2018 Issue 34
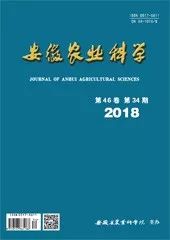
Authors: Zhang Meng, Qian Rong, Zhu Jingbo, Zhang Liping, Li Runmei, Dong Wei*
Affiliation: Anhui Academy of Agricultural Sciences, Institute of Agricultural Economics and Information
Introduction: Zhang Meng (1991—), male, from Bozhou, Anhui, research intern, master’s degree, engaged in image recognition research. *Corresponding author, associate researcher, master’s degree, engaged in plant protection information research.
Funding Projects: Anhui Academy of Agricultural Sciences Institute Co-construction Team Project (18C1424); Anhui Academy of Agricultural Sciences President Fund Project (17F1414, 17B1422).
Crop pest damage has always been an important issue in agricultural production. Early identification, monitoring, and early warning of pest damage are crucial prerequisites for controlling the widespread spread of pests, protecting crop quality, and reducing pesticide residues that pollute the environment. It is the source of decision-making information for prevention and control work.
With the development of computer technology and information technology, people have gradually applied image recognition technology to pest identification. By perceiving the geometric information of pests, images of pests can be described, stored, and understood to find a real-time, accurate, efficient, and convenient pest identification method. At the same time, field pest images are an important component of agricultural big data. Accurately identifying them and using this as a basis for pest control is also an important link in achieving smart agriculture.
1
The image recognition methods for crop pests began with the study of insect morphology, describing and identifying the morphological features of individual insects.
As early as the 1980s, Daly et al. and Zhou et al. attempted to identify insects such as bees and mosquitoes using morphological features on wing images, achieving good recognition results. Shen Zuorui et al. were among the first experts in China to use mathematical morphology for insect image recognition and verified the feasibility of applying morphological features for classification on the taxonomic level through experiments. Zhang Hongtao et al. extracted 17 morphological features from the binary images of stored grain pests and optimized them through the ant colony optimization (ACO) algorithm, ultimately using a support vector machine (SVM) classifier to classify 9 types of grain pests, achieving a recognition rate of over 95%. Wang et al. used content-based image retrieval (CBIR) methods to study the recognition of 9 types of insect images and 17 types of butterfly images, both achieving good recognition results. Zhu Leqing et al. took insect wing images as recognition objects, combining Weber local descriptor (WLD) and histogram of color (HoC) to achieve automatic classification of insect images, testing on a database containing 10 species with a total of 576 samples, achieving 100% independent prediction accuracy. However, most of the early studies were conducted in ideal laboratory environments, and further research is needed in practical applications.
To achieve pest recognition in complex environments, many scholars have conducted research on the issue of feature information loss in field pest images, focusing on multi-feature fusion, optimization learning methods, and other aspects.
Wen et al. used SIFT descriptors to extract local features and combined them with global features to establish classification models for identifying 8 types of orchard pest images, achieving an accuracy of 86.6%. Fan Weijun et al. proposed a method for extracting pose-invariant Hu moment feature vectors based on sector transformation, achieving a recognition accuracy of 100% for 24 types of larval images with different poses. Yao et al. extracted 156 features such as color, traits, and texture into an SVM classifier, achieving a recognition accuracy of 97.5% for 4 types of Lepidoptera pests through 7-fold cross-validation. Zhu Li et al. proposed a pest visual recognition technology using color features, employing histogram back projection of pest benchmark images and calculating cross-matching indices, establishing classifiers with matching indices and pest labels as training data, achieving a recognition accuracy of 92% for 5 types of pests including cabbage bugs and cabbage caterpillars.
The diversity and complexity of crop pests in natural environments pose significant challenges for automatic recognition, making it possible to apply only in specific areas and among species.
2
In recent years, the rise of deep learning has led to breakthrough developments in various fields of artificial intelligence. As a classic representative in deep learning, convolutional neural networks (CNN) have achieved great success in image detection, classification, and recognition. Compared to traditional algorithms that manually extract features or rely on prior knowledge, CNN can generate local perceptual areas directly on images, training layer by layer through multiple filters, autonomously obtaining key features in images, significantly reducing training errors caused by human misjudgment.
