BiSeNet, which utilizes attention mechanisms in semantic segmentation, has two modules: the FFM module and the ARM module. Its implementation is quite straightforward, but the author has a deep understanding of the attention mechanism and proposes a novel feature fusion method through the FFM module.
One
Introduction
-
The spatial path is used to retain semantic information and generate higher resolution feature maps (reducing the number of down-sampling). -
The context path employs a fast down-sampling strategy to obtain sufficient receptive fields. -
An FFM module is proposed, combining the attention mechanism for feature fusion.
Two
Analysis
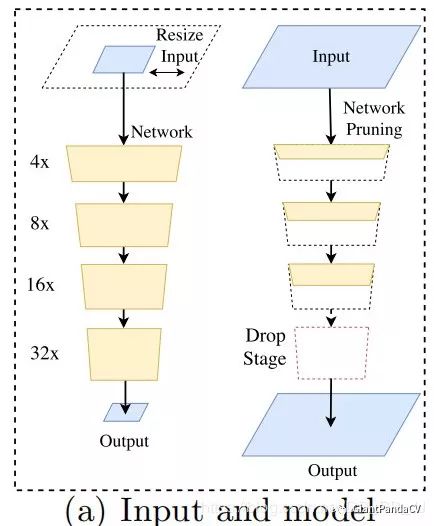
-
Limiting the input size through resizing to reduce computational complexity.The downside is that spatial details are lost, especially at the boundaries. -
Speeding up processing by reducing the number of network channels.The downside is that it weakens spatial information. -
Abandoning down-sampling in the last stage (e.g., ENet).The downside is that the model’s receptive field is insufficient to cover large objects, leading to poor discrimination ability.
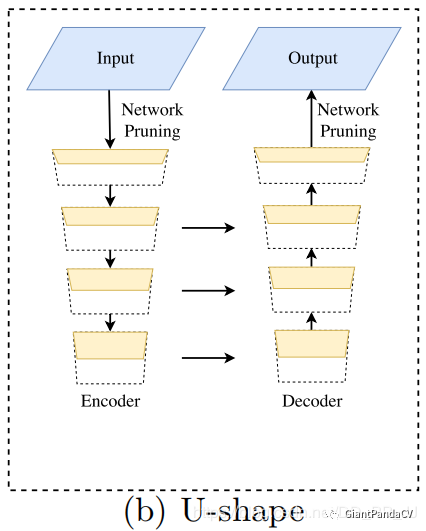
-
High-resolution feature maps have a very large computational load, affecting computation speed. -
The spatial information lost due to resizing or reducing network channels cannot be easily restored by introducing shallow layers.
Three
Details
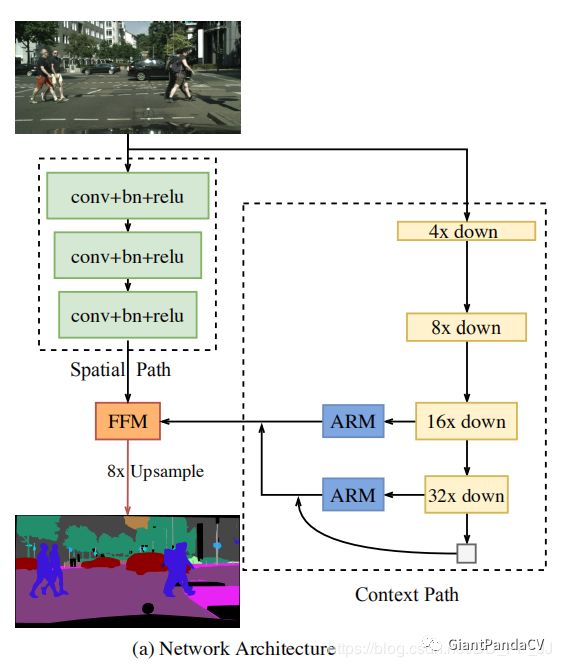
class ConvBlock(torch.nn.Module): def __init__(self, in_channels, out_channels, kernel_size=3, stride=2, padding=1): super().__init__() self.conv1 = nn.Conv2d(in_channels, out_channels, kernel_size=kernel_size, stride=stride, padding=padding, bias=False) self.bn = nn.BatchNorm2d(out_channels) self.relu = nn.ReLU() def forward(self, input): x = self.conv1(input) return self.relu(self.bn(x))class Spatial_path(torch.nn.Module): def __init__(self): super().__init__() self.convblock1 = ConvBlock(in_channels=3, out_channels=64) self.convblock2 = ConvBlock(in_channels=64, out_channels=128) self.convblock3 = ConvBlock(in_channels=128, out_channels=256) def forward(self, input): x = self.convblock1(input) x = self.convblock2(x) x = self.convblock3(x) return x
