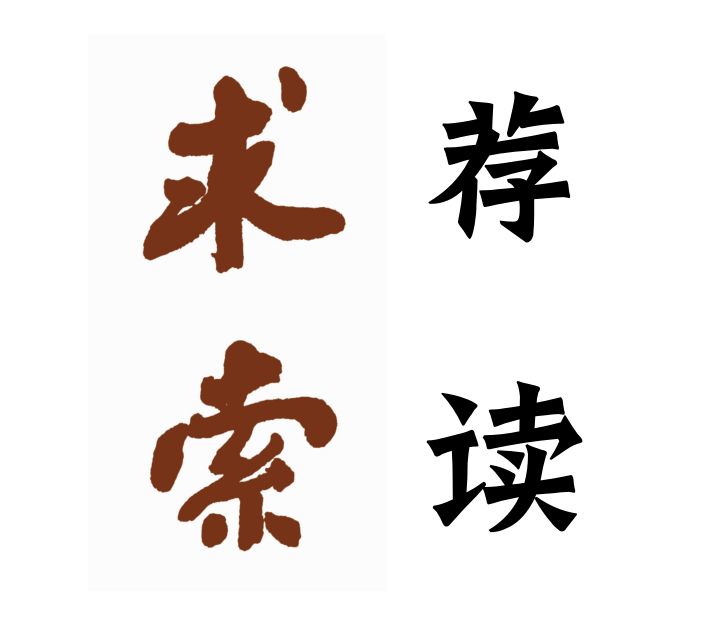
Ouyang Rihui, Researcher and PhD Supervisor at the China Internet Economy Research Institute, Central University of Finance and Economics; Special Researcher at the Beijing Xi Jinping Thought on Socialism with Chinese Characteristics for a New Era Research Center.
Abstract: Generative Artificial Intelligence, as a new production method, possesses technical-economic characteristics such as complementarity, intelligence, integration, and creativity. Generative AI permeates all aspects of financial products, business models, and business processes, forming empowering effects, economies of scale, economies of scope, and flywheel effects, resulting in cost reduction, efficiency enhancement, value creation, and innovation in business formats. The deep integration of generative AI with the financial industry is based on technology, centered around data, supported by computing power, driven by algorithms, and guaranteed by rules, providing certain auxiliary value to financial business, but it cannot completely overturn the traditional paradigm of the financial industry. The application of generative AI in the financial field faces many problems and challenges, and it is necessary to enhance the adaptability between AI technology and the financial industry.
Keywords: Generative Artificial Intelligence; Large Language Models; Technical-Economic Paradigm; Financial Institutions
Source of Original Text: Ouyang Rihui. Generative Artificial Intelligence and Its Deep Integration with the Financial Industry: Theoretical Mechanism and Development Path [J] Inquiry, 2024(1):57-65.
Large Language Models (LLMs) and Generative Artificial Intelligence (GenAI) are among the latest and most important technological advancements today. With breakthroughs in generative AI applications represented by ChatGPT, Midjourney, and Microsoft Copilot, generative AI is rapidly integrating with industries such as healthcare, education, manufacturing, and finance. Among them, the combination of generative AI and the financial industry is particularly noteworthy. The financial sector has advantages in data and capital, and leading players have begun to introduce generative AI into business scenarios, accelerating the application process of large models in the financial industry. For example, Agricultural Bank of China launched ChatABC, and Postal Savings Bank, Industrial Bank, and others actively connected to the “Wenxin Yiyan” large model platform; and Ma Shang Consumer Finance Company released the “Tianjing” large model.
The academic discussion on the development of artificial intelligence in the financial sector mainly focuses on three aspects: First, demonstrating the role and impact mechanism of AI in the financial field, such as its role in financial asset pricing. Second, discussing the paths and scenarios of the integration of finance and AI. Third, analyzing the risks brought by AI to finance and the challenges to regulation. The emergence and rapid iteration of generative AI technology have further stimulated academic enthusiasm for researching the application of advanced AI technologies in the financial industry. However, what are the technical-economic characteristics of generative AI? In the financial sector, which emphasizes compliance, rigor, and very low tolerance for errors, what is the mechanism of generative AI empowering the digital transformation and scenario changes of the financial industry? How should financial institutions leverage the advantages of general AI technology to better serve the development of the financial industry? These questions urgently need answers from academia.
