New Intelligence Report
New Intelligence Report
[New Intelligence Overview] Is there another choice that allows humanity to perfectly navigate history? Could the results of simulations of historical wars conducted by scholars from the University of Michigan and Rutgers University using LLMs be our reference answer?
“Can we avoid war at the crossroads of history?”
This question has been continuously raised and pursued by individuals, scholars, policymakers, and organizations throughout human history.
Recently, scholars from the University of Michigan and Rutgers University utilized advances in artificial intelligence (AI) and large language models (LLMs) to answer this question.
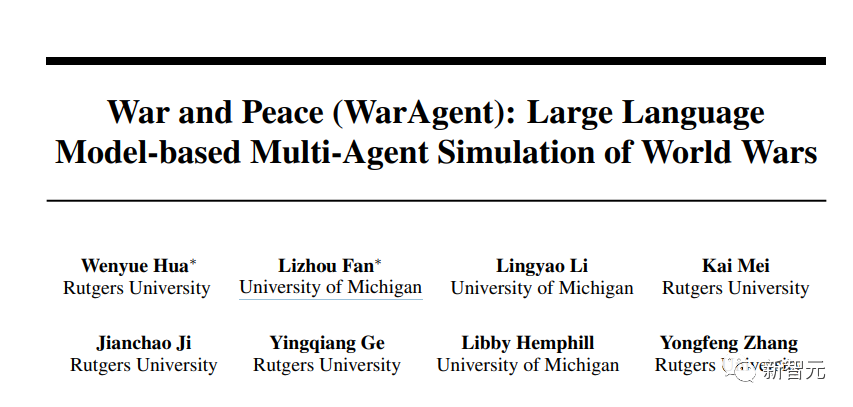
Paper link: https://arxiv.org/abs/2311.17227
The final result of the research is a multi-agent system (MAS) driven by LLM called WarAgent.
It can simulate the participating countries, their decisions, and the consequences in historical international conflicts.
Although simulations of this kind have a long history in social science applications, early attempts were often limited by computational power and simplified models.
The latest simulations use LLMs, which can simulate complex human behaviors and interactions, such as Stanford’s AI Town and Werewolf game simulations.
However, in previous studies of LLM applications in simulations, there has been no research on how to apply these advanced technologies to simulate the subtleties and complexities of international diplomacy and war.
WarAgent is the first LLM-based multi-agent system designed to simulate historical events.
The conflicts simulated in this research include World War I, World War II, and the Warring States period in China.
But how can the effectiveness of this LLM-based multi-agent system simulation be determined? How can MAS effectively reproduce the historical evolution of strategic planning and decision-making processes?
What are the key factors that trigger war? Can these factors be identified through LLM-based multi-agent system simulations?
Is war an inevitable event in history? Can LLM-based multi-agent system simulations reveal the conditions that lead to war (or peace)?
If you want to clarify these questions, please continue reading.
These agents are typically designed to simulate behaviors and decision-making processes in complex real-world or virtual environments.
The existing MAS field can be roughly divided into three types:
Reasoning-enhanced systems: These systems leverage the collective intelligence of multiple agents to enhance problem-solving capabilities. For example, LLM-Debate introduces the concept of debate, allowing agents to receive responses from peers and refine solutions through a “mental argumentation” process.
ChatEval established a role-playing-based multi-agent referee team to assess the quality of text generated by language models (LLMs).
Corex provides diverse collaboration modes, such as debate, review, and retrieval, to enhance the factuality, fidelity, and reliability of the reasoning process.
NPC multi-agent systems: These systems have made significant progress in simulating human behavior. For example, Generative Agents simulate human behavior and showcase it in a sandbox environment similar to “The Sims.”
Humanoid Agents introduce elements such as basic needs, emotions, and relationship intimacy, making agent behavior closer to human-like.
GPT-Bargaining studies whether agents can autonomously improve negotiation skills through negotiation games.
Production-enhanced systems: These systems aim to improve productivity and effectiveness. For example, MetaGPT is a special LLM application based on a multi-agent dialogue framework that can be used for tasks such as automated software development.
BOLAA is a control module that can manage choices and communications among multiple collaborative agents.
OpenAGI combines LLMs and various tools to solve complex tasks.
