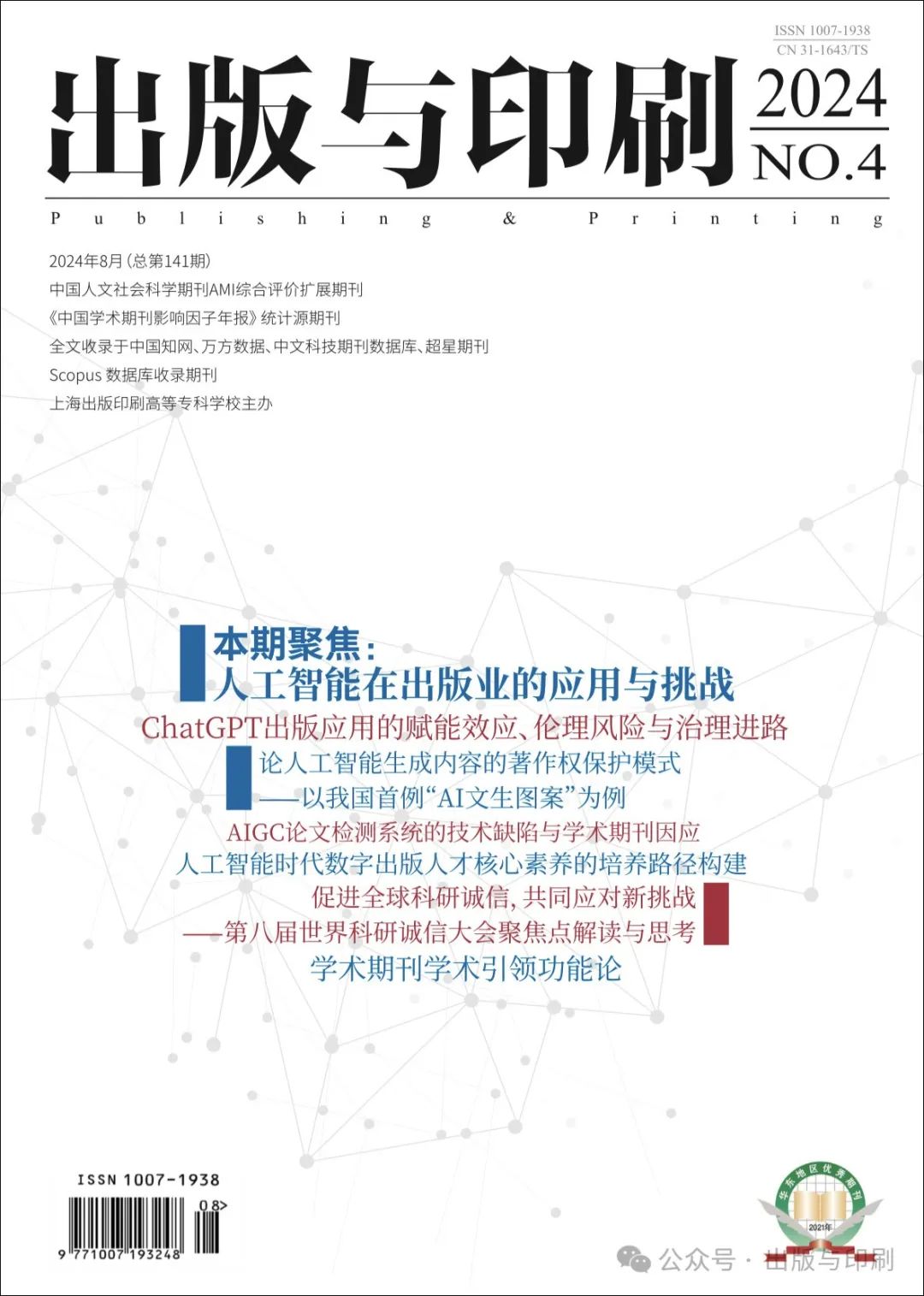
Core Viewpoints
-
The article uses the CNKI detection system and the Jianziyuan detection system to test 10 legal paper abstracts generated by ChatGPT and 10 legal paper abstracts rewritten by other AIs, to verify the AI identification capabilities of the detection tools; by analyzing the detection results, it summarizes the technical defects of the detection tools and analyzes the causes of these defects based on their technical principles; it places the defects and their causes within the publication scenarios of academic publishing units, summarizes the problems that publishers need to avoid when using AIGC detection systems, and proposes practical technical response strategies.
-
Based on empirical detection results, the current AIGC paper detection systems mainly have three technical defects: low accuracy, high discrepancy rate, and weak sensitivity. The causes are insufficient model training, improper algorithm optimization, and a lack of preset lexicons. Insufficient model training indicates that the time spent training the system model is not sufficient, the quality of the corpus is uneven, and the number of iterations achieved is far behind that of AIGC tools; improper algorithm optimization means that the detection functions designed by the system are difficult to match the vast knowledge base samples, and the newly added diverse text types and characteristics overload the computational resources, leading to slow model iterations and reduced detection efficiency; the lack of preset lexicons indicates that companies only collect and expand detection samples through the detection system, lacking human assistance in adding AIGC lexicons.
