
Abstract: Currently, the leapfrog development of Artificial Intelligence Generated Content (AIGC) is transforming the educational environment, significantly impacting graduate students’ learning and research, making their cognitive uncertainty and complexity increasingly apparent. To adapt to the AIGC environment, graduate students need to logically deduce and organize various factors and conditions to generate complex cognition. Therefore, exploring the integration of AIGC with graduate students’ learning and research, and its impact on their complex cognition is particularly necessary. This article first incorporates ChatGPT into graduate courses, and then analyzes the process data of graduate course learning using the “Evidentiary Reasoning” GoE framework and cognitive network analysis method, discovering that under the AIGC environment, graduate students’ complex cognition evolves from simple linear connections and low-level cognitive states to complex network construction and high-level cognitive states; theoretical learning exhibits “non-specialized-integrative” cognitive characteristics, and external interventions can effectively break through graduate students’ low-level cognitive states, presenting an “∞” development trajectory; academic research exhibits “analytical” cognitive characteristics, and “machine-teacher” collaborative guidance can enhance graduate students’ high-level cognitive abilities, presenting a “∑” development trajectory. Finally, the article proposes suggestions to utilize AIGC to build integrated courses to cultivate graduate students’ high-level cognitive thinking, reinforce graduate students’ reasoning practices through prompt pattern teaching, and leverage AIGC as a “cognitive mediator” to facilitate the connection between graduate students’ theoretical learning and academic research, aiming to promote the transformation and development of graduate training models.
Keywords: AIGC; Graduate Students’ Complex Cognition; Evidentiary Reasoning; ChatGPT
//Introduction
With the widespread application of ChatGPT, AIGC has garnered significant attention from all sectors of society due to its powerful text, image, and content analysis and creation capabilities, leading to a paradigm shift in content creation and knowledge learning[1], and producing revolutionary impacts in the education field. Especially in graduate education, graduate students are beginning to use AIGC to complete coherent and content-rich course assignments or academic papers[2]. While this improves efficiency, it also brings educational risks such as “plagiarism” and “copying”, sparking intense discussions among numerous educational researchers and practitioners: some scholars believe that AIGC can empower academic production, playing a role in literature retrieval, assisting in topic selection and viewpoint integration[3]; it can also enhance graduate students’ independent and personalized learning experiences[4], promoting knowledge understanding, problem-solving, and task collaboration. However, some scholars point out that AIGC will trigger educational issues such as scientific ethics, academic integrity, and over-dependence on AI by teachers and students[5], negatively impacting graduate students’ scientific research, knowledge innovation, and development of high-level thinking skills. Therefore, supporters have begun to explore practical paths for transforming graduate training models with ChatGPT, such as Dai et al.[6] transforming the roles and responsibilities of supervisors and graduate students through ChatGPT, enhancing graduate students’ research independence; while opponents propose a series of anti-ChatGPT strategies to weaken the dependence of teachers and students on ChatGPT[7] and improve awareness of academic integrity. It is evident that current discussions surrounding AIGC and its impact on graduate education tend to focus on the opportunities or challenges it brings to educators and graduate students, with the former viewing AIGC as an accelerator of educational innovation, promoting educational reform and improving educational quality; the latter believing that AIGC will lead to the destruction of the education system. These two perspectives highlight the inherent contradictions in AIGC’s role in education and imply that AIGC has become an unavoidable reality in graduate education.
Graduate education is essentially a stage for cultivating students’ abilities in scientific exploration, seeking academic truth, and creating new knowledge[8]. Unlike traditional intelligent technologies, the emergence of ChatGPT signifies that machine intelligence’s essential impact on graduate students has shifted from the instrumental realm of perception and physical extension into the cognitive domain of thinking and consciousness intervention[9], directly impacting the social value of graduate students’ “research and innovation” and the goal orientation of graduate education for high-level talent cultivation; forcing graduate education, which previously emphasized knowledge transmission, skill training, and directed cultivation, to transition towards a thinking quality cultivation model that highlights graduate students’ unique advantages. Therefore, the focus of educational researchers is not on the negative effects brought by AIGC or the enhancement of AIGC’s intelligence itself, but on how to promote the deep integration of AIGC with graduate students’ theoretical learning and academic research, strengthening graduate students’ research capabilities through theoretical learning, stimulating innovative awareness, and cultivating unique thinking styles and comprehensive abilities in graduate students, ultimately achieving the goal of cultivating high-level innovative talents.
Existing research mainly discusses the potential value and hazards of the AIGC environment on teaching and learning, scientific research, and educational management, while practical application research on how AIGC empowers the generation and development of graduate students’ complex cognition is still in the preliminary exploration stage. Therefore, this study incorporates ChatGPT into graduate courses, analyzing the specific manifestations and developmental dynamics of graduate students’ complex cognition in theoretical learning and academic research from the perspective of “evidentiary reasoning”, exploring the impact of the AIGC environment on graduate students’ complex cognition, aiming to provide references for promoting the transformation of graduate training models, facilitating the connection between scientific research and learning, and addressing the challenges of generative complex environments.
// 1 Overview of the Research
1. Graduate Students’ Complex Cognition and Real-World Dilemmas
Complex cognition refers to all psychological processes through which individuals acquire new information from given information to solve problems, make decisions, and plan actions, occurring under complex conditions where various cognitive processes interact with each other or with other non-cognitive processes[10]. It is the thinking structure that individuals rely on their environment to organize and adjust their behavior based on personal complex goals[11]. Unlike other student groups, graduate students, as a combination of learners and researchers[12], face sustainable learning and research objectives, as well as the survival needs to tackle social challenges, emphasizing high-level conceptual learning and complexity science research. Therefore, graduate students not only need to possess stronger professional knowledge, experience, and skills[13], but more importantly, they need to develop high-level cognitive thinking to drive the deep construction and sublimation of knowledge and skills, promoting academic research. Graduate students’ complex cognition is the thinking process that utilizes external information for structured reasoning within the action logic chain of “theoretical learning-academic research”[14]. Specifically, it refers to the ability of graduate students to efficiently identify, analyze, interpret, evaluate, and extract various data information when faced with more challenging and dynamic theoretical learning or academic research tasks, organically integrating it with their own knowledge and experience to achieve output and optimization[15]. This thinking process develops with graduate students’ diverse learning and research experiences, as well as external interventions (such as guidance feedback from supervisors, academic exchanges, etc.)—evolving from simple knowledge associations to conceptual constructions, and then to multidimensional complex associative applications, gradually forming a more systematic and high-level cognitive framework to support graduate students in deeply exploring the essence of problems and conducting innovative academic research. The level of graduate students’ complex cognition is a key pathway to differentiate them from domain experts and is also crucial for their growth into experts. By continuously developing and strengthening complex cognition, graduate students can flexibly respond to external environmental challenges, handle various uncertainties, and achieve self-driven personal growth.