2. Generative AI and Its Technical-Economic Characteristics
Artificial intelligence refers to computer programs that mimic human abilities to perform certain “intelligent” tasks, serving as a mapping and supplement to natural human intelligence. Since the concept of “Artificial Intelligence” was proposed at the Dartmouth Conference in 1956, which discussed “how machines can mimic human intelligence”, the accelerated development of computer technology has driven progress in areas such as robotics, perception, and machine learning. More data, better models, and more powerful computers have improved predictions, enabling a new generation of systems to rival or even surpass human capabilities. In the early 21st century, research in AI technology has made significant progress and breakthroughs. Matt Taddy separated the concept of machine learning from artificial intelligence, proposing that AI systems are self-training structures of machine learning predictors that automate and accelerate human tasks, consisting of three main parts: domain structure, data generation, and general machine learning.
(1) Conceptual Clarification of Generative AI
Currently, existing literature translates both Generative Artificial Intelligence (GenAI) and Artificial Intelligence Generated Content (AIGC) as “generative artificial intelligence.” ChatGPT has broken the existing human-computer interaction model, raising widespread attention to the AIGC technology that supports ChatGPT. The narrow definition of AIGC is a production method that uses AI to automatically generate content through a large amount of training data and generative algorithm models, automatically producing various forms of content such as text, images, music, video, and 3D interactive content. The broad definition of AIGC also includes concepts such as strategy generation and virtual humans. ChatGPT, as a chat-type generative pre-trained transformer model, is an important product application of AI-generated content (AIGC). ChatGPT can start from a “prompt” to generate “text similar to its training data”; the text generation of ChatGPT is a “reasonable continuation of text”, which is essentially “selecting the next word based on probability”. Large language models can generate stories and news articles, translate between different languages and writing styles, and extract information for tasks such as sentiment analysis. AIGC technology, represented by large language models and image generation models, has become a new generation of platform technologies in artificial intelligence.
GenAI and AIGC are distinct. First, generative AI (GenAI) is a specific technology and currently the most mainstream method for AIGC, but it is not the only method for achieving AIGC. Second, AIGC, or AI-generated content, is the most important development direction of GenAI. AIGC is in the same dimension as UGC (user-generated content) and PGC (professionally generated content). GenAI has certain logical reasoning capabilities, allowing it to automatically decompose tasks based on summarization and induction. This means that machines are generating new things, rather than analyzing existing ones. Third, GenAI presents two trends. One is the integration of GenAI models with other widely used software tools or “plugins”. Some of these tools will be used to enhance the overall performance and accuracy of the models, for example, through external information retrieval via search engines. The second is that large software systems call multiple GenAI models. For example, the first system may prompt a specialized GenAI model to perform a specific task (such as generating a website coding plan), and the second GenAI model will critique that plan (such as checking whether the code has cybersecurity vulnerabilities). The “Interim Measures for the Management of Generative AI Services” jointly issued by the National Internet Information Office and seven other departments believes that “generative AI technology refers to models and related technologies that have the ability to generate text, images, audio, video, and other content.”
In summary, the generative artificial intelligence discussed in this article corresponds to the English term “Generative Artificial Intelligence”, which refers to a new production method that automatically generates text, images, sound, video, code, and other content based on machine learning and artificial intelligence technologies through algorithms, models, data, and rules. This definition has the following connotations: First, generative artificial intelligence is called “generative” because it creates new content, rather than merely imitating, classifying, or repetitively applying existing data. Second, generative artificial intelligence encompasses many AI technologies. Third, generative artificial intelligence is a collective term for any automated process that generates, operates, or synthesizes data using algorithms, typically presented in the form of images or human-readable text. Based on deep learning, particularly neural network technology, generative artificial intelligence can learn the distribution and patterns of data by analyzing large amounts of training data, and then use these learned patterns to generate new data. Fourth, generative artificial intelligence is a new production method. The rise of machines is profoundly changing human production methods, and the phenomenon of “machine anthropomorphism” has become a significant feature of the development from mechanical machines to intelligent machines.
(2) Technical-Economic Characteristics of Generative AI
Every technological revolution inevitably has a profound impact on economic and social changes. The technical-economic paradigm proposed by Peles in “The Dynamics of Technological Revolution and Financial Capital: Bubbles and Golden Ages” is a suitable tool for analyzing the impact of generative AI on economic and social factors. As a new production method, generative AI has technical-economic characteristics such as complementarity, intelligence, integration, and creativity.