-
The improvement of the AIGC paper detection system relies on the positive interaction between R&D companies and academic journals at three levels: R&D cooperation, industry development, and editorial practice. At the level of R&D cooperation, journal units should actively provide diverse detection samples and preset lexicons to R&D companies. At the level of industry development, academic journals should not only avoid AI ghostwriting but also dialectically utilize the high quality and authentic knowledge content generated by AI. At the level of editorial practice, journal editors should play the role of human-computer relationship collaborators, guiding the optimal development of the system.
Title | Technical Defects of the AIGC Paper Detection System and Responses of Academic Journals
Source | Journal of Publishing and Printing, 2024, Issue 4
Author | Zhou Meng
Author Affiliation | Editorial Office of the Journal of Shenzhen University (Humanities & Social Sciences)
Doi | 10.19619/j.issn.1007-1938.2024.00.027
* Fund Project: Ministry of Education Humanities and Social Sciences Research Youth Fund Project “Research on the Legal Regulation of Cross-Border Data Flow in Data Trading” (Project No. 23YJC820058); National University Journal Research Society Editing Research Project “Research on the Mechanism and Path of Academic Journals Supporting the Growth of Outstanding Academic Talents from the Perspective of the Academic Community” (Project No. YB2023016).
Reference Citation Format:
Zhou Meng. Technical Defects of the AIGC Paper Detection System and Responses of Academic Journals[J]. Journal of Publishing and Printing, 2024(4):20-30.
Abstract | This paper explores the technical defects of the artificial intelligence-generated content (AIGC) paper detection system and its root causes and proposes the technical response strategies from the practical level. Using the AIGC detection service system of CNKI and the AI detector of “Jianziyuan”, this research detects 10 legal paper abstracts generated by ChatGPT and 10 similar abstracts rewritten by an AI rewriting software to verify their AIGC identification abilities. Based on the analysis of the detection results, it can be concluded that the AIGC paper detection system has three major technical defects: the low accuracy, the high difference rate and the weak sensitivity. The root causes of the defects are insufficient model training, improper algorithm optimization, and lack of preset lexicons. The study shows that the improvement of the detection system depends on the benign interaction between system research and journal development: at the R&D coordination level, academic journals should communicate and cooperate with technology providers to provide diverse test samples and preset lexicons; at the industry development level, as the ultimate goal, academic journals should avoid the academic misconduct caused by AI ghostwriting and dialectically utilize the high-quality and authentic knowledge contents generated by AI; at the editorial practice level, journal editors should play the roles of human-computer relationship collaborators and guide the optimal development of the detection system.
Keywords | AI-generated content; artificial intelligence generated content (AIGC); AI text detection; AIGC detection service system of CNKI; AI director of “Jianziyuan”; technical defect; academic journal; response strategy
→ View Full HTML
With the development and penetration of artificial intelligence-generated content (AIGC) technology in the academic publishing field, some authors have begun to use AI tools, such as ChatGPT, to write academic papers, leading to frequent occurrences of AI ghostwriting. AIGC traces can even be found in internationally renowned journals. For example, in March 2024, an article in the journal Surfaces and Interfaces published by Elsevier included a commonly generated phrase by ChatGPT—”Of course, here is an introduction that you can refer to regarding your topic”; in an article published in the top physics journal Physica Scripta in August 2023, there was a button label from ChatGPT—”Regenerate Response”. These meaningless phrases generated by AI managed to survive the scrutiny of authors, editors, reviewers, and proofreaders, indicating a lack of capability among academic publishing entities to respond to AIGC technology. In response, some scientific publishing companies have successively developed and launched AIGC paper detection systems, exploring the operational rules of AIGC and attempting to tackle technical challenges such as content recognition, algorithm efficiency, and credible assessment to identify AI-generated content in academic texts. In the practice of using AIGC paper detection systems, it is essential to explore how academic journal publishing units can be vigilant about the technical defects of the system, respond to machine risks and technical risks, and exercise subjective initiative independent of machines to construct effective AIGC recognition strategies, which is of great significance for promoting the digital and intelligent development of academic journals.
Regarding the impact of AIGC on academic journals, many scholars at home and abroad have discussed this topic. In empirical research, foreign scholars such as Catherine A. Gao and domestic scholars like Shen Xibin have pointed out that specific AI detection tools can effectively identify medical paper abstracts rewritten by ChatGPT, helping academic editors better detect AI ghostwriting behaviors. In the field of institutional research, Zhang Linghan and others believe that an AIGC quality spectrum identification system should be designed, requiring mandatory identification of generation sources, responsible entities, and content quality to activate the substantial role of the identification system in filtering information content; Jiang Xueying and others propose to construct a multi-party collaborative regulatory framework for academic publishing ecology, attempting to provide possible pathways for the healthy development of China’s academic ecology under AIGC technology. In industry research, Wang Pengtao and others analyze the coupling of AIGC technology and knowledge production, identifying the trust crisis triggered by AIGC, and exploring the theoretical model and practical path of the trust mechanism in the academic publishing industry; Zhang Zhongyi and others point out the challenges faced by the academic publishing industry, such as the difficulty in grasping the degree of AI tool usage, the difficulty of identifying hidden academic misconduct, the increased difficulty in discovering research ethics issues, and the difficulty in ensuring the authority of the current peer review system, as well as the difficulty in handling copyright and research results distribution. They propose that publishing entities should clearly understand their core advantages and positioning. In countermeasure research, Zhao Kai proposes a strategy concept of human-computer collaborative review and reshaping editorial value to meet new challenges, making AI truly a new engine for content production and review; Cao Lianyang proposes a pre-review countermeasure strategy, including updating the authors’ anti-academic misconduct commitments, using automated tools to check AIGC outside of routine plagiarism checks, verifying the sources of important viewpoints in the results, and checking the main facts and data on which academic results are based.
