ChatGPT Receives Enthusiastic Market Response, Major Players Enter the Arena
Statistics show that the daily active user growth of ChatGPT far exceeds that of Instagram. In January, there were over 13 million unique daily visitors using ChatGPT, more than double the number from December last year; both domestic and international tech giants are placing great importance on the technological wave triggered by ChatGPT, actively engaging in generative AI. Domestic companies such as Baidu and Tencent are also closely monitoring ChatGPT and actively exploring cutting-edge technologies, with related deep applications set to be launched soon.
ChatGPT Has Evolved Through Various Technical Routes, Gradually Maturing and Improving
The human intentions that ChatGPT can realize come from the accumulation of various technical models, including machine learning, neural networks, and the Transformer model. After the Transformer modeling method was established, the idea of using a unified tool to develop foundational models for various modalities matured. Subsequently, the GPT-1, GPT-2, and GPT-3 models continued to evolve and upgrade, ultimately giving rise to the ChatGPT text dialogue application.
The AIGC Cross-Modal Industrial Ecosystem is Gradually Maturing, with Promising Commercial Applications Ahead
The AIGC industrial ecosystem is currently evolving and upgrading in multi-modal interaction functions such as text, audio, and video, laying the commercial foundation for multiple scenarios. Cross-modal generation technology is also expected to become a turning point for truly achieving cognitive and decision-making intelligence.
ChatGPT Rides the Wave of Opportunity, Commercial Structure Becoming Increasingly Clear
With the release of ChatGPT Plus, the curtain on commercialization has begun to rise. ChatGPT can provide significant benefits in fields such as media, film, marketing, entertainment, and the synergy between digital and real economies, enhancing productivity curves and empowering both virtual and real economies from multiple dimensions.
Market Overview: A Milestone in the Popularization of ChatGPT-AI
OpenAI Has Been Under Capital’s Spotlight Since Its Inception, Collaborating with Microsoft to Accelerate Commercialization
ChatGPT was developed by the OpenAI team, which was founded in 2015 in San Francisco by entrepreneurs Elon Musk, Sam Altman (president of the startup incubator Y Combinator), and Peter Thiel (co-founder of the global online payment platform PayPal), among others. The company is a non-profit AI research organization supported by several heavyweight Silicon Valley investors, with initial funding as high as $1 billion. OpenAI’s founding goal is to collaborate with other institutions to conduct AI-related research and to share research results to promote the development of AI technology.
OpenAI is experiencing strong momentum, with a clear trend toward commercialization
OpenAI’s ChatGPT is Part of the Generative Artificial Intelligence Technology (AIGC) Wave
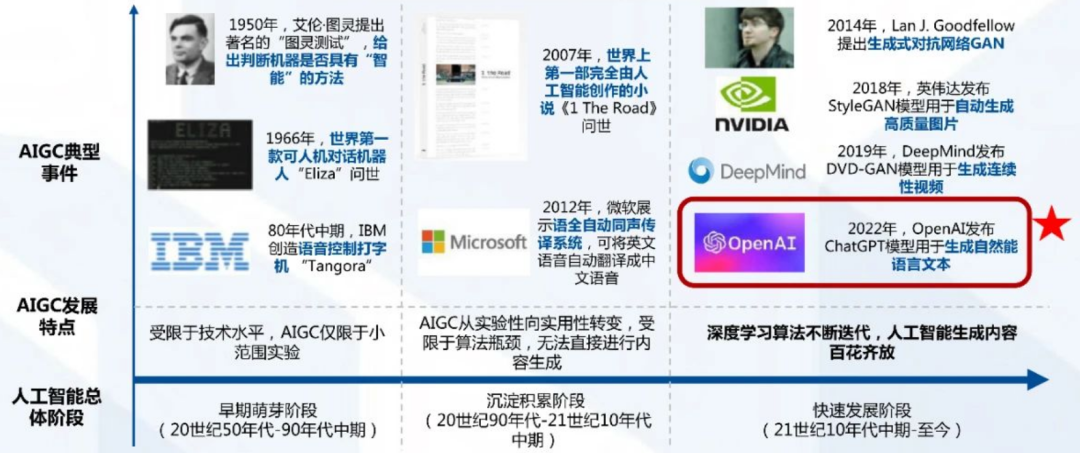
With continuous iterations of algorithms, generative artificial intelligence technology (AIGC) is constantly evolving
ChatGPT is an Advanced Natural Language Processing Model Developed Based on GPT
The GPT model is a natural language processing (NLP) model that uses multi-layer transformers to predict the probability distribution of the next word, generating natural language text by learning language patterns from a large corpus of text.
From GPT-1 to GPT-3, the level of intelligence has continuously improved, and the arrival of ChatGPT is also a prelude to the official launch of GPT-4.
