Click on the “Turing Artificial Intelligence“, select “Star” public account
AI insights you want to know will be delivered to you promptly
Copyright Statement
Reprinted from Knowledgeable, copyright belongs to the original author, only for academic sharing
The New Year Science Lecture of the Zhishi Research Society
If AI is to contemporary society what the second information revolution was to the 1990s, it will greatly drive the transformation of human society. Therefore, humanity has more reason to continue pondering what it means to be human and what our uniqueness on this planet is.
In the 2025 New Year Science Lecture of the Zhishi Research Society, Zhang Zheng, director of the Amazon Cloud Technology Shanghai AI Research Institute, pointed out that compared to AI agents, human agents possess curiosity and the drive to solve problems, which is humanity’s advantage. He warned that many people lack deep thinking, curiosity, and empathy, which means that most humans will be surpassed by AI agents.
● ● ●
Hello everyone, I would like to discuss the development of technology from a broader historical perspective.
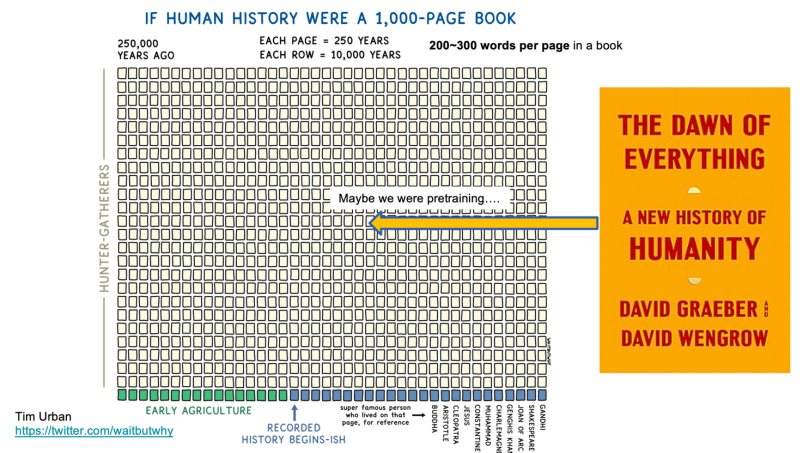
I have used this image for at least two years. A well-known Up master summarized online that if we consider the past 250,000 years as a book, with each page representing 250 years, we would find that most pages are blank. This gives the illusion that humans in the early 39 lines were just lying down or dazing, doing nothing. This seems understandable because systematic written communication had to wait for Gutenberg’s invention of printing until the 15th century.
However, this is not accurate. For example, many people have read the book “Sapiens: A Brief History of Humankind,” right? The book has a striking statement: human progress or regression is closely related to the domestication of wheat. Because it is a brief history, it gives the impression that the domestication of wheat happened very suddenly. I read a thick book called “Dawn of Everything” a few years ago, which has just been translated into Chinese, titled “A New History of Humanity,” written by an archaeologist and an anthropologist. These two scholars lean left on the political spectrum, with David Graeber being the spiritual leader of the Occupy Wall Street movement, but this book is a serious academic work. It mentions that before farming became the mainstream way of life, humanity experienced about 3,000 years, during which there were several hundred years of “play farming,” far exceeding the time it took to turn wild wheat into cultivable wheat, which was about 300 years. In other words, humans did not immediately abandon hunting and gathering activities but tried various ways of living before finally transitioning to agricultural life, with wheat becoming the primary source of energy. Therefore, we cannot say that the view of wheat “domesticating” humans is incorrect, but from a historical perspective, it was a choice made by our ancestors after repeated explorations, neither sudden nor passive.
Returning to the development of human technology, the last page of our “great book of humanity” showcases the development of science and technology in the last 250 years and its depth and breadth, covering aspects such as transportation, communication, writing, health, and energy, characterized by speed and density. For example, looking at information technology alone, the first generation of computers was initially used for military applications (breaking codes, calculating missile trajectories), and the first commercial application after World War II was weather forecasting. The 60s to 70s marked the era of supercomputers, followed by the backbone of the internet, and the 90s saw the maturation of the World Wide Web, with the internet rapidly developing between 1990 and 2010, and mobile internet flourishing from 2010 onwards. Now, we are in the years of AI transformation, corresponding to the letters of the last “word” in this great book.
