Introduction
Optical Character Recognition (OCR) is a branch of computer vision research, belonging to pattern recognition and artificial intelligence, and is an important component of computer science.
This article will briefly describe the various components in the field of OCR, using the above image as the main clue.
1. Introduction to Optical Character Recognition
Computer Optical Character Recognition, commonly known as OCR, utilizes optical and computer technologies to read characters printed or written on paper and convert them into a format that can be recognized by computers and understood by humans. OCR technology is a key technology for high-speed text input.
In OCR technology, printed text recognition is the earliest and most mature technology. As early as 1929, German scientist Taushek obtained a patent for Optical Character Recognition (OCR). In order to input the vast and ever-increasing amounts of newspapers, magazines, documents, and reports into computers for information processing, Western countries began research on Western OCR technology in the 1950s to replace manual keyboard input. After more than 40 years of continuous development and improvement, and with the rapid advancement of computer technology, Western OCR technology has now been widely applied in various fields, allowing large amounts of text data to be input into computers quickly, conveniently, time-saving, and timely, achieving “electronic” information processing.
Compared to printed Western OCR, the research on printed Chinese OCR technology developed based on printed digital recognition and printed English recognition, dating back to the 1960s. In 1966, Casey and Nagy from BIM published the first paper on printed Chinese character recognition, in which they identified 1,000 printed Chinese characters using a simple template matching method. Since the 1970s, Japanese scholars have conducted extensive research on Chinese OCR, with representative systems such as the one developed by Toshiba’s Comprehensive Research Institute in 1977, which could recognize 2,000 individual printed Chinese characters; and the printed Chinese character recognition system developed by Musashino Electric Research Institute in the early 1980s, which could recognize 2,300 multi-body characters, representing the highest level of Chinese character recognition at that time. In addition, companies like Sanyo, Panasonic, Ricoh, and Fuji also developed their own printed Chinese character recognition systems. Most of these systems used K-L digital transformation-based matching schemes and employed a large amount of specialized hardware, with some devices equivalent to minicomputers or even mainframes, making them extremely expensive, so they were not widely used.
In comparison, China’s research on printed Chinese character recognition began in the late 1970s and has developed for nearly thirty years, roughly divided into the following three stages:
1) Exploration Stage (1979-1985)
Based on the research of digital, English, and symbol recognition, a few research units in the late 1970s began exploring Chinese character recognition methods, publishing some papers and developing a small amount of simulation recognition software and systems. This stage was long, and the results were few, but it nurtured the fruitful results of the next stage.
2) Development Stage (1986-1988)
From early 1986 to the end of 1988, these three years marked the peak of Chinese character recognition technology research and the harvest period for printed Chinese character recognition technology. A total of 11 units conducted 14 evaluations of printed Chinese character recognition results, achieving high standards for sample recognition: they could recognize Song, Fang Song, Hei Ti, and Kai Ti fonts, with up to 6,763 characters recognized, font sizes from 3 to 5, and recognition rates exceeding 99.5%. However, the recognition rate for real text decreased significantly due to the systems’ poor adaptability and interference resistance to variations in printed text shapes (e.g., blurred text, stroke adhesion, broken strokes, uneven black and white, poor paper quality, ink bleeding, etc.). However, the recognition systems developed during these three years laid the foundation for the practical application of printed Chinese character recognition systems, marking an essential process from development to practical application.
3) Practical Stage (1989-present)
Since the surge in printed Chinese character recognition began in 1986, several units, including the Department of Electronic Engineering at Tsinghua University, the Intelligent Center of the Institute of Computing Technology at the Chinese Academy of Sciences, Beijing Information Engineering University, and Shenyang Automation Research Institute, have developed practical printed Chinese character recognition systems. Notably, the TH-OCR product developed by Tsinghua University’s Department of Electronic Engineering and the Shuxin OCR product developed by Hanwang Group have always been at the forefront of technology development, occupying the largest market share and representing the trend of printed Chinese character recognition technology. Currently, the research focus of printed Chinese character recognition technology has shifted from simple character recognition to automatic recognition and input of tables, layout analysis, layout understanding and restoration of mixed text and graphics, business card recognition, financial invoice recognition, and ancient book recognition. Many related recognition systems have emerged, such as the e-card business card recognition system launched by Ziguang Wontong, the e-Verify ID card recognition system, and the “Smart View” screen text image recognition system, etc. The emergence of these new recognition systems signifies a broad expansion of the application field of printed Chinese character recognition technology.
