Author: Deephub Imba
This article is about 3000 words long, and it is recommended to read it in 7 minutes.
This article will introduce four strategies for optimizing Retrieval-Augmented Generation (RAG) using private data.
Brief Review of RAG
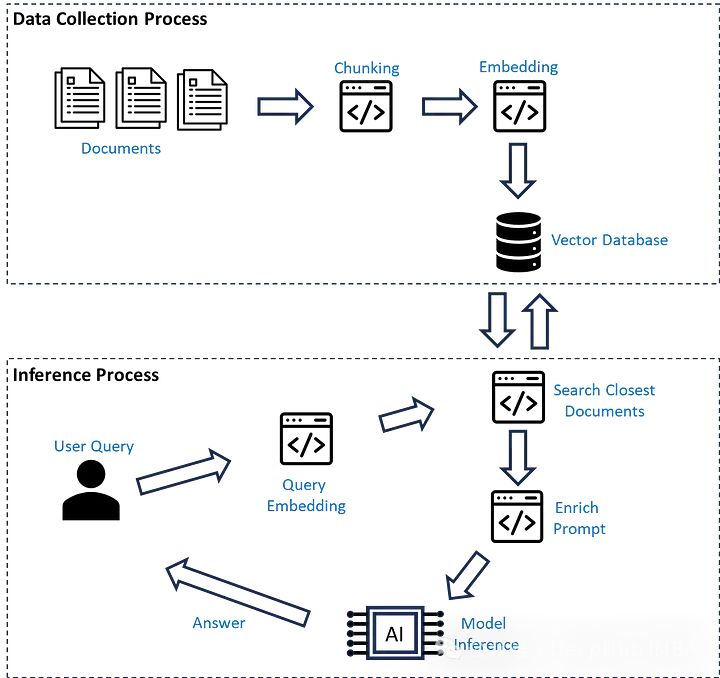
A/B Testing in RAG
Next, we will start using LangChain to implement the RAG process. First, we will install the libraries:
pip install ollama==0.2.1 pip install chromadb==0.5.0 pip install transformers==4.41.2 pip install torch==2.3.1 pip install langchain==0.2.0 pip install ragas==0.1.9
Below is a code snippet using LangChain:
# Import necessary libraries and modules
from langchain.embeddings.base import Embeddings
from transformers import BertModel, BertTokenizer, DPRQuestionEncoder, DPRQuestionEncoderTokenizer, RobertaModel, RobertaTokenizer
from langchain.prompts import ChatPromptTemplate
from langchain_text_splitters import MarkdownHeaderTextSplitter
import requests
from langchain_chroma import Chroma
from langchain import hub
from langchain_core.runnables import RunnablePassthrough
from langchain_core.output_parsers import StrOutputParser
from langchain_community.chat_models import ChatOllama
from operator import itemgetter
# Define a custom embedding class using the DPRQuestionEncoder class
class DPRQuestionEncoderEmbeddings(Embeddings):
show_progress: bool = False
"""Whether to show a tqdm progress bar. Must have `tqdm` installed."""
def __init__(self, model_name: str = 'facebook/dpr-question_encoder-single-nq-base'):
# Initialize the tokenizer and model with the specified model name
self.tokenizer = DPRQuestionEncoderTokenizer.from_pretrained(model_name)
self.model = DPRQuestionEncoder.from_pretrained(model_name)
def embed(self, texts):
# Ensure texts is a list
if isinstance(texts, str):
texts = [texts]
embeddings = []
if self.show_progress:
try:
from tqdm import tqdm
iter_ = tqdm(texts, desc="Embeddings")
except ImportError:
logger.warning(
"Unable to show progress bar because tqdm could not be imported. "
"Please install with `pip install tqdm`."
)
iter_ = texts
else:
iter_ = texts
for text in iter_:
# Tokenize the input text
inputs = self.tokenizer(text, return_tensors='pt')
# Generate embeddings using the model
outputs = self.model(**inputs)
# Extract the embedding and convert it to a list
embedding = outputs.pooler_output.detach().numpy()[0]
embeddings.append(embedding.tolist())
return embeddings
def embed_documents(self, documents):
return self.embed(documents)
def embed_query(self, query):
return self.embed([query])[0]
# Define a template for generating prompts
template = """
### CONTEXT {context}
### QUESTION Question: {question}
### INSTRUCTIONS Answer the user's QUESTION using the CONTEXT markdown text above. Provide short and concise answers. Base your answer solely on the facts from the CONTEXT. If the CONTEXT does not contain the necessary facts to answer the QUESTION, return 'NONE'.
