
In the field of maritime transportation, ensuring the safe navigation of vessels is crucial. To prevent maritime accidents, Professor Guo Jian’s team from the Information Engineering University of the People’s Liberation Army of China has developed a novel anomaly detection technology for ship trajectories based on artificial intelligence. This technology utilizes a model known as the Transformer-LSTM encoder-decoder, which combines the parallel processing capabilities of Transformers and the temporal analysis capabilities of LSTM (Long Short-Term Memory networks) to more effectively capture abnormal behaviors in vessel navigation.
By analyzing large amounts of data collected from the Automatic Identification System (AIS) for ships, this model can learn normal navigation patterns and identify trajectories that deviate from the norm. When the detected trajectory significantly differs from the learned normal patterns, the system marks it as anomalous, thereby issuing timely warnings to help monitoring personnel take appropriate actions.
The advantage of this technology lies in its ability to learn without the need for manually labeled data, allowing for unsupervised learning, which greatly enhances efficiency and practicality in real-world applications. Experimental results show that compared to traditional anomaly detection methods, the Transformer-LSTM-based model significantly improves accuracy, recall, and F1 score, demonstrating its superior performance in ship trajectory anomaly detection. With continuous technological advancements, there is hope for achieving faster and more precise online detection of vessel anomalies, providing stronger safeguards for maritime safety.
Paper Recommendation
Anomaly Detection Method for Ship Trajectories Based on Transformer-LSTM
Li Kexin, Guo Jian, Li Ranchong, Wang Yujun, Li Zongming, Miao Kun
Journal of Chinese Ship Research, 2024, 19(1)
Reference Link (Click to Read Full Text):Li Kexin, Guo Jian, Li Ranchong, et al. Anomaly Detection Method for Ship Trajectories Based on Transformer-LSTM [J]. Journal of Chinese Ship Research, 2024, 19(2): 223–232.
● Main Content of the Paper
Research Background:
Objective:
Method:
Results:
Conclusion:
Future Outlook:
Transformer-LSTM Model for Ship Anomaly Detection Architecture
For more exciting content, please click the original link below to view
Anomaly Detection Method for Ship Trajectories Based on Transformer-LSTM
http://www.ship-research.com/cn/article/doi/10.19693/j.issn.1673-3185.03291
Previous Highlights:
1. [Virtual Special Topic] Recommendations on Unmanned Ship Technology
2. [Virtual Special Recommendations] Digital Twin and Augmented Reality Technology for Ships
3. [Special Recommendations] Ship Acoustic Stealth Technology
4. Excellent Paper Recommendation | Professor Wang Ning et al.: Robust Long-Term Tracking of Visual Targets for Unmanned Boats with Multi-Feature Fusion
5. [Paper Recommendation] Professor Peng Zhouhua et al.: Optimal Coverage Control of Unmanned Ship Swarms under Interference | Special Topic on Unmanned Marine Vehicles Technology
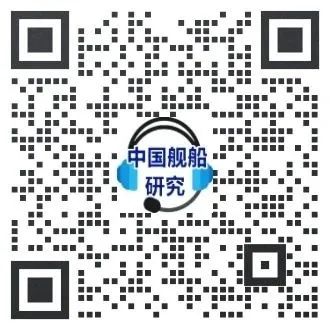
Enterprise WeChat
QR Code
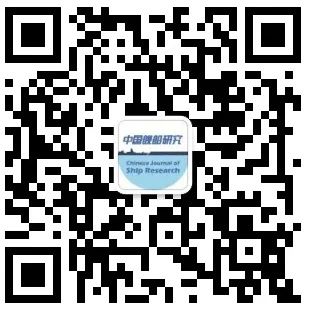
WeChat Official Account
QR Code
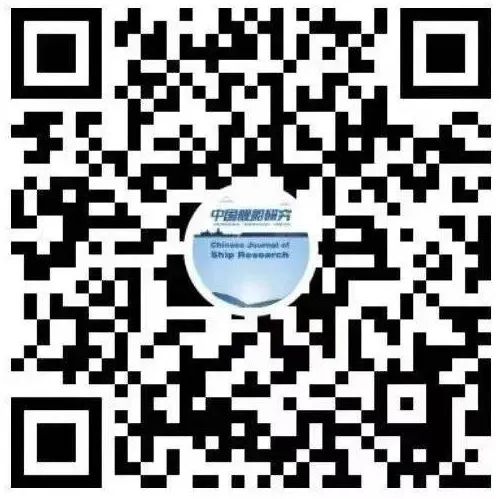
WeChat Video Account
QR Code
Contact Us:
Edit Department WeChat ID: zgjcyjbj
Website: www.ship-research.com
Email: [email protected]
SCOPUS Indexed Journal JST Indexed Journal DOAJ Indexed Journal CSCD Source Journal Chinese Core Journal RCCSE Chinese Core Academic Journal Chinese Quality Technology Journal Hubei Province’s Most Influential Academic Journal Top Ten Famous Journals in Hubei |
Journal of Chinese Ship Research |
Click me to visit the journal website
Click me to submit online
Click me to subscribe to the electronic journal
Welcome to share in the moments✬ Comment function is now open, accepting all forms of criticism and praise
Core Journal “Journal of Chinese Ship Research” academic papers free search and reading