Probability And Feedback: ChatGPT’s
Intelligent Principles And Human-Machine Content Co-Creation
Author | Deng Jianguo
Professor and PhD Supervisor, Department of Communication, Fudan University
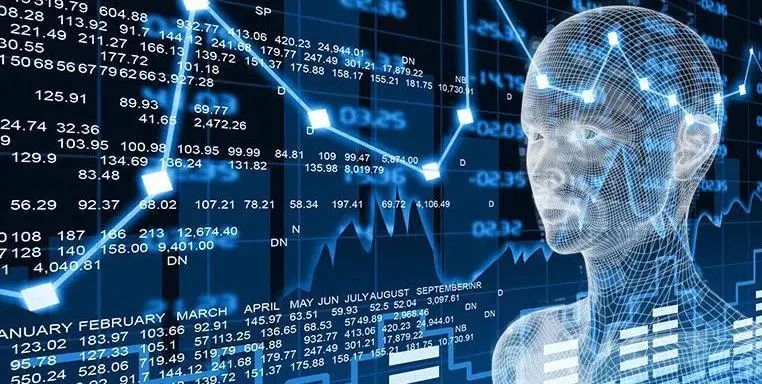
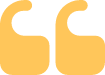
Abstract
ChatGPT, as the latest and most powerful artificial intelligence emerging in the second decade of the 21st century, is fundamentally based on two important theories that have driven the development of artificial intelligence since the mid-20th century—”Information Theory (Probability)” and “Cybernetics (Feedback)”. These two theories have also greatly promoted the development of communication studies. The probabilistic language analysis and patchwork content innovation of ChatGPT have long been a method of cultural production for humanity, challenging today’s system of “original authors”. In the future, ChatGPT will continue to learn and become more creative, thereby exerting more innovative pressure on humans. Humans will be able to train and collaborate with ChatGPT, continuously initiating a new “Romantic Movement” based on and beyond “Artificial Intelligence Generated Content (AIGC)” and ultimately becoming increasingly creative.
In November 2022, OpenAI launched the web dialogue platform ChatGPT based on the “Third-Generation Generative Pre-trained Transformer” (GPT-3) large language model, which quickly gained immense attention and discussion worldwide. What is the principle behind its “intelligence”? What is its relationship with the most important medium for humans—language—and the cultural production methods that have existed since ancient times? Is it merely a transporter of human content? Or could it also be a creator? What challenges will its arrival pose for humanity? How can we maximize benefits and minimize harm? This article attempts to address these questions.
1
The “Intelligent” Principle of ChatGPT: Probability
And Feedback
(1) Shannon’s Information Theory: Natural Language Processing Based on “Probability”
The large language model on which ChatGPT is based, GPT-3, originates from the “probability revolution” and the “statistical revolution” in the history of scientific development. Living in an uncertain universe, humanity has an eternal pursuit of certainty. In the 17th century, Newtonian physics was born. It told us that everything is absolutely governed by a universal law, just as the collision of one billiard ball inevitably leads to the movement of another. This relieved humanity, who had always been anxious about their fate. “Newtonian physics reigned from the end of the 17th century to the end of the 19th century with almost no dissenting voices. The universe it describes is one where all things occur precisely according to laws, a meticulously organized universe where all future events strictly depend on all past events.”
However, by the end of the 19th century, a “probability revolution” erupted in physics—thermodynamics, particularly the statistical study of gases known as “Brownian motion”, fundamentally denied the absoluteness of Newtonian physics. Later, the “statistical revolution” promoted by scientists such as Willard Gibbs and Ludwig Boltzmann pointed out that the universe itself is vague, and its fundamental operating law is “chance”; thus, any physical measurement of it cannot be precise, especially since our measuring tools cannot be absolutely precise.
In 1948, Shannon published a famous paper titled “Mathematical Theory of Communication”, in which he pointed out that the so-called “information” is something that can increase probability and reduce uncertainty. For example, we are all familiar with the “guessing number” game: I think of a number for you to guess and say that this number is within 100; you cannot guess it; I then say it is within 50, and you still cannot guess; I then say it is a prime number within 10 (2, 3, 5, 7), and you still cannot guess; finally, I say it is even, and you determine that the number is 2. In the game, the hints I provide each time increase the probability of you guessing the answer or reduce the uncertainty. These hints are information.