ChatGPT has gradually formed through the continuous maturation of the models from GPT-1 to InstructGPT
After the Release of ChatGPT, User Numbers Have Continued to Surge, Rapidly Increasing Market Influence
According to a research report released by UBS, ChatGPT’s monthly active user count reached 100 million in January, making it the fastest-growing consumer application in history. In comparison, TikTok took nine months to reach 100 million monthly active users, while Instagram took two and a half years. Meanwhile, according to disclosures from Similar Web, Spotify accumulated only 100 million monthly active users after four and a half years.
According to Similar Web data, there were over 13 million unique daily visitors using ChatGPT in January, more than double the number from December last year.
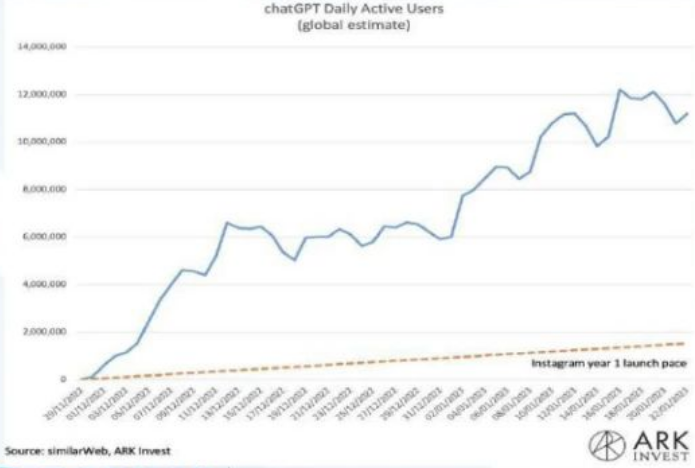
Figure 4: The growth rate of daily active users of ChatGPT far exceeds that of Instagram
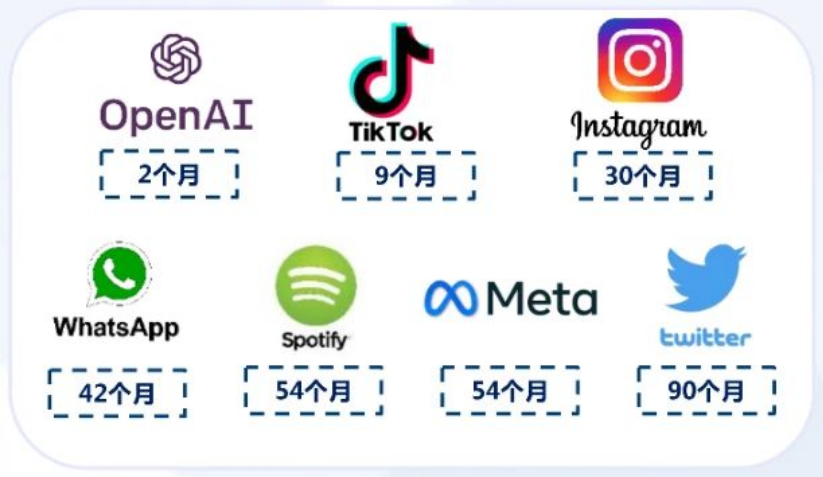
Comparing the time taken for various popular platforms to reach 100 million monthly active users, the growth rate of ChatGPT is astonishing
ChatGPT Can Cover a Wide Range of Capabilities
As ChatGPT includes data on more topics, it can handle a greater variety of niche subjects. The capabilities of ChatGPT can cover tasks such as answering questions, writing articles, summarizing texts, language translation, and generating computer code.
ChatGPT Has Many Advanced Features
ChatGPT incorporates human feedback reinforcement learning and artificial supervised fine-tuning, thus possessing many advanced features such as context understanding and coherence, unlocking a vast array of application scenarios. Currently, the dataset utilized by ChatGPT only extends up to 2021.
In conversations, ChatGPT actively remembers previous dialogue content (context understanding) to assist in responding to hypothetical questions, enabling ChatGPT to achieve continuous dialogue, enhancing user experience in interactive modes. Moreover, ChatGPT also conceals sensitive information and can provide relevant suggestions for content it cannot answer.
The core points of ChatGPT’s improvements are as follows
Tech Giants Are Continuously Investing in the AI Industry, ChatGPT Drives a New Wave of AI Development
At the beginning of 2023, both Microsoft and Google announced layoff plans but significantly increased their investments in the AI industry.
Tech giants are ramping up investments related to ChatGPT
Domestic and International Tech Giants Are Actively Engaging in Generative AI, Some Companies Already Have Established Products
Both domestic and international tech giants are placing great importance on the technological wave triggered by ChatGPT, actively engaging in generative AI.
Domestic and international tech companies are actively engaging in generative AI
Google: In Response to the Threat Posed by ChatGPT, Invests $300 Million in Competitor Anthropic
After the release of ChatGPT, Google CEO issued a “Code Red” internal alert, urging the team to address the threat ChatGPT poses to the company’s search business, while approving plans to integrate AI chatbots into Google Search.
