ClickBlue Text| Follow“Electrical Engineering”
Abstract:Short-term power load is highly random and volatile, making it difficult for traditional load forecasting methods to grasp the patterns of short-term load changes. To improve the accuracy of short-term power load forecasting, we propose a method that integrates Complete Ensemble Empirical Mode Decomposition with Adaptive Noise (CEEMDAN), Long Short-Term Memory (LSTM) network, and Convolutional Neural Network (CNN). The original load series is extracted from the dataset, and CEEMDAN is used to decompose it into multiple Intrinsic Mode Functions (IMFs) to reduce instability; the LSTM network analyzes the time series characteristics of each component to obtain multiple forecasting results; these results are then combined and processed through CNN and fully connected layers for feature extraction and data characteristic learning, yielding the final load forecasting result. The proposed method is compared with benchmark models and other methods from literature through practical examples, and the results show that the proposed method can accurately capture the patterns of load changes, achieving an accuracy of 97.32% in the one-day load forecasting problem.
Keywords:Power Load Forecasting; Complete Ensemble Empirical Mode Decomposition with Adaptive Noise; Long Short-Term Memory Network; Convolutional Neural Network; Pearson Correlation Coefficient
Beginning of Main Text
Estimated Reading Time: 12 Minutes
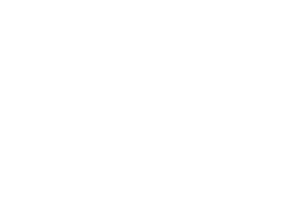
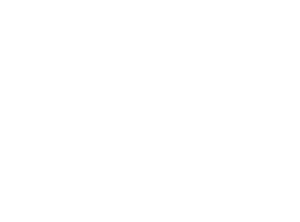
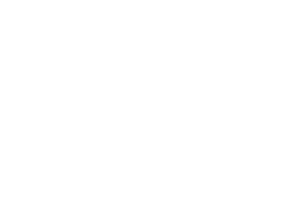
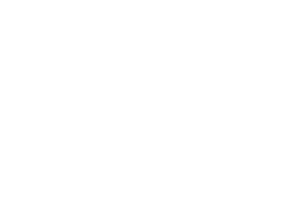
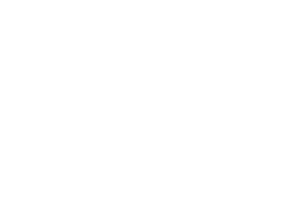
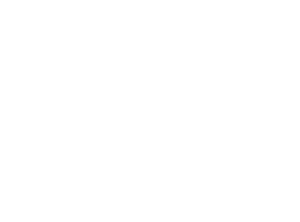
Thank you for reading “Electrical Engineering”. We look forward to your enthusiastic submissions!