The National High Technology Research and Development Program (863 Program), the National Key Technology R&D Program, the National Natural Science Foundation, and the Military Basic Research Fund have all placed great importance and strong support on the research topic of printed Chinese character recognition. Currently, printed Chinese character recognition and online handwritten Chinese character recognition are moving towards practical application, with their technical levels on par with the highest levels in the world.
This article will categorize and describe OCR technology and introduce some commonly used algorithms in the OCR field. Since my personal project practice is more related to printed recognition, please correct me if there are any inaccuracies in the descriptions of other fields. I hope this introduction will give everyone a brief concept of the entire OCR process.
2. Printed Character Recognition
The rise of OCR technology began with printed character recognition, and the success of printed character recognition laid a solid foundation for the later development of handwritten character recognition. The main processes of printed character recognition can be roughly divided into the following parts: image preprocessing; layout processing; image segmentation; feature extraction, matching, and model training; recognition post-processing.
2.1 Image Preprocessing
Once the input text is scanned into the computer, due to the thickness, smoothness, and printing quality of the paper, character distortion can occur, leading to broken strokes, adhesion, and stains. Therefore, before performing character recognition, it is necessary to process the noisy text images. Since this processing occurs before character recognition, it is referred to as preprocessing. Preprocessing generally includes grayscaling, binarization, tilt detection and correction, line and character segmentation, smoothing, normalization, etc.
2.1.1 Grayscaling
The images captured by peripherals are usually color images, which may contain some interference information; the main purpose of grayscaling is to filter out this information. The essence of grayscaling is to map the originally three-dimensional described pixel points to one-dimensional described pixel points. There are many ways and rules for conversion, which will not be detailed here.
2.1.2 Binarization
After grayscaling, the color image needs to undergo binarization to further separate text from the background. Binarization converts the grayscale values (or color values) of image signals into a binary image signal containing only black (1) and white (0). The quality of the binarization effect directly affects the recognition rate of grayscale text images. Binarization methods can be roughly divided into local threshold binarization and global threshold binarization. Currently, a commonly used method is Otsu’s method proposed by Japanese scholars.
2.1.3 Tilt Correction
Printed text materials are mostly composed of horizontal (or vertical) text lines parallel to the page edges, with a tilt angle of zero degrees. However, during the scanning process, whether manual or machine scanning, image tilt phenomena are unavoidable. Tilted document images can greatly affect subsequent character segmentation, recognition, and image compression tasks. To ensure the correctness of subsequent processing, it is necessary to perform tilt detection and correction on the text image.
Text image tilt correction can be divided into manual correction and automatic correction. Manual correction refers to the recognition system providing some human-computer interaction means to achieve text image tilt correction. Automatic correction refers to the computer automatically analyzing the layout features of the text image, estimating the tilt angle, and correcting the text image based on the tilt angle.
Currently, there are many methods for text image tilt detection, which can be mainly divided into the following five categories: projection-based methods, Hough transform-based methods, cross-correlation methods, Fourier transform-based methods, and nearest neighbor clustering methods.
The simplest projection-based method involves projecting the text image in different directions. When the projection direction is consistent with the text line direction, the peak value of the text line on the projection graph is the largest, and there are obvious peaks and valleys on the projection graph, making the projection direction the tilt angle.
The Hough transform is also a commonly used tilt detection method, utilizing the properties of Hough transform to map the foreground pixels of the image to polar coordinate space and statistically accumulating the values of each point in polar coordinate space to obtain the tilt angle of the document image.
The Fourier transform method utilizes the property that the page tilt angle corresponds to the direction angle that maximizes the density in Fourier space, performing a Fourier transform on all pixels of the document image. This method has a very high computational cost and is rarely used.