On February 4, Google invested $300 million in ChatGPT competitor Anthropic, acquiring approximately 10% of its shares. Anthropic plans to use the Series B funding to purchase computing resources from Google’s cloud computing division; Anthropic has developed an intelligent chatbot named Claude, which is said to be comparable to ChatGPT (yet to be released). Anthropic has deep ties to OpenAI, with its co-founders having previously held vice president roles in research at OpenAI.
Microsoft: The Largest Investor in OpenAI, Begins to Utilize ChatGPT to Enhance Product Competitiveness
Microsoft views ChatGPT as a new generation of technological revolution, integrating ChatGPT into products such as Bing search engine, the Office suite, Azure cloud services, and Teams. Microsoft recently announced the launch of a premium version of its video conferencing and remote collaboration platform, Microsoft Teams Premium, where subscribers can enjoy large language model technology powered by OpenAI’s GPT to automatically generate meeting notes. This move could pose a significant impact on platforms like Zoom and Google Meet.
Amazon: ChatGPT Is Receiving Significant Attention and Is Widely Applied Across Various Job Functions
ChatGPT has already been used by Amazon across various job functions, including answering interview questions, writing software code, and creating training documents. Employees in Amazon Web Services (AWS) have reported that a small working group has been established to better understand the impact of AI on their business.
Buzzfeed, a New Media Giant in the U.S., Capitalizes on the ChatGPT Trend, Tripling Its Stock Price in Two Days
On January 29, Buzzfeed announced plans to use ChatGPT to assist in content creation, causing its stock price to surge nearly 120% overnight and over 300% within two days, with trading volume exceeding 438 million shares (its monthly average trading volume is less than 25 million shares).
Following this announcement, stocks of similar companies also saw historic highs in trading volumes: C3.ai’s trading volume exceeded 72 million shares this month, the highest since June of last year, while SoundHound AI’s trading volume was approximately 64.5 million shares, nearly three times its monthly average.
Stability AI: Stable Diffusion Gains Popularity, OpenAI Has Great Potential in Image Generation AI
Stability AI shares the same entrepreneurial philosophy as OpenAI: to build open-source AI projects to promote AI development. Its success proves that OpenAI also has great potential in the image generation field. The company’s open-source model, Stable Diffusion, can generate images based on text in just a few seconds, producing images with high resolution and clarity without losing authenticity and artistic quality.
Jasper: Utilizing Similar Underlying Technologies, Further Proves ChatGPT’s Huge Commercial Potential
The copywriting automation platform Jasper is built on OpenAI’s GPT-3, achieving a valuation of $1.5 billion just 18 months after its establishment. Major companies like IBM and Autodesk are paying customers of Jasper, demonstrating that ChatGPT’s underlying technology possesses tremendous commercial potential. After the emergence of ChatGPT, its technological leadership and popularity have posed a strong impact on Jasper.
Domestic Companies (Baidu & Tencent): Paying Close Attention to ChatGPT and Actively Exploring Cutting-Edge Technologies
Baidu:On January 10, Baidu announced it would upgrade the “generative search” capabilities of Baidu Search to intelligently answer user search queries; on February 7, Baidu announced it would complete internal testing of its ChatGPT product by March, making it publicly available under the name Wenxin Yiyan (ERNIE Bot).
Baidu pointed out that generative AI and search are complementary rather than substitutive; according to Reuters, Baidu plans to launch an AI dialogue service similar to ChatGPT as an independent application in March, gradually integrating it into its search engine thereafter.
Tencent:On February 3, Tencent announced a human-machine dialogue patent that enables natural and smooth communication between machines and users.
AIGC Startup Competition, Foreign ChatGPT’s Advantages Are Vastly Ahead and Expected to Continue
Foreign startups are involved in a wide range of AIGC product fields, with related applications maturing
AI Requires Significant Funding, Human Resources, and Data Accumulation; Domestic Giants Have More Advantages in the Market
Artificial intelligence not only requires massive investment but also a substantial user data foundation, which only internet giants have the capability to produce great products.
Foreign giants include Microsoft, Google, and Amazon, while domestic giants such as Baidu and Tencent have the most potential; compared to foreign giants, domestic giants are investing heavily in funding and human resources to rapidly develop AI technology. In the competition of AI without gunpowder smoke, Chinese companies are also expected to rise.
Technical Pathways: ChatGPT Assists in Cross-Modal AI Generation Applications Based on Human Feedback Systems
ChatGPT Has Evolved Through Various Technical Routes, Gradually Maturing and Improving
The human intentions that ChatGPT can realize come from the accumulation of various technical models, including machine learning, neural networks, and the Transformer model.
ChatGPT has formed a large-scale pre-trained language model aimed at learning from human feedback information through various technical accumulations.