Therefore, promoting the generation and development of graduate students’ complex cognition and advancing their theoretical innovation and academic research has become an important issue that the field of graduate education needs to focus on. Liao Xiangyang et al.[16] point out that graduate training should not be confined to specific knowledge and skills, but should emphasize cognitive leaps, transforming and innovating graduate students’ methodologies for understanding, interpreting, and observing things in a new way, thereby adapting to the development of the intelligent era. However, in reality, graduate students’ complex cognition faces numerous dilemmas: 1) Narrow cognition caused by a superficial curriculum system. Du Yanyan et al.[17] point out that the knowledge content of graduate courses is outdated, boundaries are chaotic, and structures are singular, neglecting the cultivation of high-level cognition and innovation abilities, leading to fragmented knowledge structures for graduate students, hindering their problem-solving and research innovation capabilities. 2) Cognitive disconnection caused by the separation of research and teaching. The essence of graduate education lies in organically integrating research and teaching to cultivate students’ problem awareness, academic literacy, and comprehensive abilities. However, in practice, the problem of fragmented knowledge transmission contradicts the interdisciplinary characteristics of topic research, with graduate students primarily conducting scientific research from a single disciplinary perspective, lacking academic reflection on solving cross-disciplinary complex problems and knowledge transfer[18], resulting in cognitive disconnection and weakened motivation between research and learning. 3) Cognitive alienation brought about by the “human-like” nature of AIGC technology. General-purpose large models represented by ChatGPT possess certain human-like thinking abilities, capable of replacing humans in learning and even becoming new “knowledge centers”, challenging graduate students’ cognitive limits, leading to issues such as self-cognitive inadequacy and homogenized thinking. It is evident that we must deeply consider the opportunities and challenges brought by the new technological environment to effectively stimulate graduate students’ complex cognition, break through their real-world dilemmas, and achieve high-quality development for graduate students.
2. The Multidimensional Impact of the AIGC Environment on Graduate Students’ Complex Cognition
Compared to general databases and search engines, large language models like ChatGPT and Wenxin Yiyan have broken through the technical bottlenecks of natural language processing, relying on powerful computing power, algorithms, and data feeding[19], achieving autonomy, adaptability, and creativity in content generation, while also exhibiting considerable levels of awareness and thinking, accelerating the intelligent development of graduate education, further highlighting the talent cultivation goals of complex cognition and high-level thinking. Theoretical learning and academic research are the two main approaches to achieving graduate education objectives[20], where theoretical learning focuses on cultivating graduate students with a broad knowledge base and profound expertise, while academic research emphasizes the cultivation of graduate students’ independent research, exploratory innovation abilities, and scientific thinking and academic quality. The widespread application of AIGC technology in graduate students’ theoretical learning and academic research brings both positive and negative impacts on their complex cognition. In terms of theoretical learning, AIGC acts as a virtual mentor, providing personalized tutoring and answering questions based on graduate students’ individual learning needs and progress[21], helping them bridge knowledge gaps and promoting learning growth. Simultaneously, AIGC takes on the role of domain expert, generating specific professional knowledge and research methods through in-depth dialogue[22], providing professional guidance for graduate students to solve complex problems, achieving cognitive transfer and contextual transformation of theoretical knowledge. In academic research, AIGC serves as a research assistant, helping graduate students organize literature, collect and summarize information, expand research ideas, and stimulate inspiration[23]; it can also enhance the quality of paper writing, guiding graduate students to focus on knowledge innovation and scientific discovery, achieving cognitive leaps.
The duality of technology means that graduate students must recognize the potential problems and limitations of large language models when applying them for theoretical learning and academic research: 1) Data bias. Due to the limitations of the training dataset, biases in AIGC-generated data may lead to erroneous information or discrimination[24], affecting the objectivity and accuracy of graduate students’ problem analysis and research, even hindering them from forming and developing a systematic professional knowledge structure[25], leading to cognitive biases. 2) Information uncertainty. AIGC’s content generation does not provide corresponding materials or literature citations, reference lists[26], increasing the difficulty for graduate students to identify, evaluate, and verify literature viewpoints, hindering academic research innovation, and even making them absolute advocates of AIGC content authority, forming a “cognitive cocoon”. 3) Technological opacity. AIGC’s model algorithms and operational processes still exhibit “black box” characteristics, meaning users cannot know the internal structure, working principles, and information processing processes of the system, only passively accepting the output data from the model[27], weakening graduate students’ logical deduction, factual reasoning, and other cognitive processes, making it difficult to ensure coherence, integration, and effectiveness of knowledge content and academic issues, falling into a “cognitive black box”. Therefore, the increasing complexity of the AIGC environment necessitates that graduate students engage in multi-faceted, comprehensive evidentiary reasoning and exploration to promote the generation of complex cognition and drive the innovative development of their theoretical learning and academic research.
3. The Research Basis and Theoretical Framework of “Evidentiary Reasoning”
The concept of “Evidentiary Reasoning” originates from the practical argumentation in the field of science education[28], and is a complex skill specification that learners must possess to participate in knowledge society proposed in the American “Next Generation Science Standards” and “K-12 Science Education Framework”, which refers to applying scientific evidence for inquiry, argumentation, and construction, specifically applying disciplinary theories, norms, and methodological frameworks to generate evidence and assess the validity of scientific claims, as well as their consistency and relevance to real-world problems[29]. The core skill of “Evidentiary Reasoning” has become increasingly important in the context of the continuous development of intelligent technologies and the proliferation of erroneous and false information, requiring learners to maintain cognitive vigilance and construct viewpoints based on evidence[30]. Although numerous studies have focused on the complex processes of learners’ evidentiary reasoning and the developmental outcomes of scientific knowledge, delving into specific domain knowledge and external intervention scaffolds that influence learners’ advanced evidentiary reasoning, there is a lack of definitions for the nature of evidence and examinations of learners’ intrinsic cognition. At the same time, in practice, learners’ use and cognitive understanding of scientific evidence are limited, making it difficult to accurately assess the quality and strength of evidence and weigh and integrate evidence from different sources and types to support claims[32], hindering their scientific research and development of high-level abilities. To address these challenges, Duncan et al.[33] proposed the “Grasp of Evidence” (GoE) framework, systematically interpreting the true forms of evidence and reasoning processes, which specifically includes understanding how to combine relevant evidence operational elements; how to adaptively participate in evidentiary reasoning in various environments and implement higher-level metacognitive regulation. This framework consists of five dimensions: non-professional use of evidence, evidence analysis, evidence evaluation, evidence interpretation, and evidence integration, which are interrelated and reflect the cognitive foundation and development process required for individuals to grow from non-professionals to domain experts. Many studies have used this theoretical framework as an analytical tool for practical verification, revealing learners’ internal cognitive mechanisms; for example, Kim et al.[34] used this framework as a perspective to examine undergraduate students’ critical evaluation of knowledge claims related to social science issues, revealing different types of evidence practices by learners and the cognitive changes behind them, providing references for promoting learners’ evidentiary reasoning and critical thinking development; Duncan et al.[35] verified that the GoE framework could cultivate students’ cognitive vigilance, effectively reducing the cognitive risks brought by erroneous information, and prompting them to form the ability to reason about knowledge claims and evidence in complex cognitive environments.