Cheng Xi et al. used GoogLeNet and AlexNet models to recognize stored grain pest images, achieving accuracy rates of over 95%. Liu et al. designed an agricultural pest classifier using deep convolutional neural networks that learned local features, achieving an average accuracy of 95.1% on test datasets. Yang Guoguo et al. proposed a deep learning model based on convolutional neural networks that can quickly and accurately locate and identify 23 types of pests in tea garden environments, with a recognition accuracy of 91.5%.
Due to the high interspecific similarity, high intraspecific variability, rich pose changes, and susceptibility to crop occlusion of pest images, CNN has better adaptability compared to traditional methods and can achieve higher accuracy in recognizing multiple types of pest images. In recent years, the rapid development of algorithms and computer hardware, along with data sharing enabled by the internet, has laid a solid foundation for the rise of deep learning.
3
Existing traditional recognition methods are mostly semi-interactive and require manual selection of feature parameters, which necessitates a large number of experiments and experience in selecting the best parameters, a challenge that has not been well resolved. Moreover, traditional methods mostly focus on pest recognition within small scopes, while field pests are complex, and dozens or even hundreds of pest species may appear in the same area, making application difficult.
Deep learning has high hardware requirements and needs massive labeled data; the development of deep learning relies on the accumulation of computational power and data. Most pests exhibit strong stress and concealment, making the collection of pest images much more difficult than other images, and the data volume is far from meeting the standards for deep learning. Additionally, due to the high interspecific similarity among pests, some pests require a sufficient number of high-resolution sample images to build usable recognition models, posing significant challenges for both data accumulation and computational power.
Pest image recognition is an interdisciplinary field combining information technology and agricultural technology. Compared to traditional methods, real-time recognition of pest images in complex field environments using deep learning is an inevitable trend. How to reduce the sample size requirements for deep learning and minimize the impact of complex field environments on recognition accuracy are urgent problems to be solved. In recent years, the emergence of transfer learning has provided good development prospects in this field. Traditional methods are more suitable for small sample processing, and when the image sample size is insufficient, they can more easily achieve high recognition accuracy for less complex images.
4
Given the diversity of crop pests and their morphological and behavioral changes with the environment, recognition models also need to be continuously improved based on actual conditions. In this context, constructing deep learning-based pest image recognition models and systematically optimizing them from both sample and algorithm perspectives is the development trend for achieving automatic pest recognition in complex field environments. Combining deep learning technology with sensors to achieve automated and intelligent real-time monitoring of crop pests in the field, and integrating data information into agricultural big data through the Internet of Things, will establish an information service platform based on “Internet+” and truly realize smart agriculture.
Recommended Papers
Paper|Analysis of the Competitiveness of Anhui Agricultural Products Export from the Perspective of the Belt and Road Initiative
Paper|Mutation Analysis of White Spot Syndrome Virus WSV006 in Some Regions of China from 2016 to 2017
Paper|Design of a Tillage Machine Suitable for Fermentation Bed Management of Cage-raised Chickens
Paper|Soil Nitrogen and Phosphorus Storage Characteristics under Different Land Use Patterns in Hilly Areas of Purple Soil
Paper|Evaluation and Development Strategies of Tourism Resources in Green Tree Ge Scenic Area
Paper|The Relationship between Melatonin and Plant Hormones during Plant Growth and Development
Paper|Effects of Potassium-releasing Bacteria on Soil Microbial and Enzyme Activity with Different Clay Mineral Contents
Paper|Degradation of Mulching Film and Its Effects on Soil Moisture and Cotton Yield
Paper|Research on Water Resource Management Information System in the Niyang River Basin Based on GIS
Paper|Effects of Freeze-thaw Action on Soil Carbon and Nitrogen Mineralization in the Greater Khingan Mountains
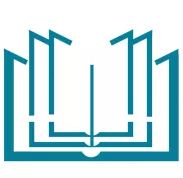
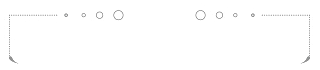
Anhui Agricultural Sciences
Academic|Popular Science|Three Rural Issues|Focus|Learning|Life
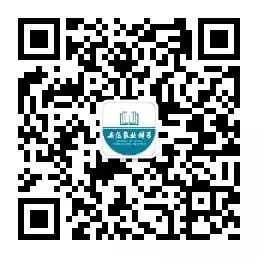
A down-to-earth academic journal public account
Paper Submission: [email protected]
✄ Editing: Jiang Jiang ✄ Typesetting: Xiao Tong