ChatGPT Model Has Significant Improvements Over Previous Models
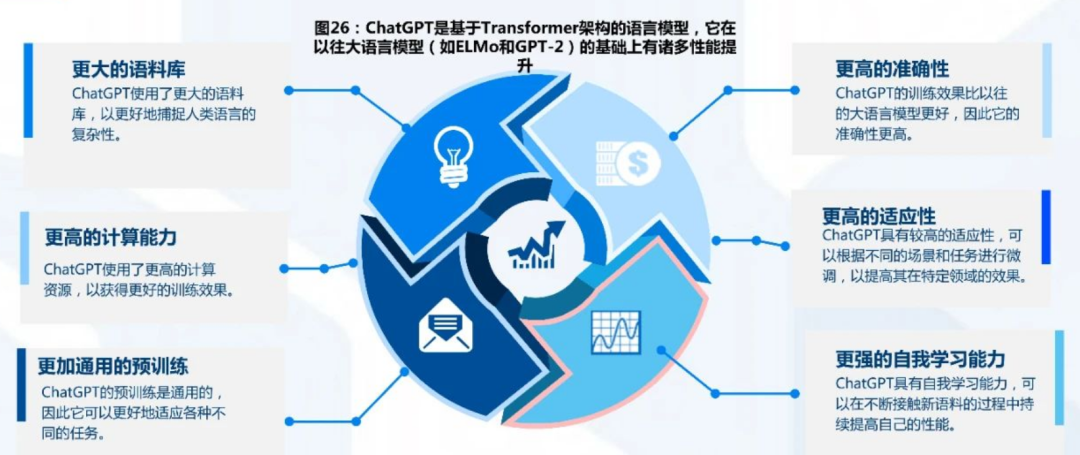
The Application of Transformers Marks the Beginning of the Foundational Model Era
Transfer Learning Makes Foundational Models Possible
Technically, foundational models are realized through transfer learning and scale. The idea of transfer learning is to apply the “knowledge” learned from one task (for example, object recognition in images) to another task (for example, activity recognition in videos).
In deep learning, pre-training is the main method for transfer learning: training a model on alternative tasks (often just a means to an end), and then fine-tuning it for the desired downstream task. Transfer learning makes foundational models possible.
Scaling makes foundational models more powerful, allowing the formation of GPT models.
Scale requires three elements:
-
Improvements in computer hardware, for example, GPU throughput and memory have increased tenfold in the past four years;
-
Development of the Transformer model architecture (Vaswani et al. 2017), which leverages hardware parallelism to train more expressive models than before;
-
And the availability of more training data.
Transformer-based sequence modeling methods are now applied to text, images, speech, tabular data, protein sequences, organic molecules, and reinforcement learning, and the gradual formation of these examples has matured the idea of using a unified tool to develop foundational models for various modalities.
For example, GPT-3 (Brown et al. 2020) has 175 billion parameters compared to GPT-2’s 1.5 billion parameters, allowing for context learning. In context learning, only prompts (natural language descriptions of tasks) need to be provided to the downstream tasks, and the language model can adapt to downstream tasks, which is an emerging property.
Transformers Set the Game Rules in the Generative AI Field
Transformers overcome the limitations of manually labeled datasets, allowing for better model quality, easier parallelization, and significantly reduced training time. Transformers have successfully demonstrated their application in both large and limited training data, making them well-suited for generalizing to other tasks.
In the paper “Attention Is All You Need” by Ashish Vaswani et al. in 2017, considering that the best-performing models were proven to be those that connect encoders and decoders through attention mechanisms, a new simple architecture was proposed in “Attention Is All You Need”—the Transformer, which is completely based on attention mechanisms without relying on recurrence and convolution. These models are superior in quality, easier to parallelize, and require significantly less training time.
After the emergence of Transformers, they quickly replaced the RNN series variants and became a mainstream model architecture (the flaw of RNNs lies in their pipeline sequential computation).
The Transformer model architecture is shown below
Different Technical Principles Corresponding to Different Technical Scenarios Implemented by Transformers
The Transformer architecture can be divided into autoregressive series (e.g., GPT-3, which prefers generative tasks), bidirectional Transformer + Masked autoregressive series (e.g., BERT, which prefers natural language understanding), and encoder-decoder architecture (e.g., T5, which uses bidirectional/unidirectional attention, preferring conditional text generation).
GPT-1: Utilizing Pre-training for Unsupervised Training and Supervised Fine-tuning
The GPT-1 model, based on the Transformer, eliminates the premise of sequential association and dependency, adopting a generative model approach that emphasizes the ability to effectively learn from raw text, which is crucial for alleviating the reliance on supervised learning in natural language processing (NLP).
The GPT (Generative Pre-trained Transformer) model was first proposed by OpenAI in June 2018. The GPT model considers that there are numerous different tasks in natural language understanding, and although there is a wealth of unmarked text corpus, the labeled data available for learning these specific tasks is scarce, making it difficult for discriminatively trained models to perform adequately. Additionally, most deep learning methods require a large amount of manually labeled data, limiting their applicability in many areas lacking annotated resources.
