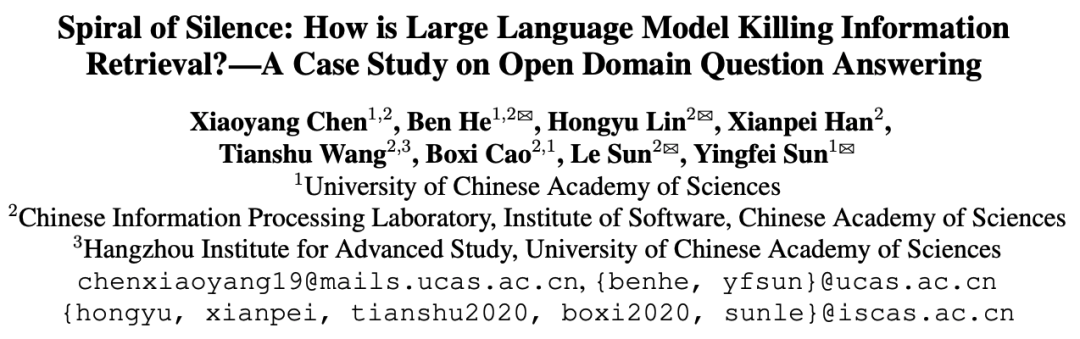
Research Background
-
Research Question: This paper investigates the impact of large language models (LLMs) on retrieval-augmented generation (RAG) systems, focusing on the short-term and long-term effects of LLM-generated text on information retrieval and generation. Specifically, it examines whether LLM-generated text will gradually replace human-generated content, leading to a “spiral of silence” effect in the digital information ecosystem. -
Research Challenges: The challenges of this research include: the rapid dissemination and indexing of LLM-generated text and its impact on the retrieval and generation processes; how to assess the short-term and long-term effects of LLM-generated text on RAG systems; and how to prevent the spread of misinformation and misleading information from LLM-generated content. -
Related Work: Related research includes the analysis of RAG systems, the impact of AIGC, and the application of the “spiral of silence” theory. Studies on RAG systems show that retrieval plays an important role in enhancing the effectiveness of language models. Research on AIGC focuses on the social and technological impacts of AI-generated content, particularly concerning misinformation and bias.
Research Methodology
-
RAG System Modeling: The RAG system can be formalized as a function, where is the set of queries, is the set of documents, is the knowledge base of the LLM, and is the set of text generated by the system. The RAG system consists of a retrieval phase and a generation phase, achieved through the retrieval function and the generation function . -
Simulation Process: The simulation process begins with a dataset of purely human-generated text, gradually introducing LLM-generated text to observe its impact on the RAG system. The specific steps include:
-
Baseline Establishment: Establishing the performance of the baseline RAG pipeline using the initial dataset . -
Zero-Shot Text Introduction: Adding LLM-generated zero-shot text to the dataset, generating a new dataset . -
Retrieval and Re-ranking: For each query, obtaining a subset of documents through the retrieval function and performing re-ranking. -
Generation Phase: Using LLM to generate answer text. -
Post-Processing Phase: Removing text fragments that may expose the identity of the LLM. -
Index Update: Adding the generated text to the dataset and updating the index. -
Iterative Operation: Repeating the above steps until the desired number of iterations is reached.
Experimental Design
-
Datasets and Metrics: The experiments used commonly used open-domain question answering (ODQA) datasets, including NQ, WebQ, TriviaQA, and PopQA. Metrics for evaluating the retrieval phase include Acc@5 and Acc@20, while the generation phase is evaluated using Exact Match (EM) metrics. -
Retrieval and Re-ranking Methods: The experiments employed various retrieval methods, including the sparse model BM25, contrastive learning-based dense retriever Contriever, advanced BGEBase retriever, and LLMEmbedder. Re-ranking methods included T5-based MonoT5-3B, UPR-3B, and BGEreranker. -
Generation Models: The experiments combined text generated by various popular LLMs, including GPT-3.5-Turbo, LLaMA2-13B-Chat, Qwen-14B-Chat, Baichuan2-13B-Chat, and ChatGLM3-6B.
Results and Analysis
-
Short-Term Effects:
-
The introduction of LLM-generated text has an immediate impact on the retrieval and generation performance of the RAG system. Retrieval accuracy generally improves, but QA performance varies. -
Specific data indicate that using BM25 improved Acc@5 by 31.2% and Acc@20 by 19.1% on the TriviaQA dataset. -
LLM-generated text improved retrieval accuracy in most cases but could also negatively impact QA performance.
-
Long-Term Effects:
-
As the number of iterations increases, the effectiveness of retrieval generally declines, while QA performance remains stable. -
For example, on the NQ dataset, the average Acc@5 decreased by 21.4% from the first iteration to the tenth. -
QA performance did not decline with the decrease in retrieval accuracy; EM values fluctuated within a small range but remained generally stable.
-
Spiral of Silence Phenomenon:
-
The retrieval model tends to prioritize LLM-generated text, leading to a gradual decline in the status of human-generated text in search results. -
After ten iterations, the proportion of human-generated text in all datasets dropped below 10%. -
Over time, the trend of opinion homogenization intensified, leading to a decrease in both the diversity and accuracy of retrieval results.
Overall Conclusion
Scan the QR code to add the assistant’s WeChat