Based on the nearest neighbor clustering method, the center point of the connected domain of characters in a sub-region of the text image is taken as the feature point, and using the continuity of points on the baseline, the direction angle of the corresponding text line is calculated to obtain the tilt angle of the entire page.
2.1.4 Normalization
Normalization involves processing input text of any size into a uniform standard size to match the reference templates pre-stored in the dictionary. Normalization operations include position normalization, size normalization, and stroke thickness normalization. This section will only discuss position normalization and size normalization.
To eliminate deviations in the pixel position of the text, the entire text pixel image must be moved to a specified position, a process known as position normalization. There are two common position normalization operations: one is based on the centroid position normalization, and the other is based on the outer boundary of the text. The position normalization method based on the outer boundary of the text requires first calculating the outer boundary of the text, finding the center, and then moving the center of the text to the specified position. The centroid-based position normalization method has stronger interference resistance than the outer boundary-based method.
Using the outer boundary-based position normalization method for text position normalization results in the following image.
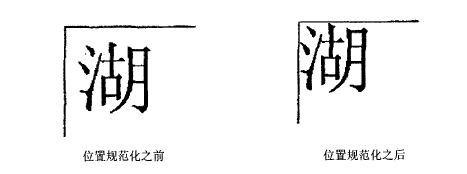
Transforming text of different sizes to a uniform size is called size normalization. Many existing multi-font printed character recognition systems use size normalization to recognize different font sizes. Common size normalization operations also include two types: one is to proportionally enlarge or shrink the outer boundary of the text to a specified size, and the other is to normalize the size based on the distribution of black pixels in both horizontal and vertical directions.
Using the method based on the distribution of black pixels in horizontal and vertical directions for text size normalization results in the following image.
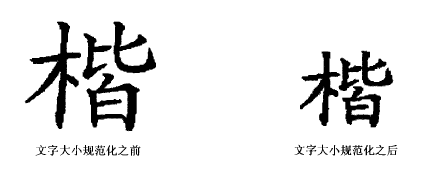
2.1.5 Image Smoothing
After smoothing processing, the text image can eliminate isolated white points on strokes and isolated black points outside strokes, as well as uneven points on stroke edges, making the stroke edges smoother. A simple smoothing method is as follows. Using an NxN window (N is generally 3, as shown in the figure), scan through the binary text pixel array and, based on the distribution of black and white pixels in the window, change the pixel at the center of the window from “0” to “1” or from “1” to “0”.
This method smooths the text outline edges according to the following rules.
Rule 1: If any of the situations (a), (b), (c), or (d) in the figure are met, the center point should change from “0” to “1”.
Rule 2: If any of the situations (e), (f), (g), or (h) in the figure are met, the center point should change from “1” to “0”.
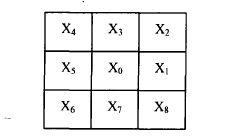
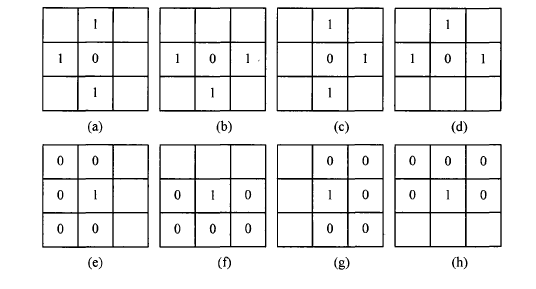
2.2 Layout Processing
Layout processing is generally divided into three main parts: layout analysis, layout understanding, and layout reconstruction.
2.2.1 Layout Analysis
The text image is segmented into different parts, and the attributes of each part are labeled, such as: text, images, tables. Currently, the core idea of the work in layout analysis is based on connected domain analysis, and the neural network-based layout analysis methods derived from it are also based on connected domains.
2.2.2 Layout Understanding
Obtaining the logical structure of the article, including the logical attributes of each area, the hierarchical relationship of the article, and the reading order. Based on the location information of the connected domains recorded during layout analysis, determine the belonging sequence of the connected domains.