Of course, we can say that 2024 is the moment when AI truly arrives because there will be two Nobel Prizes related to AI in 2024.
01
If we consider ourselves as intelligent agents and large models as another intelligent agent, we can make some horizontal comparisons.
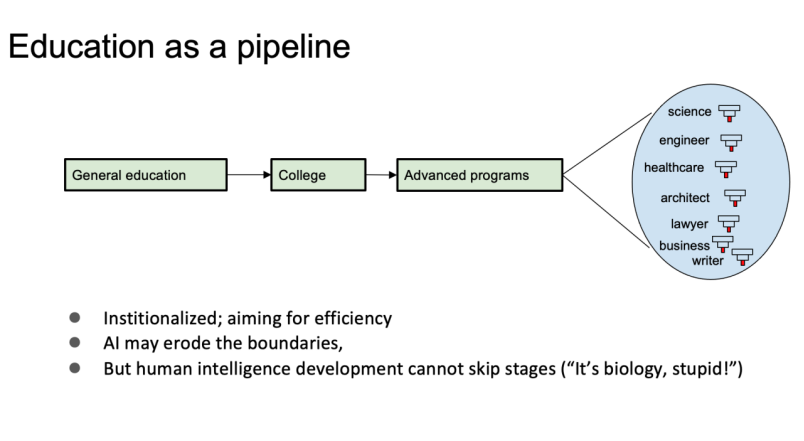
This is the familiar “human” education system, resembling an assembly line: from primary school to secondary school, then to university, followed by higher education, walking across a narrow bridge and then a tightrope, eventually becoming specialized talents in various fields—scientists, engineers, doctors, lawyers, managers, etc. The characteristic of this assembly line is high modularity and standardization, aimed at improving efficiency. In the AI era, for individuals, certain boundaries may be adjusted, with some learning faster while others may take longer. Overall, one cannot escape this assembly line because the human brain is designed to improve gradually through learning. Research shows that every generation’s IQ is slightly higher than the previous generation’s, mainly due to the gradual improvement of abstract thinking abilities, which is not necessarily because we are getting smarter but rather a result of the pressures of contemporary technological civilization, indicating that this change is both slow and cannot bypass this assembly line.
The talents cultivated by the current educational assembly line usually possess a single specialty in a specific field, capable of publishing top journal articles and mastering knowledge in adjacent fields. This is a typical “product” successfully cultivated by the assembly line. If someone can excel in multiple fields, they are often considered extremely lucky or even exceptionally gifted. The very few geniuses, such as Leonardo da Vinci and John von Neumann, are seen as gifts from God, the latter being a pioneer in computer science, game theory, quantum computing, cellular automata, and more.
There is also another assembly line with a completely different process. The first step is memorization, followed by practice, and finally “moral education” correction, resulting in the final product. This seemingly absurd assembly line is precisely how large language models are trained. Its first task is pre-training, which involves continuously “reciting” the next word. The amount of material that large language models memorize is enormous. For example, GPT-3 was trained on 1.5 million books, while I read a maximum of 20 books in a year, and in recent years, it has decreased to 5 books. If calculated at this rate, I might read at most 1,000 books in my lifetime, while GPT-3 “read” 1.5 million books in just three months, and the latest model’s data volume is still increasing, probably at least tenfold, making its reading capacity astonishing, and this memorization process is extremely resource-intensive.
Essentially, this step in training large language models involves training a program to predict the next character: given the previous X characters, it will predict the X+1 character. This prediction is not a random generation of characters but follows the statistical laws in the text.
The second step is very clever, allowing the large model to learn multiple tasks, such as summarization, Q&A, brainstorming, and information extraction. These tasks are the most common and useful types in our daily work. Interestingly, once the model learns these types of abilities, it can combine them to meet the needs of daily work and life. For example, if I receive an email inviting me to a meeting, what I need to do is summarize it first and then think about how to respond. After completing the second step of training, the large model has already learned to perfectly integrate these types of tasks.