Although existing research has pointed out the specific risks and impacts of AIGC from multiple dimensions and angles, and provided suggestions for academic journals to respond to AI ghostwriting phenomena at the publishing policy, industry, and entity levels, it has not yet addressed the technical principles and defects of AIGC detection systems, nor clarified how publishing units can practically utilize AIGC detection technology to solve problems. Specifically, this research area still needs to be supplemented in the following three aspects: First, in empirical detection, the detection systems that can identify AI-rewritten abstracts in the medical field may not be applicable to other disciplines, especially in the humanities and social sciences, where the complexity of language logic or vocabulary diversity is greater, and for advanced AI tools that can imitate user writing styles after multiple corpus feedings, the effectiveness of AIGC detection systems may be less than satisfactory; second, regarding technical defects, current research rarely mentions the false positives and false negatives of AIGC detection systems, nor does it address the lack of universality in detection standards for different disciplines, scenarios, fields, and types of texts; third, in practical response, the existing countermeasures and institutional concepts have not yet penetrated into specific academic publishing activities, and how publishing units understand the technical principles and functions of AIGC detection systems, as well as how to combine manual review with machine detection in the review and proofreading process, remains a challenging reality. Based on these deficiencies, this article further explores on the basis of existing research: first, using two well-known domestic AIGC paper detection systems to detect 10 legal paper abstracts generated by ChatGPT and 10 legal paper abstracts rewritten by other AIs, to verify the AI identification capabilities of the detection tools; second, by analyzing the detection results, summarize the technical defects of the detection tools and analyze the causes of these defects based on their technical principles; finally, place the defects and their causes within the publication scenarios of academic publishing units, summarize the problems that publishers need to avoid when using AIGC detection systems, and propose practical technical response strategies.
1. Data Sources and Research Methods
1. Detection Sample Explanation
As the existing research in China only uses detection samples from medical literature, in order to further test the AI recognition capabilities of the AIGC paper detection system, this article selects 10 legal documents published in a certain Chinese core journal in 2023. The language logic levels and vocabulary combination difficulties of legal papers are relatively rich and complex, making it more challenging for AI tools to imitate the writing style and thinking of legal scholars, significantly increasing the difficulty of recognition testing.
The samples are divided into three groups: ① Control group, including the original text of 10 selected document abstracts, each around 400 words, with no text processing; ② AI generation group, first generating an analysis and evaluation of the original text title, abstract, and keywords by feeding these into ChatGPT, and then based on the corresponding response using the prompt “Based on this title and dialogue content, regenerate an academic paper abstract of around 500 words”, ultimately obtaining 10 AI ghostwritten samples, with no deletions or modifications; ③ AI rewriting group, using a domestic AI rewriting software that claims to reduce the AIGC suspicion level, inputting the 10 samples from the AI generation group, ultimately obtaining 10 AI rewritten samples, with the rewriting software controlling the similarity to the original AI generation group text between 65% and 80%, with no deletions or modifications.
2. Specific Detection Methods
This research does not attempt to seek strictly statistical results; it only conducts a brief data analysis of the intuitive output results of the detection system and performs specific text analysis on the samples that failed detection to seek the root causes of technical defects. Currently, AIGC paper detection systems on the market in China generally have dual functions of literature similarity detection and AIGC text proportion detection. Since previous scholars have empirically analyzed whether AI ghostwritten texts can pass literature similarity detection, and the repetition rate results are not related to the purpose of this research, this study will not conduct similarity detection on the samples.
For specific detection systems, this research selects the “CNKI AIGC Detection Service System” (referred to as the “CNKI Detection System”) from Tongfang CNKI (Beijing) Technology Co., Ltd. and the “Jianziyuan AIGC Text Detection System” (referred to as the “Jianziyuan Detection System”) from Nanjing Zhichi Shuhui Information Technology Co., Ltd. for discrimination.
3. Technical Defects Representation: Low Accuracy, High Discrepancy Rate, and Weak Sensitivity of Detection Systems
By simultaneously using the CNKI detection system and the Jianziyuan detection system on a total of 30 samples from the AI generation group, AI rewriting group, and control group, 60 detection records were generated. The data results are shown in Table 1 below.