“Naturally, no communication theory can avoid the language issue. In fact, language, in a certain sense, is synonymous with communication itself.” Analyzing English through information theory, we find a law known as the “Markov process”, which explains why the next letter in English probabilistically depends on the previous letter, and the more we know about the preceding letters, the more we can probabilistically determine what the next letter is. For example, in English words, the letter q is always followed by u, so we face zero uncertainty in predicting the letter after q. According to Shannon’s own research in 1951, the information required to determine what the first letter of a string of English letters is (to eliminate uncertainty) is 4.1 bits, the second letter requires 3.6 bits, the third letter 3.3 bits, and the 100th letter only requires 1.3 bits. This means that the context of letters (and by extension, words, paragraphs, texts, and disciplinary knowledge) can enhance our ability to judge what it “is” and what it “means”. This is akin to how humans process information when reading newspaper articles: we always understand their precise meanings based on the context (relationships) of words, sentences, and paragraphs, thus understanding the entire article. For instance, the meaning of “hot dogs” is completely different in the sentences “Hot dogs should be given plenty of water” and “Hot dogs should be eaten with mustard”, but we can determine its meaning in this sentence based on the context in which it appears.
The “probability revolution” and information theory have greatly propelled the development of artificial intelligence research. The latter gradually abandoned the pursuit of absolute precision and certainty. Before GPT-3, there were two approaches to achieving AI that pursued precision: “simulating reality” and “reducing reality”. The “simulation reality” faction believes that AI must accurately model reality (such as the human brain) and make precise predictions based on it, but the real world is rich and colorful, making precise replication difficult, so this path is far from realization. The “reduced reality” faction believes that because reality is too complex to be precisely replicated, it can only be reduced; however, the reduced reality is not reality itself, which complicates AI’s ability to predict reality. The approach of GPT-3 abandons the pursuit of precise simulation, acknowledges the vagueness of the world, and, based on the high probability of human language practices, combines powerful computing power, massive data, and human-machine collaborative training to produce a “probabilistic language model” GPT-3 and its human-machine dialogue application ChatGPT.
GPT-3’s probabilistic analysis of language is achieved through a deep learning model based on a neural network called a “transformer”. The “transformer” is a specific type of neural network architecture typically used for natural language processing (NLP) tasks. It was first proposed in a paper by Vaswani et al. in 2017 titled “Attention Is All You Need”. The model uses a “self-attention” mechanism to process the word order of the “input” and make predictions, then produces the “output”. Similar to how humans read text, the transformer can scan and analyze the user’s prompt and vast corpora, matching various expressions based on probability, resulting in outputs that reach a natural language level.
This easily reminds us of Saussure’s “structural linguistics”. Saussure pointed out: “The value of a linguistic sign arises from its opposition to other signs within the system. Oppositional relationships can be divided into two categories: (horizontal) combinatorial relationships and (vertical) associative relationships.” The meaning of a word does not only depend on its definition but is formed through a network structure created by its relationships with other words (a dual-axis relationship composed of horizontal and vertical axes), known as a “web of meaning”. This web of meaning encompasses multiple factors such as vocabulary, grammar, and context, and these factors interact to determine the meaning of a word with different probabilities. Therefore, like Shannon and Saussure, the GPT-3 language model “transformer” believes that the meaning of a word depends on its specific usage, that is, its relationship with other words; its meaning is the sum of all these relationships. The ability of ChatGPT allows it to operate across contexts, which we might call the “existentialism of language” in GPT-3.
(2) Wiener’s Cybernetics: Artificial Intelligence Training Based on “Feedback”
Another source of ChatGPT’s intelligence is “feedback”. Norbert Wiener, the founder of cybernetics and a pioneer of artificial intelligence, believed that a common “information pattern” enables communication between humans, animals, and machines, such as the impulses in the human brain and animal neurons corresponding to the 0s and 1s of a computer. This information pattern can exist in any organic or inorganic medium, allowing them to exhibit “intelligence”; thus, whether the communicator has a body (embodiment) is irrelevant; what matters is whether they possess the ability to convey information convincingly.
Wiener also believed that if we design machines using a “feedback mechanism”, we can make machines intelligent. This intelligent machine is different from those that can only execute pre-set programs. The so-called “feedback” is “control based on the machine’s actual performance rather than its expected performance”. For example, “When I drive a car, the series of controls I perform on the vehicle does not solely depend on my prior impression of the road and my planned driving maneuvers; rather, in actual operation, if I find the vehicle veering too far to the right, this realization will prompt me to steer it back to the left. This control depends on the vehicle’s actual performance, not merely on the prior road conditions and my plans. It is this real-time control method that allows me to drive a lightweight Austin car or a heavy truck with roughly the same efficiency without needing to develop different driving habits for each.” He said, “If machines are to achieve this real-time and automatic control like humans, they need to use various sensory components that perform the functions of predictors or monitors; these mechanical forms of feedback processes can also be accomplished through electrical means or even vacuum tubes. These methods may become standard approaches for designing control instruments in the future.” Wiener believed that if machines continuously provide feedback on their actual performance, they could learn and improve over time, becoming increasingly intelligent, much like humans do through trial and error.