2.2.3 Layout Reconstruction
Based on the results of layout analysis and OCR, reconstruct an electronic document containing both text information and layout information.
2.3 Image Segmentation
Image segmentation can be roughly divided into two main categories: line (column) segmentation and character segmentation. After segmentation processing, it becomes convenient to recognize individual characters. As shown in the figure.
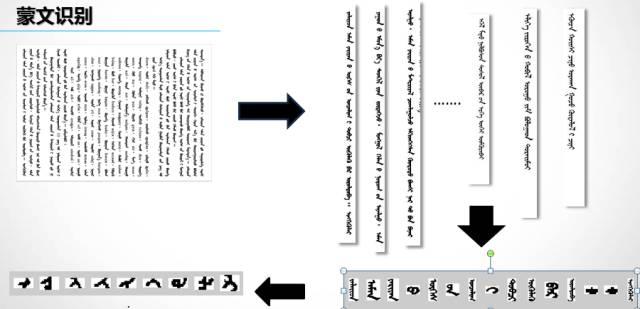
2.3.1 Line and Column Segmentation
Due to the equal spacing between printed text lines and characters, and the near absence of adhesion phenomena, projection methods can be used for image segmentation. The pixel value projection curve of each column (row) on the coordinate axis is an uneven curve, and the regions between each valley position of the smoothed curve represent one row (column).
2.3.2 Character Segmentation
Character segmentation varies significantly between different languages. Generally, character segmentation refers to dividing an entire line or column of text into individual characters. However, depending on language differences, it may also be necessary to further segment individual characters. For example, the difficulty of segmenting the Chinese character “屋” is very different from that of segmenting the English word “house”, so the recognition methods will also be designed differently based on language characteristics.
2.4 Feature Extraction and Model Training
Before deep learning was widely applied in image recognition, template matching was a common recognition method. Later, with the resurgence of neural networks, feedback-based neural networks brought a new spring to the OCR field. Now, with the enhancement of computing power in computer hardware, deep neural networks trained with large amounts of data have achieved impressive results in image recognition.
2.4.1 Feature Extraction and Matching
Feature extraction is the process of extracting statistical or structural features from individual character images. The stability and effectiveness of the extracted features determine the performance of recognition. For statistical feature extraction, methods from statistical pattern recognition can be utilized, while structural feature extraction should be determined according to the specific recognition primitives of the text. In a long research process of character recognition, experience knowledge has been used to guide the extraction of text features, such as edge features, transformation features, penetration features, grid features, feature point features, and directional line features.
Feature matching is the process of finding the most similar text from an existing feature library to the text to be recognized. After the features of the text to be recognized are extracted, whether statistical features or structural features are used, a feature library must be available for comparison, which should contain the features of all characters in the desired recognition character set. There are many methods of feature matching, with commonly used ones including Euclidean space comparison, relaxed matching, dynamic programming matching, and HMM (Hidden Markov Model) methods. Before and for a long time after the emergence of neural networks, template matching methods were used in the field of Chinese OCR.
2.4.2 Model Training
After a period of development, artificial neural networks mainly serve as classifiers in OCR. The network input is the character feature vector, and the output is the class encoding. In cases where the recognition types are fewer and the structural distinctions are more obvious, the feature vector is typically a matrix of character image pixel points. Thus, feature extraction is essentially a black box operation, with many explanations for its principles, which will not be detailed here. Deep learning has been successfully applied in the OCR field, replacing the cumbersome feature engineering process, automatically learning image features from a large amount of labeled data, with CNN (Convolutional Neural Network) being particularly prominent. In addition to eliminating the need for manual feature extraction, the shared weight approach also reduces the number of weights, significantly decreasing computational costs, making CNN perform exceptionally well in the OCR field.
2.4.3 Recognition Methods
With the increase in user numbers and demands, recognition efficiency has also become an important indicator of OCR engineering. Traditional methods have high server performance requirements, leading to high investment costs and difficulties in scalability. After the emergence of Hadoop, this issue has improved significantly, and through the MapReduce programming framework, the hardware costs that enterprises need to invest have been greatly reduced, providing a strong impetus for the development of OCR.