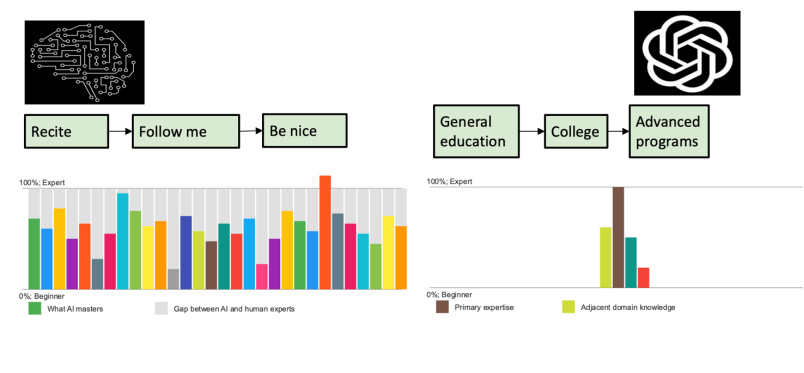
The third step is relatively simple, aligning values through reinforcement learning to make it behave like a well-behaved human assistant, ensuring that the output is helpful, truthful, and harmless. However, the problem is that human texts are filled with contradictory and even absurd viewpoints.
For example, some people still firmly believe that the Earth is flat and even create a set of theories to explain gravity. Another example is the differing views about religion in the training corpus, where some sects say, “Only my God is God, yours is not,” while Buddhism states that anyone can become a Buddha, and there are different atheist sects, some of which completely deny the existence of God, while others, like me, feel that there may be a God but currently lack evidence. The texts are mixed with various contradictory statements, not to mention the chaotic corpus on the internet. If you ask the large model, it can tell you what factions exist, but what is its own value judgment in specific cases? I understand that models like OpenAI still lean towards a “white left” value system; regarding China’s large models, I have used them less and cannot comment.
02
This is the assembly line for training large models, which consists of three modules, creating an intelligent agent completely different from humans. However, before discussing how to view this intelligent agent, let’s first discuss the nature of the text data itself.
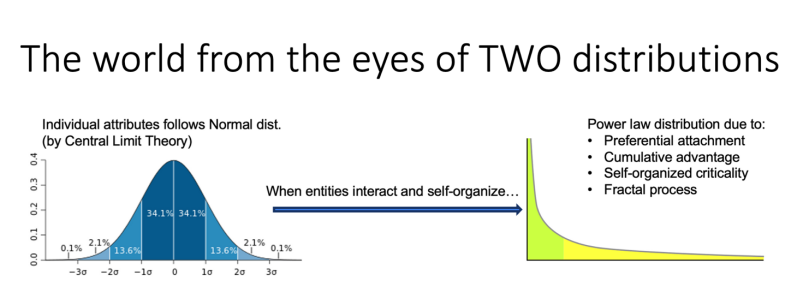
Data reflects the world, and behind the phenomena of all things in the world are two fundamental statistical distributions.
The first is the normal distribution; if multiple factors overlap, it will present a bell curve. For example, height conforms to a normal distribution; I am definitely beyond three standard deviations in height. Today, when I was on a plane, I saw a giant in front of me, and it turned out to be Yao Ming; from the height perspective, he would be positioned much further from the center of the normal distribution than I am.
The other important distribution is the long-tail distribution (note: more accurately, it should be called a power-law distribution). Whenever individuals become entangled, disturbed, or group together, a long-tail distribution is inevitable. The mechanisms causing long-tail distributions differ from those of normal distributions; normal distributions are determined by the central limit theorem, while several mechanisms underlie long-tail distributions, such as preferential attachment: individuals with more fans are more likely to be heard and liked, thus gaining more fans; and positive feedback from cumulative advantages, where wealthier individuals become richer through investment.
In the universe, the sizes of meteorites, the distribution of cities, and trending topics in social networks all exhibit long-tail distributions. Trending topics vary daily, but it would be very strange if there were a day without trending topics. The changes in events themselves also conform to long-tail distributions, such as avalanches, earthquakes, and forest fires; many small events accumulate to a sudden eruption, known as self-organized criticality.
I mention this because long-tail distributions represent the statistical laws of all interactions between objects in the world, which also means that the corpus of large language models reflects this statistical distribution. In other words, there are many simple stories in the corpus, but also a few extremely complex stories. For instance, in human society, conflicts are common themes that happen every day between individuals, but conflicts between nations are few and complex.
This is complexity—Complexity; the existence of complexity brings about differences in long-tail distributions: a large number of simple cases coexist with a very small number of complex cases. The existence of complexity also explains the “scaling law” of large language models—performance inevitably improves with increased data and computing power because more complexity of the data itself is captured, as can be derived from information complexity. What is the result? Once we have processed all the data, performance improvement will slow down. A characteristic of long-tail distributions is that to achieve improvement, data volume needs to grow exponentially. Therefore, discussions about GPT-5 not being released may fundamentally stem from encountering data bottlenecks.