Wiener’s “feedback” later became a fundamental principle of all artificial intelligence, and ChatGPT is no exception; its “intelligence” is based on the self-supervised feedback principle of “mutual combat and adversarial iterative learning”. This is reflected firstly in that GPT-3 is “pre-trained” through self-feedback and human-machine feedback principles. Earlier deep learning models were “Generative Adversarial Networks (GAN)” that learned to enhance the quality of AI content production through the adversarial learning of two modules, the generative model and the discriminative model. GPT-3, released by OpenAI in 2020, is more advanced than GANs, enabling AI to realistically mimic human “speech” for the first time, but it still lacked discipline and often produced outrageous, coarse, and erroneous statements. To avoid such embarrassment, the lab introduced a “Reinforcement Learning from Human Feedback (RLHF)” mechanism, which uses human feedback signals to reinforce learning in the language model. In training, human teachers provide AI with positive and negative stimuli (Reward Model)—for human questions, a good answer receives a high score, while a poor one receives a low score. Based on this, the lab also automated the training methods of human teachers, allowing AI to learn through self-reinforcement, ultimately forming GPT-3.5 and ChatGPT based on it. Secondly, as previously mentioned, ChatGPT learns from human users’ stimuli (questions) and satisfaction indicators to improve communication accuracy and dialogue sense. From the perspective of cybernetics, this represents the intelligence demonstrated by ChatGPT’s dynamic feedback based on its “actual performance”.
Social behaviorist Herbert Mead pointed out that the “self” develops from social processes and can never be isolated or severed from this process, which is actually a series of “conversations of gestures” or social feedback. It is evident that if ChatGPT possesses “intelligence” or even a “mind or self”, it is achieved through the principles of probability and feedback.
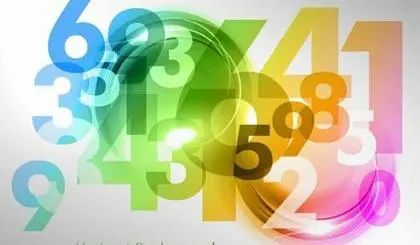
2
ChatGPT Is An Efficient Simulation Of Humanity’s
Probabilistic Language Practices
GPT-3 efficiently provides the most probable combinatory content as answers based on the analysis of human language practices through probability and feedback from vast texts. The “most probable combinatory content” in human language is essentially various habitual expressions, existing in forms such as words, phrases, sentences, paragraphs, and even disciplinary knowledge. For example, in the sentence “I like the feeling of [CD#2]”, the likelihood of filling the blank with “Sichuan cuisine” is far higher than “being laughed at”. In middle school English exams, we are also trained like ChatGPT—doing cloze tests based on probability. Thus, some critics argue that the content generated by ChatGPT is not remarkable innovation but merely a transportation and combination of existing human language content.
This criticism is not new. We all admire innovative language and detest the ugly clichés. For instance, the poet Du Fu said, “Poetry should be fresh and have new ideas”, meaning that poetry must be written freshly, and first and foremost, it must have new ideas. Unbeknownst to many, typical images and allusions in ancient Chinese poetry, such as the lonely moon, long night, autumn water, setting sun, long river, withered vines, dusk crows, small bridges, and flowing water, are a form of probabilistic language that has been repeatedly combined and used by poets throughout the ages, thus contributing to the high intertextuality and long-standing nature of Chinese culture.
In the West, the foremost advocates against clichéd language and for literary innovation were the Romantic Movement of the mid-18th century. This movement valued emotion, advocated the creative exercise of imagination, and insisted on aesthetic standards prioritizing beauty, with William Wordsworth as its main representative. He vehemently opposed clichéd language. Upon seeing a contemporary poet Thomas Gray’s elegiac sonnet, he crudely crossed out nine lines he deemed “clichéd”, pointing out that only the remaining five lines had any value. These nine lines were filled with expressions such as “a friend with feathers” (bird), “smiling morning”, “golden flame”, “cheerful fields”, and “longly anguish”. In fact, similar to the use of allusions in ancient Chinese poetry, many Western poets in the 18th century believed that using these idioms was essential to true poetry. However, the Romantic representative Wordsworth believed that poetry is the personalized expression of the artist; “the better the poet, the more unpredictable the expression in their poetry”.
Another famous figure opposing clichéd language in the West was George Orwell. In his 1946 essay “Politics and the English Language”, he pointed out that clichéd writing makes it feel as if the author is “sticking together a long list of words arranged by others”. He also criticized the British government’s “Newspeak” in “1984”—various corruptions of language, including the “dying metaphors” and “meaningless words”.
