Qianming from Aofeisi Quantum Bit Report | WeChat Official Account QbitAI
The golf course, a long-standing high-end social venue, has a hidden cost: the invasion of resources and the environment.
It not only occupies a large amount of land and consumes water resources but also uses a lot of chemical fertilizers and pesticides in maintaining the lawn, leading to serious pollution.
How serious is it?
Xu Ming, former deputy governor of Jiangsu Province, gave a comparison in an interview with “China Economic Weekly”:
“The pollution from a golf course is even more severe than that from an ordinary factory.”
Since 2004, relevant departments have begun to introduce a series of policies to restrict the construction of golf courses and launched special cleanup operations around 2017.
But how can the effectiveness of the cleanup be verified?
Golf courses are relatively dispersed and cover a large area, so detecting them through remote sensing images is a better solution. The popularity of high-resolution optical remote sensing imagery provides strong data support for golf course detection.
Even with this data, detection is not easy.
Below is a remote sensing image; ignoring the green box, can you spot how many golf courses are in it and where they are?
A skilled interpreter would take about 15 minutes to detect all the golf courses from such a remote sensing image.
But now, deep learning technology has changed the face of this work.
In just 10 seconds, it can automatically detect golf courses in such images.
In contrast, efficiency has improved by 90 times, with an accuracy rate of 84%.
This is not an isolated case but a collective improvement in the entire application direction, which is indeed happening at the Institute of Remote Sensing and Digital Earth, Chinese Academy of Sciences.
How did this leap occur? What kind of process is it?
AI has made significant achievements in image recognition for many years; why has its capability only come to light now?
To answer these questions, we need to first address —
Why Was Processing Remote Sensing Images So Slow?
Using remote sensing images to monitor the surface is a continuous process.
Researchers at the Institute of Remote Sensing and Digital Earth, Chinese Academy of Sciences, say that the biggest difficulty lies in the fact that the environment and climate of the same place change every year.
This greatly impacts the algorithms used to understand remote sensing images.
The most direct manifestation is that the algorithms originally built for these places need targeted adjustments after a year to adapt to these changes; otherwise, they will “break down.”
Moreover, these algorithms are strongly related to human experience; if the person who designed the algorithm leaves, the entire algorithm becomes difficult to sustain.
It is important to note that these algorithms are not automated; they still require manual cooperation.
In China, covering 9.6 million square kilometers, completing a full pass would require at least a thousand people working together for 2 to 3 months.
What to do? Deep learning can be used. Now, the remote sensing institute does the following:
Build a sample library for a specific area, then train a deep learning model based on the images in the sample library.
The following year, if the environment and climate of that area change, you only need to add new images to the sample library and retrain the model.
This also reduces dependence on humans; the adjustment of the model is no longer limited by expert experience but relies on data changes.
Moreover, as data increases, it is no longer a burden but “nourishment” for improving model accuracy.
Although it seems that everything is efficient and straightforward now, the transition from the traditional manual + algorithm model to the current deep learning model has encountered many difficulties.
What Are the Challenges of Using AI to Understand Remote Sensing Images?
Image recognition can be said to be a relatively mature technology in the current AI field, with various deep learning models for image understanding emerging one after another, and reaching human-level performance in specific domains.
However, the problem lies in the fact that these deep models are mainly designed for natural images; directly applying them to understand remote sensing images leads to significant performance degradation.
This is because there are substantial differences between the two types of images.
First, remote sensing images have many more spectral bands; in addition to the three bands (RGB) of natural images, remote sensing images must have at least one near-infrared band. Some satellite-acquired remote sensing images have 8 bands, while hyperspectral images can have as many as 200 bands.
Second, the scale differences in images are also very large; compared to the multi-scale recognition using scale pyramids in natural images, the scale differences in remote sensing images may need to exceed 1:30 to effectively identify various target features.
Third, there is the issue of local spatial feature distortion. The distortion of natural images is mainly due to sensor edge distortion and lens distortion, which is controllable overall. However, the distortion in remote sensing images arises from errors in image acquisition, which is relatively uncontrollable.
The existence of these problems makes it difficult to directly apply existing deep learning algorithms to remote sensing image understanding tasks. Not only do the models need further optimization, but the framework also needs to provide support:
It must offer multi-band support in remote sensing image reading and add image enhancement algorithms specifically for remote sensing images, considering aspects like multi-band color enhancement and local spatial feature deformation enhancement.