With the advancement of graduate education’s popularization, the student population will become increasingly diverse, requiring graduate education to optimize quality while expanding scale and innovating development concepts[36]. Practice shows that current graduate courses are generalized, lacking unified standards and normative guidance for the basic knowledge, literacy, and high-level thinking skills of graduate students. Meanwhile, the basic characteristics of research and innovation in graduate education, as well as the reality of machine intelligence challenging human cognitive formation, require graduate students to possess highly organized complex cognition to drive their capability transformation, achieving skill development and intelligent symbiosis through human-machine collaboration. Existing research has focused on uncontrollable external factors such as institutional types and course systems[37], as well as supervisor support and feedback[38], on graduate students’ academic research and innovation capabilities, while “evidentiary reasoning” emphasizes internal factors, aiming to promote learners’ participation in reasoning practices across different dimensions, enhancing their cognitive understanding of complex information, thereby forming high-level thinking skills that adapt to and transform the external world. This is essentially a reflection of graduate students’ complex cognition, which is the process of converting internal thoughts into external actions, namely analyzing, deducing, and weighing different dimensions of knowledge or problems to achieve problem-solving or scientific discovery. This process can reflect the occurrence and changes of complex cognition among graduate students during theoretical learning and academic research, revealing the specific impact of the AIGC environment on graduate students’ complex cognition.
Research on ChatGPT in education suggests that it will impact graduate students’ cognitive thinking in theoretical learning and academic research, but there is little discussion on the specific impacts and how to respond. Therefore, this study focuses on the following two questions to deeply explore the interactions and cognitive processes of graduate students with ChatGPT during learning: 1) What changes occur in graduate students’ complex cognition in the AIGC environment? 2) What characteristics and developmental differences do graduate students’ complex cognition exhibit when applying ChatGPT in theoretical learning and academic research?
// 2 Research Design
1. Research Subjects
This study is based on the “Information Technology Course Teaching Research” course for the 2022 graduate students of the Educational Technology major at University A in Jiangsu Province, with participants being 33 students from the same class. This course is divided into theoretical learning and academic research, aiming to help graduate students master research theories and methods, construct professional knowledge systems, solve academic research problems, and enhance research literacy.
2. Course Design
This course lasts for 6 weeks, and the specific design is as follows—
1) Theoretical Learning Part: Week 1 “Specific Topic Discussion”, requiring graduate students to ask questions to ChatGPT about a given topic, exploring application techniques and generation mechanisms, and initially understanding relevant concepts. Weeks 2-4 “Open-ended Course Problem Exploration”, where graduate students use prompts to interact with ChatGPT, forming answers to questions through analysis, interpretation, and integration reasoning. Weeks 5-6 “Restricted Complex Tasks”, where graduate students collaborate with ChatGPT to complete tasks such as “Teaching Plan Evaluation Criteria Construction” and “Selection of Excellent Teaching Plans”, providing specific processes, evaluation criteria, preferred reasons, and final results.
2) Academic Research Part: Week 1 “Independent Topic Selection for Papers”, requiring graduate students to set specific scenarios to analyze and evaluate the authenticity and reliability of the content generated by ChatGPT to determine the topic. Week 2 “Paper Framework Construction”, where graduate students use ChatGPT to deeply understand the logical relationships between various parts such as theoretical content and research methods, constructing the paper framework. Weeks 3-5 “Paper Writing Training”, requiring graduate students to use ChatGPT to verify the quality of evidence such as methods, strategies, and data results, systematically explaining research problems and assisting in paper writing. Week 6 “Teacher Guidance”, where teachers provide feedback on graduate students’ papers, allowing them to optimize and refine their papers.
The course requires students to submit the final learning outcome text and the process documents of their interactions with ChatGPT. Additionally, this study provides progressive prompt scaffolding in Week 2 to guide graduate students in their dialogue with ChatGPT to obtain target content.
3. Coding Framework
This study reinterprets the definitions of each dimension based on the GoE framework, arranging them from low-level to high-level according to cognitive levels, which include non-professional use of evidence, evidence analysis, evidence evaluation, evidence interpretation, and evidence integration, forming the “Evidentiary Reasoning” coding framework as shown in Table 1.
Table 1: “Evidentiary Reasoning” Coding Framework
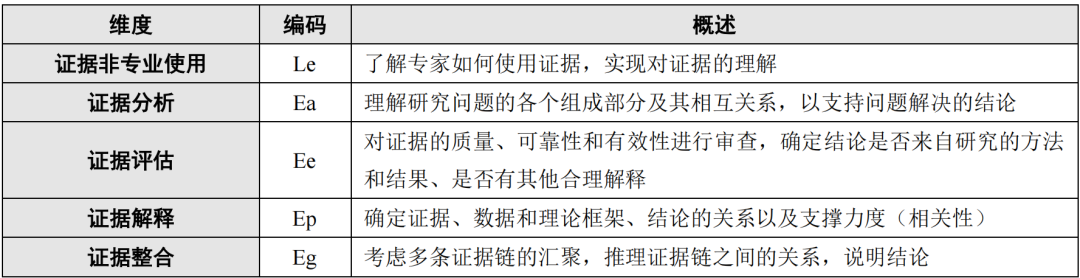
4. Data Collection and Processing
After the course, this study collected course learning process data from 33 students, including complete dialogues between graduate students and ChatGPT under different learning topics, as well as final outcome texts. Some dialogues unrelated to the topic content or “Evidentiary Reasoning” (e.g., “Question: You don’t need to write now, wait for me to provide it to you for rewriting”) were excluded, resulting in 1261 valid data entries. Then, two researchers used Nvivo 12 to conduct back-to-back coding based on the coding framework, determining whether each text data entry met the coding dimensions. Finally, after discussion and negotiation, a structured dataset was obtained, with a coding consistency coefficient of 0.876, indicating good reliability of the research data. After coding was completed, this study used ENA Webkit to conduct cognitive network analysis on the dataset, using the five dimensions of “Evidentiary Reasoning” as code elements in the ENA model, with the window set to Whole Conversation.
//3 Results and Discussion
1. Establishing a Plane Reference Frame for the Representation of “Evidentiary Reasoning” Dimensions
This study overlays the cognitive networks of all graduate students through ENA, performing spherical normalization and singular value decomposition dimensionality reduction to construct a plane reference frame for representing the dimensions of “Evidentiary Reasoning” (as shown in Figure 1). Here, the Pearson and Spearman coefficients of the x-axis (SVD1, 62.8%) are both 1, while those of the y-axis (SVD2, 20.8%) are both 0.99, indicating high goodness of fit. The nodes correspond to five cognitive elements, with their sizes reflecting the relative frequencies of element occurrences, and positions representing the network association structure of graduate students’ complex cognition.