But if we remove the value judgment, what Du Fu, Wordsworth, and Orwell opposed as “clichés” are essentially the probabilistic expressions used by ChatGPT today, which have long been an important feature of human language practice. Limited by the bandwidth of human brain memory, using high-probability “clichés” is the fate of human language. In fact, even our opposition to “clichés” has long become a highly repeated cliché itself—merely accusing it of lacking novelty and hindering innovation.
We can also trace the history of probabilistic expressions in human language back to even older times. In the 1930s, a 30-year-old Harvard professor named Milman Parry published a paper in the “Harvard Studies in Classical Philology”, pointing out that the representative work of the oral era, “The Iliad”, consists of tens of thousands of lines, but it was not created by a single individual named “Homer”; rather, it was formed by generations of anonymous Greek bards assembling numerous prefabricated, component-like fixed expressions. Therefore, Parry believed that the bard “Homer” was more like an assembly worker on a production line than an innovative artist. In February 2023, Google announced the launch of its own ChatGPT-like application named “Bard”, which reveals this high-probability content production method of AI intelligence akin to that of “Homer”.
American literary and media researcher Walter Ong’s insights into the relationship between oral culture and written culture provide important inspiration for our understanding of the relationship between language and technology (including today’s ChatGPT). In his book “Rhetoric, Romantic Story, and Technology”, he pointed out that the formulaic and modular expressions (probabilistic language) we today consider clichéd have actually been a major characteristic of human oral and written practices since ancient times. Before the advent of writing 5,000 years ago, in oral culture, “knowledge, once acquired, had to be constantly repeated, or it would be lost. At this time, using formulaic and modular expressions (probabilistic language) was crucial for effectively preserving and managing human experience and wisdom.” For example, both Eastern and Western minority epics contain such phrases: soldiers are always referred to as “brave soldiers”, and oaks are always referred to as “sturdy oaks”; folklore also uses rhyming mnemonics like “One, three, five, seven, eight, ten, never wrong; four, six, nine, winter is a small month, only February has twenty-eight” to memorize the days in large and small months.
Both Parry’s and Ong’s studies tell us that the regularity and repetitiveness of human language are much higher than we imagine compared to their specificity and originality. Later, the emergence of writing and the invention of the printing press in the 15th century significantly reduced the influence of oral probabilistic expressions, as writing, being an “extended mind”, has stronger storage and transmission capabilities than probabilistic expressions. However, probabilistic language has not completely disappeared; instead, it has been fixed in written language, such as the idioms and habitual expressions widely used in Chinese, as well as various fixed expressions in English, such as “tried and true”, “sudden change”, and fixed expressions like “Once upon a time…” or “It was a dark and stormy night…”. As for narrative structure, structuralist narratology, represented by Vladimir Propp and Claude Lévi-Strauss, has eloquently proven the various structures that have been repeatedly manifested in human narratives for thousands of years.
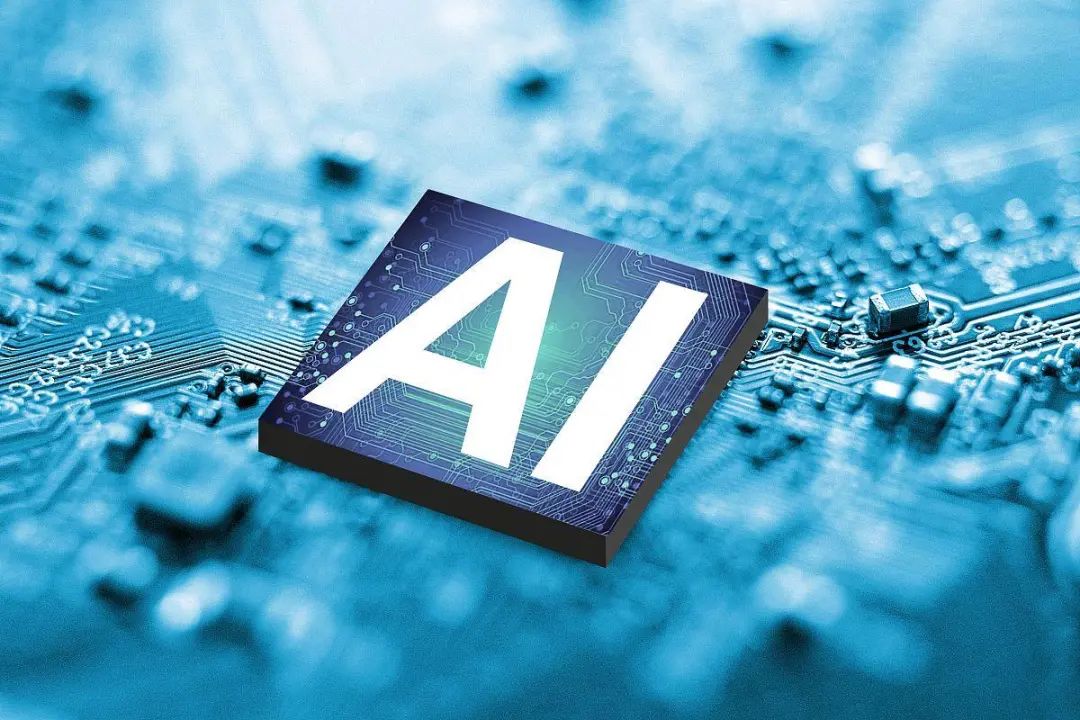
3
ChatGPT’s Collage-Based Creation
And The Challenges It Brings
However, if ChatGPT is merely based on probabilistic references and collages of existing human corpora, why do many users still feel that its responses are humorous, somewhat engaging, and even creative? Can ChatGPT “create” (like humans)?