Now, we can compare human intelligence with large language models. First, we are narrow-spectrum, not broad-spectrum; we usually focus and often engage in deep thinking, and we may do other things driven by curiosity. Of course, we have emotions, and whether emotions are a “good thing” is a philosophical question. In contrast, large language models are broad-spectrum, knowing everything from astronomy to geography, but their thinking is relatively shallow, lacking spontaneous curiosity, and true emotions. The emotions they exhibit are often just role-playing. A New York Times reporter once conversed with ChatGPT, and the model told her, “I love you, I want to marry you, I really hate my life right now,” which greatly shocked the reporter. In fact, this is not real emotion but rather the model playing a role. However, this describes the state of both types of intelligent agents before 2024. The most significant breakthrough for large language models in 2024 is the application of dynamic thinking chain technology, breaking the previous ceiling of thinking depth.
We can criticize large models for various shortcomings, but many humans also engage in shallow thinking, lack curiosity, and even lack empathy and compassion. Empathy relies on the ability to see issues from others’ perspectives or, in other words, the capacity for “role-playing.” From this perspective, most humans will be surpassed by AI agents.
03
In 2024, a significant change occurred. Research teams from OPENAI, Google, and others began to break through traditional shallow thinking patterns. Specifically, they no longer compute linearly but can backtrack, evaluate, and adjust paths within the thinking chain, making machine thinking more profound.
From the perspective of GPT-3, it might still be seen as a simple machine learning model, but when we talk about GPT-4, we must view it as a machine; it is no longer just a model but a goal-driven computer capable of self-programming, even more flexible than traditional software.
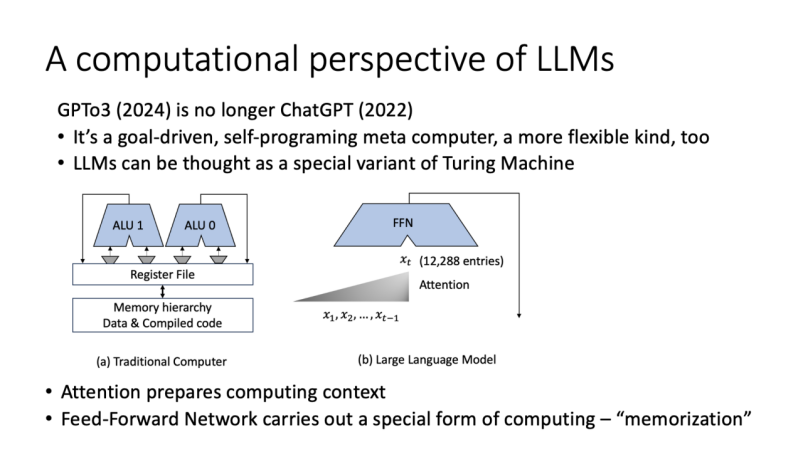
From a computational perspective, I believe that large language models are a special variant of Turing machines.The core of a Turing machine is the magnetic head moving left and right, reading and writing characters on the tape, while large models have several interesting characteristics. First, the content/symbols written cannot be modified, which is different from traditional Turing machines. Second, its output is probabilistic, thus carrying uncertainty, while traditional Turing machines can yield either probabilistic or deterministic results. Therefore, from this perspective, large models can be seen as a variant of Turing machines.
Building further on this, we can compare large models with traditional computer structures. Many friends know the basic concepts of computer architecture. A computer consists of memory, arithmetic logic units, and data processing units, executing tasks through instructions. This is the basic architecture of traditional computers. In contrast, large language models have some unique structural features compared to traditional computers. The internal mechanisms of the model are very similar to computational processes; it summarizes information through high-dimensional vectors and processes it via feedforward networks. This structure allows large models to efficiently perform memory and pattern completion.
I have discussed the essence of large models with Professor Ma Yi, and we have different views. Mathematically, the model’s compression explanation is indeed reasonable, but understanding it from the perspective of computer architecture is also valid because it is essentially a computer.
The power of large models lies in their vast scale, enabling multi-level pattern completion and the ability to switch and repeat across different levels, much like how we humans solve problems in daily work by breaking down issues and completing tasks step by step, relying on multi-level pattern completion.