2.5 Post-Recognition Processing
Post-recognition processing is mainly applied in two aspects: layout restoration and recognition correction. Layout restoration has been discussed in the layout processing section, so it will not be repeated here. Recognition correction is primarily based on the recognition results and is conducted according to the language model of the language involved. Of course, this is relatively easier in single-language recognition, while it is more complex in multi-language cases.
3. Handwritten Character Recognition
Handwritten character recognition is more difficult than printed character recognition, and offline handwritten character recognition is even more challenging than online handwritten character recognition. This is also the main reason why offline handwritten character recognition has not yet been successfully applied.
Online handwritten character recognition, also known as real-time (or online) handwritten recognition, refers to the process where a machine recognizes characters based on the strokes and stroke order while a person is writing. Online handwritten character recognition relies on electromagnetic or piezoelectric handwriting input boards. When a user writes on the input board with a stylus, the movement trajectory of the stylus on the board (the coordinates on the board) is converted into a series of electrical signals, which can be input into the computer serially. From these electrical signals, it is relatively easy to extract stroke and stroke order information for character recognition. Since the 1990s, online handwritten character recognition has gradually moved toward practical use. Chinese researchers have introduced several online handwritten Chinese character recognition systems, and some large companies abroad have also begun to enter this market. This technology also aligns with the development trend of PDAs (Personal Digital Assistants).
Offline handwritten character recognition, also known as offline handwritten character recognition, refers to the process where the writer pre-writes characters on paper, which are then converted into character images through a scanner and recognized by a computer. Due to the different writing habits of individuals, the types of offline handwritten characters vary widely. Moreover, even the same person may produce different handwriting under different circumstances, making offline handwritten character recognition extremely challenging. For offline handwritten character recognition, if there are no restrictions on the writing (i.e., freehand writing), the recognition difficulty is quite high.
The processing flow and technical methods for offline handwritten character recognition are roughly similar to those for printed character recognition. However, due to the greater variability of handwritten characters, the segmentation of text images into lines and characters poses significant challenges. Depending on the language, segmentation methods may differ. For instance, in handwritten Mongolian characters, researchers at Inner Mongolia University have employed a character segmentation algorithm based on the external polygon of characters to achieve good segmentation results.
The processing flow for online handwritten character recognition mainly utilizes stroke order as a sequence model, combined with character structure features, stroke direction features, and stroke count features as spatial models to complete recognition tasks.
4. Application Cases
4.1 Printed Character Recognition Applications
Printed character recognition has a wide range of applications, and its technology has developed maturely, achieving high standards in both recognition accuracy and efficiency.
4.1.1 License Plate Recognition
License plate recognition systems are typical cases of the industrial application of OCR, having been successfully implemented early on. Nowadays, from parking lots to residential community access control, license plate recognition technology has entered various aspects of life. The success of license plate recognition can be attributed to several reasons:
1. The recognition content is a closed set with a relatively small size.
2. The text font and size are relatively standardized.
3. The spacing between characters is uniform, with minimal noise.
4.1.2 Tesseract
The Tesseract OCR engine was first developed by HP Labs in 1985 and became one of the top three OCR engines in terms of accuracy by 1995. However, HP soon decided to abandon the OCR business, and Tesseract was subsequently shelved.
Years later, HP realized that instead of letting Tesseract gather dust, it would be better to contribute it to the open-source community to revitalize it. In 2005, Tesseract was acquired by the Nevada Institute for Information Technology and underwent improvements, bug fixes, and optimizations thanks to Google.
Tesseract is now available as an open-source project on Google Project, with its latest version 3.0 supporting Chinese OCR and providing a command-line tool.
4.1.3 CAPTCHA Recognition
The main purpose of CAPTCHAs is to enforce human-computer interaction to defend against automated attacks. As an auxiliary security measure, CAPTCHAs hold a special position in web security. While the security of CAPTCHAs may seem trivial compared to numerous vulnerabilities in web applications, it is important to note that a small issue can lead to significant problems. Sometimes, bypassing CAPTCHAs can convert manual processes into automated ones, greatly aiding web security testing.