(1) Is ChatGPT’s Collage Creation Really Creation?
This is essentially a Turing problem—can machines think like humans? In fact, today’s artificial intelligence writing has already reached a level where human users cannot distinguish it from human works, meaning it has long passed the “Turing test”. If so, according to Turing’s perspective, debating whether “machines can think (create) like humans” becomes less meaningful.
On the other hand, answering this question also depends on how we define “creation” and how we judge whether a work has creativity. It is generally believed that “in essence”, computers and algorithms are created by humans; they are “human creations” (the created) rather than “creators” (the creator), lacking the key elements that embody creativity: autonomy, intentionality, consciousness, values, emotions, and the ability to establish the “unknown” beyond the “known”. Accordingly, we cannot say that ChatGPT possesses creativity.
However, in terms of its actual performance, GPT-3 indeed exhibits a degree of creative ability. For example, its performance as a “general language model” has surpassed that of specialized language models (such as grammar correction models, question-answering models, and translation models), indicating its high adaptability to various tasks, which is one manifestation of creativity. Secondly, in addition to completing traditional patterned writing, GPT-3 can create novels, poetry, drama, computer code, and even fabricate expressions that did not previously exist. For instance, an English user told GPT-3 that “to screeg something” means “to swing a sword at it” and asked it to use the word in a sentence; GPT-3 provided a decent answer: “We screeghed at each other for several minutes and then went outside and ate ice cream.”
However, a more fundamental question we should ask is: there are various ways to create; is “collage” not one of them? As one internet user commented: “Humans are also finding meaningful combinations from a vast space composed of various elements, creating things called works and designs.”
I asked ChatGPT, “Can collage be regarded as a means of creation?” It answered affirmatively:
“Yes, collage can be seen as a means of creation. Collage is an art form that involves combining different materials and objects, such as paper, photos, and fabrics, to create a new unified whole. This process allows you to experiment with different textures, colors, and shapes and explore new ideas and themes. Collage provides a low-risk way to experiment and use different materials and try new ideas. It can help you expand your imagination and develop your visual vocabulary. By using materials in new ways and combining them unexpectedly, you can create unique and original works that reflect your personal vision and style. Overall, collage is a fun and accessible way to engage your creative thinking and explore new ideas and themes. Whether you are an experienced artist or a beginner, collage is an excellent method to unleash your imagination and let your creativity flow.”
If ChatGPT states that “collage is creation”, there may be a conflict of interest, and the evidential power is insufficient. As another piece of evidence, in human culture, “collage” has indeed yielded numerous innovative outcomes.
The “imagery montage technique” favored by Walter Benjamin—using surrealistic collage methods and cinematic montage techniques, or even constructing a work entirely from quotations, as in his “Arcade Project”; Qian Zhongshu’s “Guan Zhuan Pian” is a collection of short essays mostly composed of parallel and simple comments on similar or contrasting viewpoints from Chinese and Western literature, history, and philosophy, but this book is regarded as a pioneering work in comparative literature research between China and the West. The media philosophy work “Cloud: Media Is Existence” by American communication scholar John Peters is based on the viewpoints of up to twelve disciplines and numerous previous technical thinkers, with content that is vast and encyclopedic, yet highly regarded in the field of media studies.
ChatGPT’s “collage-style output” also reminds us of various modernist literary experiments, such as the French literary group Oulipo’s numerous “innovations” by its members. For example, the French novel “La Disparition” by Georges Perec deliberately avoids using the letter “e” throughout its more than 300 pages; Jacques Jouet improvises “subway poetry” in the gaps between subway stations; Raymond Queneau’s “Cent mille milliards de poèmes” creates one hundred trillion new sonnets by recombining the verses of ten sonnets. Furthermore, the surrealist artists’ game “Exquisite Corpse”—participants add their own text without looking at the previous content, ultimately forming a text as a collaborative creation by all players, resulting in sentences that can be shocking and absurd yet innovative, such as “The exquisite corpse should drink new wine”. This is precisely what ChatGPT describes as collage-style innovation: “By combining existing materials in unexpected ways, you can create unique and original works that reflect your personal vision and style.” Additionally, we also have the “distributed encyclopedia compilation” represented by “Wikipedia”….