These are precisely the efforts made by Baidu in its deep learning framework PaddlePaddle. With the help of this framework, the Institute of Remote Sensing and Digital Earth, Chinese Academy of Sciences, is also completing a new round of technological iteration.
Applications Are Becoming More Widespread
Specifically, regarding the golf course recognition issue mentioned at the beginning, researchers at the Institute of Remote Sensing have used the support of the PaddlePaddle framework to apply the Faster R-CNN object detection model.
In a professional and standard remote sensing dataset for golf courses, it only takes 10 seconds to detect all the courses in the remote sensing image.
Using manual + algorithm recognition would take 15 minutes.
The new deep learning method has improved work efficiency by 90 times, with a detection accuracy of 84%.
Moreover, deep learning is not only used for the automated detection of golf courses but is also being applied to understand airports in remote sensing images and wind and solar power stations built in mountainous areas.
With deep learning technology, researchers can quickly identify how many solar panels are in a region based on remote sensing images, potentially providing a clear estimate of how much electricity can be generated in that area and offering decision support for grid construction, avoiding the dilemma of “having electricity but no grid” or “having a grid but no electricity.”
According to data from the National Energy Administration, in 2018 alone, photovoltaic power generation wasted 5.49 billion kilowatt-hours, equivalent to the annual electricity consumption of over 2 million households (calculated based on an average household monthly consumption of 200 kWh).
The social value behind this is evident.
Furthermore, understanding remote sensing images is just one example of how PaddlePaddle solves practical problems.
In the field of computer vision, this framework can already support models in completing tasks such as image classification, object detection, image semantic segmentation, scene text recognition, image generation, human keypoint detection, video classification, and metric learning.
Finally, here is a usage guide. If you’re interested, feel free to bookmark it and watch it later~
An Overview of Eight Major Tasks in Computer Vision: PaddlePaddle Engineers Explain Popular Visual Models
— The End —
Subscribe to AI Insights for AI Industry News
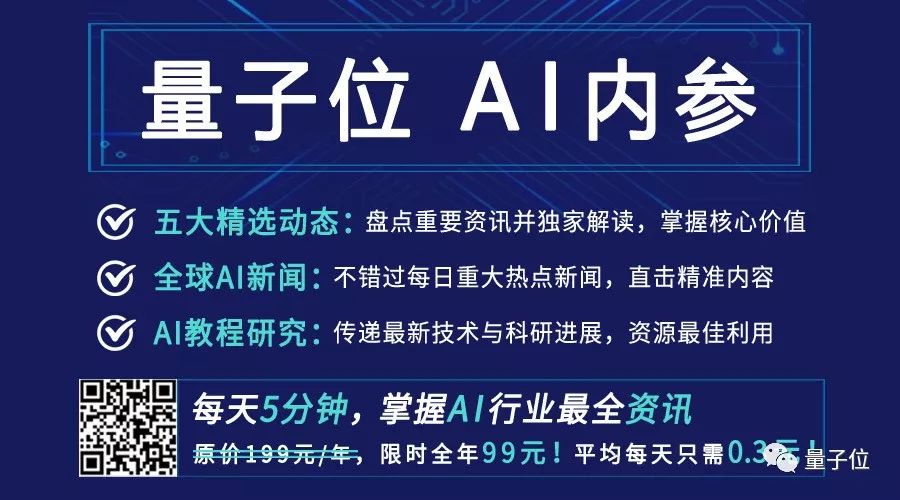
Join the Community
The Quantum Bit AI community is now recruiting! The community includes: AI discussion groups, AI + industry groups, and AI technology groups;
Students interested in AI are welcome to reply with the keyword “WeChat group” in the Quantum Bit WeChat official account (QbitAI) dialogue interface to get the joining method. (Technical groups and AI + industry groups require approval, and the review is strict; please understand.)
Hiring
Quantum Bit is recruiting editors/reporters, with the work location in Zhongguancun, Beijing. We look forward to talented and passionate students joining us! For details, please reply with the word “Recruitment” in the Quantum Bit WeChat official account (QbitAI) dialogue interface.
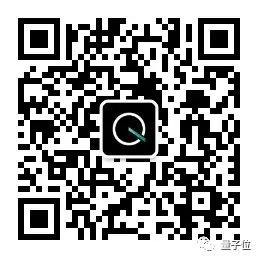
Quantum Bit QbitAI · Signed Author on Toutiao
Tracking new trends in AI technology and products
If you like it, please click “Like”!