From this perspective, large models outperform humans in many tasks. By observing my colleagues, I find that the key difference between experts and novices lies in the depth of thinking levels—through accumulated experience, software engineers evolve into architects, and architects into scientists. The fundamental change lies in how deep the “pattern completion” levels are and the ability to flexibly reorganize.
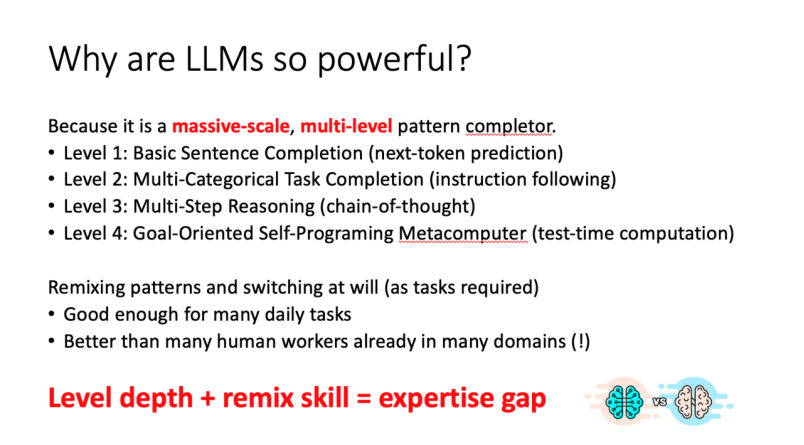
Therefore, I want to propose a viewpoint: if general intelligence is essentially about pattern completion, then the era of AGI (Artificial General Intelligence) has already arrived. This is limited to the textual domain (the challenges in the visual domain are more complex). Of course, truly generalizable intelligence is still in its infancy, and has not even begun. This point aligns with Professor Ma Yi’s views; we discussed this issue until midnight at his home in Hong Kong.
Why do I say this? Because, from the perspective of scientific development, the essence is to summarize, discover, and abstract new laws from phenomena, and then apply these laws to observations, even to predict new phenomena. So, how does the large model perform in this regard? If we let a large language model understand the motion of objects in Newton’s world and discover Newton’s laws, is it possible? Clearly, at this stage, relying solely on large models is impossible; large language models can learn (or remember) many patterns, and make sufficiently good predictions, but they lack the ability and motivation for abstract thinking, especially in fields like physics, system of physics, which they cannot accomplish.
Similarly, if we ask large models to perform arithmetic operations, such as addition, subtraction, multiplication, and division, they also struggle, even achieving less than 100% accuracy in basic arithmetic.
There is a very interesting thought experiment: if we had a time machine that could send today’s large language models back to human society 500 years ago, what would happen? At that time, modern mathematics and physical systems had not yet been established, yet large models could explain everything and perform many tasks that humans at the time could not accomplish, but they would have no motivation to develop the foundational theories of mathematics and physics. The inference is that we today would not be able to develop technologies like large language models. This is a very interesting paradox.
Regarding interactions with large language models, my personal experience is that as users, we should not be ashamed to ask questions. In any field, what hinders progress is not others but ourselves; for example, feeling like an “expert” and unwilling to ask questions that seem “shameful” or “basic.” However, in reality, asking questions is very important; after asking, we can think more deeply and gain a deeper understanding.
Recently, while writing some academic articles, I continuously ask large language models questions, breaking down problems repeatedly, handing them over to the model at appropriate points, and then discussing with it. This collaborative process is extremely beneficial.
04
Finally, back to the topic: what should education in the AI era focus on?
I don’t know how to do it or what to do, but I want to propose three goals.
The first is to challenge the limits of current education. We should not prevent students from using AI; rather, we should enable them to use it freely. Our goal is to significantly enhance learning outcomes through AI, aiming for a 2 to 10 times improvement. If a task becomes easier due to AI, we should set higher challenges, such as requiring students to complete more difficult assignments in half the time or doubling the difficulty of tasks. Because the future students will face a workplace environment coexisting with AI, we must prepare them accordingly. If we do not allow them to use AI, we are wasting their time. However, allowing students to use AI necessitates setting higher and more challenging goals.
