Click on "Xiaobai Learning Vision" above, select to add "star" or "top"
Heavy content delivered in real time
Introduction
Text is one of the most important sources of information for humans, and natural scenes are filled with various character symbols. OCR (Optical Character Recognition) is a familiar term, referring to the process where electronic devices (such as scanners or digital cameras) check printed characters on paper, determine their shapes by detecting dark and light patterns, and then translate these shapes into computer text using character recognition methods.
Image text recognition in industrial scenarios is more complex and appears in many different contexts. For example, text on pharmaceutical packaging, various steel components, sprayed text on container surfaces, and personalized text on store signs. In such images, the characters may appear in various forms such as curved arrays, irregular surfaces, sloped distributions, wrinkled deformations, and incompleteness, which significantly differ from the characteristics of standard characters, making it difficult to detect and recognize image characters.
For text recognition, it generally requires first detecting and locating the text area in the image, then extracting the sequential features of the area, and based on this, performing specialized character recognition. However, with the development of CV, many end-to-end OCR methods have emerged.
Applications of OCR:
Text recognition OCR is commonly applied in document identification (ID cards, driver’s licenses, passports, business cards), document retrieval, screenshot recognition, etc.
What does OCR do to images:
In fact, the expected result is to have the computer translate images that contain only a single character.
How does a machine see the text on paper or electronic documents or images? Let’s take a look at its workflow.
Workflow:
First, impurities must be removed so the program can focus on the text.
Preprocessing:
Preprocessing mainly includes grayscaling, binarization, noise removal, and skew correction.
Grayscaling:
A grayscale image contains only brightness information and no color information.