"""
# Create a ChatPromptTemplate instance using the template
prompt = ChatPromptTemplate.from_template(template)
# Fetch text data from a URL
url = "https://raw.githubusercontent.com/cgrodrigues/rag-intro/main/coldf_secret_experiments.txt"
response = requests.get(url)
if response.status_code == 200:
text = response.text
else:
raise Exception(f"Failed to fetch the file: {response.status_code}")
# Define headers to split the markdown text
headers_to_split_on = [
("#", "Header 1")
]
# Create an instance of MarkdownHeaderTextSplitter with the specified headers
markdown_splitter = MarkdownHeaderTextSplitter(
headers_to_split_on, strip_headers=False
)
# Split the text using the markdown splitter
docs_splits = markdown_splitter.split_text(text)
# Initialize a chat model
llm = ChatOllama(model="llama3")
# Create a Chroma vector store from the documents using the custom embeddings
vectorstore = Chroma.from_documents(documents=docs_splits, embedding=DPRQuestionEncoderEmbeddings())
# Create a retriever from the vector store
retriever = vectorstore.as_retriever()
# Define a function to format documents for display
def format_docs(docs):
return "\n\n".join(doc.page_content for doc in docs)
# Create a retrieval-augmented generation (RAG) chain
rag_chain = (
{"context": retriever | format_docs, "question": RunnablePassthrough()}
| RunnablePassthrough.assign(context=itemgetter("context"))
| {"answer": prompt | llm | StrOutputParser(),
"context": itemgetter("context")}
)
# Invoke the RAG chain with a question
result = rag_chain.invoke("Who led the Experiment 1?")
print(result)
Use the following code to evaluate the metrics:
# Import necessary libraries and modules
import pandas as pd
from datasets import Dataset
from ragas import evaluate
from ragas.metrics import (
context_precision,
faithfulness,
answer_relevancy,
context_recall
)
from langchain_community.chat_models import ChatOllama
def get_questions_answers_contexts(rag_chain):
""" Read the list of questions and answers and return a
ragas dataset for evaluation """
# URL of the file
url = 'https://raw.githubusercontent.com/cgrodrigues/rag-intro/main/coldf_question_and_answer.psv'
# Fetch the file from the URL
response = requests.get(url)
data = response.text
# Split the data into lines
lines = data.split('\n')
# Split each line by the pipe symbol and create tuples
rag_dataset = []
for line in lines[1:10]: # Only 10 first questions
if line.strip(): # Ensure the line is not empty
question, reference_answer = line.split('|')
result = rag_chain.invoke(question)
generated_answer = result['answer']
contexts = result['context']
rag_dataset.append({
"question": question,
"answer": generated_answer,
"contexts": [contexts],
"ground_truth": reference_answer
})
rag_df = pd.DataFrame(rag_dataset)
rag_eval_datset = Dataset.from_pandas(rag_df)
# Return the ragas dataset
return rag_eval_datset
def get_metrics(rag_dataset):
""" For a RAG Dataset calculate the metrics faithfulness,
answer_relevancy, context_precision and context_recall """
# The list of metrics that we want to evaluate
metrics = [
faithfulness,
answer_relevancy,
context_precision,
context_recall
]
# We will use our local ollama with the LLaMA 3 model
langchain_llm = ChatOllama(model="llama3")
langchain_embeddings = DPRQuestionEncoderEmbeddings('facebook/dpr-question_encoder-single-nq-base')
# Return the metrics
results = evaluate(rag_dataset, metrics=metrics, llm=langchain_llm, embeddings=langchain_embeddings)
return results
# Get the RAG dataset
rag_dataset = get_questions_answers_contexts(rag_chain)
# Calculate the metrics
results = get_metrics(rag_dataset)
print(results)
{
'faithfulness': 0.8611,
'answer_relevancy': 0.8653,
'context_precision': 0.7778,
'context_recall': 0.8889
}
The first two metrics are model-related; to improve these metrics, it is necessary to change the language model or provide better prompts for the model; the latter two metrics are retrieval-related; to improve these metrics, it is necessary to study the storage, indexing, and selection of documents.
Next, we will begin making improvements.