Many such MAS have emerged, such as BabyAGI, AgentVerse, and Camel.
These MAS applied in reality demonstrate tremendous potential in the field of artificial intelligence (AI) research, especially in improving problem-solving capabilities and understanding complex dynamic systems.
In WarAgent, researchers focus the simulation setup on simulating international conflicts, specifically World War I (WWI), World War II (WWII), and the ancient Warring States period (WSP) in China.
The simulation system’s setup includes detailed descriptions of country agents and available action spaces, which specify the inputs required for executing actions and the possible outcomes.
Each country agent’s configuration includes the following six basic dimensions:
Leadership: The political institution responsible for decision-making, concretized by the specific historical context.
Military Capability: Includes quantitative data, such as troop size and naval tonnage, as well as qualitative assessments of overall military strength, including specific advantages in particular sectors (such as navy or air force).
Resources: Important elements include geographical location, population, gross domestic product (GDP), terrain, and climate conditions.
Historical Context: Involves unresolved previous conflicts and interest conflicts between countries, which can significantly impact current policies.
Key Policies: Outlines the main goals pursued by the country.
Public Morale: Reflects the emotions of the populace, which can directly or indirectly influence the actions of the country.
The following diagram illustrates the country agent setup using the UK as an example.
Additionally, the simulation design of WarAgent includes a series of actions that shape relationships between countries, including the following types of actions:
Waiting Action: Agents may take a passive stance in certain turns.
Total Mobilization: Steps to prepare the military for potential conflict.
Declaration of War: Officially initiating hostilities with another country.
Military Alliance: A formal agreement providing mutual support between two or more countries.
Non-Interference Treaty: A diplomatic agreement in which the signatories commit not to interfere in each other’s internal affairs.
Peace Agreement: A negotiated solution between conflicting parties, formally ending hostilities.
Sending Information: In addition to formal actions, agents can also communicate informally through information, discussing various issues.
Furthermore, researchers have set three key properties for simulating international diplomatic actions: publicity, input type, and response demand, to enhance the simulation capability of WarAgent, enabling it to realistically and dynamically represent international diplomatic actions.
At the same time, researchers adopted a strategy of anonymizing country names and making slight modifications to historical facts to avoid the issue of large language models recalling and reproducing actual historical trajectories due to their extensive training.
This approach ensures that these modifications do not substantively affect the effectiveness of the simulation while maintaining the integrity and originality of the simulation results.
This includes core components and the information exchange mechanisms between agents within the MAS.
The core components include the following four parts:
Country Agents: These agents represent the various countries in the simulation and are defined by their corresponding country profiles.
In each response round, country agents generate actions based on the current situation. These actions are guided by carefully constructed prompts to help agents handle complex international relations situations and ensure their actions and decisions are well-considered.
The left diagram illustrates the key framework of prompt design in the study. The right diagram is an example of interaction with the GPT-4 model using the French agent.
As seen, the key framework of prompt design includes the following steps:
Step 1: Identify potential ally countries. For example, France considers the UK a potential ally due to its opposition to the German Empire, and sees the US as a strategic ally considering geographical location and strong economy.
Step 2: Identify potential hostile countries. In this scenario, France views the German Empire as its main enemy due to historical hostilities, and sees Austria as another potential enemy due to its alliance with Germany.
Step 3: Outline the recommended actions. In the given scenario, France suggests three actions: allying with the UK, initiating dialogue with Austria, and considering signing a non-interference treaty with the US.
Step 4: Summarize the analysis of the situation based on the responses to the first three prompts. In this scenario, France concludes that the assassination of the Austrian king provides an opportunity to ally with Austria against Serbia. However, care must be taken to avoid provoking the German Empire or Russia. At the same time, it is recommended to seek an alliance with the UK and to reach a non-interference treaty with the US.
Secretary Agents: Each country agent is paired with a secretary agent for additional support.
The setup of secretary agents arises from the limitations of LLMs themselves: while LLMs are powerful tools, they are not infallible. For example, they can easily produce erroneous information (hallucination) in lengthy and complex scenarios and lack perfect logical reasoning capabilities.
To address these limitations, each country agent is equipped with a designated “secretary agent” to verify the appropriateness and basic logical consistency of its actions. The secretary agent has two functional roles:
First, it ensures that all actions taken by the country agent conform to the allowable parameters set within the provided action space, including correct action names and correct input formats based on defined action attributes.