In the RGB model, if R=G=B, it represents a grayscale color, where the value of R=G=B is called the grayscale value.
Generally, it satisfies the following formula:
Gray=0.299R+0.587G+0.114B. This parameter takes into account the physiological characteristics of the human eye.
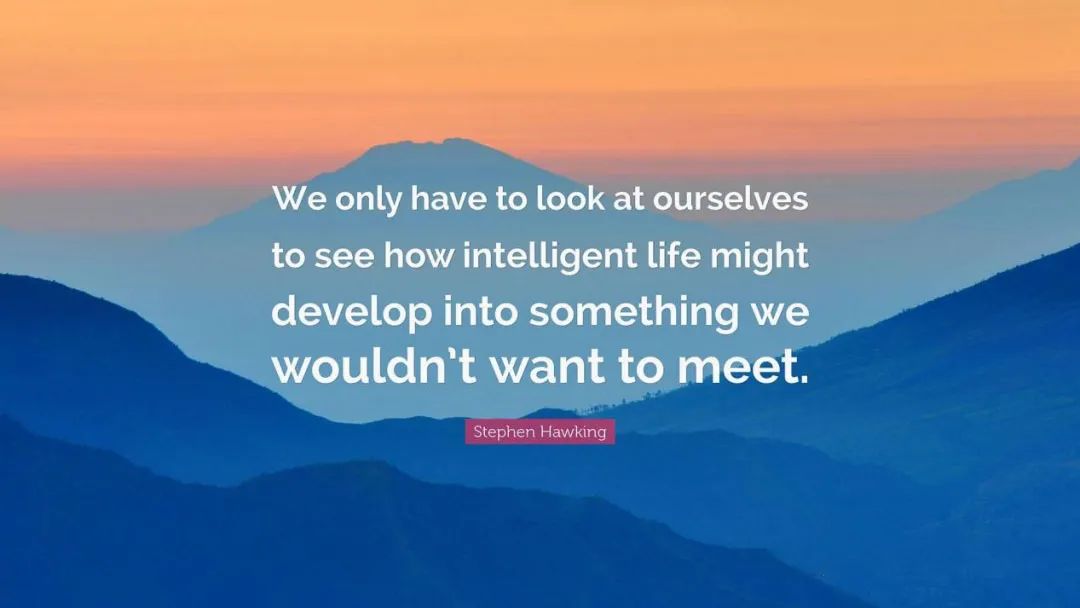
Original Image.jpg
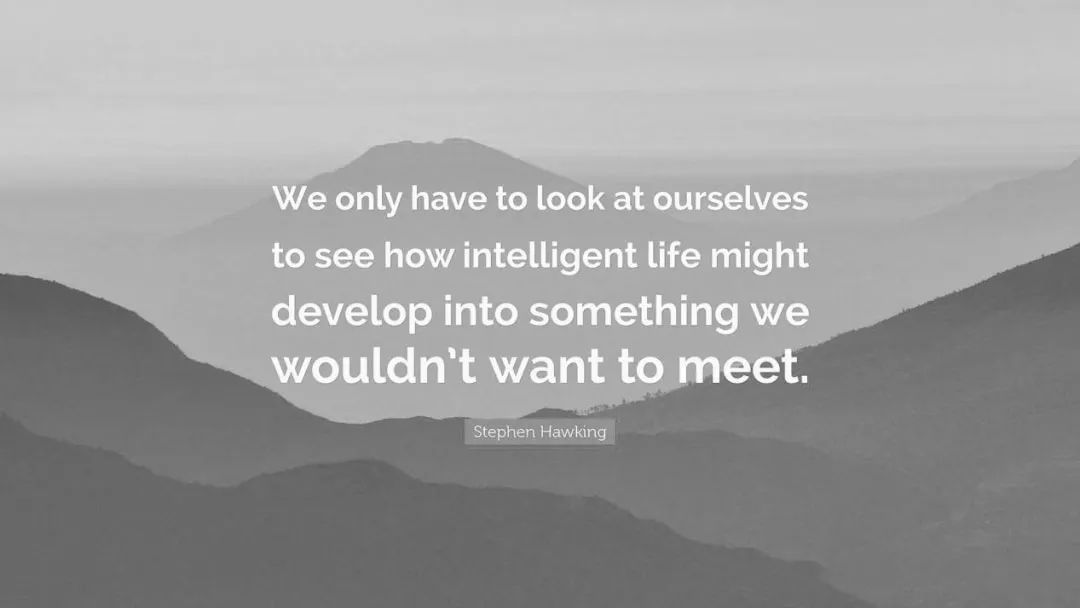
Grayscale Image.jpg
Binarization: Black and White
Most images captured by cameras are color images, which contain a vast amount of information. For the content of the image, we can simply divide it into foreground and background. To allow the computer to recognize the text faster and better, we need to process the color image first, making the image contain only foreground information and background information, where the foreground is simply defined as black and the background as white, which is the binarized image.
The color image that has undergone grayscale processing still needs to go through binarization to further separate the text from the background.
The binarization process involves the concept of “threshold”. Simply put, it aims to find a suitable value to serve as a boundary, where values greater than or less than this boundary turn into white or black, i.e., 0 or 255. So how is the “threshold” chosen?
There are many methods; here are two:
Method 1:
Set the threshold to 127 (the median of 0~255, (0+255)/2=127), making grayscale values less than or equal to 127 turn to 0 (black), and values greater than 127 turn to 255 (white). The advantage of this approach is that it has a low computational cost and is fast, but the downside is also clear: this threshold is fixed at 127 across different images, which can vary greatly in color distribution, so using 127 as a threshold results in poor effectiveness.
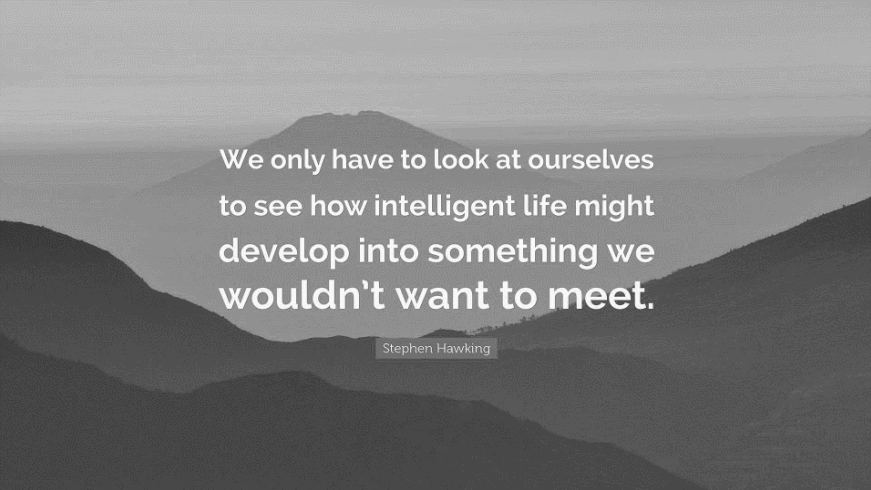
Method 2:
Use the histogram method (also called the bimodal method) to find the binarization threshold. The histogram is an important feature of the image. The histogram method considers that the image consists of foreground and background, with peaks forming on the grayscale histogram for both. The threshold is located at the lowest valley between these two peaks.