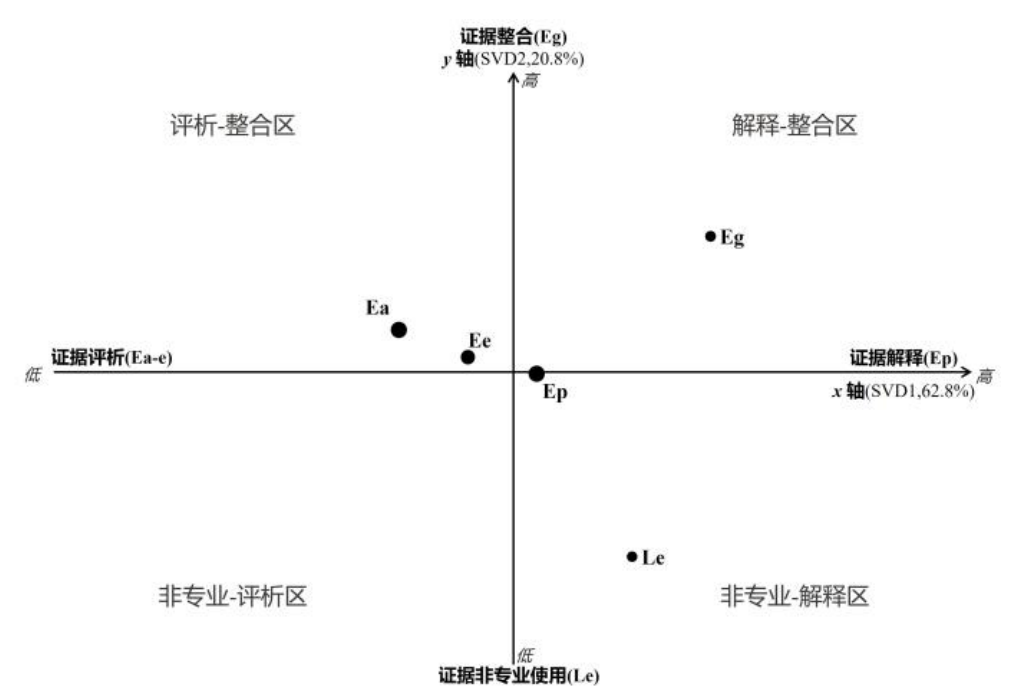
Figure 1: Plane Reference Frame for the Representation of “Evidentiary Reasoning” Dimensions
Based on the node position distribution in Figure 1, this study defines the x-axis as “evidence evaluation (Ea-e) – evidence interpretation (Ep)” and the y-axis as “non-professional use of evidence (Le) – evidence integration (Eg)”. The intersection of the two axes forms four areas, namely the “interpretation-integration zone”, “evaluation-integration zone”, “non-professional-evaluation zone”, and “non-professional-interpretation zone”. The first quadrant indicates that graduate students construct multi-angle, high-quality evidence chains based on in-depth research and professional knowledge, explaining complex problems more accurately and thoroughly, refining core viewpoints through induction and transformation; it possesses scientific rigor and innovation, representing a high-level cognitive state. The second quadrant indicates that graduate students analyze various information content using professional theoretical frameworks and systematic methodological strategies, re-integrating them according to their logical relationships; it possesses professionalism and comprehensiveness, situated at a relatively high cognitive level. The third quadrant indicates that graduate students analyze and evaluate a particular problem or content using general knowledge, demonstrating a degree of orderliness and accuracy, but facing professional limitations, making it difficult to generate highly specialized viewpoints and solutions, situated at a lower cognitive level. The fourth quadrant indicates that graduate students explain problems or topics based on personal daily experiences and subjective feelings, exhibiting subjectivity and partiality, situated at a low-level cognitive state. Thus, this study divides the plane reference frame from low to high into four cognitive states to reflect the cognitive changes and main characteristics of graduate students in different contexts.
2. Overall Process Changes in Graduate Students’ “Evidentiary Reasoning”
Figure 2 illustrates the cognitive network structure exhibited by graduate students’ “Evidentiary Reasoning” over the 6 weeks, representing the generative changes of complex cognition under the AIGC environment. The thickness and saturation of the connecting lines in the figure reflect the degree of association between the dimensions of “Evidentiary Reasoning”, with small dots representing the centroids of each graduate student’s “Evidentiary Reasoning” cognitive network structure.
Integrating the cognitive network structure and centroid dispersion and displacement states in Figure 2 reveals that in Week 1, the centroids scatter across various quadrants, exhibiting weak connections of Le, Ea, Ee, and Ep, indicating that at the beginning of the course, graduate students’ cognition shows significant differences, focusing more on surface phenomena of problems while neglecting the implicit inner essence and complex relationships, revealing the limitations of their original cognition. In Week 2, the centroids gather more in the first quadrant, activating multiple dimensions of graduate students’ “Evidentiary Reasoning,” establishing rich connections among them, forming a “←” structure based on Le and Eg, indicating that prompt scaffolding effectively promotes the generation of complex cognition among graduate students. In Weeks 3 and 4, the centroids display a significant linear distribution in the second and third quadrants, with the former presenting a tightly connected triangular structure of Le-Ea-Ee-Ep, while the latter derives connection lines with the high-level “integration (Eg)”, indicating that in Week 3, graduate students tend to reason using the “non-professional evaluation-interpretation” logical chain, while Week 4 shows development towards a higher cognitive level. In Week 5, some centroids scatter towards the first quadrant, with lines associated with Eg also increasing and strengthening, suggesting that graduate students’ cognitive structure gradually becomes more complex; in Week 6, the centroids overlap in the first quadrant, showcasing a trend of high-level cognitive development, with the cognitive network structure of graduate students comprehensively developing, allowing them to construct research problems from a professional domain perspective and achieve advanced evidentiary reasoning. In summary, graduate students’ complex cognition under the AIGC environment gradually becomes structured, presenting a transition from low to high cognitive states.
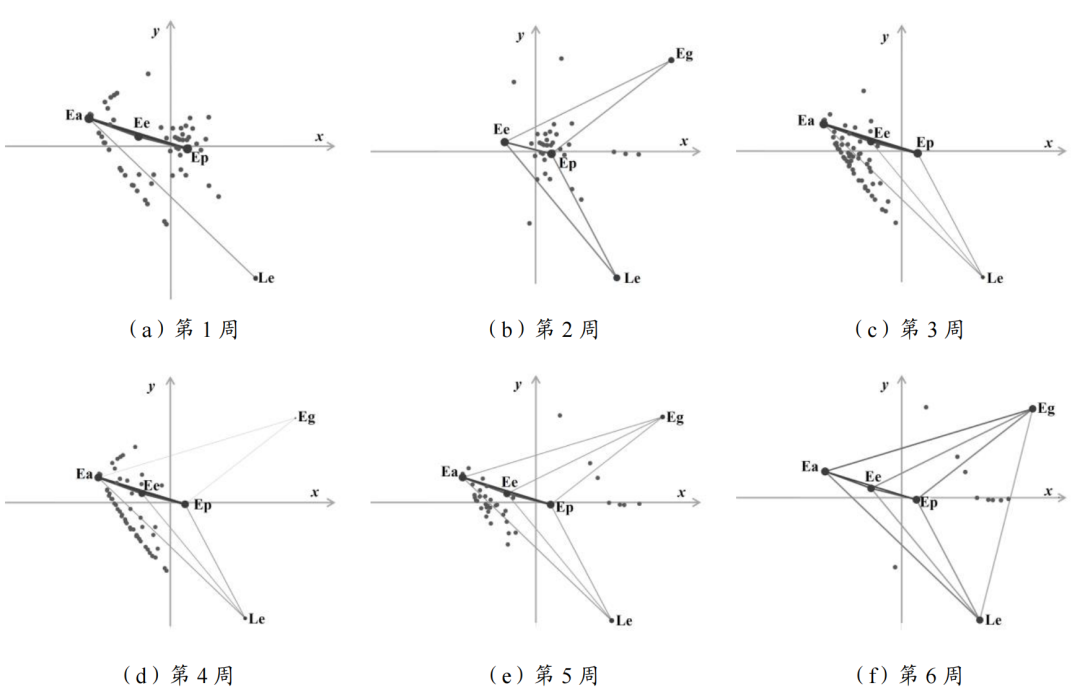
Figure 2: Cognitive Network Structure of Graduate Students’ “Evidentiary Reasoning” Over 6 Weeks
3. Main Characteristics and Development Trajectories of “Evidentiary Reasoning” in Theoretical Learning and Academic Research
(1) Main Characteristics
Figure 3 compares the cognitive networks of “Evidentiary Reasoning” in graduate students’ theoretical learning and academic research, highlighting the main characteristics and differences of complex cognition in the two domains.