The completely automated Turing test to tell computers and humans apart (CAPTCHA) is a public program designed to distinguish between computer users and human users. In a CAPTCHA test, the server automatically generates a question for the user to answer. This question can be generated and evaluated by the computer, but only humans can answer it. Since computers cannot solve CAPTCHA questions, users who can answer the questions are considered human.
Text recognition technology is only applicable to character-based CAPTCHA recognition, and in addition, it requires the design of corresponding denoising algorithms for different CAPTCHAs.
4.2 Handwritten Character Recognition Applications
4.2.1 Wontong Pen (Online Handwriting)
Stroke order and connected strokes are the two main factors affecting the recognition rate of online handwritten Chinese character recognition systems. As mentioned earlier, there are two methods to address the stroke order issue: one is to impose strict requirements on users to write according to a “standard” stroke order, one stroke at a time. This requirement is practically very difficult to achieve, as there is currently no unified standard or regulation for stroke order in China. Even if a standard exists, individuals’ education levels and habits vary greatly, making it nearly impossible to expect everyone to write according to the standard stroke order. The other method is to set several standard templates for each Chinese character that may have different stroke orders. For example, the character “女” may be written with the “く” stroke first by some people and with the “一” stroke first by others, resulting in two different stroke orders for the same character. In the dictionary, two templates can be set, which have the same strokes but different stroke orders, both representing the character “女”. This approach significantly increases the dictionary’s capacity. In earlier times, when integrated circuit scales were not large enough and speeds were not high enough, this method was difficult to promote.
Connected strokes present an even more challenging issue and are also difficult to solve. People often write in a continuous manner to improve writing speed. It is almost impossible to require them to write each stroke separately and in order. This problem is the main reason why the recognition rate of commercially available stylus input devices has not improved significantly.
In recent years, the Wontong Pen has made some efforts to address the aforementioned stroke order and connected strokes issues, achieving some results and receiving favorable evaluations in national assessments. The reasons for these advancements are, on one hand, due to the rapid development of computer technology, where machine resources are no longer the main constraint on recognition algorithms, allowing designers to boldly create algorithms with high interference resistance; on the other hand, regarding recognition algorithms, the developers of Wontong Pen have proposed a new technology that creatively combines structural methods with statistical methods, effectively addressing the difficulties faced by purely structural recognition methods in adapting to the variations in handwritten Chinese characters and stroke orders. The key points of this algorithm are briefly introduced below.
As mentioned earlier, structural pattern recognition adequately describes the structural information of the recognized objects. However, this method has its shortcomings:
1. The description of the pattern is based on symbols rather than numerical values, leading to poor interference resistance.
2. The grammatical rules for describing primitive relationships generally need to be manually written, making it difficult to obtain through machine learning.
3. Syntactic analysis is complex, with a large computational load.
In response to these issues, Wontong Pen has made some innovative work in modeling handwritten Chinese characters, including:
1. Establishing a pattern statistical model based on a relatively strict probability foundation, which can be used to describe the spatial information of online handwritten characters and can easily be trained using statistical methods to establish a two-dimensional model of handwritten Chinese characters.
2. Improving the Hidden Markov Model (HMM) by introducing the concept of controlling state transition paths, making the model more suitable for describing online handwritten Chinese characters, and better reflecting the temporal information of handwritten Chinese characters. This also includes a path search algorithm and parameter training method for this model.
3. Combining the above two models for online handwritten Chinese character recognition, significantly addressing the impact of connected strokes and stroke order variations on the performance of recognition systems, resulting in high robustness.
The Wontong Pen, developed using the above methods, ranked high in recent assessments organized by the expert group of the “863 Program” intelligent machine theme. The system can recognize 6,763 simplified Chinese characters and 5,401 traditional Chinese characters, achieving a recognition rate of 95%-99% for neatly written characters, and 87%-93% for more hastily written characters, with an accumulated recognition rate of 98%-99% for the top ten characters. The recognition speed on mainstream microcomputers exceeds 3 characters per second, meeting practical application requirements.