class resnet18(torch.nn.Module): def __init__(self, pretrained=True): super().__init__() self.features = models.resnet18(pretrained=pretrained) self.conv1 = self.features.conv1 self.bn1 = self.features.bn1 self.relu = self.features.relu self.maxpool1 = self.features.maxpool self.layer1 = self.features.layer1 self.layer2 = self.features.layer2 self.layer3 = self.features.layer3 self.layer4 = self.features.layer4 def forward(self, input): x = self.conv1(input) x = self.relu(self.bn1(x)) x = self.maxpool1(x) feature1 = self.layer1(x) # 1 / 4 feature2 = self.layer2(feature1) # 1 / 8 feature3 = self.layer3(feature2) # 1 / 16 feature4 = self.layer4(feature3) # 1 / 32 # global average pooling to build tail tail = torch.mean(feature4, 3, keepdim=True) tail = torch.mean(tail, 2, keepdim=True) return feature3, feature4, tail
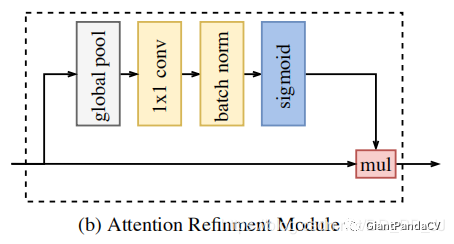
class AttentionRefinementModule(torch.nn.Module): def __init__(self, in_channels, out_channels): super().__init__() self.conv = nn.Conv2d(in_channels, out_channels, kernel_size=1) self.bn = nn.BatchNorm2d(out_channels) self.sigmoid = nn.Sigmoid() self.in_channels = in_channels self.avgpool = nn.AdaptiveAvgPool2d(output_size=(1, 1)) def forward(self, input): # global average pooling x = self.avgpool(input) assert self.in_channels == x.size( 1), 'in_channels and out_channels should all be {}'.format( x.size(1)) x = self.conv(x) # x = self.sigmoid(self.bn(x)) x = self.sigmoid(x) # channels of input and x should be same x = torch.mul(input, x) return x
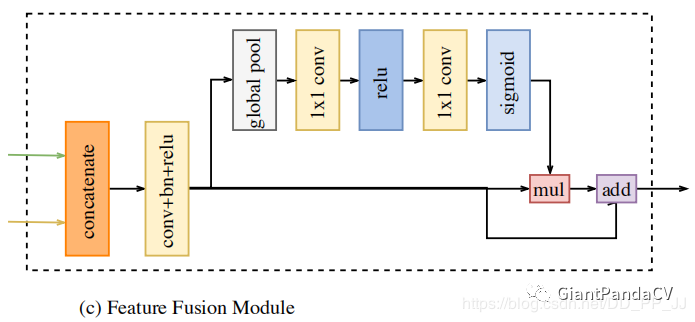