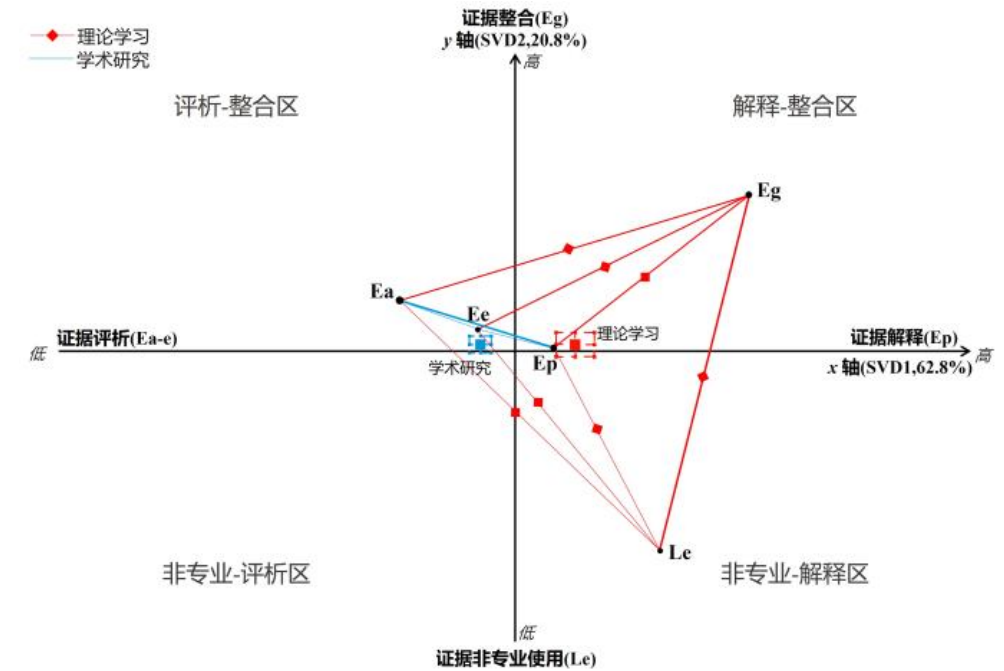
Figure 3: Comparison of “Evidentiary Reasoning” Cognitive Networks in Theoretical Learning and Academic Research
Figure 3 shows that graduate students exhibit significant differences in complex cognition during theoretical learning and academic research. In theoretical learning, the cognitive centroids of graduate students are located on the right side of the coordinate system, focusing more on the basis of “non-professional use of evidence” (Le) and “evidence integration” (Eg), constructing rich connections with “evidence analysis” (Ea), “evidence evaluation” (Ee), and “evidence interpretation” (Ep), indicating that graduate students tend to conduct logical reasoning from an external perspective, deepening conceptual learning with the aid of ChatGPT, clarifying the internal connections of “advanced knowledge”, and constructing personal knowledge structures, demonstrating “non-professional-integrative” cognitive characteristics. In academic research, the cognitive centroids of graduate students are located on the left side of the coordinate system, highlighting the connections of Ea and Ep compared to theoretical learning, with a co-linearity coefficient of 0.18. This indicates that during the academic research process, graduate students are more inclined to use ChatGPT for “evidence analysis and interpretation” to grasp cutting-edge knowledge in their disciplines, explore research problems, and form personal understanding, exhibiting “analytical” cognitive characteristics.
(2) Development Trajectories
This study uses weeks as the analysis unit, plotting the centroids of graduate students’ “Evidentiary Reasoning” cognitive networks in theoretical learning and academic research over 6 consecutive weeks, dynamically presenting development trajectories, as shown in Figures 4 and 5.
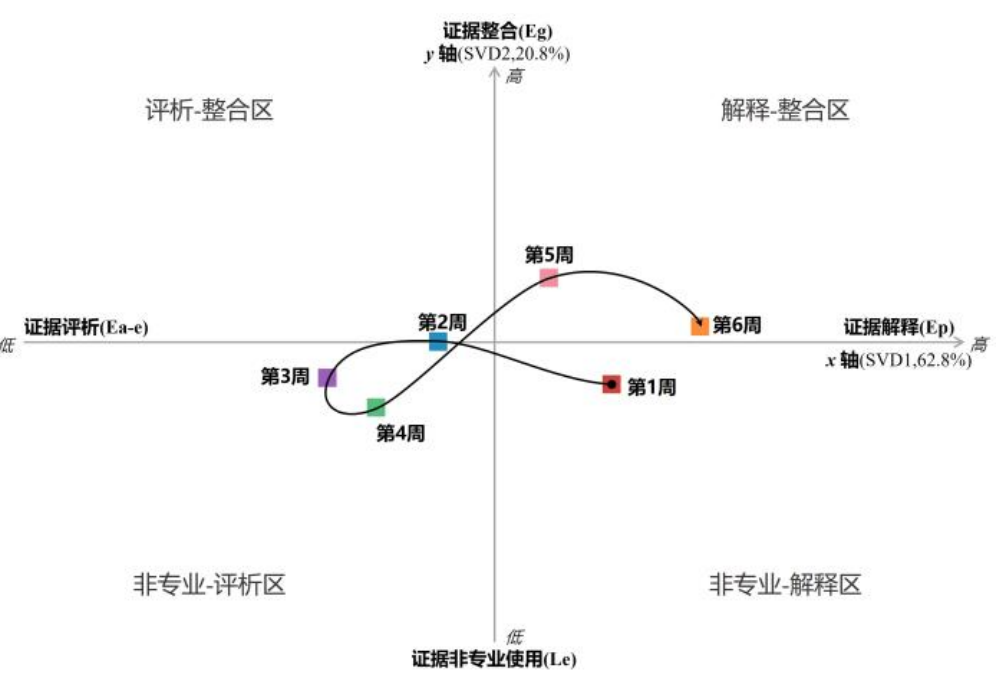
Figure 4: Development Trajectory of “Evidentiary Reasoning” Cognitive Network in Theoretical Learning
From Figure 4, it can be seen that in Week 1, the cognitive network centroid of graduate students in theoretical learning is located in the fourth quadrant, primarily reflecting a shallow understanding of problem content. This is because graduate students are in the preparatory stage of research, with weak research awareness, focusing more on leveraging ChatGPT’s powerful information search and analysis capabilities to initially recognize and understand paper topics, presenting a “non-professional-interpretation” low-level cognitive state. In Week 2, the centroid shifts towards the left half of the x-axis, as specific prompt support helps graduate students quickly analyze, dissect, and verify professional knowledge, discovering contradictions and conflicts between the content generated by ChatGPT and the target content, forming a relatively comprehensive “evaluation” cognition. In Weeks 3 and 4, the centroid remains in the third quadrant, revealing lower-level “non-professional-evaluation” cognitive characteristics. During this stage, graduate students lack prompts and problem scaffolding to intervene in the cognitive process, making it difficult to abstractly integrate and apply the analysis and conclusions of complex cognition, limiting the development of high-level cognition. In Week 5, by setting complex task scenarios, graduate students are guided to break down and coordinate multiple sub-task contents to produce results. In this process, graduate students gradually improve their multidimensional dialectical thinking abilities, as well as their abilities to disassemble, reorganize, and refine knowledge, with the “interpretation-integration” high-level cognition developing, and the cognitive network centroid moving to the first quadrant. In Week 6, the centroid continues to evolve deeply in the first quadrant, indicating that during the solution of complex tasks, graduate students timely update their objectives to activate high-quality outputs from ChatGPT, continuously honing their rational examination and reflective awareness, enhancing knowledge transfer abilities, with the “interpretation-integration” high-level cognitive characteristics further highlighted. It is evident that through the theoretical learning process utilizing ChatGPT, graduate students’ complex cognition evolves from “non-professional-interpretation, evaluation” states to “interpretation-integration” states, presenting an “∞” development trajectory; simultaneously, external interventions (prompt patterns and complex tasks) can break through the low-level cognitive states of “non-professional-interpretation, non-professional-evaluation”, promoting the generation and development of high-level cognition in graduate students’ theoretical learning.