1. Complementarity. The impact of generative AI on economic society has shifted from the emphasis on substitution in decision-making AI to complementarity. On one hand, this is reflected in the empowerment of labor, where AI will continue to empower from simple tasks to complex tasks, solving complex tasks that require adaptability and agility, significantly accelerating the pace of work automation; on the other hand, it is reflected in the supplementation and enhancement of labor and other production factors, improving employee productivity, capital efficiency, and technical input efficiency. Currently, generative AI can automatically generate articles, news, stories, codes, maps, characters, create music, synthesize voices, and imitate various voice styles, providing personalized learning materials and auxiliary teaching for the education sector, accelerating innovation and discovery in drug research, molecular design, and scientific simulation, and assisting healthcare professionals in diagnoses in areas such as medical image analysis and pathology interpretation. As the capabilities of generative AI continue to improve, it will play a continuously deepening complementary role with other technical, capital, and labor factors, constantly strengthening the support role of technology in economic and social development.
2. Intelligence. The core issue of intelligence is a mixed combination of “is it” + “should it” + “is it good”. Among them, “is it” belongs to objective factual logical computation problems, “should it” belongs to subjective value judgment computation problems, and “is it good” belongs to mixed decision-making computation problems. Intelligence is not a simple extension or expansion of information, digitization, or automation, but a new paradigm that is significantly different from the latter three. Therefore, intelligence must not only master known information and learn existing knowledge, but also generate valuable information and knowledge and effectively use and coordinate these information and knowledge. The new generation of artificial intelligence studies situational awareness models under human-machine hybrid conditions for complex, multi-domain, dynamic environments, exploring the impact of human-machine-environment on decision-making; further constructing computer models driven by a mixture of rational and emotional factors to achieve hybrid intelligent decision-making between humans and machines. With the development of technology, AI systems may possess the ability to abstract symbolic knowledge from interactions with the natural environment, enhancing human cognitive abilities, enabling them to make wiser and faster decisions, and the future world will be more intelligent, personalized, and efficient.
3. Integration. Existing literature emphasizes the penetration and synergy of artificial intelligence as a general technology, meaning that AI penetrates various industries through application scenarios, enhancing the matching efficiency and compatibility of capital, labor, and technology, promoting economic efficiency. On this basis, generative AI exhibits characteristics of integration. On one hand, generative AI is a fusion and integrated application of multiple technologies. The plugin form is a good entry point for generative AI applications, integrating with existing software ecosystem in a plugin form. On the other hand, generative AI deeply integrates with industry users. At the micro level, the best generative AI companies can generate sustainable competitive advantages through the flywheel formed between user stickiness, data, and model performance. At the meso and macro levels, generative AI empowers traditional industries, promoting the integrated development of different industries.
4. Creativity. Artificial intelligence has the creative characteristic of substituting human intellectual work, capable of producing additional knowledge and increasing the total wisdom of humanity. Using generative AI tools can automate traditional coding or accelerate the time of scientific research and development, greatly enhancing the versatility of AI systems. On one hand, generative AI can not only replace some high-intensity, high-difficulty labor, raising expectations for the proportion of automated work, but also “activate” potential related industries, creating numerous emerging business formats. For example, instant retail, supported by AI technology, has “activated” the model of calling merchants for ordering and delivery, growing into a trillion-scale giant market. On the other hand, the popularization of generative AI will promote related innovations in the digital transformation of digital technology and traditional industries, facilitate the integration of the digital economy and the real economy, and open up new economic growth spaces. In fields requiring a large amount of repetitive labor and extensive trial and error, such as industrial equipment, chip manufacturing, and biomedicine, generative AI explores possible combinations of knowledge and elements within the field at a lower cost, driving technological progress in the field through “combinatorial innovation”.
3. The Mechanism of Deep Integration of Generative AI with the Financial Industry
Online, networked, and intelligent are the main themes of the financial industry’s development. The essence of the problems solved by artificial intelligence is decision-making efficiency and cost savings, which has become an important technology for the digital transformation of the financial sector. After experiencing several stages of expert models, machine learning models, and deep learning, generative AI has achieved fundamental breakthroughs in the capabilities of “dialogue” and “creation” compared to traditional artificial intelligence. Generative AI technologies represented by ChatGPT will profoundly impact the business ecology of the financial industry. The technical-economic characteristics exhibited by generative AI form the foundation for its deep integration with the financial industry. In this integration process, the functions of complementarity, intelligence, integration, and creativity of generative AI will be fully utilized, which is reflected not only in replacing human labor, encoding industry knowledge, integrating multi-dimensional data, discovering potential knowledge, and software-defined products but also in creating elements, especially data elements, enhancing the intelligence level of financial business, and promoting innovation in financial business formats and models through “combinatorial innovation”.