class FeatureFusionModule(torch.nn.Module): def __init__(self, num_classes, in_channels): super().__init__() self.in_channels = in_channels self.convblock = ConvBlock(in_channels=self.in_channels, out_channels=num_classes, stride=1) self.conv1 = nn.Conv2d(num_classes, num_classes, kernel_size=1) self.relu = nn.ReLU() self.conv2 = nn.Conv2d(num_classes, num_classes, kernel_size=1) self.sigmoid = nn.Sigmoid() self.avgpool = nn.AdaptiveAvgPool2d(output_size=(1, 1)) def forward(self, input_1, input_2): x = torch.cat((input_1, input_2), dim=1) assert self.in_channels == x.size( 1), 'in_channels of ConvBlock should be {}'.format(x.size(1)) feature = self.convblock(x) x = self.avgpool(feature) x = self.relu(self.conv1(x)) x = self.sigmoid(self.conv2(x)) x = torch.mul(feature, x) x = torch.add(x, feature) return x
class BiSeNet(torch.nn.Module): def __init__(self, num_classes, context_path): super().__init__() self.spatial_path = Spatial_path() self.context_path = build_contextpath(name=context_path) if context_path == 'resnet101': self.attention_refinement_module1 = AttentionRefinementModule( 1024, 1024) self.attention_refinement_module2 = AttentionRefinementModule( 2048, 2048) self.supervision1 = nn.Conv2d(in_channels=1024, out_channels=num_classes, kernel_size=1) self.supervision2 = nn.Conv2d(in_channels=2048, out_channels=num_classes, kernel_size=1) self.feature_fusion_module = FeatureFusionModule(num_classes, 3328) elif context_path == 'resnet18': self.attention_refinement_module1 = AttentionRefinementModule( 256, 256) self.attention_refinement_module2 = AttentionRefinementModule( 512, 512) self.supervision1 = nn.Conv2d(in_channels=256, out_channels=num_classes, kernel_size=1) self.supervision2 = nn.Conv2d(in_channels=512, out_channels=num_classes, kernel_size=1) self.feature_fusion_module = FeatureFusionModule(num_classes, 1024) else: print('Error: unsupported context_path network \n') self.conv = nn.Conv2d(in_channels=num_classes, out_channels=num_classes, kernel_size=1) def forward(self, input): sx = self.spatial_path(input) cx1, cx2, tail = self.context_path(input) cx1 = self.attention_refinement_module1(cx1) cx2 = self.attention_refinement_module2(cx2) cx2 = torch.mul(cx2, tail) cx1 = torch.nn.functional.interpolate(cx1, size=sx.size()[-2:], mode='bilinear') cx2 = torch.nn.functional.interpolate(cx2, size=sx.size()[-2:], mode='bilinear') cx = torch.cat((cx1, cx2), dim=1) if self.training == True: cx1_sup = self.supervision1(cx1) cx2_sup = self.supervision2(cx2) cx1_sup = torch.nn.functional.interpolate(cx1_sup, size=input.size()[-2:], mode='bilinear') cx2_sup = torch.nn.functional.interpolate(cx2_sup, size=input.size()[-2:], mode='bilinear') result = self.feature_fusion_module(sx, cx) result = torch.nn.functional.interpolate(result, scale_factor=8, mode='bilinear') result = self.conv(result) if self.training == True: return result, cx1_sup, cx2_sup return result
Four
Experiments
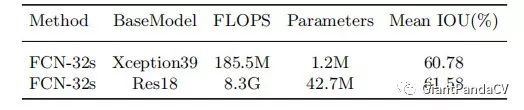
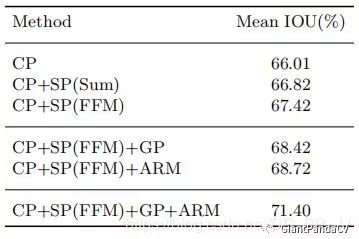
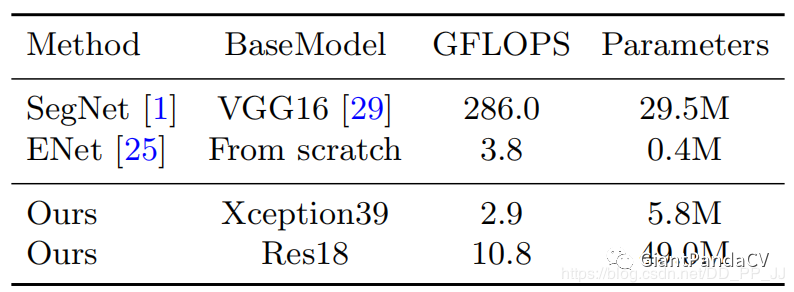
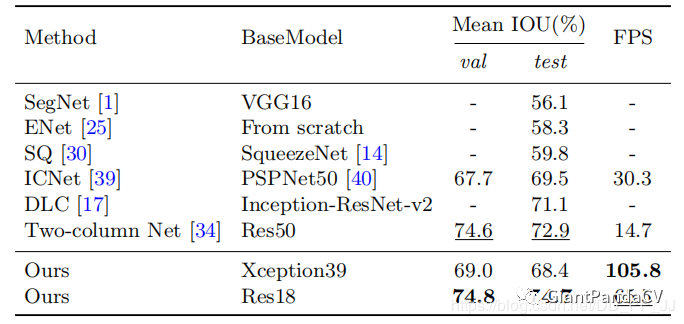
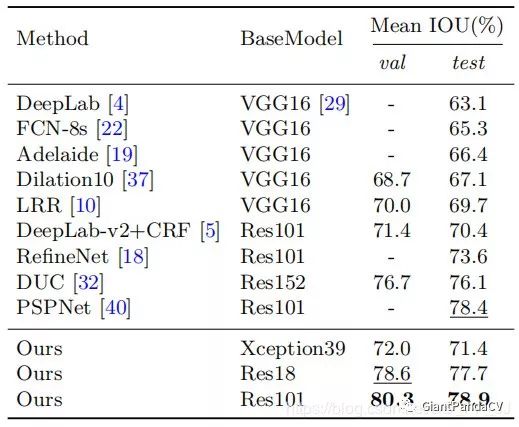
Five
Conclusion
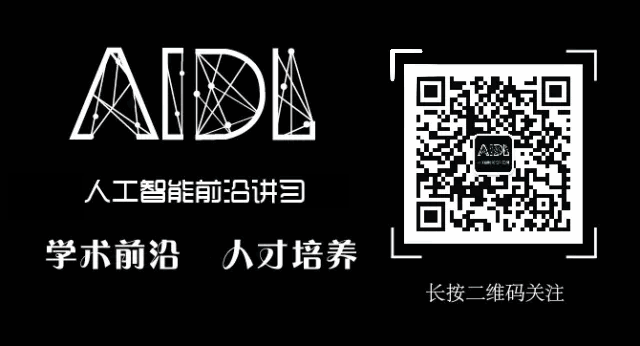

Recommended Historical Articles
-
Does CNN Really Need Down-Sampling (Up-Sampling)?
-
What Can AI Do in the Face of the New Pneumonia Epidemic?
-
The Past, Present, and Future of Human Pose Estimation
-
Top 10 Reviews of 2018-2019
-
[Awesome] Few-Shot Learning Paper Reading List
-
What Experiences Do You Have in Tuning Deep Learning (RNN, CNN)?
-
Advice for New Researchers: Just Reading Papers Is Not Enough; You Must Read Books, Read Books, Read Books!
-
So Young Sohn: Overview of AI Technology in Credit Rating and Patent Protection
-
Shai Ben-David: Flowers and Thorns in Unsupervised Learning
-
It’s Not That We Prefer the New Over the Old, but RAdam Is Indeed Useful, the New State-of-the-Art Optimizer RAdam
-
Evaluation Metrics in Machine Learning
-
CVPR2019 | Capsule Networks Overview, Including 93 Pages of PPT Download
-
AiLearning: A Chinese Machine Learning Resource with Over Ten Thousand Stars on GitHub
-
Summary of Data Augmentation Methods in Deep Learning
Are You Watching? 👇