Now, AI can showcase information from the human brain in the form of high-definition video!
For example, the scenic views you enjoy from the passenger seat can be reconstructed by AI in just a minute:
Even the fish in the water and horses on the grassland are not a problem:
This is the latest research jointly completed by the National University of Singapore and the Chinese University of Hong Kong, with the project named Mind-Video.
This operation resembles how Lucy reads the memories of the villain in the sci-fi movie Lucy:
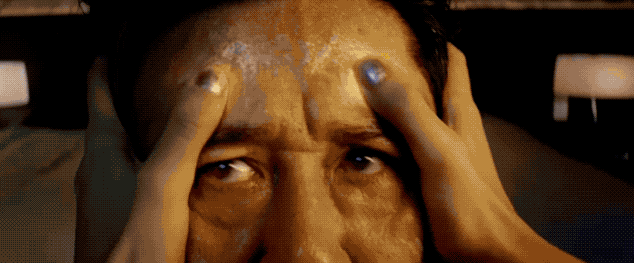
This has led netizens to exclaim:
Advancing the frontiers of artificial intelligence and neuroscience.
It is worth mentioning that the popular Stable Diffusion also played a significant role in this research.
How Was It Achieved?
Reconstructing human visual tasks from brain activity, especially using functional magnetic resonance imaging technology (fMRI), a non-invasive method, has garnered considerable attention in academia.
Such research is beneficial for understanding our cognitive processes.
However, previous studies mainly focused on reconstructing static images, and there has been relatively limited work presented in the form of high-definition video.
The reason for this is that, unlike reconstructing a static image, the scenes, actions, and objects we see are continuous and diverse.
The essence of fMRI technology is to measure blood oxygen level-dependent (BOLD) signals and capture snapshots of brain activity every few seconds.
In contrast, a typical video contains about 30 frames per second; to reconstruct a 2-second video using fMRI, at least 60 frames need to be presented.
Thus, the challenge lies in decoding fMRI and recovering video at a frame rate far exceeding fMRI’s temporal resolution.
To bridge the gap between image and video brain decoding, the research team proposed the MinD-Video method.
Overall, this method consists of two main modules, which are trained separately and then fine-tuned together.
This model learns progressively from brain signals, and during multiple stages in the first module, it gains a deeper understanding of the semantic space.
Specifically, it first utilizes large-scale unsupervised learning and mask brain modeling (MBM) to learn general visual fMRI features.
Then, the team uses a labeled dataset to extract semantically relevant features and trains an fMRI encoder using contrastive learning in the language-image pre-training (CLIP) space.
In the second module, the team fine-tunes the learned features through co-training with an enhanced Stable Diffusion model, which is specifically tailored for video generation under fMRI technology.
With this method, the team compared their results with many previous studies, clearly showing that the images and videos generated by the MinD-Video method are of significantly higher quality than other methods.
Moreover, during the continuous changes in the scene, it can also present high-definition, meaningful continuous frames.
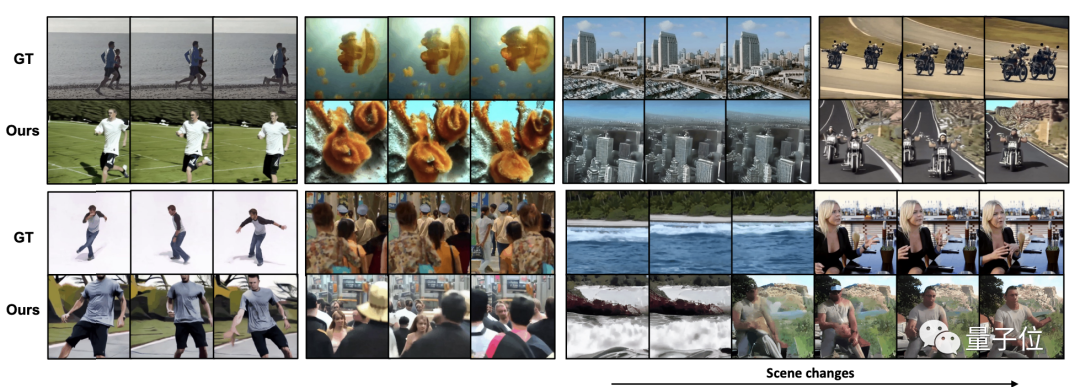
Research Team
This study’s co-first authors include a PhD student from the National University of Singapore, Zijiao Chen, who is currently in the university’s Multimodal Neuroimaging Laboratory for Neuropsychiatric Disorders (MNNDL_Lab).
The other co-first author is Jiaxin Qing from the Chinese University of Hong Kong, majoring in the Department of Information Engineering.
Additionally, the corresponding author is Associate Professor Juan Helen ZHOU from the National University of Singapore.
This new research is an extension of their previous work on MinD-Vis, which focused on functional magnetic resonance imaging image reconstruction.
MinD-Vis has been accepted by CVPR 2023.
Source: Quantum Bits
References: [1]https://mind-video.com/[2]https://twitter.com/ZijiaoC/status/1660470518569639937[3]https://arxiv.org/abs/2305.11675
Shenzhen Longgang Intelligent Audiovisual Research Institute
Artificial Intelligence | Ultra HD
Industry Innovation | Technology Incubation | Achievement Transformation