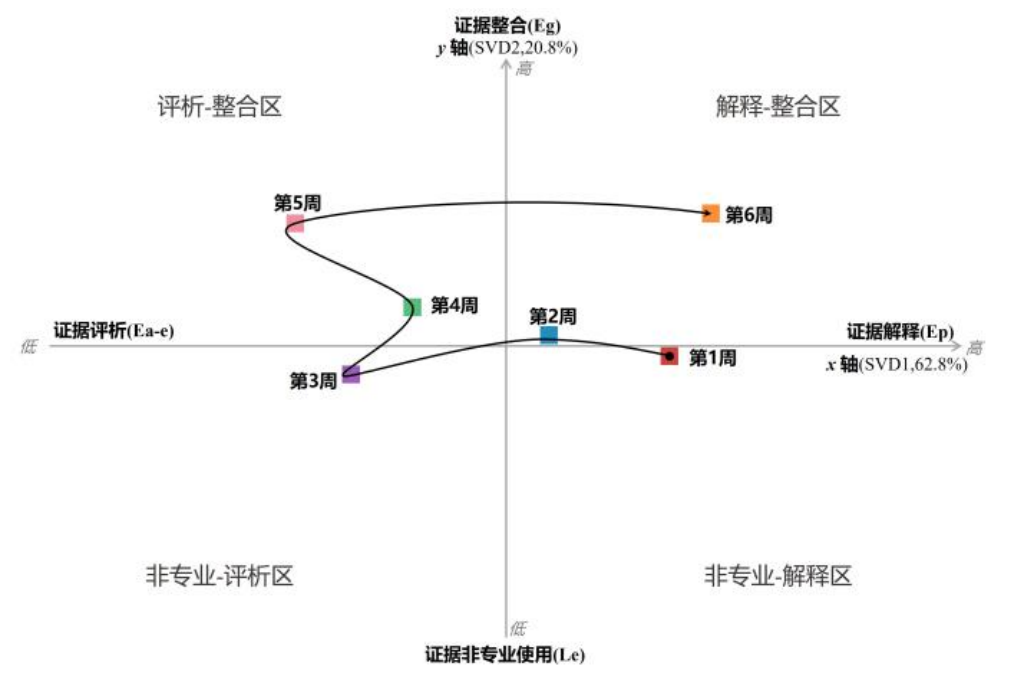
Figure 5: Development Trajectory of “Evidentiary Reasoning” Cognitive Network in Academic Research
From Figure 5, it can be seen that in Week 1, the centroid of academic research starts in the fourth quadrant, primarily reflecting a shallow understanding of problem content. This is because graduate students are in the preparatory stage of research, with weak research awareness, focusing more on leveraging ChatGPT’s powerful information search and analysis capabilities to initially recognize and understand paper topics, presenting a “non-professional-interpretation” low-level cognitive state. In Week 2, the centroid shifts towards the first quadrant, as prompt scaffolding regarding paper topics gradually guides graduate students to delve deeper into the content scope and research problems, forming a rough paper framework, and the complex cognition of graduate students also develops. In Week 3, the centroid moves to the third quadrant as graduate students begin writing their papers, attempting to use ChatGPT to analyze and extract relevant research literature and materials, judging the suitability and importance of the literature content for their papers, laying the groundwork for writing. However, graduate students are easily constrained by their existing knowledge and experience, making it difficult to achieve effective academic innovation and critical thinking, exhibiting “non-professional-evaluation” cognitive characteristics. In Week 4, the centroid is in the second quadrant and continues to develop upwards in Week 5, indicating that with the advancement of systematic paper writing training, graduate students can continuously refine various sub-research contents through ChatGPT and conduct consistency evaluations of related materials, attempting to present their thinking discoveries in a structured manner, drafting the initial version of their papers, exhibiting “evaluation-integration” cognitive characteristics. In Week 6, the centroid moves to the “interpretation-integration zone”, as teachers, combining their professional knowledge and in-depth understanding of ChatGPT, provide developmental feedback for graduate students’ evidentiary reasoning and targeted guidance for papers, prompting graduate students to achieve a qualitative leap in high-level cognition based on prior accumulation. Graduate students can integrate content from ChatGPT with external information, incorporating personal understanding and viewpoints to solve problems, construct research theories, and produce quality research papers. Therefore, this research concludes that in academic research, graduate students’ complex cognition gradually elevates from the “non-professional-interpretation” low-level cognitive state to the “interpretation-integration” high-level cognitive state, presenting a “∑” development trajectory; simultaneously, the collaborative guidance of teachers and ChatGPT’s intelligence can significantly enhance graduate students’ high-level cognitive abilities in academic research.
In summary, the theoretical learning of graduate students exhibits “non-professional-integrative” cognitive characteristics, while academic research exhibits “analytical” cognitive characteristics, both showing a deep developmental trend from low-level cognitive states to high-level cognitive states. In this process, the role and effects of ChatGPT on graduate students’ complex cognition manifest differently; specifically, ChatGPT demonstrates significant advantages in conceptual analysis, problem decomposition, task refinement, and feedback optimization in theoretical learning, but may be overly general and prone to errors in practical applications; in academic research, ChatGPT performs excellently in literature integration, topic focusing, framework construction, viewpoint comparison, and method selection, assisting graduate students in improving research efficiency, but the content’s innovativeness, contextuality, and practicality require graduate students to perceive and enhance independently.
// 4 Research Recommendations
1. Utilize AIGC to Construct Integrated Courses to Cultivate Graduate Students’ High-Level Cognitive Thinking
Courses are key to enhancing graduate students’ research levels and innovative capabilities, and the quality of the course system directly impacts talent cultivation quality. However, the current graduate course system lacks breadth and depth, as well as unified standards for the unique thinking skills of graduate students, leading to a disconnect between what graduate students learn and research and societal needs[39]. At the same time, with the growth of machine intelligence like AIGC, which develops classification, clustering, and other thinking skills, the core value of graduate education is no longer just the knowledge transmitted by disciplines or courses, but rather the need to cultivate graduate students’ high-level thinking to adapt to the development of knowledge-based intelligent society, achieving knowledge innovation and scientific discovery[40]. Analysis results show that under the AIGC environment, graduate students’ complex cognition gradually becomes structured, presenting a transition from low to high cognitive states, indicating that incorporating ChatGPT into graduate courses is beneficial for the generation and high-level development of graduate students’ complex cognition, achieving multidimensional thinking and reasoning for complex problems.