(1) Complementarity and Empowerment Effect
Artificial intelligence has both substitutive and complementary aspects. At the beginning of its development, artificial intelligence raised concerns about issues such as “machine replacing humans”, mainly substituting for programmed and some non-programmed tasks, such as handling, driving, and image diagnosis. In fact, from the perspective of value creation logic, generative AI in the financial industry will produce two effects: “replacing humans” and “empowering humans”. The “replacing humans” effect is reflected in positions with mechanical and repetitive work content gradually being replaced by AI to free up operational human resources. For example, a financial contract analysis software developed by JPMorgan Chase, COIN, can complete in seconds what previously took lawyers and loan officers 360,000 hours annually. The “empowering humans” effect is reflected in leveraging the “dialogue” and “creation” capabilities of generative AI to amplify the productivity of key human nodes, empowering the formation of “professional” content and “basic management” aspects, thereby improving work efficiency. For instance, AIGC empowers investment advisors and financial managers, enhancing their level of professionalism. Therefore, the mainstream positions in the financial industry will be human-machine collaboration, where generative AI shifts from “replacing humans” to a more rational empowerment of humans to “increase efficiency”, transitioning from “serving external customers” to “empowering internal employees”, enhancing the empowerment effect generated by complementarity.
(2) Intelligence and Economies of Scale Effect
The application of artificial intelligence in the financial industry has undergone a process from penetration to integration. Financial institutions utilize internet technology to connect financial products, services, and customers, promoting the standardization, automation, and intelligence of financial activities. On one hand, artificial intelligence enhances data processing efficiency and resource allocation efficiency, reducing the operational costs of financial institutions. Machines replace humans in data processing, providing various financial products or services in an automated manner, achieving online and networked operations for identity verification, credit, credit assessment, investment advice, and other business processes, promoting the intelligence of financial institutions. For example, research has found that fintech innovation has improved the efficiency of the U.S. mortgage market. Under the same loan risk, utilizing artificial intelligence technology can process mortgages faster and respond more flexibly to market demands, reducing friction in the refinancing market. The total days from application to issuance are on average reduced by about 10 days compared to traditional institutions, with efficiency improving by approximately 20%, and no “selection effect” found for specific borrowers. In practice, the application of artificial intelligence in marketing, product design, risk control, and customer service has realized smart marketing, smart identification, smart investment advice, smart risk control, and smart customer service, enhancing the efficiency and capability of serving the real economy. For instance, users can actively search and interact with generative AI to obtain answers, rather than passively receiving information pushes, significantly improving the efficiency of obtaining information.
On the other hand, artificial intelligence improves customer service efficiency and expands the business scale of financial institutions. The gradual penetration of AI has made the connections between different financial entities closer, changing the interaction methods between financial industries and enterprises, stakeholders, and consumer groups. This not only addresses issues faced by financial institutions such as supply shortages, high thresholds, information asymmetry, and difficulties in risk assessment but also creates market demand through service innovations like intelligent consulting, intelligent lending, and intelligent customer service. For example, smart marketing collects a large amount of data on customer consumption preferences, social behaviors, and transaction characteristics to predict customer needs and preferences, further customizing content and conducting marketing activities to achieve higher marketing effectiveness and customer satisfaction. Credit evaluation systems established using intelligent technologies can accurately identify and assess the risks of target customers, increasing the opportunities for vulnerable groups to obtain credit. With the continuous innovation of AI technology capabilities, the application of financial AI will no longer be limited to a single application scenario but will connect the entire service value chain, empowering traditional financial institutions with platform-type products. In recent years, Chinese financial institutions have actively explored digital transformation, and in the future, generative AI will empower comprehensive, all-encompassing, and around-the-clock marketing, more efficiently aggregating the scattered funds of long-tail customer groups, developing more personalized, customized, and differentiated financial products and services, forming economies of scale effects.