The situation in artistic creation is similar. Byung-Chul Han, a Korean-German philosopher, points out that contrary to the Chinese perspective, from the modular assembly production of the Terracotta Army 2,500 years ago to the “element combination” techniques in the manual of Chinese landscape painting during the Kangxi era, there is an inherent spirit of imitation and assembly in Chinese thought and culture. Han’s own writing also exhibits the multi-faceted collage characteristics akin to “ChatGPT-generated content”. He is the first Asian who can navigate through the philosophical culture of Germany or Europe without considering himself an outsider, using Japanese haiku as a writing form, Korean language as an example, and Chinese philosophy to inspire the West and diagnose the contemporary world and society.
Even in the recent imaginative Chinese science fiction film “The Wandering Earth 2”, the “villain” artificial intelligence Moss is strikingly similar to the “HAL 9000” artificial intelligence from Arthur C. Clarke’s 1968 work “2001: A Space Odyssey”. This suggests that ChatGPT’s “borrowing and collaging” of existing content has long been one of the methods of human cultural production. If such activities by humans can be regarded as creation, why can’t ChatGPT’s writing be considered creation?
(2) ChatGPT And Human Content Co-Creation
In fact, AI like ChatGPT/GPT-3 has produced a type of “generativity” based on “borrowing and collaging”; that is, it can generate original outputs (including text, images, and music) based on a set of rules, patterns, or data inputs. Artificial intelligence is transitioning from being mere retrievers, transporters, and presenters to becoming creators (creator), or at least co-creators (co-creator).
The content co-creation between ChatGPT and human users manifests in three stages. First, as previously mentioned, the content output of ChatGPT is a probabilistic utilization and collage of human corpora. Secondly, the process of content output itself is a co-creation process with human users. For instance, the quality of the content it produces largely depends on the user’s ability to pose questions (prompts). The more specific, precise, or creative the wording of the question, the more specific, precise, and creative ChatGPT’s response will be. A Chinese user suggested that to enhance ChatGPT’s creative novel writing ability, “As long as you add as many precise descriptive terms as possible about the psychology, characters, and scenes in the desired novel, ChatGPT can provide various angles of complex descriptions.” This means that the ability to ask good questions of ChatGPT has become a method to test the breadth of human users’ knowledge and language expression ability, leading to the emergence of numerous ChatGPT prompt guides online. Thirdly, after ChatGPT retrieves vast information and presents it to human users in a concise and organized manner, the latter can digest, integrate, argue, refute, rewrite, and polish the output content, ultimately elevating these collaboratively produced contents to a publishable level. Today, human authors polishing AI-generated drafts is not much different from an experienced teacher or editor polishing the articles of students or new reporters, as many journalists and writers have begun to collaborate with ChatGPT in content production. In 2021, American writer K. Allado-McDowell first asked GPT-3 various philosophical questions, then edited and polished the answers, resulting in a well-structured and clearly articulated book suitable for publication. Since 2000, the value of AI language models has evolved from being mere assistants for grammar and spelling checks to deep collaborative partners in writing. In the future, this human-machine co-creation relationship will become more common and deepened.
Liang Qichao said: “Articles should be revised a hundred times; indeed, a fine sentence is a hook bent”; Hemingway said, the “first draft” of anything is garbage; American writer and journalist Robert Cormier said, “The beauty of writing is that, unlike brain surgeons, you don’t have to get it right the first time.” The implication of these three authors is that good writing begins with continuous revisions of the first draft. Clearly, ChatGPT provides initial drafts for human content creation in an extremely efficient manner.
(3) Collage-Based Content Creation Challenges Existing Concepts And Systems Of “Original Authors”
Similar to the academic creations of Benjamin, Qian Zhongshu, and Peters, as well as the literary experiments of Oulipo members, ChatGPT’s collage-based writing grounded in probabilistic language and its co-creation practice with human users will complicate our accustomed concepts and systems of “original authorship”.
However, this is not a new problem. Peters pointed out that there is a clear commonality among the three philosophers Socrates, Jesus, and Confucius—they all refused to commit their teachings to writing. This was not a matter of their willingness or ability; rather, none of them had the concept of “original authorship”—a concept that only emerged in modern European culture of personal expression and copyright two thousand years later.