Based on this, we can utilize the interdisciplinary integration features of AIGC to construct a curriculum system that integrates cross-disciplinary knowledge and thinking skills: 1) By integrating the inherent logical connections between different disciplinary knowledge through AIGC, constructing course content that innovates through the intersection of various research tools and methods, and conducting multi-level, multi-type course activities oriented towards cutting-edge academic ideas, enhancing graduate students’ cognitive and emotional experiences[41], helping them conduct academic research from different theoretical and methodological perspectives. 2) By creating complex problem scenarios that integrate multiple disciplines using AIGC, associating specific thinking with knowledge from multiple disciplines, prompting graduate students to form a cross-disciplinary knowledge system and thinking skills in the layered problem-solving process; through the transformation of different contexts, regulating, critiquing, innovating, and reconstructing existing thinking skills, achieving high-level cognitive leaps.
2. Implement Personalized Prompt Pattern Teaching to Strengthen Graduate Students’ Reasoning Practices
The educational application potential of AIGC technologies like ChatGPT and Wenxin Yiyan depends on the types and quality of the prompt patterns users formulate[42]. Research has found that progressive prompt scaffolding can fully stimulate high-quality outputs from ChatGPT, guiding graduate students’ deep evidentiary reasoning to achieve specific learning goals. This aligns with research on the evaluation and feedback of student argumentation content supported by ChatGPT, indicating that well-designed questioning helps ChatGPT generate higher quality feedback, positively impacting students’ arguments[43]. Other studies also emphasize that teachers must provide guidance and explanations for different types of prompt content and its relevance to specific tasks, helping students effectively guide AIGC to generate high-quality, multimodal knowledge to solve practical problems[44].
Therefore, in practical teaching processes, teachers need to combine learning tasks with prompt patterns, guiding graduate students to select, design, or combine different types of prompts in various task-solving processes, fully mastering the specific abilities of AIGC to strengthen reasoning practices, meet learning needs, and break through cognitive development dilemmas: 1) Teachers can combine professional knowledge and experience to determine the expected uses of AIGC outputs in different learning or research tasks for graduate students, providing explanations and application conditions for different types of prompt patterns, laying the foundation for graduate students to combine prompts and analyze problems. 2) Teachers can set collaborative tasks for creating prompts, prompting graduate students to continuously explore different prompt types (such as flipped interaction prompts, reflective prompts, etc.) during reasoning processes, understanding whether specific prompt types will guide AIGC to generate desired outputs, and combining and expanding initial prompt patterns based on task conditions, enhancing the cultivation of graduate students’ unique reasoning practices and AIGC prompting abilities, thereby addressing cognitive challenges in complex environments.
3. Leverage the “Cognitive Mediator” Role of AIGC to Facilitate the Connection Between Theoretical Learning and Academic Research
This study finds that graduate students’ theoretical learning exhibits “non-professional-integrative” cognitive characteristics, presenting an “∞” development trajectory; while academic research exhibits “analytical” cognitive characteristics, showing a “∑” development trajectory. The cognitive characteristics of both areas differ, yet their developmental trajectories exhibit consistency in transitioning from “non-professional-interpretation” states to “interpretation-integration” states. This indicates that AIGC’s powerful information processing, aggregation, integration, and generation capabilities can help graduate students adopt new theoretical perspectives and methodological strategies to solve complex problems in research, while also improving their understanding and transfer application abilities of theoretical knowledge in research exploration, thus becoming a key cognitive mediator for the connection and conversion of the two.[45] This promotes the collaborative development of graduate students’ theoretical learning and academic research.
Effectively leveraging the “cognitive mediator” role of AIGC requires multi-dimensional verification and problem adjustment strategies. Among them, multi-dimensional verification refers to employing various methods (such as scientific verification, practical verification, cross-field intersection verification[46], generative verification, etc.) to scientifically analyze and validate AIGC’s data, algorithms, content, and logic, enhancing awareness of the demystification of AIGC’s knowledge magic and discernment abilities; problem adjustment involves adjusting AIGC’s content output based on specific problems to better complete tasks. Therefore, firstly, graduate students should verify AIGC’s output content and generation logic from multiple angles by comparing information data from different sources, consulting authoritative literature, etc., guiding AIGC to construct a specific theoretical background. In this process, graduate students’ personal knowledge and thinking logic are continuously overturned and reorganized, achieving higher-level development through reflection and abstraction. Secondly, introducing research problems into the specific contexts created by AIGC, designing different research scenarios around the problems, generating metacognitive knowledge and strategies, guiding graduate students to integrate personal theoretical hypotheses, explore practical solutions, and thereby verify viewpoints, argue plans, and construct theories, achieving integration and connection between theoretical learning and academic research.
References
[1]Wang Y, Pan Y, Yan M, et al. A survey on ChatGPT: AI-generated contents, challenges, and solutions[J]. IEEE Open Journal of the Computer Society, 2023,(4):280-302.
[2]Zhai X. Chatgpt for next generation science learning[J]. XRDS: Crossroads, The ACM Magazine for Students, 2023,(3):42-46.
[3]Luo Fei, Ma Yuxuan. The impact of AI-generated content on the academic ecosystem and responses—Discussion and analysis based on ChatGPT[J]. Modern Educational Technology, 2023,(6):15-25.
[4]Firat M. How ChatGPT can transform autodidactic experiences and open education?[OL].
<https://doi.org/10.31219/osf.io/9ge8m>
[5]Wang Youmei, Wang Dan, Liang Weiyi, et al. “Aladdin’s Lamp” or “Pandora’s Box”: The potential and risks of ChatGPT’s educational applications[J]. Modern Distance Education Research, 2023,(2):48-56.
[6]Dai Y, Lai S, Lim C P, et al. ChatGPT and its impact on research supervision: Insights from Australian postgraduate research students[J]. Australasian Journal of Educational Technology, 2023,(4):74-88.
[7]Yiwen L, Yahui Y, Jinrong F, et al. Human-AI collaboration: A study on anti-ChatGPT strategies employed in innovative practical homework towards “one-click-answer” issue in AIGC[A]. International Conference on Computer Science and Education[C]. Singapore: Springer Nature Singapore, 2023:343-353.
[8]Wang Xia, Bian Qing, Sun Junhua. On the construction of the curriculum system for academic degree graduate students[J]. Degree and Graduate Education, 2015,(10):30-34.
[9][45]Qiu Zeqi. Cognitive Domain: From Familiarity to Human-Machine Co-Evolution[J]. People’s Forum: Academic Frontiers, 2023,(11):12-27.
[10]Knauff M, Wolf A G. Complex cognition: The science of human reasoning, problem-solving, and decision-making[J]. Cognitive Processing, 2010,11:99-102.
[11]Anderson J R. ACT: A simple theory of complex cognition[J]. American Psychologist, 1996,(4):355.