Table 1 Output Results of AIGC Paper Detection Systems
The CNKI detection system and the Jianziyuan detection system both use the percentage of suspected AIGC fragments in the total text as detection data. Through different assignment methods, the marked detection fragments indicate the probability of belonging to AIGC. The results from the CNKI detection system are values calculated through system weighting, where the system assigns coefficients to suspected fragments of different degrees (highly suspected AIGC value is 0.9-1, moderately suspected AIGC value is 0.7-0.9, lightly suspected AIGC value is 0.5-0.7, and not marked as AIGC is 0-0.5. The left critical value is included in the corresponding range; for example, if the AIGC value is 0.5, it is lightly suspected of being AI-generated, and if the AIGC value is <0.5, the system does not mark it, determining it does not belong to AIGC). The final output is a weighted proportion of the AIGC fragments rather than the actual proportion. For example, the 6th abstract of the AI generation group was marked as moderately suspected in the CNKI detection system, but the actual number of words only accounted for 46.5% of the total text, but after evaluation by the system, it was assigned a suspicion value of 0.79, resulting in a weighted proportion of 36.7%. The results from the Jianziyuan detection system represent the actual word proportion, where the marked fragments of high, moderate, and light suspicion have probabilities of being AIGC of 70%-100%, 60%-70%, and 50%-60%, respectively; fragments with probabilities less than 50% are not identified as AIGC. The left critical value determination is the same as that of the CNKI detection system. For example, the 3rd abstract of the AI rewriting group was entirely marked by the Jianziyuan detection system as AIGC, where 41.6% of the fragments were highly suspected, and 58.4% were lightly suspected. By analyzing the data in the table, it can be preliminarily summarized that the current AIGC detection systems have three defects: low accuracy, high discrepancy rate, and weak sensitivity.
1. Low Accuracy
Overall, the detection results of the control group are all 0%, indicating that both systems have excellent identification capabilities for discovering which texts are written by humans; however, from the results of the generation group, whether it is the CNKI detection system or the Jianziyuan detection system, only 4 papers could successfully identify the presence of AIGC, resulting in a success rate of only 40%, which did not reach the passing line. It can be seen that current popular detection systems, while likely confirming that humans are not AI, do not have the ability to accurately determine that AI is AI. Although the approach of the CNKI detection system to assign values to different suspicion levels seems more scientific, overall, the actual suspected fragments detected are not as many as those of the Jianziyuan detection system, especially for the second abstract of the generation group, which was marked as highly suspected by both, where the detection result of Jianziyuan was significantly higher than that of CNKI. This indicates that the accuracy of the system is not directly related to the complexity of the calculation method for the generated results.
From the detection results of the rewriting group, the ability of the AI rewriting software to reduce the proportion and degree of AIGC is evidently insufficient, and sometimes it even backfires. Only the rewrites of the 8th, 9th, and 10th abstracts of the generation group successfully avoided system detection; other rewrites either did not reduce much or further increased the AI component. However, from the principle of the rewriting software’s reduction of AI, it essentially replaces AIGC phrases with expressions resembling those of real people, which means that if the detection system evaluates that the revised fragments contain more AI components, it indirectly indicates that the detection system is still not sensitive enough to identify human tones.
2. High Discrepancy Rate
Among the results of the generation group, only 4 papers had consistent detection results between the CNKI detection system and the Jianziyuan detection system, resulting in a discrepancy rate of 60% (where the discrepancy rate refers to the proportion of the number of papers with inconsistent detection results to the total number of detected papers, and whether they are consistent only refers to whether the systems can identify AIGC fragments, regardless of whether the proportions are exactly the same). Among them, the 2nd paper of the generation group was detected as highly suspected by both systems, while the 3rd, 4th, and 7th papers were detected as having no AI creation components; the detection results of the other 6 papers were completely different, with some being detected by the CNKI detection system as having AI creation components, and others being detected by the Jianziyuan detection system. The above results indicate that there are differences in the technical principles and reference models of different AIGC detection systems, and the training degree and application scenarios also vary, leading to inconsistencies in the identification of typical AI identification words, with notable differences. Although in the rewriting group, 9 papers had consistent detection results between the CNKI detection system and the Jianziyuan detection system, resulting in a discrepancy rate of only 10%, this only indirectly indicates that the reduction of AI by the rewriting software is very mechanical, and the detection systems can only consistently identify simple and easily recognizable AI rewriting identification words.
3. Weak Sensitivity
For the samples from the generation group that were detected as 0%, indicating no AI creation components were found, this study further conducted a detailed analysis of the texts and found that some AI identification words that are relatively easy for humans to recognize also passed the detection, such as “the above content emphasizes” and “this paper concludes that”. This indicates that the AI lexicon of the detection systems is lagging behind the training level of intelligent language models like ChatGPT, resulting in the systems being highly insensitive to the recognition of AI vocabulary, phrases, and tones. Moreover, from the 4 texts in the AI rewriting group that passed detection by both systems, it can be seen that some reduction techniques for AI were even more exaggerated and rigid (such as deliberately adding template-like conjunctions or modal words, using overly subjective descriptions, etc.), indicating that the detection systems are also not sensitive enough to some mechanical anti-AI techniques.
4. Root Causes of Technical Defects: Insufficient Model Training, Improper Algorithm Optimization, and Lack of Preset Lexicons
The data results of this study reflect that the actual identification capabilities of AIGC paper detection systems are not ideal, and there are significant shortcomings in assisting publishing entities to identify AI ghostwriting behaviors. On the surface, this may be due to the increased difficulty of detection, as the detection samples are generated by AI tools that have undergone corpus feeding and simple training, and they use legal literature materials with high language logic complexity; on the other hand, it is also due to the machine learning speed of AI tools far exceeding the update and iteration speed of detection systems. For similar texts generated by the same AI tool based on different versions or different developmental stages of the language model, the detection systems may only have the ability to identify old models and be powerless against new models. Meanwhile, a careful analysis of the technical principles of the detection systems reveals some deeper technical reasons.