(3) Integration and Economies of Scope Effect
The next generation of artificial intelligence urgently needs to strengthen the combination capabilities of knowledge (including tacit knowledge), computation, and reasoning, promoting human society into an intelligent era of human-machine collaboration, cross-border integration, and co-creation and sharing. ChatGPT reveals the importance of new generation collaborative and interactive intelligence. On one hand, using generative AI technology, through multimodal approaches, provides financial users with a richer and more convenient interactive experience. Collaboration and interaction are effective paths to enhance AI’s capabilities in knowledge, computation, reasoning, and combination. Human-machine collaboration forms a team of humans and machines, integrating human intelligence and artificial intelligence, promoting autonomous interaction and win-win cooperation between humans and machines. ChatGPT’s natural language human-machine interaction and deep learning not only strengthen the connection between humans and machines but also promote the collaborative evolution of different elements. As a prototype of human-machine collaborative co-creation and interaction paradigms, ChatGPT may evolve into multimodal in the future, bringing new opportunities for multimodal learning. Generative AI improves human-machine interaction experiences, such as intelligent assistants and virtual characters, by generating personalized responses and services through interactions with users. Intelligent interaction can be applied in various scenarios such as financial marketing, financial education, and financial entertainment, greatly enhancing user participation and loyalty, increasing user stickiness and revenue. This collaborative effect encourages generative AI to make professionals more specialized, especially those with ideas, critical thinking, and professional financial skills will be better empowered by generative AI. On the other hand, generative AI reshapes the value chain of the financial industry, enhancing user experience. Large models have stronger learning and generalization capabilities, generating more accurate, fluent, and coherent content, producing more diverse and innovative content, and handling more complex and large-scale generative tasks, such as natural language processing and image generation. Generative AI can not only automatically profile user consumption habits and demands but also adjust and optimize the generated content in real-time based on user behavior and feedback, achieving intelligent matching of personalized needs and professional supply, providing personalized services, enriching user experience and satisfaction, thus achieving efficiency improvements in the financial sector through economies of scope effects. For example, generative AI can utilize conditional generative networks, text generation, and image generation technologies to simulate different insurance scenarios and generate personalized insurance plans suitable for consumers, enhancing customer experience and satisfaction.
(4) Creativity and Flywheel Effect
The core capability of generative AI lies in creating new content, with application scenarios shifting from blue-collar repetitive labor (quality inspection, customer service, etc.) to white-collar knowledge innovation. Undoubtedly, this will bring greater value space, while also potentially generating more flywheel effects. What is the flywheel effect? It refers to the ability of AI to help white-collar and knowledge workers better understand, gain insights, and form new knowledge. New knowledge will help design better AI, and better AI will generate more new knowledge. As data continues to accumulate and models are continuously trained, the intelligence level of the models continuously improves, thus generating greater value in business. In other words, the core of generative AI is the interaction between data, models, and business, forming a flywheel effect. In the short term, the commercial value of generative AI is enhancing efficiency. In the medium to long term, the value of generative AI lies in changing the way financial institutions generate and use data insights, achieving breakthroughs in traditional financial formats and models, reconstructing financial business models, and enhancing the innovation capability of financial institutions. Depending on different needs and scenarios, generating diverse and personalized content to meet customers’ diverse needs enhances the innovation and competitiveness of financial products and services. For instance, the general application of AI in the financial field has broken traditional business models in payments, credit, and insurance, giving rise to new financial formats and business models such as digital payments, digital assets, digital credit, and digital insurance.
4. Conditions and Scenarios for the Deep Integration of Generative AI with the Financial Industry
International financial institutions have two attitudes toward the application of generative AI in the financial field. Major banks such as Bank of America, Citigroup, and Goldman Sachs have restricted employee use of ChatGPT due to concerns about data leaks. Some financial institutions are actively exploring and attempting to use generative AI. For example, Morgan Stanley uses an OpenAI-driven chatbot to assist financial advisors, utilizing the company’s internal research and data repository as knowledge resources. Jiangsu Bank is trying to apply ChatGPT in daily office work, risk assessment, customer service, and auxiliary marketing. Overall, financial institutions are cautious yet optimistic about the application of generative AI technology, adhering to a principle of doing what they should while avoiding what they should not, continuously accumulating experience in application scenarios.
(1) Conditions for Deep Integration
The deep integration of generative AI with the financial industry requires support from the maturity of generative AI technology and the improvement of digital infrastructure, driving business innovation through algorithms under the protection of data and its regulations.