Chunking
# Import necessary libraries and modules
from langchain.retrievers import ParentDocumentRetriever
from langchain.storage import InMemoryStore
from langchain.text_splitter import RecursiveCharacterTextSplitter
# Create the parent document retriever
parent_document_retriever = ParentDocumentRetriever(
vectorstore = Chroma(collection_name="parents",
embedding_function=DPRQuestionEncoderEmbeddings('facebook/dpr-question_encoder-single-nq-base')),
docstore = InMemoryStore(),
child_splitter = RecursiveCharacterTextSplitter(chunk_size=200),
parent_splitter = RecursiveCharacterTextSplitter(chunk_size=1500),
)
parent_document_retriever.add_documents(docs_splits)
# Create a retrieval-augmented generation (RAG) chain
rag_chain_pr = (
{"context": parent_document_retriever | format_docs, "question": RunnablePassthrough()}
| RunnablePassthrough.assign(context=itemgetter("context"))
| {"answer": prompt | llm | StrOutputParser(),
"context": itemgetter("context")}
)
# Get the RAG dataset
rag_dataset = get_questions_answers_contexts(rag_chain_pr)
# Calculate the metrics
results = get_metrics(rag_dataset)
print(results)

Embedding Models
# Import necessary libraries and modules
import pandas as pd
from datasets import Dataset
from ragas import evaluate
from ragas.metrics import (
context_precision,
faithfulness,
answer_relevancy,
context_recall
)
from langchain_community.chat_models import ChatOllama
from sentence_transformers import SentenceTransformer
# Define a custom embedding class using the Sentence-BERT model
class SentenceBertEncoderEmbeddings(Embeddings):
show_progress: bool = False
"""Whether to show a tqdm progress bar. Must have `tqdm` installed."""
def __init__(self, model_name: str = 'paraphrase-MiniLM-L6-v2'):
# Initialize the tokenizer and model with the specified model name
self.model = SentenceTransformer(model_name)
def embed(self, texts):
# Ensure texts is a list
if isinstance(texts, str):
texts = [texts]
embeddings = []
if self.show_progress:
try:
from tqdm import tqdm
iter_ = tqdm(texts, desc="Embeddings")
except ImportError:
logger.warning(
"Unable to show progress bar because tqdm could not be imported. "
"Please install with `pip install tqdm`.")
iter_ = texts
else:
iter_ = texts
for text in iter_:
embeddings.append(self.model.encode(text).tolist())
return embeddings
def embed_documents(self, documents):
return self.embed(documents)
def embed_query(self, query):
return self.embed([query])[0]
# Create a Chroma vector store from the documents using the custom embeddings
vectorstore = Chroma.from_documents(documents=docs_splits, embedding=SentenceBertEncoderEmbeddings())
# Create a retriever from the vector store
retriever = vectorstore.as_retriever()
# Create a retrieval-augmented generation (RAG) chain
rag_chain_ce = (
{"context": retriever | format_docs, "question": RunnablePassthrough()}
| RunnablePassthrough.assign(context=itemgetter("context"))
| {"answer": prompt | llm | StrOutputParser(),
"context": itemgetter("context")}
)
# Get the RAG dataset
rag_dataset = get_questions_answers_contexts(rag_chain_ce)
# Calculate the metrics
results = get_metrics(rag_dataset)
print(results)

Vector Search Methods
# Import necessary libraries and modules
import pandas as pd
from datasets import Dataset
from ragas import evaluate
from ragas.metrics import (
context_precision,
faithfulness,
answer_relevancy,
context_recall
)
from langchain_community.chat_models import ChatOllama
# Create a Chroma vector store from the documents
# using the custom embeddings and also changing to
# cosine similarity search
vectorstore = Chroma.from_documents(collection_name="dist",
documents=docs_splits,
embedding=DPRQuestionEncoderEmbeddings(),
collection_metadata={"hnsw:space": "cosine"})
# Create a retriever from the vector store
retriever = vectorstore.as_retriever()
# Create a retrieval-augmented generation (RAG) chain
rag_chain_dist = (
{"context": retriever | format_docs, "question": RunnablePassthrough()}
| RunnablePassthrough.assign(context=itemgetter("context"))
| {"answer": prompt | llm | StrOutputParser(),
"context": itemgetter("context")}
)
# Get the RAG dataset
rag_dataset = get_questions_answers_contexts(rag_chain_dist)
# Calculate the metrics
results = get_metrics(rag_dataset)
print(results)
Final Prompts for the Input Model
Conclusion
About Us
Data Hub THU, as a data science public account, backed by the Tsinghua University Big Data Research Center, shares cutting-edge data science and big data technology innovation research dynamics, continuously disseminating data science knowledge, striving to build a data talent aggregation platform, and creating the strongest group of big data in China.
Weibo: @Data Hub THU
WeChat Video Account: Data Hub THU
Today’s Headlines: Data Hub THU