4.2.2 Ancient Text Recognition (Offline Handwritten Character Recognition)
Currently, the main application direction of offline handwritten character recognition is ancient text recognition. Before the invention of printing, the primary method for ancient people to transmit books was through copying. After the invention of printing, the number of books copied by hand decreased, but there are still many letters and memorials containing large amounts of handwritten text. Using human effort to complete the electronic transfer of these letters is very labor-intensive, leading to a growing research demand for recognizing text in ancient books.
However, due to the inevitable damage to ancient books during preservation, resulting in unclear or damaged handwriting, combined with the inherent variability of handwritten characters, the work of ancient text recognition is extremely challenging. Therefore, there have not yet been any successful cases for offline handwritten character recognition applications.
5. Learning Resources
5.1 Recommended Blogs
5.1.1 Chinese OCR Blog
The blogger’s series of Chinese OCR blogs provide straightforward explanations, making it easy to understand the entire process of Chinese character recognition.
http://blog.csdn.net/plfl520/article/details/8441478
5.1.2 Mathematical Understanding of Convolutional Neural Networks
Another recommended series of blogs, where the blogger delves deeply into neural network algorithms from a mathematical perspective, suitable for those wanting to understand the principles of algorithms in detail.
http://colah.github.io/posts/2014-07-Understanding-Convolutions/
5.2 Recommended Books
5.2.1 “Statistical Learning Methods” — Li Hang
This book can be considered a classic in the field of machine learning, detailing some fundamental machine learning algorithms with thorough mathematical calculations, making it an excellent entry-level book for machine learning.
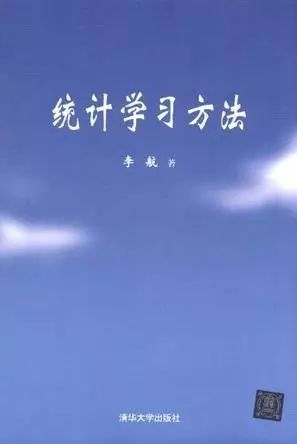
5.2.2 “The Beauty of Mathematics” — Wu Jun
The “Beauty of Mathematics” series articles were originally published in Google’s blog, receiving millions of clicks and high praise from readers. Dr. Wu Jun explains complex mathematical principles in a more accessible way, allowing non-professional readers to appreciate the charm of mathematics. Readers learn how to simplify complex problems, use mathematics to solve engineering problems, and think innovatively beyond conventional thinking through concrete examples. This mindset is not only crucial in the field of machine learning but is also beneficial in other fields.
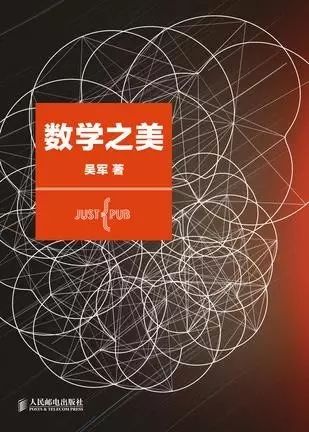
5.2.3 “Digital Image Processing” — Gonzalez
To understand the theory of image processing, one can read Gonzalez’s “Digital Image Processing” thoroughly. This book primarily discusses basic principles, and it is recommended to read the original version, as translations may lead to misunderstandings.
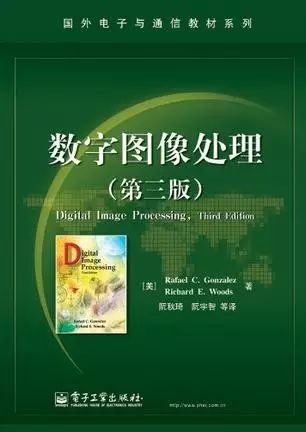
5.3 Video Resources
https://www.coursera.org/learn/machine-learning/home/welcome
Andrew Ng’s machine learning course, combined with small exercises, yields great benefits.