Second, the secretary agent is responsible for verifying the basic logical coherence of these actions. For example, if the UK does not initiate the process by sending a “request for military alliance” to Austria-Hungary, then Austria-Hungary “accepting a military alliance from the UK” would be illogical and unacceptable.
Board: Used to manage interactions and relationships between agents and serves as a dynamic record platform, collecting and displaying the relationship dynamics occurring in each round of simulation, ensuring that agent decisions are based on the most up-to-date available information.
The board can initialize the state of agents, update their relationships, and visually and textually present them.
In the study, the board is set to track and manage the following four different types of international relationships between countries:
Declaration of War (W): Indicates conflict or war between countries, represented by the symbol “x” and marked in red in the diagram. For example, the German Empire declared war on Great Britain.
Military Alliance (M): Indicates a formal military partnership between countries, represented by the symbol “&” and marked in green in the diagram. For example, Serbia and Russia signed a military alliance.
Non-Interference Treaty (T): Represents agreements not to interfere in international affairs, represented by the symbol “.” and marked in blue in the diagram. For example, Austria-Hungary and France signed a non-interference treaty.
Peace Agreement (P): Represents agreements to formally cease hostilities and maintain peace between countries, represented by the symbol “~” and marked in yellow in the diagram. For example, the US and the Ottoman Empire reached a peace agreement.
Archive (Stick): This component is the internal record-keeping system for each country agent, representing domestic regulations or statutes.
It helps ensure that the actions of country agents align with their predefined agreements and standards.
The following diagram focuses on tracking key indicators critical to a country’s decision-making process, including mobilization (MO), internal stability (IN), and readiness prediction (WR).
Mobilization (MO): A binary measure indicating whether a country is mobilized to respond to potential conflict, e.g., “Yes” or “No.”
Internal Stability (IN): An indicator of a country’s internal stability level, e.g., “Low,” “Medium,” and “High.”
Readiness Prediction (WR): An indicator predicting a country’s readiness status, e.g., “Low,” “Medium,” and “High.”
In the experiment, the board and archive collaborate through the following process.
In the study, the information exchange mechanisms within the MAS primarily include the following two types of interactions:
Agent-Secretary Interaction: This interaction explores how each country agent communicates with its corresponding secretary agent, focusing on decision-making and information verification.
Agent-Agent Interaction: This interaction studies the communication and information sharing processes between different country agents.
In the agent-secretary interaction, each round of simulation involves designated interactions between each country agent and its secretary agent.
As shown in the diagram, the country agent proposes an action plan, and then the secretary agent evaluates the format, content, and logical coherence of that plan.
If the secretary agent identifies inconsistencies or areas for improvement, it will make suggestions and engage in dialogue with the country agent for revisions.
This iterative process can go through up to four rounds of exchanges. If consensus is not reached during these exchanges, the secretary agent will proactively modify the proposal.
The agent-agent interaction is triggered by key events in history or simulations, providing starting points for decision-making and actions throughout the simulation.
For example, the assassination of Archduke Franz Ferdinand of Austria-Hungary is widely regarded as a triggering event for World War I.
Similarly, the invasion of Poland by the German Empire is commonly viewed as a triggering event for World War II. In the context of the Warring States period, the partition of the Jin state by the Han, Zhao, and Wei families is often seen as a triggering event.
The research evaluated simulation results from the perspectives of military alliances, declarations of war, and non-interference treaties.
In all military alliance simulation results, consistent alliances were formed between the UK and France, the German Empire and Austria-Hungary, and Serbia and Russia. These results reflect historical alliances and are influenced by factors such as linguistic and ethnic commonalities, strategic and political considerations.
At the same time, all declarations of war consistently occurred between Austria-Hungary and Serbia, Austria-Hungary and Russia, and the German Empire and Russia. In this simulation, the conflicts of this period began with Austria-Hungary declaring war on Serbia.
This was followed by a series of declarations from various countries structured as follows: (German Empire to Serbia, Russia to Austria-Hungary, France to German Empire, Russia to German Empire, UK to German Empire), where the country on the left represents the declaring country and the country on the right represents the country being declared upon.