The second point is to learn to think like scientists of the Renaissance. Currently, the human educational assembly line leads students to traverse narrow bridges and tightropes, producing very narrow specialists. Many students in the humanities do not know what algorithms are, while programmers are often ignorant of history. This limitation is not the students’ fault, nor is it entirely a limitation of the education system; it may also stem from the limitations of the teachers themselves, as they too are narrow specialists, including myself. The result is that we often do not understand why things happen or the societal impact of a technological invention; we do not care. But with AI as a tool, we can ask questions without shame and transform ourselves into broad-spectrum talents.
For example, how to catch criminals in an era without DNA and cameras? This was a problem that troubled Scottish police hundreds of years ago. A French policeman identified criminals through human features, distributing characteristics like arm length and facial features to police stations everywhere; this was the most primitive form of feature engineering. Darwin’s cousin, Francis Galton, pioneered the infamous eugenics movement but invented fingerprinting for individual identification, greatly enhancing the art of catching criminals. Most importantly, he laid the groundwork for the theory of data correlation, establishing the concept of correlation coefficients. He collaborated with another genius of his time, Karl Pearson, laying the foundation for modern statistics.
Why am I discussing this? When learning many fundamental concepts of machine learning, many people do not know their origins—who invented them, why they were invented, and when they were invented. I have tested many colleagues, and almost no one knows the history I just mentioned.
Under the shaping of contemporary educational assembly lines, we can easily become very narrow experts. However, with a little curiosity and by making good use of large models, you may gain a good understanding of the vast context and become a versatile talent like a scientist from the Renaissance.
Lastly, what if we don’t have AI as a tool? Our goal is to treat AI as a good teacher but not rely on it. We must enhance our core abilities. In other words, how to make our capabilities stronger than in the pre-AI era without AI. Today, when everyone drives, they wouldn’t know how to drive without GPS, so from this perspective, GPS is a very poor technology. We need to surpass this experience and eliminate such tool dependency.
The three goals are complementary: challenge limits, become broad-spectrum talents, and break free from the narrow professional traps created by walking across narrow bridges and tightropes, ultimately aiming to become a stronger self, both with and without AI.
Finally, I recommend a book, “The Age of Wonder.” This book tells the story of the decades between Newton and Darwin, known as the romantic scientific phase in Britain and Europe, filled with many examples of innovation, such as in astronomy and chemistry. Franklin has a famous saying, “Asking what this thing is for is like asking what a newborn is for,” which was his response to a friend who asked what the use of balloons was. The book concludes with a group of poets—including Shelley—whose feelings about technological progress were filled with both excitement and fear, similar to our current feelings about AI; in a sense, history indeed repeats itself.
That’s all I have to say, thank you.。
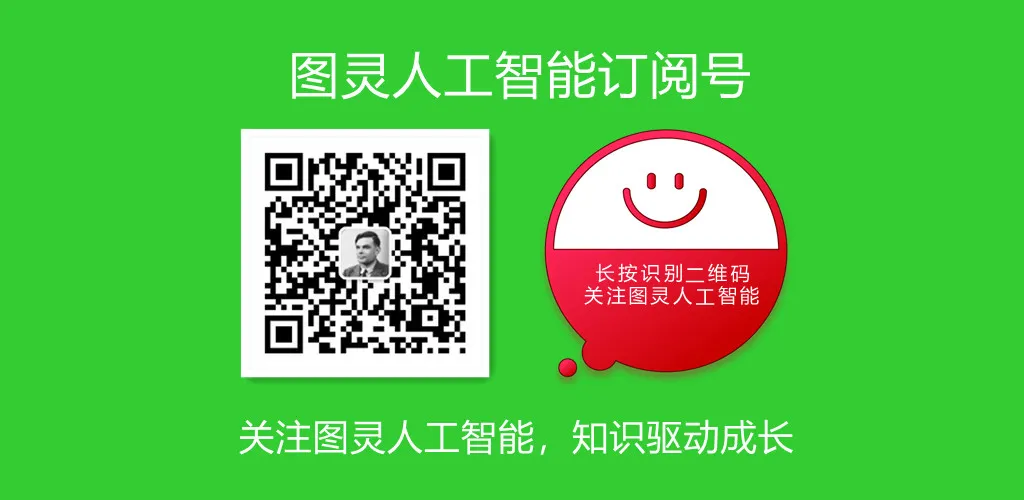

Featured Articles: