Li Haopeng, Wang Yu, Yao Shanglong
Department of Anesthesiology, Union Hospital, Tongji Medical College, Huazhong University of Science and Technology, Wuhan 430022
International Journal of Anesthesiology and Resuscitation, 2023, 45(03): 313-316.
DOI: 10.3760/cma.j.cn321761-20230914-01001
REVIEW ARTICLES
[Review]
Computer Vision (CV) technology has been widely applied in various fields of medical diagnosis and treatment. In recent years, traditional CV methods and deep learning algorithms have continuously progressed and begun to be applied in the field of anesthesiology. Anesthesiologists need to make clinical decisions based on different medical images and physiological parameters during the perioperative period, which is crucial for ensuring patient safety and surgical outcomes. However, traditional methods that rely on subjective judgment have certain limitations. CV can achieve automatic detection and recognition by analyzing medical images, providing effective support for anesthesiologists. This article systematically explores the advancements of CV in the field of anesthesiology, including but not limited to airway management, nerve block and puncture, and depth of anesthesia (DoA) monitoring. To provide a comprehensive perspective, it will also discuss the key issues and challenges faced by CV in medical applications, covering various aspects such as data quality, insufficient labeling, algorithm generalization, interpretability, and reliability. This article aims to review these applications and challenges for CV in anesthesiology research and clinical application.

1 Principles and Tasks of CV
CV helps computers understand and interpret digital images and video content by simulating the human visual system, following a basic process of image acquisition, preprocessing, feature extraction, model training, and testing. CV is mainly divided into traditional CV methods and deep learning algorithms (Table 1). Traditional CV methods rely on manually designed and selected features, using classical machine learning algorithms for model training, such as support vector machines, random forests, K-nearest neighbors, etc. These methods have lower computational requirements and are suitable for handling small-scale and low-dimensional data. However, because features depend on human experience and domain knowledge, they may not fully express the complexity and diversity of images. Deep learning algorithms, on the other hand, use multi-layer neural networks to automatically learn features and conduct end-to-end model training, such as convolutional neural networks (CNN), recurrent neural networks, and long short-term memory networks. They can adaptively learn features from data, capturing high-level semantic information and abstract representations of images, with feature extraction and model training being unified, allowing for joint optimization. However, this also requires a large amount of precise labeled data and strong computational support.
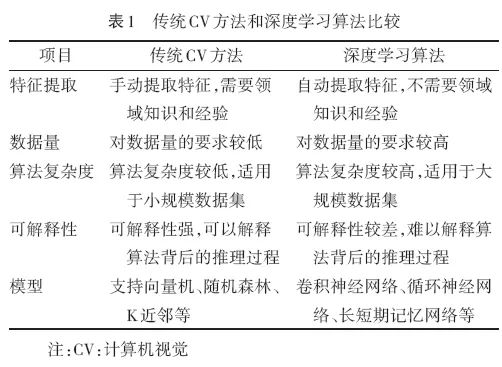

2 Applications of CV in Anesthesiology
In recent years, CV has been widely applied in the medical field. In clinical anesthesia, CV can assist anesthesiologists in airway management, nerve block and puncture, DoA monitoring, and early warning for anesthesia and perioperative complications, thereby enhancing the safety and efficiency of anesthesia.
2.1 Airway Assessment
Traditional airway assessment (common indicators include Mallampati score, Cormack Lehane classification, etc.) relies on the subjective judgment and experience of clinical physicians. Insufficient detection of difficult airways can lead to serious complications and even death. CV can objectify and quantify these indicators. Currently, Zhang et al. proposed an AI-based image analysis algorithm to analyze patient images for automating the Mallampati score. Aguilar et al. categorized the Mallampati score into high-risk and low-risk categories to improve the accuracy of airway assessment and reduce intubation complications. Cuendet et al. proposed a fully automatic and non-invasive difficult airway detection system that collects patient photos, videos, and baseline data to learn facial statistical models for automatic parameterization of facial morphology. They used the random forest algorithm to rank the importance of the most distinguishing morphological features and trained classifiers using these features to detect morphological features associated with difficult airways, thus enhancing predictive capability. The AI model based on CNN introduced by Hayasaka et al. can predict the risk of difficult airways by analyzing lateral photos of the supine closed-mouth facial images of patients.
2.2 Establishing and Maintaining Artificial Airways
Endotracheal intubation is a technically demanding and high-risk procedure, and errors may lead to airway injury, aspiration, reduced oxygen saturation, and other complications. During the process of establishing and maintaining the airway, CV can assist anesthesiologists. For example, Carlson et al. used CV to guide and monitor the endotracheal intubation process. By using deep learning algorithms, they automatically identified the glottis from endoscopic images, providing real-time feedback on the intubation position and depth, thereby improving the success rate of endotracheal intubation. Biro et al. achieved real-time image comparison recognition by pre-storing glottis images in a database. In manual mode, the endoscope is slowly and steadily advanced to the oropharynx, and after the endoscope recognizes the glottis structure, it automatically inserts the tracheal tube into the airway for automatic intubation.
2.3 Nerve Block and Puncture
Ultrasound-guided nerve block has become standard practice, but the effectiveness of the block relies on the skills and experience of the anesthesiologist. By using CV, it is possible to automatically identify structures such as nerves, blood vessels, and needle tips from ultrasound images, providing real-time visual feedback to guide anesthesiologists for more accurate and safer nerve blocks. In the study of brachial plexus segmentation, Tian et al. applied deep learning algorithms for automatic segmentation of brachial plexus nerves in ultrasound images and comprehensively evaluated the performance of different deep learning models in brachial plexus ultrasound image segmentation. Mbarki et al. proposed a CNN-based classification method for lumbar intervertebral discs, using magnetic resonance imaging for training and testing, which can classify lumbar intervertebral discs and assist physicians in accurately and quickly completing lumbar punctures.
2.4 DoA Monitoring
DoA monitoring mainly relies on physiological parameters (such as heart rate, blood pressure, etc.) and clinical observations (such as pupil reaction, muscle tone, etc.), but these indicators have certain delays and non-specificities. Clinically, the bispectral index is used to assess DoA based on electroencephalogram (EEG) signals, and the monitoring effects are influenced by drugs and other factors. Therefore, it is necessary to utilize CV for more accurate and real-time monitoring of DoA to enhance the accuracy of anesthesia state classification. For example, Roy Chowdhury et al. developed a predictive model based on deep learning algorithms to explore predicting DoA through electrocardiogram and pulse wave transduction signals. Afshar et al. proposed a hybrid deep learning structure combining CNN and long short-term memory models to extract features from EEG signals, improving DoA prediction. Madanu et al. employed various decomposition methods to extract features from EEG and, after obtaining the signal values required in image format, used several CNN models to classify DoA based on their bispectral index and signal quality index, achieving an accuracy of 83.2%.
2.5 Early Warning for Anesthesia and Perioperative Complications
CV can assist anesthesiologists in early detection and warning of complications by analyzing medical images or physiological signals. For example, Hannun et al. used deep learning algorithms to analyze electrocardiograms, automatically detecting signs of arrhythmia. Deep learning algorithms are also used to analyze chest X-rays after central venous catheterization to detect pneumothorax. Neumann et al. monitored changes in skin pigmentation or vascular filling using deep learning algorithms, providing warnings for allergic reactions or shock risks. Additionally, Drzazga and Cyganek applied CV to analyze respiratory waveforms. All these indicate that applying CV in patient monitoring during anesthesia allows anesthesiologists to monitor complications more effectively, and timely interventions can enhance patient safety.
2.6 Application of Automated Anesthesia Systems
Anesthesiologists face increasing pressure and professional burnout, negatively impacting patient care and the healthcare system. To address this challenge, some researchers have begun exploring the use of automated systems to assist or replace anesthesiologists, which offers the potential to improve anesthesia quality and safety. CV can provide real-time monitoring and feedback on patient status and surgical processes by analyzing images and physiological signals. For example, Joosten et al. applied a combination of closed-loop anesthesia control systems for DoA, cardiac blood flow, and protective lung ventilation in non-cardiac surgical patients. The closed-loop automated anesthesia system can automatically adjust drug infusion and maintain appropriate DoA based on CV analysis of images and physiological signals. The results showed that the combination of automated anesthesia control systems outperformed manual control and may positively impact cognitive function recovery.
2.7 Anesthesia-Related Teaching and Training
There is a significant gap in practical experience levels among anesthesiologists at different levels of hospitals. CV can provide realistic clinical experiences for medical students and residents in anesthesiology through virtual reality and augmented reality, potentially bridging the gap in practical experience among anesthesiologists at different levels of hospitals and improving the quality of medical services. Casso et al. developed a portable, high-fidelity virtual reality bronchoscopy simulator, while Moo-Young et al. designed a virtual simulator for epidural puncture training to replace current lumbar puncture simulators. Both simulators can provide virtual training and simulation for anesthesia-related procedures and skills (such as endotracheal intubation, lumbar puncture, etc.). The application of these simulators may increase physicians’ practical experience, reduce learning costs, and ultimately improve patient outcomes.
3 Challenges and Future Directions
CV has made some progress in anesthesiology applications, but still faces challenges such as data acquisition, model training, interpretability, and robustness. Strategies to address these challenges include improving data scale and quality, especially by building specialized datasets, and using unsupervised or semi-supervised learning to reduce reliance on labeled data. Enhancing model interpretability, developing models with transparent decision processes, and using visualization and attention mechanisms to reveal internal workings are also essential. Furthermore, integrating these technologies into clinical practice seamlessly, designing user-friendly interfaces, ensuring device compatibility, and providing training for users are necessary. At the same time, protecting patient privacy and data security, adhering to regulations, and employing encryption, data monitoring, and access control to prevent data breaches or misuse are crucial.

4 Limitations of Computer Vision
CV still has many limitations that need to be addressed. Firstly, the labeling process of medical images is not only time-consuming but also requires specialized medical knowledge, limiting the construction of large-scale datasets. Secondly, the decision logic of most current deep learning models lacks transparency, which may raise trust issues in clinical decision-making. Additionally, the generalization ability of models is restricted by different datasets and labeling standards. In terms of system implementation, compatibility and real-time responsiveness are also crucial factors to consider. Lastly, clinical applications must strictly adhere to ethical regulations regarding data security and patient privacy.

5 Summary and Outlook
CV provides a new perspective for understanding and solving problems in anesthesiology, with broad application prospects in areas such as airway management, nerve block and puncture, DoA monitoring, and early warning of perioperative complications. However, to effectively apply CV in clinical practice, several challenges must be overcome, such as data acquisition, model training, interpretability, and robustness. To enhance the performance and safety of CV, future approaches may require the adoption of new methods or technologies such as hybrid learning, transfer learning, and federated learning. Additionally, strengthening collaboration between experts in anesthesiology and computer science is essential to advance the development and innovation of CV in anesthesiology.
If you are interested in this article, please log in to our journal submission system platform (www.gjmzyfs.com) to download the full text for free in the “Journal Browse” section.
International Journal of Anesthesiology and Resuscitation
International Journal of Anesthesiology and Resuscitation
Supervised by: National Health Commission of the People’s Republic of China
Sponsored by: Chinese Medical Association, Xuzhou Medical University
ISSN: 1673-4378 CN: 32-1761/R
Phone: (0516) 85708135 (Consultation); 85807157 (Editing); 85802018 (Fax)
Email: [email protected]; [email protected]
Online Submission: www.gjmzyfs.com
Address: No. 99 Huaihai West Road, Xuzhou City
Long press the QR code
One-click follow
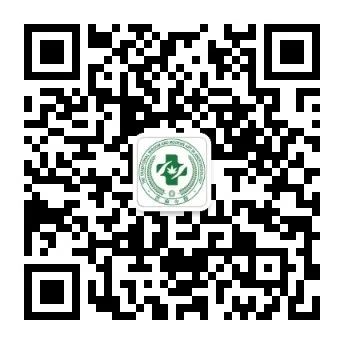
Quickly view the “International Journal of Anesthesiology and Resuscitation” by following the Guma Jinzui Network