Given these limitations, the GPT paper demonstrates that by conducting generative pre-training on unlabeled text across various corpora and then performing discriminative fine-tuning for each specific task, significant gains can be achieved in these tasks. Unlike previous methods, GPT employs task-aware input transformations during fine-tuning to achieve effective transfer while minimizing changes to the model architecture.
Combining to form a “semi-supervised approach” for language understanding tasks using unsupervised pre-training and supervised fine-tuning
GPT-1: A More Simplified Model, Accelerated Computation, More Suitable for Natural Language Generation Tasks (NLG)
GPT has significantly simplified compared to models like Transformers.
In contrast to Transformers, GPT trained a 12-layer decoder-only model (the original Transformer model includes both Encoder and Decoder). Compared to Google’s BERT (Bidirectional Encoder Representations from Transformers), which uses context-based bidirectional prediction, GPT only employs unidirectional prediction of the next word.
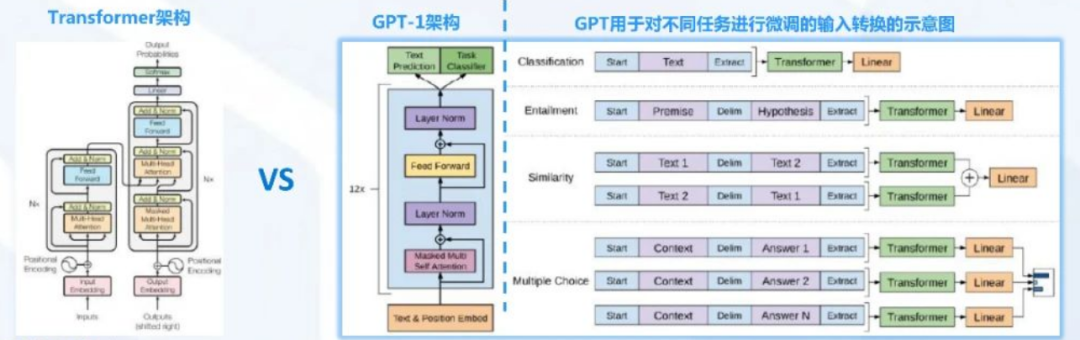
GPT-2: Adopting a Multi-task System, Optimized Based on GPT-1
GPT-2 is an improvement over GPT-1, achieving task diversity and beginning to learn to perform an astonishing number of tasks without explicit supervision. During the GPT-2 phase, OpenAI removed the supervised fine-tuning from the GPT-1 phase, becoming an unsupervised model.
The large model GPT-2 is a 15 billion parameter Transformer, achieving state-of-the-art results on 7 out of 8 tested language modeling datasets. The model stacked Transformers to 48 layers, and the dataset for GPT-2 expanded to 8 million web pages, totaling 40GB of text.
GPT-2 Has Yet to Resolve Many Bottlenecks in Applications
GPT-2 focuses on unsupervised and zero-shot learning, however, the training results of GPT-2 have not met expectations, and the existing issues still need optimization.
During the GPT-2 phase, although the architecture is task-agnostic, it still requires task-specific datasets and task-specific fine-tuning: to achieve strong performance on the desired task, it usually requires fine-tuning on thousands to hundreds of thousands of examples specific to that task.
GPT-3 Achieves Breakthrough Progress, Task Results Are Hard to Distinguish from Human Works
GPT-3 improves on GPT-2’s focus on unsupervised and zero-shot learning features, leveraging filtered compressed text from 45TB, achieving strong performance across various NLP datasets.
GPT-3 is an autoregressive language model with 175 billion parameters, ten times more than any previous non-sparse language model. For all tasks (testing its performance in few-shot settings), GPT-3 is applied without any gradient updates or fine-tuning, specifying tasks and few-shot demonstrations solely through text interaction with the model.
GPT-3 has strong performance across many NLP datasets (including translation, question answering, and cloze tasks), as well as tasks requiring dynamic reasoning or domain adaptation (such as interpreting words, using a new word in a sentence, or performing three-digit arithmetic). GPT-3 can generate samples of news articles that are increasingly difficult to distinguish from those written by humans.
InstructGPT Model Further Enhances on the Foundation of GPT-3
InstructGPT uses a reinforcement learning framework from human feedback (RLHF) to fine-tune large language models, thereby achieving superior functionality compared to GPT-3 with fewer parameters.
Background of InstructGPT:Simply increasing the size of language models does not mean they will better follow user intentions; for example, large language models can generate outputs that are untrue, toxic, or unhelpful to users, meaning these models are inconsistent with their users. Additionally, while GPT-3 employs few-shot learning and continues to adhere to the unsupervised learning of GPT-2, its few-shot effects are slightly inferior to supervised fine-tuning.
Based on this background, OpenAI trained a reward model to train the learning model (i.e., the idea of AI training AI) based on the RLHF framework on top of GPT-3.