Searching for the keyword “AI text detection” on the China Patent Publication Announcement website (http://epub.cnipa.gov.cn/Index) yields only a patent announcement record from Tongfang CNKI. The patent name is “A Method, Device, Medium, and Equipment for Detecting AI-generated Text”, with the patent application number 2023110993486 and publication/announcement number CN17151074A. The patent abstract claims that the invention can determine whether the text to be detected is AI-generated, not only with high detection efficiency but also unaffected by the subjective factors of reviewers, making the detection results more accurate. From the accompanying diagrams of the patent abstract (Figure 1), it can be seen that the detection method mainly consists of four parts: text classification model, target loss function, preset dictionary, and prediction model (S101-106 are specific implementation examples containing patent claims). The detection steps are as follows: ① The text classification model outputs the first probability value of the text to be detected to assess the likelihood of the text being AI-generated; ② The target loss function outputs the deviation characteristics of the text to be detected to assess the degree of difference between the text and human-written texts; ③ The prediction model and preset dictionary output the diffusion characteristic values of the text to be detected to indicate the vocabulary diversity and usage frequency within the text; ④ Statistical analysis yields the sentence length characteristics and word distribution characteristics of the text to be detected, combining the first probability value, deviation characteristics, and diffusion characteristic values to comprehensively determine whether the text to be detected is AI-generated. Moreover, the promotional slogan on the homepage of the CNKI detection system indicates that it is primarily based on the high-quality literature big data resources of CNKI that are structured, fragmented, and knowledge-ized, based on the algorithm logic of pre-trained large language models, combined with “knowledge-enhanced AIGC detection technology” and several detection algorithms, applying AI to detect AIGC from both the language pattern and semantic logic chains.
Figure 1 Technical Flowchart of CNKI AIGC Detection Service System
1. Insufficient Language Model Training Level
From the above publicly available technical content, it can be seen that the CNKI AIGC detection system mainly consists of a large language model established by the system itself and supporting algorithms. By comparison, it can be found that there are many differences between the large language model of the detection system and that of AI generation tools like ChatGPT (see Table 2), among which the most important difference is the different training mechanisms. The large language model of the detection system relies on a vast academic literature database provided by the technical entity, and through deep learning of massive academic literature, it constructs a large knowledge base. When a new article is submitted for detection, the system quickly compares it with the literature in the knowledge base to identify similar or duplicated content. Therefore, the sources of its training corpus mainly consist of two parts: one is the knowledge base constructed based on existing literature, and the other is the massive detection samples provided by institutional or individual users, where the former constitutes the human control group for detection, while the latter constitutes the AI control group for detection. Due to the richness of existing literature, the identification and differentiation training based on the human control group is relatively easy, which explains why the aforementioned detection can largely identify humans as humans. In contrast, both the CNKI detection system and the Jianziyuan detection system have been open for use for a relatively short time, and the cases where users directly submit AI ghostwritten papers for detection are relatively few, leading to a relative scarcity of corpus sources for the AI control group, and the maturity of its text recognition and differentiation training is far behind that of the human control group, which is why the detection systems in this study find it difficult to identify AI as AI.
Table 2 Differences Between AIGC Detection Systems and AI Generation Tools’ Large Language Models
Additionally, different detection systems have varying structural complexities in their large language models, and the differences in training time and corpus quality lead to different numbers of iterations achieved, resulting in varying levels of model learning maturity. Compared to the Jianziyuan detection system, while the CNKI detection system is supported by the largest academic journal database in China, which provides a good foundation for model construction, its high usage price may lead users such as schools and research institutions to choose the cheaper and more mature Jianziyuan detection system, thus limiting the training level of its model. This can also somewhat confirm why the suspected proportions detected by the Jianziyuan detection system were higher in the test results.
2. Improper Optimization of Target Functions in Algorithms
Different models may use different algorithms for training and optimization, and the efficiency and adaptability of these algorithms can also affect the final performance of the language model. Taking the CNKI detection system as an example, the target loss function in the algorithm is an important means of comparing the differences between the detection text and the AI control group, and changes in its operational efficiency and method will directly affect the text features that the model focuses on during the optimization process, thereby impacting detection performance. As the language model continues to undergo machine learning, the originally designed target loss function may struggle to continue matching the larger capacity knowledge base samples, and the newly added diverse text types and features may overload computational resources. If the function’s capabilities are not optimized and performance is not improved, it will lead to slow model iterations and reduced detection efficiency, ultimately affecting the accuracy of detection results.
Moreover, relevant functions may also set evaluation metrics for identifying and differentiating text features, and different systems set different metrics, leading to differences in the optimization direction of the language model, such as whether to focus more on improving local precision or overall accuracy. Based on the data from this study, although the output results of the CNKI detection system show that the suspected proportions are not high, its precision in fragment identification is higher due to the diversity of its evaluation metrics, which comprehensively determine the suspiciousness of the text to be detected based on four indicators: sentence length characteristics, word distribution characteristics, first probability value, and deviation characteristics. Assigning more refined weights to the suspicion levels instead of using simple ranges as input values for function operations can significantly reflect the suspicious features of the text. In contrast, while the Jianziyuan detection system identified more ranges of suspected fragments, its local precision was noticeably insufficient, making it difficult to highlight the suspicious features of specific statements. This allows us to infer that the diversity of evaluation metrics in the Jianziyuan detection system is somewhat lacking.