[12]Zhang Song, Zhang Guodong, Wang Yaguang. Research on graduate students’ role cognition and value orientation—Constructing an intrinsic model of graduate education satisfaction and its attributes[J]. Degree and Graduate Education, 2016,(3):69-73.
[13]Fawns T, Aitken G, Jones D, et al. Beyond technology in online postgraduate education[J]. Postdigital Science and Education, 2022,(2):557-572.
[14]Jiang Yingzhou. The tension in cultivating graduate students’ abilities and teaching—Based on a survey of political science graduate students from five institutions[J]. Journal of the National Academy of Education Administration, 2016,(9):78-83.
[15]Zhong Baichang, Gong Jiaxin. Evaluation of students’ innovation abilities: Core elements, issues, and prospects—A systematic review based on papers from core Chinese journals[J]. China Distance Education, 2022,(9):34-43,68.
[16]Liao Xiangyang, Zhu Huifang. Reconstruction of graduate students’ ability cultivation in the era of artificial intelligence—Reflections based on the Moravec Paradox[J]. Degree and Graduate Education, 2022,(8):13-20.
[17][37]Du Yanyan, Niu Junming. Reconstruction and mechanism innovation of the curriculum system for academic graduate students in education[J]. Educational Theory and Practice, 2022,(33):48-53.
[18]Zhong Baichang, Gong Jiaxin. Construction and empirical research of evaluation index system for interdisciplinary innovation capabilities[J]. China Educational Technology, 2022,(12):27-34.
[19]Wang Hongcai, Zhang Qifu. ChatGPT will open a new accelerated paradigm for the development of higher education[J]. Research on Educational Development, 2023,(11):29-33.
[20]Luo Yaocheng. On the three dimensions of integrating graduate course learning and research training—Insights from a survey of 30 newly appointed doctoral young teachers[J]. Degree and Graduate Education, 2010,(11):57-61.
[21][24]Baidoo-Anu D, Owusu Ansah L. Education in the era of generative artificial intelligence (AI): Understanding the potential benefits of ChatGPT in promoting teaching and learning[J]. Journal of AI, 2023,(1):52-62.
[22]Tan K, Pang T, Fan C. Towards applying powerful large AI models in classroom teaching: Opportunities, challenges and prospects[OL].
[23]Dergaa I, Chamari K, Zmijewski P, et al. From human writing to artificial intelligence generated text: Examining the prospects and potential threats of ChatGPT in academic writing[J]. Biology of Sport, 2023,(2):615-622.
[25]Wu Qing, Liu Yuwen. Higher education responses in the ChatGPT era: Prohibition or reform?[J]. Higher Education Management, 2023,(3):32-41.
[26]Rudolph J, Tan S, Tan S. ChatGPT: Bullshit spewer or the end of traditional assessments in higher education?[J]. Journal of Applied Learning and Teaching, 2023,(1):342-362.
[27]Ruan Yifan, Wang Zhibo. Ethical risks of ChatGPT in ideological and political education and response strategies[J]. Research on Core Socialist Values, 2023,(3):50-58.
[28]Feinstein N. Salvaging science literacy[J]. Science Education, 2011,(1):168-185.
[29]Liu S, Liu C, Samarapungavan A, et al. A framework for evidentiary reasoning in biology: Insights from laboratory courses focused on evolutionary tree-thinking[J]. Science & Education, 2023,(2):1-32.
[30]Britt M A, Rouet J F, Blaum D, et al. A reasoned approach to dealing with fake news[J]. Policy Insights from the Behavioral and Brain Sciences, 2019,(1):94-101.
[31]Duncan R G. The role of domain-specific knowledge in generative reasoning about complicated multileveled phenomena[J]. Cognition and Instruction, 2007,(4):271-336.
[32][33]Duncan R G, Chinn C A, Barzilai S. Grasp of evidence: Problematizing and expanding the next generation science standards’ conceptualization of evidence[J]. Journal of Research in Science Teaching, 2018,(7):907-937.
[34]Kim W J, Alonzo A C. Undergraduates’ grasp of evidence for evaluating scientific knowledge claims associated with socioscientific issues[A]. ESERA 2019[C]. Bologna: Springer International Publishing, 2021:149-160.
[35]Duncan R G, Cavera V L, Chinn C A. The role of evidence evaluation in critical thinking: Fostering epistemic vigilance[A]. Critical Thinking in Biology and Environmental Education.Contributions from Biology Education Research[C].Cham: Springer International Publishing, 2022: 75-92.
[36]Zhao Qinp, Ma Yonghong, Bie Dunrong, et al. Graduate education and research in the new era (Discussion)[J]. Degree and Graduate Education, 2022,(10):1-11.
[38]Wei Jinyang, Lu Xianliang. The impact of developmental feedback from supervisors on graduate students’ proactive innovation behavior[J]. Heilongjiang Higher Education Research, 2021,(11):124-129.
[39]Wang Xia. The connective design of graduate courses: Principles, experiences, and strategies[J]. Research on Graduate Education, 2019,(3):22-28.
[40]Zhu Zhitian, Dai Ling, Hu Jiao. High-awareness generative learning: Innovation of the learning paradigm empowered by AIGC technology[J]. Research on Educational Technology, 2023,(6):5-14.
[41]Wang Fan, Zhang Di, Liu Hui, et al. Analysis of the “spiral” cycle cultivation and application of online learning experience[J]. Research on Educational Technology, 2021,(9):62-68,83.
[42]White J, Fu Q, Hays S, et al. A prompt pattern catalog to enhance prompt engineering with ChatGPT[OL].
<https://arxiv.org/pdf/2302.11382.pdf>
[43]Wang Li, Li Yan, Chen Xinya, et al. Evaluation and feedback of student argumentation content supported by ChatGPT—An empirical comparison based on two question designs[J]. Modern Distance Education Research, 2023,(4):83-91.
[44]Woo D J, Guo K, Susanto H. Cases of EFL secondary students’ prompt engineering pathways to complete a writing task with ChatGPT[OL]. <https://arxiv.org/ftp/arxiv/papers/2307/2307.05493.pdf>
[46]Yang Xin. The educational transformation scenario based on generative artificial intelligence—What does ChatGPT mean for education?[J]. China Educational Technology, 2023,(5):1-8,14.
Article cited from: Xing Yao, Wang Fan, Li Wenjie.AIGC Environment and the Generation Evolution of Graduate Students’ Complex Cognition—Based on ChatGPT’s “Evidentiary Reasoning”[J]. Modern Educational Technology, 2024,(4):47-59.
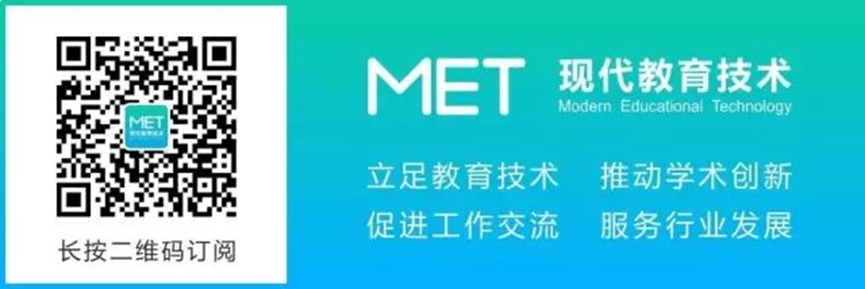