Training Steps for InstructGPT:Supervised fine-tuning of GPT-3 – Train reward model – Reinforcement learning optimization (the second and third steps can be selected for multiple iterations)
Core Technical Advantages of ChatGPT: Enhancing the Accuracy of Understanding Human Thought
InstructGPT and ChatGPT belong to the same generation of models, with ChatGPT simply adding chat attributes to InstructGPT and opening public testing.
The reason ChatGPT enhances the accuracy of understanding human thought is that it utilizes a system trained on human feedback data.
ChatGPT Benefits from a New Paradigm of AI Systems Built on Foundational Models
Foundational models integrate methods for building machine learning systems across a wide range of applications, providing a powerful leverage for many tasks.
“Foundational models are evolved from deep neural networks and self-supervised learning. Any model trained on broad data (usually using large-scale self-supervision) that can adapt (e.g., fine-tuning) to a wide range of downstream tasks, examples include BERT (Devlin et al.), GPT-3 (Brown et al. 2020), and CLIP (Radford et al. 2021). Machine learning homogenizes learning algorithms (e.g., logistic regression), deep learning homogenizes model architectures (e.g., convolutional neural networks), while foundational models homogenize the models themselves (e.g., GPT-3).
The development of artificial intelligence shows a process of homogenization
ChatGPT Leverages Foundational Models to Be Applicable to Various Downstream Tasks
ChatGPT adopts the GPT-3.5 (InstructGPT) large-scale pre-trained model, achieving significant performance improvements in natural language understanding and content generation.
Considering the limitations of traditional NLP technologies, large language models (LLMs) help to fully utilize massive amounts of unlabeled text for pre-training, thus allowing large text models to have better understanding and generation capabilities even in smaller datasets and zero-data scenarios. The lack of standardized text collections allows ChatGPT to excel in sentiment analysis, information extraction, and reading comprehension tasks.
As the amount of training data increases, the variety of data gradually enriches, and the increase in model scale and parameter count will further enhance the model’s semantic understanding and abstract learning capabilities, achieving ChatGPT’s data flywheel effect (using more data to train better models attracts more users, thus generating more user data for training, creating a virtuous cycle).
Research shows that each increase in parameters brings improvements in text synthesis and/or downstream NLP tasks, with evidence indicating that log loss is closely related to many downstream tasks, and with increasing scale, log loss shows a stable improvement trend.
ChatGPT’s Large Model Architecture is Also an Inevitable Product of the Development of ML to Its Third Stage
The computational history in ML is divided into three eras: pre-deep learning era, deep learning era, and large-scale era; in the large-scale era, the demand for training advanced ML systems is rapidly increasing.
The progress of modern machine learning (ML) is guided by three fundamental factors: advancements in computation, data, and algorithms. Before 2010, the growth of training computation followed Moore’s Law, roughly doubling every 20 months. Since the early 2010s with the advent of deep learning, the scale of training computation has accelerated, roughly doubling every six months. By the end of 2015, with the development of large-scale ML models, the demand for training computation increased by 10 to 100 times, leading to a new trend—the rapid growth of demand for training advanced ML systems. Around 2015-2016, a new trend of large-scale models emerged, which began with AlphaGo at the end of 2015 and continues to this day (GPT-3 emerged in 2020).
Industry Progress: AIGC Multi-Modal Interaction Functions Continue to Evolve, Laying the Foundation for Commercial Applications Across Multiple Scenarios
AIGC: Utilizing AI to Generate Content, Enhancing Productivity Curves
AIGC: Artificial Intelligence Generated Content, which can utilize AI technology to automatically generate content, commonly seen in code generation, text Q&A, etc.
ChatGPT Has Become an Important Part of the AIGC Function Matrix
ChatGPT is a significant component of the AIGC “Digital Content Intelligent Editing” function, and the emergence of the ChatGPT model is crucial for text/audio modality AIGC applications.
With rapid breakthroughs in deep learning technologies and the massive growth of digital content, AIGC-related technologies have broken the limitations of predefined rules, making it possible to quickly, conveniently, and intelligently output multi-modal digital content.
Under continuous breakthroughs in technological innovation and multi-modal models, AIGC can include three main practical functions in order based on different functions and targets: digital content generation, intelligent editing of digital content, and intelligent creation of digital content. These three functions are interwoven and combined, allowing AIGC products to possess creative potential that surpasses human capabilities.ChatGPT is an important component of the intelligent editing of digital content in the AIGC function domain.