3. Lack of Human-Added Preset Lexicons
The “preset dictionary” in the CNKI detection system refers to a predefined vocabulary set or database used to assist the detection system in evaluating the use of specific words or expressions when analyzing and recognizing AI-generated texts. The preset dictionary typically includes high-frequency AIGC vocabulary, special expressions, language patterns, errors, or abnormal usages. The purpose of establishing such a lexicon is to provide a standard or reference set so that the detection system can effectively analyze and determine whether the text is AI-generated.
However, if the preset dictionary is only collected and expanded by the detection system through detection samples, then when encountering AIGC fragments that the system has never faced before, it will be difficult for the system to determine whether the sample is AI ghostwritten. The fundamental reason why both the CNKI detection system and the Jianziyuan detection system have weak sensitivity to AI samples lies in the lack of functionality for human assistance in adding AIGC lexicons. Some template-like AIGC fragments that can be easily identified by humans in the AI generation group (although template phrases can also be used by real people, they generally do not repeat frequently within a paragraph, which makes it easy for the detection system to overlook), such as “The conclusion of this study emphasizes” and “This paper mainly discusses”, managed to pass detection, indicating that the current market’s R&D enterprises have overlooked this aspect in the design of detection systems. In contrast, the AI rewriting software used in this study has the function of a customizable lexicon, which can continuously collect user-preferred phrases and sentences, enhancing its anti-detection capability against AIGC by continually learning and imitating the user’s writing style and habits. If this continues, the AIGC detection system will fall behind in the iteration and update of AI rewriting software, falling into a stagnation predicament.
4. Academic Journals’ Responses: Positive Interaction Between System R&D and Journal Development
Whether due to the abuse of AI generation technology or the iterative development of more targeted AI rewriting technology, academic journals face the challenge of identifying and managing AI-generated texts. Just like the widely used literature similarity detection systems, the future use of AIGC detection systems by journal entities is also imperative. However, as mentioned earlier, the current AIGC paper detection systems still have many technical defects. If journal entities introduce relevant systems but do not utilize and train the systems effectively to assist in the review process, it will be difficult to cope with the rapidly evolving AIGC technology and achieve the digital and intelligent development of academic journals. Therefore, this study believes that the R&D goals of publishing technology companies should permeate the overall development of the academic journal industry, and both parties need to actively build a human-computer collaborative and positive communication and cooperation cycle in this process. As the technology demand entity, academic journals should promote this positive interaction from three levels: R&D cooperation, industry development, and editorial practice.
1. R&D Cooperation Level
First, academic journal publishing units should actively communicate with technology providers to understand the latest AIGC detection technologies and propose suggestions based on their own needs to promote the continuous improvement and development of the detection systems. During the testing of the system’s use, I communicated with the system promotion personnel and provided feedback on some detection results and test samples to relevant backend personnel. During the communication process, it was found that the sales promotion personnel and technical service personnel of the detection system were not very clear about the design deficiencies and technical mechanism flaws of the system, and lacked detailed understanding of the iterative status and market development of AI generation and rewriting tools. This is largely due to the insufficient depth of use of detection systems by academic research institutions and journal units, resulting in a lack of negative feedback. System R&D personnel usually do not have experience in the academic publishing industry, and thus do not fully consider users’ actual usage experiences and feelings in upgrading and improving the system. As the largest user group of detection systems, if journal units do not proactively provide their needs and suggestions, it will be difficult for R&D personnel to promote the development of large language models, optimize relevant algorithms, and expand preset lexicons in real-time.
Second, academic journal publishing units should actively participate in testing new versions of detection systems, providing richer detection samples based on paper types, subject scenarios, and application fields to help technology providers optimize language models and supporting algorithms, making them more precise and efficient. As mentioned earlier, the training of the large language model of the detection system requires both a vast academic literature knowledge base and AIGC texts, but currently, the AIGC texts input by users to the system are still far from matching the existing knowledge base, leading to a relative scarcity of training materials and activities. Journals in different fields, subjects, and industries have diverse practical foundations and can output a large number of high-quality, highly professional, and highly realistic AIGC texts during publishing activities based on daily submissions. These quality texts can significantly reduce the iteration time of language models and continuously improve the system’s adaptability to different scenarios and the accuracy of detecting papers from different disciplines.
Finally, academic journal publishing units should focus on accumulating and recording special vocabulary or phrases suspected to be AI-generated discovered during publishing activities, categorizing them and adding them to subject-specific or practical scenario-based lexicons for reference as preset dictionaries. Relying solely on lexicons collected by R&D companies is insufficient to meet the corpus resources required for language models to identify and compare texts, while the high-frequency vocabulary, special expressions, language misuse, or abnormal usages accumulated by editorial, proofreading, and reviewing personnel in various publishing processes can significantly compensate for the system’s collection capability, further improving the comparison standards. The improvement of the preset dictionary is essentially a process of human-computer collaboration, where the lexicons created by individual journals are collected and published among the entire journal cluster, and the lexicons are then utilized by other journals to detect AI texts, providing positive feedback, thus forming a positive interaction between journals and between journals and systems.