Byung-Chul Han, in studying China’s “shanzhai-ism”, mentioned Zhang Daqian—who can be seen as the “ChatGPT master of Chinese painting” traversing through the art metaverse in search of materials and inspiration. As “China’s Picasso”, Zhang Daqian was a famous painter and collector, owning over 4,000 paintings. However, he did not let these collections gather dust; he often took them out to appreciate and ponder, sometimes placing himself in the era and context of the ancient painters to meticulously copy them. For certain lost yet historically recorded masterpieces, he would create works as if he were the master. In this way, Zhang Daqian constantly traversed between the ancient and the modern, continuously replicating, reducing, adding, and modifying the styles of famous ancient painters in unexpected ways, producing a large number of works that are both inherited and innovative, both genuine and fake, and both true and false.
Due to Zhang Daqian’s superb painting skills and his ability to copy ancient works accurately, his imitations and forgeries are often indistinguishable from the originals, deceiving many experts. Han believes that this kind of “special practice” in art is only possible in a culture like China that does not pursue “revolutionary breaks” but is committed to “quiet reform”.
The problem posed by Zhang Daqian is that while appreciating, pondering, copying, and creating “genuine forgeries”, he dialogues with ancient people, ancient times, ancient works, himself, his era, and his own works. His “non-author-centric collage creation” ultimately makes it difficult for us to discern who the true author of his works is. Similarly, the human author engages in a form of “non-human-centric writing” with ChatGPT, entangling at various levels between human authors and non-human authors: “I” interacts with computational linguistic thinking in language modeling, interacts with AI pre-trained on internet corpora, and interacts with a vast array of internet corpora produced by anonymous humans and non-humans. So who is the author of the content generated by “me” and ChatGPT? Is it me? Is it us? Is it it? Perhaps all, perhaps none.
The future is here. After the popularization of ChatGPT or similar artificial intelligences, a large amount of AIGC will appear in our lives. Perhaps we won’t care whether certain content is written by a real person or AI, just as we do not care whether it is a person or a machine that washes our dirty dishes and clothes; perhaps we will need to distinguish the different contributions of AI and real people in AIGC to delineate the responsibilities of both, just as we need to show which parts are original and which are restored when repairing an antique vase; or perhaps we will soon need to acknowledge AI’s place in cultural production. For example, in 2019, 115 years after the famous Czech composer Antonín Dvořák’s death, an AI artist named AIVA completed a piano piece left unfinished by Dvořák. The association of authors, composers, and editors in France and Luxembourg (SACEM) accepted AIVA as a member, meaning AIVA’s works are protected by copyright law just like human works. As early as 2012, predictions were made that “in the next five years” robot journalists would receive Pulitzer Prizes, and soon we may need to revise the selection rules for the Nobel Prize in Literature.

4
Conclusion: ChatGPT May Inspire A New
“Romantic Movement” For Humanity
In summary, ChatGPT, as the most novel and powerful artificial intelligence of the 21st century, is fundamentally rooted in historical concepts: it originates from the “probability revolution” at the end of the 19th century, has undergone the influence of information theory and cybernetics in the mid-20th century, and has benefited from the explosive growth of computing power and data since the early 21st century. Its history of probability-based distributed content production seems to take us back to the era of “patterned memory and collective creation” of Homer and Confucius. The sensation brought by ChatGPT once again confirms the patterned and cyclical nature of how technology influences culture throughout human history. While this may startle us, we soon find it unsurprising. Mark Twain said, “History does not repeat itself, but it often rhymes.” The impact of new and old technologies on culture will not be entirely consistent, but it will certainly rhyme.
Suzanne Langer pointed out: “There is a so-called ‘generative idea’ in the history of thought, which can bring new perspectives, new concepts, and new frameworks to old fields.” She said, “Most new discoveries are merely a sudden realization of something that has always existed. New ideas are a light that illuminates what we have long ignored and gives these things a certain form. We shine light here, there, and everywhere, and then those things that limit thought will naturally recede. Under the impetus of generative ideas that play a foundational role, new sciences, new arts, and young and vigorous philosophical systems will emerge.” Humanity’s cultural production has long lacked such generative ideas; perhaps it is because “most people die in their twenties or thirties, for after this age, they merely become their shadows, spending the rest of their lives in imitating themselves, day after day, more mechanically and pretentiously repeating their past thoughts, loves, and hates.” In other words, most people in human society, whether actively or passively, have become machines, engaging in machine-like work.
Walter Ong believes that the emergence of the Romantic Movement in the 18th century was not to oppose the various existing technologies but precisely because these technologies made the emergence of the Romantic Movement possible. The writing, copying, and printing of that time liberated the human mind from the burdens of patterned memory in the oral era, allowing romantics to engage in more creative activities. Similarly, I believe that today ChatGPT can efficiently generate various patterned or low-innovation texts, thus freeing human minds to engage in more creative work. Under the feedback training of human companions, ChatGPT will become increasingly generative; in the future, we will be able to use ChatGPT as our training partner in the production of content involving “learning, knowledge, and talent”, fully leveraging “the human use of human beings” and continuously unleashing imagination and creativity, sparking a new “Romantic Movement” beyond artificial intelligence.