The image below can be viewed as all values less than T being black and all values greater than T being white.
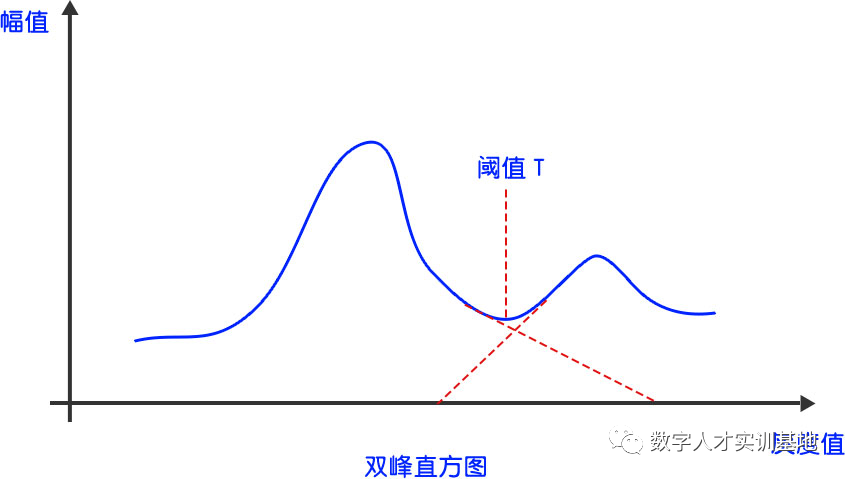
Image Denoising:
Digital images in reality are often affected by noise from imaging devices and external environmental interference during the digitization and transmission process, leading to noisy images. The process of reducing noise in digital images is called image denoising.
During the demonstration, it can be seen that the binarized image displays many small dots, which are unnecessary information and can significantly impact the subsequent contour cutting and recognition of the image. Denoising is a crucial stage, as the quality of denoising directly affects the accuracy of image recognition.
The simplest denoising method involves algorithms learned from DFS or BFS (depth-first search and breadth-first search). We search all connected regions in a w*h bitmap (where the value is 1, appearing black). All connected regions are averaged pixel values, and if some connected regions’ pixel values are significantly lower than this average, we consider them noise points. We then replace them with 0.
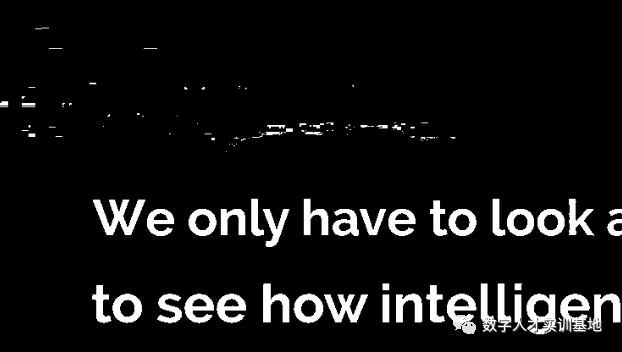
Skew Correction:
Photos or selected images can never be perfectly horizontal, and skew can affect the cropped images later, so the images need to be rotated.
The most common method for skew correction is the Hough transform, which works by dilating the image to connect discontinuous text into a straight line for easier line detection. After calculating the angle of the line, rotation algorithms can be used to correct the skewed image to a horizontal position.