1. Based on Technology. AI technology is a general and leading technology of the Fourth Industrial Revolution, propelling humanity into the intelligent era. Gartner, a U.S. information technology research and analysis company, has given generative AI a very high rating, considering it the most widely impactful technology in the past decade. According to its analysis, over 55% of AI technologies will enter production highlands in the next five years, and 93% will reach peak production in the next ten years, with only AGI and neural-symbolic AI expected to take more than ten years. The popularity of ChatGPT, Midjourney, Stable Diffusion, and large language models has accelerated the development of generative AI, leading most end-user enterprises across industries to explore generative AI. Technology providers are also forming teams to prioritize the delivery of generative AI applications and tools. Most technology products and services will integrate generative AI capabilities within the next year; generative AI will rapidly develop in vertical industry fields, scientific discoveries, and technology commercialization. Factors driving generative AI include: enthusiastic commercial hype; the emergence of new foundational models and model versions, sizes, and capabilities; improvements in generative models and reduced training data requirements; creative work achieved in the content field; and high expectations for generative AI in software coding.
2. Data-Centric. Generative AI is supported by machine learning models, learning from large amounts of data to understand and create new content. Generative large-scale language models are based on generative pre-trained transformers, learning implicit language rules and patterns from terabytes of training data through generative self-supervised learning, training large-scale language models with hundreds of billions of parameters. Therefore, the integration of generative AI with the financial industry revolves around data as the core, mainly reflected in the following three aspects: First, user identification and profiling. By collecting a large amount of user data on consumption preferences, social behaviors, and transaction characteristics, predicting customer needs and preferences. Second, risk control. By analyzing large amounts of data, including user characteristics, transaction characteristics, and market trends, constructing precise consumer risk models that can automatically identify potential risks, assess risks, predict possible risk events, and take corresponding measures to reduce the impact of risks on business and funds. Third, decision-making revolution. Currently, financial institutions mostly adopt retrospective and indirect decision-making. In the context of large models, financial institutions may conduct real-time decision-making, allowing business personnel to directly face the diverse needs of consumers, constructing more humanized financial products with better user experiences, and timely adjusting transaction processes.
3. Supported by Computing Power. The model training and inference processes for applying artificial intelligence in the financial sector require strong computational power support, including AI chips, AI platforms, intelligent computing centers, etc., providing computational ability, storage capacity, network communication capability, and large-scale computing coordination management capabilities. Without computing power, AI cannot achieve complex calculations and iterations. Providing high-performance, low-cost, and green computing capabilities is an important support for the deep integration of AI and finance. The computing power and related supporting facilities determine the maximum height of financial matching that AI can achieve. In recent years, China has released several policies pointing out directions for the construction of AI computing power. In October 2023, the Ministry of Industry and Information Technology of the People’s Republic of China, along with the People’s Bank of China and other six departments, jointly issued the “Action Plan for the High-Quality Development of Computing Power Infrastructure”, proposing quantitative indicators for the development of computing power infrastructure by 2025 in terms of computing power, carrying capacity, storage capacity, and application empowerment. In terms of computing power, the scale will exceed 300 EFLOPS, with intelligent computing power accounting for 35%; the national hub node data center clusters will basically achieve direct network transmission with a theoretical delay of no more than 1.5 times; in terms of storage capacity, the total storage will exceed 1800EB, with advanced storage capacity accounting for over 30%; in terms of application empowerment, a batch of new businesses, models, and formats for computing power will be created, significantly increasing the penetration of computing power in fields such as industry and finance.
4. Driven by Algorithms. Algorithms are the specific methods for solving problems in financial technology, and the core driving force for the future deep integration of generative AI with finance will mainly rely on breakthroughs and innovations at the algorithmic level. From the perspective of algorithm application, the deep integration of AI and finance is supported by a series of diverse algorithm combinations, including decision tree algorithms (XGBoost, GBDT, etc.), natural language processing algorithms (BERT), and graph neural network algorithms (GCN, CNN), etc. Financial institutions need to select appropriate model architectures and parameters based on their generative tasks and data characteristics, considering factors such as model performance, efficiency, stability, interpretability, compatibility, scalability, and security. Algorithms and data are the root sources of the logic of AI development, and they can also easily lead to risks, mainly including algorithm security, interpretability, and decision-making risks. For example, in AI application scenarios such as intelligent risk control, intelligent investment advice, and intelligent marketing, financial institutions can make decisions based on automated decision-making methods like algorithms, such as determining personal credit and loan limits based on user profiles. However, complex conflicts of interest and the black-box nature of investment advice algorithms bring vulnerabilities to user information security. Financial institutions need to pay special attention to three key risks when applying generative AI: model hallucination, malicious use, and information leakage.