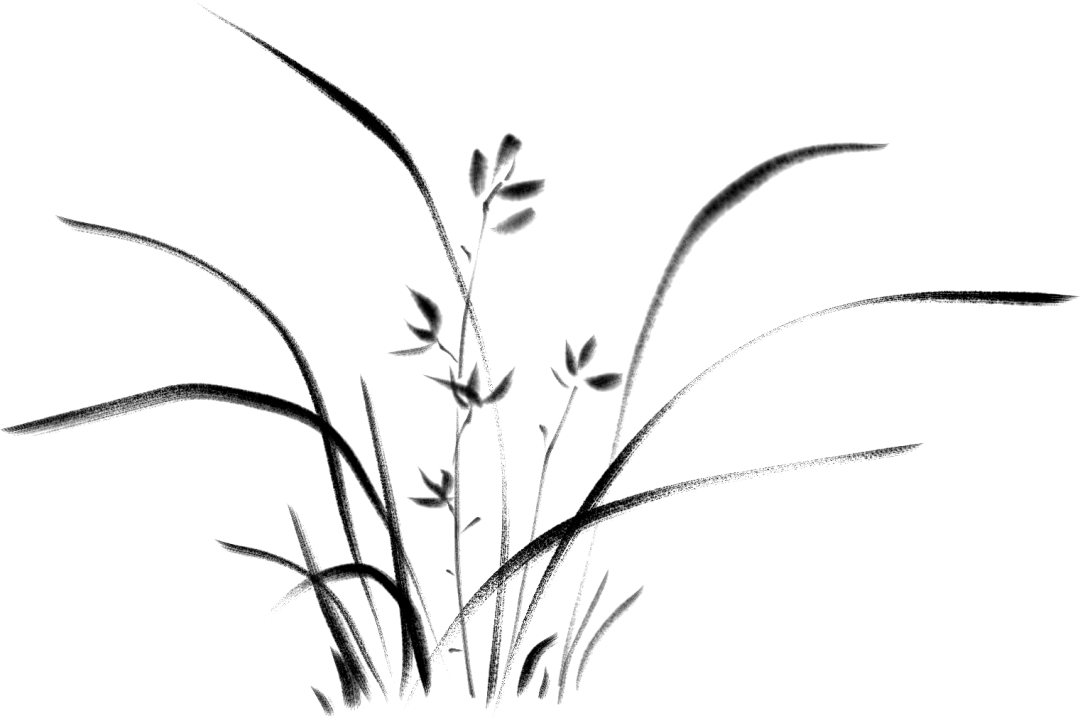
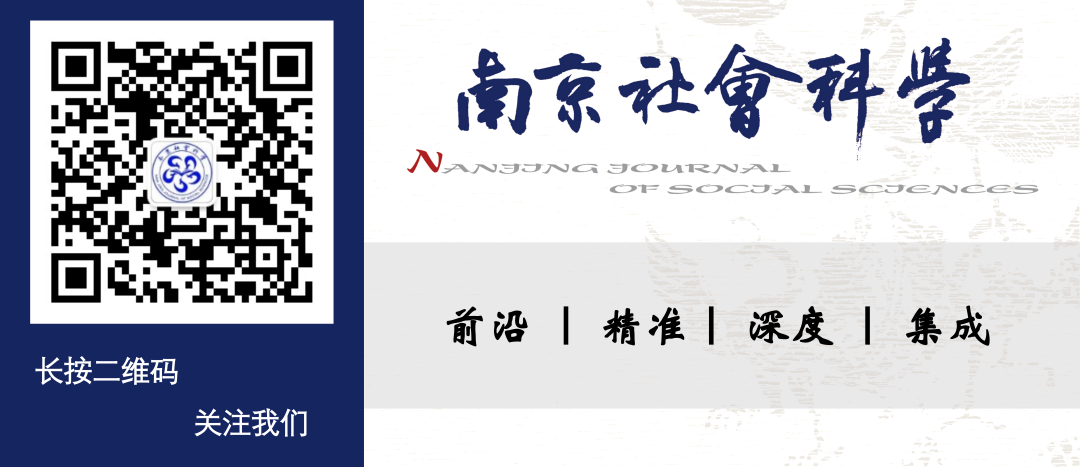
This journal insists on originality and welcomes reprints
All rights reserved. If reprinted, please indicate:
This article is reprinted from Nanjing Social Sciences
Personal forwarding is welcome
Media reprints should contact the backstage for authorization
Submission website: http://www.njsh.cbpt.cnki.net
Contact phone: 025-83611547