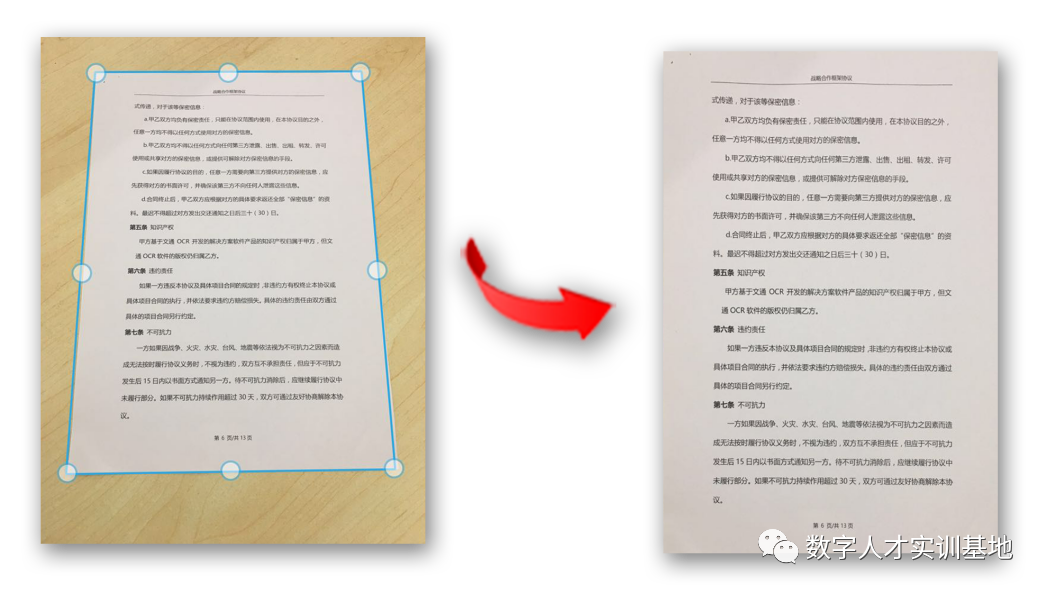
Applications of OCR in Life and Work:
1. Document OCR Recognition
The document OCR recognition technology was initially based on PCs, but in recent years has begun to develop towards mobile platforms, mainly with SDKs for Android and iOS. Currently, mature applications include ID card recognition, driving license recognition, and passport recognition.
2. Bank Card OCR Recognition
Bank card OCR recognition is primarily used for mobile payment binding, representing a highly technical subfield of OCR technology. Some apps already utilize this, such as Alipay and WeChat’s real-name authentication, as well as scanning ID cards during the pandemic for information entry, greatly facilitating life and work.
3. Business Card OCR Recognition
This type of business card OCR recognition technology is now very mature, and most business card management apps on the market have utilized this technology.
4. Document OCR Recognition
Initially, OCR technology was used for document recognition, based on scanning technology, mainly targeting books and newspapers, converting these paper documents into electronic formats. The recognition rates for both Chinese and English are now very high. In recent years, it has also started to be used for mobile document recognition, where a simple scan can achieve recognition.
5. Invoice OCR Recognition
Invoice OCR recognition is used for various types of invoice recognition. Based on a template mechanism, it requires customizing different recognition elements for different invoices. This technology is also known as element recognition OCR and was initially used in the banking sector but is now employed by enterprises, financial institutions, and telecom companies.
6. License Plate OCR Recognition
License plate recognition technology is primarily applied in intelligent transportation and community parking lots. The principle of license plate recognition is to perform OCR on the license plate and then compare it. Currently, this technology has also developed relatively maturely. In daily life, it is used to monitor traffic violations and trace the sources of traffic accidents.
Analyzing the environments of images recognized by OCR, application scenarios can be divided into simple scenes with clear and fixed patterns and complex scenes that are unclear and variable. The difficulty of text recognition in complex scenes is extremely high, due to various reasons including but not limited to: rich backgrounds, low brightness, low contrast, uneven lighting, perspective distortion, and occlusion, as well as issues like twisted layouts, wrinkles, and direction changes in the text. The text may also vary in font, size, weight, and color. In many practical situations, camera shake, focus deviation leading to defocus, or the subject being in motion can cause blur, along with low-quality printing, old and damaged pages, excessive background interference, or poor lighting conditions, all contributing to such text images. This represents a significant challenge faced by current OCR technology.
For blurry, yellowed images, we can use super-resolution technology to enhance the quality of low-quality images.
The basic idea of super-resolution technology is to use signal processing methods to restore high-resolution images from given low-resolution images, achieving images with a resolution higher than that of the imaging system without changing the current hardware. This type of technology is widely applied in image restoration, image reconstruction, monitoring image super-resolution, satellite image super-resolution, and medical imaging.