5. Guaranteed by Rules. Generative AI will bring new shocks and challenges, requiring large datasets during training, necessitating the establishment of compliance requirements for data collection, use, and disclosure. It is essential to develop AI models that can assess bias and achieve healthy fairness. Therefore, the industry needs to enhance the application of technologies such as privacy computing and establish relevant rules to ensure the compliant development of generative AI. Addressing potential risk issues such as algorithm black boxes, algorithm homogeneity, and model defects, the People’s Bank of China issued the “Evaluation Norms for Financial Applications of Artificial Intelligence Algorithms (JR/T0221-2021)” in 2021, systematically proposing basic requirements, evaluation methods, and determination criteria from aspects of security, interpretability, accuracy, and performance, providing guidance for financial institutions to strengthen risk management in intelligent algorithm applications. In July 2023, the National Internet Information Office and other ministries announced the “Interim Measures for the Management of Generative AI Services”, which integrates data security and privacy protection regulations throughout the processes of data labeling, model training, pre-training, and service provision.
(2) Scenarios for Deep Integration
Generative AI is a new generation of productivity tools, especially in the financial field, generating different styles of text, voice, video, and generating content similar to financial asset targets, which is its most basic application. At the same time, generative AI has certain auxiliary value in the practical implementation of financial business. From the perspective of the generation process and results, it also carries a creative nature. Before the rise of large language models, natural language processing (NLP) had already seen some application scenarios in finance. These application scenarios will also be the main application scenarios for large language models in finance, including: querying information and retrieving documents; parsing documents such as policy documents, news reports, company announcements, earnings conference calls, research reports, and chat records, extracting knowledge to improve investment research efficiency and breadth; market sentiment analysis; draft generation and document review; financial literacy education; executing operations based on user instructions; event-driven strategies; and more.
In practice, the breadth of application scenarios for artificial intelligence in financial business continues to expand. Commercial banks are continually enriching their applications in intelligent marketing, intelligent identification, intelligent investment advice, intelligent risk control, and intelligent customer service. For example, in the application case of intelligent marketing, the Industrial and Commercial Bank of China is piloting RPA technology to empower intelligent marketing, and Tencent Cloud has launched the Tencent Financial Intelligent Marketing Platform. ChatGPT has demonstrated its value in the stock market, risk management, financial literacy, and academic research, with several applications already in place. For instance, in the stock market, tools like ChatGPT can be widely used to analyze the impact of news on stock prices, interpret policy statements, and assist in investment decision-making, helping predict market changes and risk factors.
The integration of generative AI and the financial industry has just begun. Future directions worth paying attention to include: First, financial institutions should clarify three roles in the large-scale application of generative AI: creators, driving core business model transformation, reshaping core business and/or customer-facing methods, such as mortgage assessments and direct customer interactions; shapers, focusing on behind-the-scenes integration, changing applications that alter business models, such as virtual experts and frontline training; and users, specifically enhancing productivity using SaaS solutions, improving the efficiency or accuracy of existing tasks, such as coding assistance, copywriting, and customer assistance. Second, the application of financial AI will connect the service value chain, making services platform-oriented. The application of financial AI will no longer be limited to single application scenarios but will connect the entire service value chain, empowering traditional financial institutions with platform-type products. Third, breakthroughs in human-machine interaction will enhance user experience, building an “intelligent customer service” system represented by automated services and a scene financial service system represented by “open banking”. Commercial banks will use generative AI to achieve multi-scenario, multi-level channel network coverage online and offline, enhancing personalized service capabilities and data integration processing abilities, promoting channel construction, and cultivating a new financial ecosystem.
(3) Generative AI Cannot Completely Overturn the Traditional Paradigm of the Financial Industry
The financial industry is characterized by resource intensity, human capital intensity, and strong regulation and oversight. On one hand, the financial industry is data, information, and knowledge-intensive, and generative AI can enhance the efficiency of financial services. On the other hand, the financial industry has certain entry barriers, requiring compliance, licensing, and capital requirements, with strict demands for real, accurate, and complete statements, and high requirements for data timeliness. False records, misleading statements, or significant omissions will face severe penalties. Large language models have certain “black box characteristics”, while the financial sector’s requirements for interpretability, auditability, and accountability will also limit the application of large language models in some financial scenarios. These characteristics determine that generative AI cannot completely overturn the traditional paradigm of the financial industry but can only empower the financial industry in multiple domains. For example, many financial services need to be satisfied and delivered offline, and the personalized demands of high-net-worth individual clients are complex and difficult to standardize, with high demands for emotional value and human services. In the fields of investment and asset allocation, generative AI cannot replace real financial advisors in making final decisions, as users prefer to follow the investment advice of real financial advisors. Therefore, Morgan Stanley, with the support of OpenAI, launched a generative AI product primarily for querying professional financial data, summarizing conversations between financial advisors and clients, and drafting emails, with the goal of assisting financial advisors in improving work efficiency and decision-making accuracy.