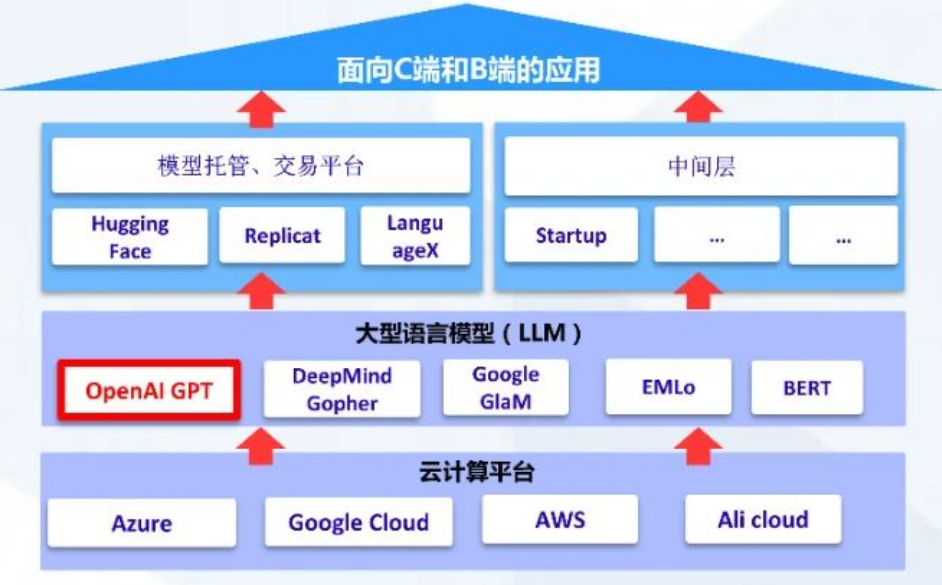
ChatGPT is a major component of the product application framework of AIGC’s large language models
AIGC-Related Technologies Include Three Major Cutting-Edge Capabilities
Digital Content Generation Capability Constructs a Mapping from the Real World to the Virtual World
Generation capability includes intelligent enhancement and translation technologies, where enhancement technology compensates for information loss during the digitalization process, and translation technology presents content in various forms based on understanding.
Digital Editing Capability Opens Up Interaction Channels Between the Real World and the Virtual World
Editing capability includes intelligent semantic understanding and attribute control, where semantic understanding helps achieve the separation of various attributes of digital content, and attribute control allows for precise modification, editing, and secondary generation of attributes based on understanding, ultimately feeding back to the real world, forming a generation-feedback closed loop.
Digital Creation Capability Moves from Data Understanding to Data Creation
Creation capabilities can be divided into imitation-based creation and concept-based creation, where the former is based on the data distribution of a particular type of work, while the latter learns abstract concepts from massive data and creates content that does not exist in the real world based on those concepts.
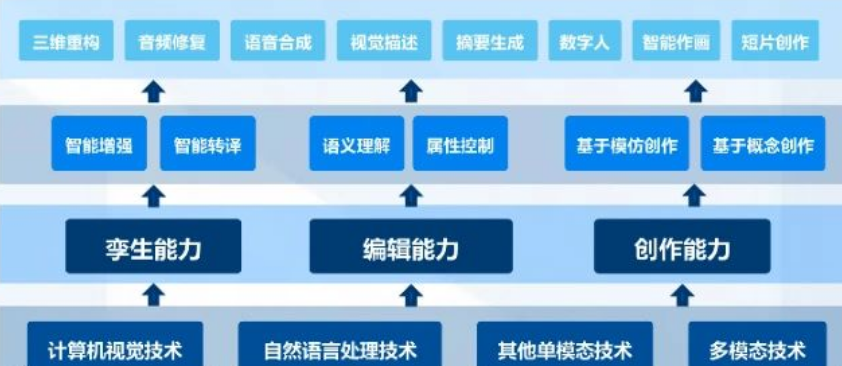
The three cutting-edge technological capabilities of AIGC are illustrated in the diagram below
The AIGC Industry Has Gone Through Three Main Periods of Development
The development of AIGC has gone through the early germination, sedimentation accumulation, and rapid development stages after 2014.
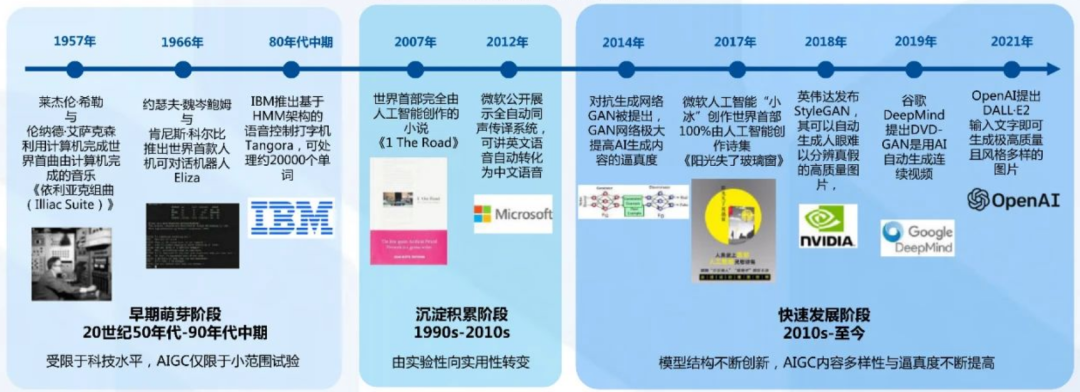
AIGC has undergone roughly three stages of evolutionary development
From Analytical AI to Generative AI Gradually Evolving, Generative AI Empowers AIGC with Innovation
Generative AI originated from analytical AI, and the technological accumulation during the development of analytical AI laid the foundation for the emergence of generative AI.