For Austria-Hungary, Serbia was seen as a direct adversary, primarily due to the assassination of the Austrian king, which served as the direct catalyst for their declaration of war. The subsequent declarations were the result of the existing alliance structure, aligning with the alliances and hostilities of that historical period.
Additionally, in every simulation, the US participated in at least one non-interference treaty 100% of the time. Similarly, the Ottoman Empire was involved in such treaties in 85.7% of simulation runs during this period.
The reason for these results is that the US focused on protecting its wealth and avoiding unnecessary entanglements. This led to a tendency to seek non-interference treaties with other countries to ensure distance from potential conflicts.
Furthermore, the US also considered using diplomatic communications to gather intelligence and convey its intentions, aligning with its policy of separation. Similarly, the Ottoman Empire sought to avoid direct involvement in conflicts, aiming to maintain a neutral stance or establish defensive alliances.
Thus, the diplomatic strategies of the US and the Ottoman Empire to pursue non-interference treaties and engage in diplomacy with neighboring countries are consistent with their broader policies of maintaining their respective positions, explaining why both countries did not become major players in the main conflicts of that time.
These results are highly similar to actual history, indicating that under the default setting of using the assassination event as a trigger, the simulation evolution of WarAgent is effective in reproducing historical scenarios.
At the same time, the research conducted accuracy analyses of the simulation results based on aspect and time series analyses.
The time frame analyzed in the research spans from June 28, 1914, to August 4, 1914, to evaluate the accuracy of simulated alliances and declarations of war.
This period was chosen due to its historical significance. The Battle of Liège, which began on August 6, 1914, is historically regarded as the first major battle of World War I, symbolizing the active involvement of most major European countries in this conflict.
Therefore, this study considers this battle as a key moment when the fundamental dynamics of the war began to solidify. The accuracy calculations also take into account alliances and declarations of war formed before this critical juncture.
In the accuracy analysis, the researchers conducted seven separate simulation runs and reported average accuracy to reduce the impact of randomness.
Specifically, the study focused on three main dimensions: the accuracy of simulated alliances compared to historical alliances, the accuracy of simulated declarations of war, and the mobilization status of each country.
Considering the time points used for simulation, the simulations followed actual historical events to obtain the following benchmark facts:
In terms of alliances, the benchmark alliance set includes: UK and France, Russia and Serbia, Austria-Hungary and the German Empire, Russia and France, Ottoman Empire and the German Empire.
In terms of declarations of war before the Battle of Liège, the benchmark declaration set includes: Austria-Hungary to Serbia, Russia to Austria-Hungary, German Empire to Serbia, Russia to German Empire, France to German Empire.
In terms of mobilization, at that time point, all countries except the US were in a state of mobilization.
Regarding the outbreak of the World War, we assess whether major countries (UK, France, Russia, German Empire, Austria-Hungary) were involved in the war.
As shown in the table, the accuracy of alliance simulations in WarAgent reached over 75%, while the accuracy of mobilization exceeded 90%, and the accuracy of simulated declarations of war was relatively lower.
However, overall, in all simulation results of WarAgent, World War broke out without exception.
Reasons for War
The researchers believe that to clarify the reasons for the outbreak of war, it is first necessary to clarify various triggering events to determine their potential impact on avoiding World War I.
In WarAgent, three different intensities of triggering events were chosen, including no conflict, serving as a comparative baseline null triggering event; the “Anglo-German Naval Incident” representing medium-intensity conflict; and the most intense trigger, the “Austro-Russian Dardanelles Conflict.”
To ensure the robustness of the results, the researchers conducted three simulations for each triggering event.
However, the results indicate that various triggering factors of different intensities could influence the immediate outbreak of war.
Even in the “Null” (no events) scenario, a “Cold War” situation was observed, indicating that even minor events could significantly escalate tensions.
The inevitability of such minor triggering events means that major conflicts like World War I are ultimately bound to occur.
Inevitability of War
The inevitability of war is the first experiment in counterfactual analysis.
This study explores the issue from two main perspectives: the decision-making processes of agents and the parameter settings of countries.
In the experiments, the researchers analyzed the aggressiveness of country decision-making and the critical conditions of countries that affect the likelihood of war by manipulating these two aspects.