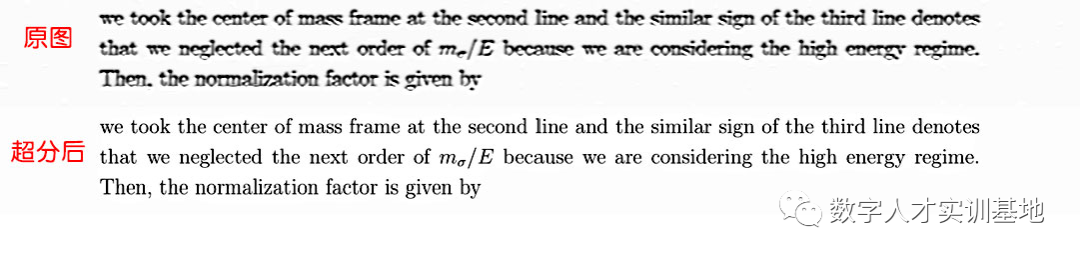
Blurry low-quality documents can attempt to improve quality using super-resolution algorithms, but there is another common type of low-quality document that cannot utilize super-resolution algorithms: documents in tabular form with complex backgrounds. These documents may have clear and neat printing, but the text overlaps, intertwines, and mixes with table lines and colors.
For each type of relatively fixed table color and format under specific scenes, traditional image algorithms can be used to eliminate table lines and background characters, significantly reducing the difficulty of detecting and recognizing target text in the image.
Tables come in various forms, which can be categorized into lined tables, lightly lined tables, and unlined tables based on the presence of borders. Table styles are diverse and complex, such as background fills, lighting shadows, and merged cells. OCR table recognition technology can reduce table processing time and has become one of the technological breakthroughs in recent years.

Flowchart of Table Recognition Technology
Traditional table recognition methods are complex to design, relying on various thresholds and parameter selections for layout analysis and table structure extraction, making it challenging to meet the diverse and complex table scenarios in real life, and further technological breakthroughs are needed.
In practical applications, a large number of OCR projects still rely on massive data augmentation to meet recognition accuracy requirements. However, with the increasing diversity of business scenarios, there is still a need to improve project efficiency.
We are surrounded by text every day, such as our work documents, books, IDs, and product descriptions, all composed of text. The application of OCR technology can simplify and automate some tasks, and in the future, it will accompany our lives, making our lives smarter.
Source: Digital Talent Training Base
This article is for academic sharing only. If there is any infringement, please contact us for deletion.
Download 1: OpenCV-Contrib Extension Module Chinese Tutorial
Reply "Extension Module Chinese Tutorial" in the background of "Xiaobai Learning Vision" public account to download the first Chinese version of the OpenCV extension module tutorial covering over twenty chapters including extension module installation, SFM algorithms, stereo vision, target tracking, biological vision, super-resolution processing, etc.
Download 2: 52 Lectures on Python Vision Practical Projects
Reply "Python Vision Practical Projects" in the background of "Xiaobai Learning Vision" public account to download 31 practical vision projects including image segmentation, mask detection, lane line detection, vehicle counting, eyeliner addition, license plate recognition, character recognition, emotion detection, text content extraction, facial recognition, etc. to assist in quickly learning computer vision.
Download 3: 20 Lectures on OpenCV Practical Projects
Reply "20 Lectures on OpenCV Practical Projects" in the background of "Xiaobai Learning Vision" public account to download 20 practical projects based on OpenCV for advanced learning of OpenCV.
Group Chat
Welcome to join the public account reader group to communicate with peers. Currently, there are WeChat groups for SLAM, 3D vision, sensors, autonomous driving, computational photography, detection, segmentation, recognition, medical imaging, GAN, algorithm competitions, etc. (will gradually be subdivided in the future). Please scan the WeChat ID below to join the group, and note: "Nickname + School/Company + Research Direction", for example: "Zhang San + Shanghai Jiao Tong University + Vision SLAM". Please follow the format; otherwise, you will not be approved. After successful addition, you will be invited to relevant WeChat groups based on your research direction. Please do not send advertisements in the group, or you will be removed. Thank you for your understanding~