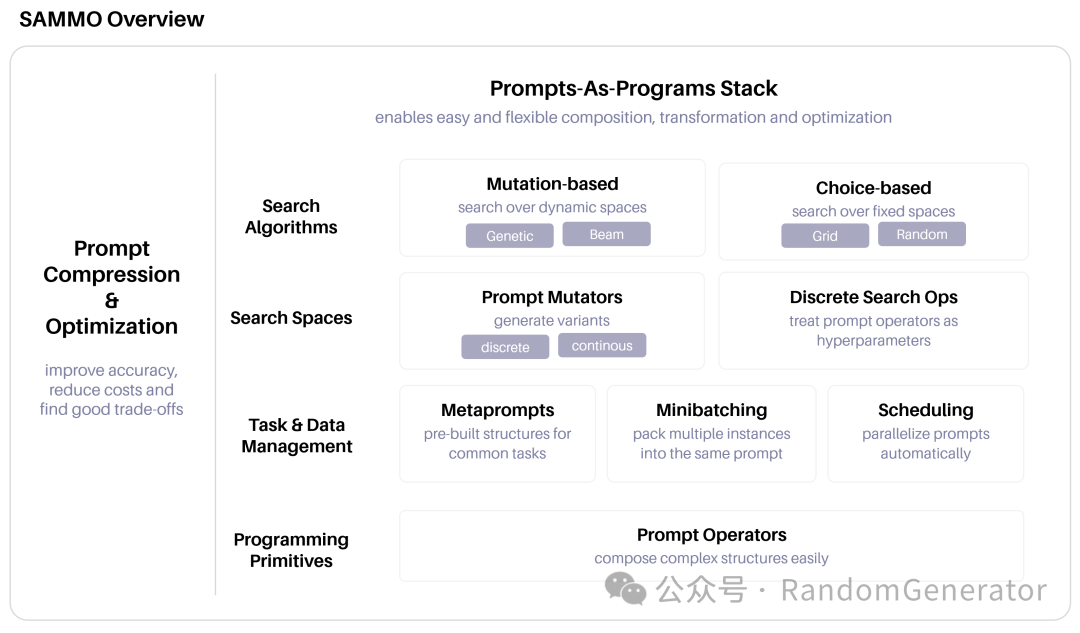
References
RAG: https://www.bilibili.com/video/BV1TC41177rC/
[2]DSPy: https://github.com/stanfordnlp/dspy
[3]Instructor: https://github.com/jxnl/instructor
[4]AutoML: https://zhuanlan.zhihu.com/p/212512984
[5]LLM Controllable Generation: https://zhuanlan.zhihu.com/p/642690763
[6]gpt-prompt-engineer: https://github.com/mshumer/gpt-prompt-engineer
[7]AutoPrompt: https://github.com/Eladlev/AutoPrompt
[8]SAMMO: https://github.com/microsoft/sammo