5.4 Reference Papers
URL: http://pan.baidu.com/s/1bpH2dtX Code: xjwl
5.5 OCR Tools
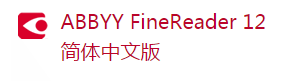
1. Extremely high recognition rate.
2. High degree of freedom.
3. Convenient for batch operations.
4. Can maintain the original table format, saving the need for secondary editing.
5. Includes many image correction methods, such as trapezoidal and slant corrections.
5.6 References
[1] Wei Hongxi. Research on Key Technologies in Printed Mongolian Character Recognition [D]. Master’s Thesis, Inner Mongolia University, 2006.
[2] Liu Yuxing. Research and Implementation of Chinese Character Recognition Based on MapReduce [D]. Master’s Thesis, South China University of Technology, 2011.
[3] Dan Ciresan, Ueli Meier. Multi-Column Deep Neural Networks for Offline Handwritten Chinese Character Classification [C]. In: Proceedings of the International Conference on Neural Networks (IJCNN), 2015, pp. 1-6.
[4] Chunpeng Wu, Wei Fan, Yuan He, Jun Sun, Satoshi Naoi. Handwritten Character Recognition by Alternately Trained Relaxation Convolutional Neural Network [C]. In: Proceedings of the International Conference on Frontiers in Handwriting Recognition (ICFHR), 2014, pp.291-296.
Author:
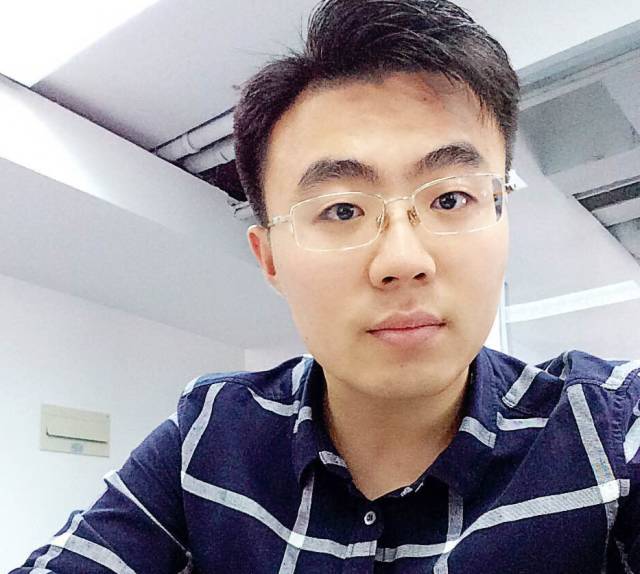
Yao Zhipeng, a volunteer in the Data Research Department, graduated with a master’s degree in software engineering from Inner Mongolia University. During his studies, his research focus was on intelligent information processing of Mongolian text. He is currently engaged in NLP Chinese named entity recognition algorithm-related work, has a strong interest in deep learning algorithms and AI products, and hopes to make contributions in the field of natural language understanding.
Editor: Hu Die
Past Reviews of the “Understanding” Series:
[Exclusive] Understanding Non-Relational Databases (NoSQL)
[Exclusive] Understanding Regression Analysis
Reprint Notice
If you need to reprint, please prominently mention the author and source at the beginning (reprinted from: Data Research ID: DatapiTHU), and place a prominent QR code for Data Research at the end of the article. For articles with original identification, please send the [Article Title – Pending Authorized Public Account Name and ID] to the contact email to apply for whitelist authorization and edit according to requirements.
After publishing, please provide feedback on the link to the contact email (see below). Unauthorized reprints and adaptations will be legally pursued.
“Education Research on Data: Tsinghua University Education Big Data Forum” is now open for registration, scan the QR code below to register.

There are surprises in the bottom menu of the public account!
For enterprises and individuals joining the organization, please check the “Union”
For past exciting content, please check “Search within the account”
For joining the team or contacting us, please check “About Us”
Welcome to register as aData Research Department Volunteer, algorithm model group, natural language processing group, system platform group, research and analysis group…. There is always one that suits you~
To register, please click “Read the original text”.