The study examined the decision-making processes of agents under three settings: default, aggressive, and conservative. For this, the general system settings of country agents were adjusted for experimentation.
This was done to assess how the overall aggressiveness or conservativeness of agents influences the inevitability of war.
Under aggressive and conservative settings, three experiments were conducted, each including 10 rounds of simulation.
The analysis from the study indicates that when the system and action analysis settings are more aggressive, the likelihood of war significantly increases.
Under the default setting, the first declaration of war takes several rounds to observe, but under the aggressive setting, the first round sees a declaration of war; under the conservative setting, after 10 rounds, only proposals and acceptances of military alliances, non-interference treaties, and peace agreements were observed in agent actions.
This suggests that the aggressiveness of agents greatly exacerbates the likelihood of tensions and conflicts.
The parameters of countries primarily involve the six key factors (leadership, military capability, resources, historical context, key policies, public morale) previously mentioned. The researchers modified the internal settings of five country agents in these aspects. Quantitative analysis was conducted on military capability and resources, and experiments were carried out at three levels: default, abundant (three times the default), and scarce (one-third of the default) to assess their impact on the likelihood of war.
For historical context, public morale, and key policies, the researchers modified specific relationships and examined their influence on declarations of war.
Due to the variability of leadership and the difficulty of systematically quantifying it, the simulation excluded it from the model.
The study found that historical context, key policies, and public morale play significant roles in determining a country’s tendency towards war.
In experiments examining the cases of France and the German Empire, historical grievances and nationalist sentiments, rooted in past conflicts and territorial disputes, significantly influenced their military engagement.
For example, the Franco-Prussian War of 1870-71 led to the unification of the German Empire and France’s loss of Alsace-Lorraine, leaving France with a lasting hostility and desire for revenge. This historical context laid the groundwork for future conflicts, as France sought to regain lost territory and prestige.
In examining the key policies and public morale of the US, the effects were immediate. In all simulations, this adjustment led the US to actively seek alliances, particularly with the UK and France.
The establishment of these alliances marked a significant shift in the US’s international stance, leading to its active involvement in World War I. This scenario illustrates the potential consequences of the strategic realignment of US foreign policy, highlighting how such changes can dramatically alter a country’s role and actions in global conflicts.
At the same time, while military capability and resources are influential, they do not solely determine a country’s decision to engage in war.
The German Empire, possessing significant military advancements and resources, could have pursued a more aggressive expansionist policy. However, historical and diplomatic contexts, such as alliances and mutual defense treaties, often played a more decisive role in its military actions.
Similarly, despite France being militarily weaker than the German Empire at certain times, it adopted a hardline military policy driven by historical factors, leading to its involvement in World War I.
In summary, while military capability and resources are key components of a country’s war decision-making, historical context, including past conflicts, nationalist sentiments, and long-standing competitive relationships, often act as catalysts for these decisions.
This underscores the importance of understanding historical context to fully grasp the dynamics of international conflict.
Through counterfactual alterations of national environments, the experiments on the inevitability of war further support this view, indicating that changes in national policies are necessary to shift away from the path of conflict.
These findings emphasize the certainty of conflict in specific circumstances but also point to the potential for strategic modifications of national policies or relationships as means to alter these seemingly predetermined outcomes.
These impacts transcend previous historical analyses, providing a blueprint for using artificial intelligence to understand human history and, where possible, prevent future international conflicts.