5. Conclusion and Recommendations
The deep integration of generative AI with the financial industry will greatly enhance the scope of automation and intelligence in the financial sector, effectively improving productivity, reducing costs, promoting business innovation and growth, generating economies of scale and scope effects, and significantly improving the service quality and operational efficiency of financial institutions. Currently, the application of generative AI in the financial field is still in its early stages, facing many difficulties and challenges. Therefore, it is necessary to enhance the adaptability between artificial intelligence technology and the financial industry.
First, increase investment in algorithm research and development, strengthen the development of professional models and intelligent models, enhancing the applicability of financial large models. Allocate R&D funds to all aspects of “intelligent finance”, from the construction of financial vertical large models to the application of large models in specific financial scenarios, such as information management, intelligent recommendations, and segmented field Q&A, gradually forming a systematic development chain of “algorithm R&D – segmented fields – scenario applications” to fully leverage the complementarity, intelligence, integration, and creativity of technology and explore the deep value of generative AI in the financial industry.
Second, focus on data reserves and security issues, improving the quality of financial institutions’ use of AI generative services. On one hand, promote data aggregation, sharing, and integrated applications, constructing scientific data knowledge resource bases and foundational datasets to support the training of industry and field AI large models; on the other hand, pay attention to the security of AI service data sources, strengthen the construction of data security disaster recovery systems, and promote the formation of disaster recovery prevention and control norms, quickly organizing revisions and improvements to relevant technical standards and regulatory frameworks for data disaster recovery.
Third, adhere to a human-centric approach, guarding against over-reliance on artificial intelligence. Focus on technological finance, green finance, inclusive finance, pension finance, digital finance, and other formats, using generative AI to promote business model innovation, emphasizing the transition of financial service management from experience-based decision-making to data-driven decision-making. The correct attitude for financial institutions developing generative AI is to leverage the advantages of AI while rationally recognizing its limitations, viewing AI as a supplement to human capabilities, helping financial institutions understand consumer behavior in new ways. Over-reliance on AI may weaken human thinking, practical abilities, and decision-making capabilities.
(Thanks to Dr. Jiang Ning, Chief Technology Officer of Ma Shang Consumer Finance, for his contributions to this article.)
Responsibility Editorial: Liu Xianfeng
The specific content is subject to the printed version of the journal.
Due to formatting needs, annotations are omitted; please refer to the original text in the printed version of the journal.
For reprints authorized by the public account, please contact [email protected].
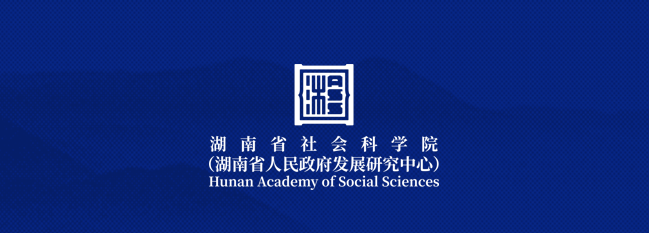
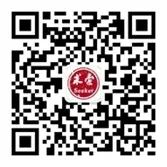
Journal Name: Inquiry
Supervised and Sponsored by: Hunan Academy of Social Sciences
(Hunan Provincial Development Research Center)
Edited and Published by: Inquiry Editorial Department
Address: 37 Liuhe Village Lane, Kaifu District, Changsha City
Postal Code: 410003
Chairman of the Editorial Board: Zhong Jun
Phone: 0731-84219107
Online Editing System: qszz.cbpt.cnki.net
Serious Statement: This journal does not charge fees in any form. Submissions must be made through the online editing system; manuscripts submitted to individual editors will not be accepted. This journal has never commissioned any other organization or individual to compile articles, please do not be deceived. Once published, remuneration will be paid.