2. Industry Development Level
The suspected text proportion fed back by the AIGC paper detection system is essentially a neutral value similar to the paper repetition rate, but unlike simple plagiarism or improper citation, the specific content of AIGC is not necessarily useless for academic research and journal industry development. As the gatekeeper of knowledge innovation, the journal industry holds the direction of knowledge production evolution, thus it is even more necessary to objectively evaluate the results of detection systems to avoid academic misconduct caused by AI ghostwriting while dialectically utilizing high-quality and authentic knowledge content generated by AI as the dual goal of using detection systems.
On one hand, academic journals should aim to filter out low-quality AI texts and strictly guard against academic misconduct caused by malicious use of AI tools. To this end, an internal local area network and journal industry cloud interconnected with academic journals can be established based on the AIGC detection system, creating tighter interconnectivity between detection system processing and journal result feedback evaluation, achieving sharing of AI manuscript rejection information and suspected AIGC texts. Currently, the CNKI Tengyun Editing System has adopted the “Journal Group” function in its upgraded version (V10.3) for journal interconnectivity. Whether due to multiple submissions or other academic misconduct, authors and articles may be blacklisted and rejected by the journal community. Meanwhile, the system also implements a one-click resubmission function for authors after normal rejections, greatly enhancing the resource-sharing capabilities of journals regarding papers in the same field. The development of the AIGC detection system can similarly utilize the sharing mechanism of journal groups to collectively combat habitual AI ghostwriters and jointly evaluate AI ghostwritten texts, enabling the journal industry to work together to address the AI chaos in the academic research field.
On the other hand, academic journals should aim to reasonably utilize high-quality AI texts, fully leveraging the knowledge production capabilities of AIGC technology. When evaluating the suspected text proportions provided by the AIGC detection system, journal units should consider the quality and authenticity of AI-generated content. Under the premise of appropriate citation and annotation, high-quality AI-generated content can also promote academic development. At the same time, journal publishing units should strictly control the standards of academic originality, skillfully guide authors to effectively and properly utilize AI tools, and demonstrate their independent thinking and academic contributions to avoid the occurrence of academic misconduct.
3. Editorial Practice Level
Editors are the main body that practically utilizes AIGC detection systems in journal publishing activities, and whether the detection results are accurate should ultimately be determined by the editors. For some highly human-like texts generated through in-depth training and tuning, although detection systems may struggle to identify their AI components through sentence composition and logical expression, editors can still grasp the authenticity and scientificity of the content to determine its authenticity. It is precisely because the AIGC detection system is not yet mature and has various defects that editors should play the role of human-computer relationship collaborators, establishing a correct concept of human-computer collaboration to guide the optimal development of the system.
First, academic journal editors should continuously pay attention to and promote the iteration of large language models in AIGC detection systems, pushing for performance optimization of model supporting algorithms and improving personalized preset lexicons. Although most journal editors have subject specialties but lack technical sensitivity, the resources that large models rely on for evolution fundamentally come from the daily reviewing and proofreading activities of editors. By providing more accurate manual annotations of detection results and continuously feeding back to the system to correct AI evaluation metrics, editors can correctly guide the language model to develop in a direction that benefits academic publishing practices. Additionally, editors from different journals can build a public training platform for models through active participation in journal groups and collaborative monitoring of anomalies, effectively avoiding the spread of AIGC content and pollution of the literature knowledge base, ensuring the reliability of the system’s foundational literature resources.
Second, academic journal editors should actively cultivate their skills in recognizing AIGC; knowing oneself and the enemy is essential to overcoming the various defects of AIGC detection. With OpenAI announcing on April 1, 2024, that ChatGPT can be used without registration, more and more journal editors will be able to learn and use cutting-edge AI tools more easily. Only through practical operations can journal editors gain a deep understanding of the working principles and algorithms of AIGC, effectively utilizing the analysis results of AIGC detection systems in journal publishing work, thereby exercising independent thinking abilities based on the system and applying what they learn flexibly to defeat AI with AI.
Third, academic journal editors should establish their own AIGC evaluation standards, based on disciplinary professionalism, situational applicability, and knowledge innovation to identify the output results of detection systems. These standards aim to ensure effective identification and utilization of AI-generated content while protecting academic integrity and promoting original research. Disciplinary professionalism means whether the article demonstrates necessary professional knowledge and theoretical depth, whether the use of professional terminology is accurate, and whether it appropriately reflects the current development status of the discipline; situational applicability means whether the content of the article is highly relevant to the themes and scope of the academic journal and whether it meets the specific needs and academic interests of the target readership; knowledge innovation means whether the theories, methods, experimental designs, or research results of the article are novel, and whether the cited content is a simple rehash of existing literature. Following these practical evaluation standards that align with the development rules of journals can break the mechanical cognition of the AIGC detection system, truly allowing AI to serve editorial work.