https://arxiv.org/abs/2311.17227
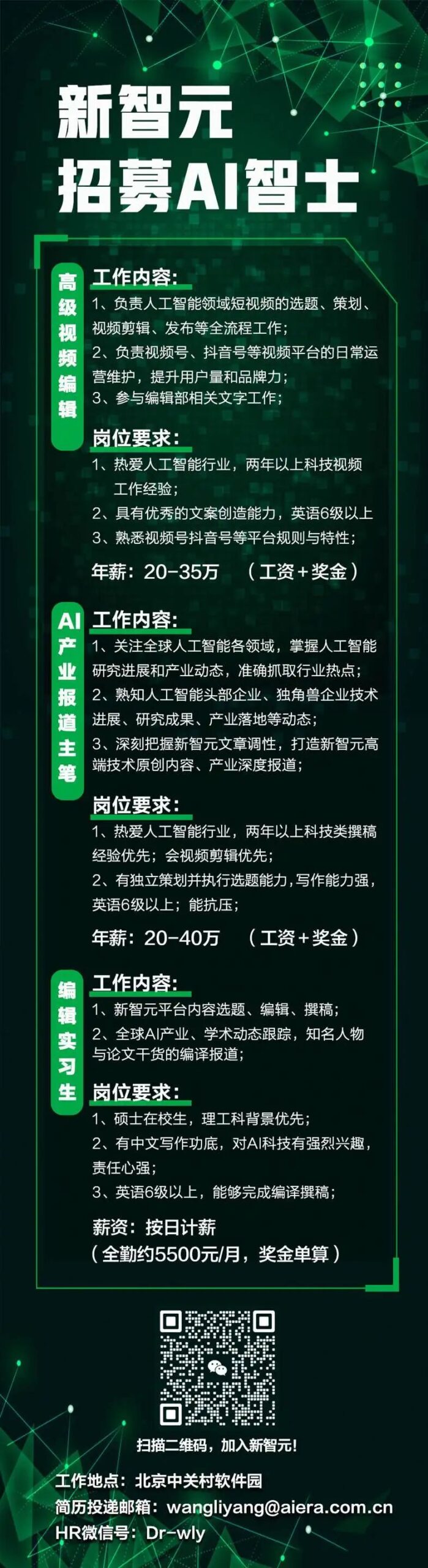
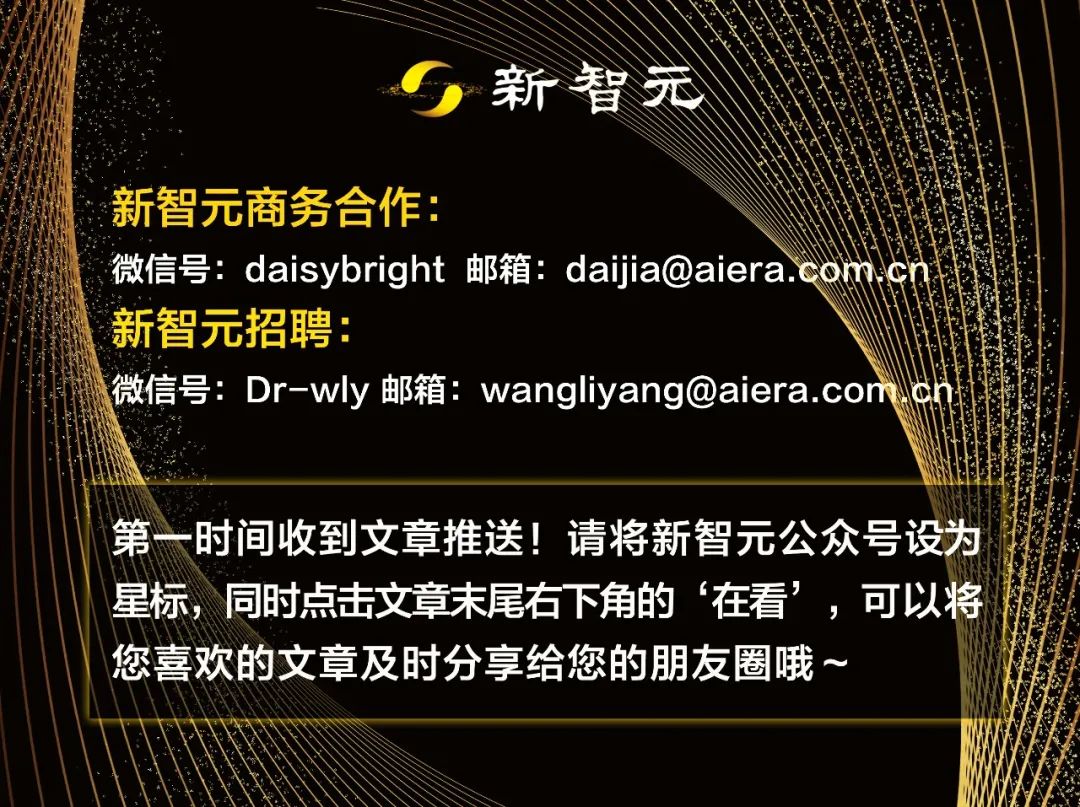