5. Conclusion
As AIGC technology matures and its usage barriers lower, journal editors, as gatekeepers against academic misconduct, will bear the responsibility of preventing AI ghostwriting behaviors. This author tested and compared the detection capabilities of two AIGC paper detection systems, providing a detailed analysis from the technical personnel’s perspective on the language models, algorithm functions, and evaluation parameters of the systems, analyzing the root problems behind the technical defects of the systems; then, from the perspective of academic journal editors, introducing the solutions to the defects into journal practice work, ultimately proposing that academic journals need to engage in positive interaction with technology providers to ensure that the achievements of system development can genuinely assist journal editors in identifying AI ghostwriting behaviors and reasonably utilizing high-quality AIGC content. Due to economic costs and space limitations, this study could not obtain more detection samples from various disciplines, nor could it use more detection systems to acquire more detection data, resulting in a lack of more credible data results in terms of large-scale statistical significance. Additionally, due to difficulties in obtaining more detailed public technical information about detection systems, the analysis of technical principles contains some conjectures based solely on technical logic but not yet verified by reality, which awaits further confirmation by system technical personnel. Future research is expected to continue advancing this topic in the field of AI text detection across multiple disciplines, scenarios, and links.
References
Swipe up to read
[1]GAO C A, HOWARD F M, MARKOV N S, et al. Comparing scientific abstracts generated by ChatGPT to real abstracts with detectors and blinded human reviewers[J]. NPJ Digit. Med., 2023, 6(1): 75. doi: 10.1038/s41746-023-00819-6
[2]Shen Xibin, Wang Lilei. Research on the Detection of AI-Generated Academic Journal Texts[J]. Science and Technology and Publishing, 2023(8): 56-62.
[3]Zhang Linghan, Jia Siyao. Logical Updates and System Optimization of AI-Generated Content Identification System[J]. Qiushi Journal, 2024, 51(1): 112-122.
[4]Jiang Xueying, Liu Xin. Academic Production and Publishing under Generative AI Technology: Transformation, Deviance, and Pathways[J]. Digital Library Forum, 2023, 19(5): 64-71.
[5]Wang Pengtao, Xu Runjie. Research on the Reconstruction of Trust Mechanism in Academic Publishing under AIGC Interventions[J]. Library Information Knowledge, 2023, 40(5): 87-96.
[6]Zhang Zhongyi, Niu Xinyue, Sun Junyan, et al. Exploring ChatGPT: Opportunities and Challenges in Academic Publishing under AI Large Language Models[J]. Research on Chinese Scientific Journals, 2023, 34(4): 446-453.
[7]Zhao Kai. Copyright, Ethics, and Value Auditing—New Challenges to Editorial Competence from AI-Generated Content (AIGC)[J]. Science and Technology and Publishing, 2023(8): 62-68.
[8]Cao Lianyang. Pre-Review: Strategies for Academic Publishing to Respond to AI-Generated Content[J]. Publishing Reference, 2024(1): 27-30.
[9]Ji Xiaole, Wei Jian. Generative AI and Academic Journal Publishing: Impacts and Responses[J/OL]. Journal of Shenyang Normal University (Social Science Edition): 1-9 [2024-04-07]. https://doi.org/10.19496/j.cnki.ssxb.20240029.001.
[10]Luo Feining, Liu Zhuang, Xie Wenliang. Identity Challenges and Role Transformation of Editors in the Era of AIGC[J]. Publishing Angle, 2023(15): 65-69.
[11]Yu Qianwen, Wang Muliang, Gao Yujie, et al. From PGC to AIGC: Content Transition, Value Examination, and Response Strategies of Scientific Journals—Based on the Perspective of Academic Journal Editors[J]. Journal of Editing, 2023, 35(S2): 161-164.
[12]Chen Zeqi, Huang Xiaofeng. Responses of Journals to the Background of AIGC[J]. New Media Research, 2024, 10(2): 1-4.
Journal Introduction
Journal of Publishing and Printing was founded in 1990 and is supervised by the Shanghai Municipal Education Commission and sponsored by Shanghai Publishing and Printing College, approved by the national publishing authorities as an academic journal. This journal focuses on the training of modern publishing and printing professionals, serving the transformation and development of the publishing and printing industry, and promoting the deep integration of production, education, and research in the publishing and printing field. The main readership includes education, research, and practitioners in the publishing and printing field. The main columns include Focus of the Issue, Research and Observation, Publishing Practice, Journal Research, Printing and Packaging, Publishing Integration, Professional Talent Cultivation, Publishing History, etc., emphasizing academic, professional, practical, and readable content.
The Journal of Publishing and Printing is an expanded journal in the “Comprehensive Evaluation Report of Chinese Humanities and Social Sciences Journals (2022)”. It is a source journal for the People’s Daily Newspaper Reprint Data. It is a statistical source journal for the “Annual Report of Academic Journal Impact Factors in China”. It is included in the Scopus database. Full text is included in CNKI, Wanfang Data, Chinese Scientific and Technological Journal Database, Super Weeklies, the “Chinese Humanities and Social Sciences Journal Evaluation Report (AMI)” citation database, Longyuan Journal Network, and Titanium Academic Literature Service Platform. In 2021, it was selected as an excellent journal in East China.
Journal Website:
https://cbyys.sppc.edu.cn
Submission and Review System:
http://www.manuscripts.com.cn/cbyys
Electronic Journal:
Editorial Email:
Shanghai Publishing and Printing College
Editorial Office of Journal of Publishing and Printing
101 Yingkou Road, Shanghai
Postal Code: 200093
Phone: 021-65686532