Analytical AI’s knowledge is limited to the data itself, while generative AI can generate samples that do not exist in the data based on summarizing and generalizing data knowledge. The latest generative AI technologies such as GANs and Diffusion have spawned various AIGC products like the OpenAI series, DALLE2 (Diffusion), and Stability AI (based on GAN).
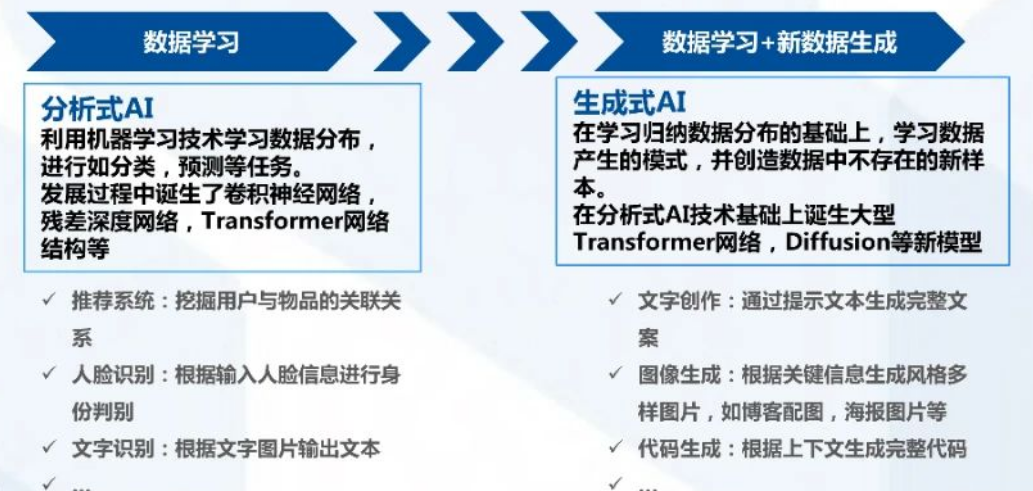
AIGC is based on analytical AI, learning data generation patterns to create new sample content
AIGC: Updates in Learning Paradigms Lay the Foundation, Model Structure Upgrades Assist in Takeoff
AI technology promotes the continuous development of the AIGC industry, where updates in learning paradigms give AI models the ability to learn actively, and upgrades in model structures enhance AI models’ learning, summarizing, and innovative capabilities.
The upgrade and iteration of AI models lay the foundation for the leapfrog development of AIGC performance
The AIGC Industry Chain Covers a Wide Range of Areas from Hardware to Various End Applications
The AIGC-related industry can be divided into application layer, model layer, cloud computing platform, and computing hardware layer
The computing hardware layer, combined with the cloud computing platform, provides machine learning training and inference power for AIGC, where GPUs and TPUs are core hardware. Major participating vendors include NVIDIA (GPU) and Google (TPU); cloud platform vendors include AWS, GCP, Azure, and Coreweave. In the computing hardware layer, cloud computing platform vendors are stable, with competition emerging at the model and application levels.
At the model level, closed-source foundational model providers like OpenAI provide services to users via APIs, while open-source foundational models make model weights public on hosting platforms like Hugging Face and Replica. The high computational power demands for model training drive collaborations between model layer vendors and cloud computing vendors, such as OpenAI + Azure and GCP + DeepMind.Closed-source models are more common at the model level, with vendors relying on models to establish technological barriers.
The AIGC market framework can be divided into infrastructure layer, model layer, hosting platforms, and application layer
Upstream and Downstream Players in the AIGC Industry Are Flourishing
The upstream of AIGC mainly includes data suppliers, algorithm institutions, creator ecosystems, and underlying cooperative tools, while the midstream includes text, image, audio, and video processing vendors, with numerous players, and the downstream mainly consists of various content creation and distribution platforms and content service agencies.
Classification of upstream and downstream participants in the AIGC industry chain is shown in the diagram below
Competition Among AIGC Vendors Lies in the Model Layer
Ultimately, AIGC relies on underlying machine learning models to generate content, so the model is where AIGC industry vendors’ true competitiveness lies.
Text generation products largely depend on the GPT series models, while image/video modality products typically have their own trained models (image/video modality products usually have their own trained models instead of calling OpenAI’s model services for text modality).
In comparison, OpenAI has established a first-mover competitive advantage based on its models, with relatively outstanding technology-to-product conversion.
There is fierce competition among AIGC model products
AIGC Can Complement Each Other and Is Expected to Become the Mainstream Content Production Model
The development of the content production ecosystem belonging to AIGC has gone through four stages: expert-generated content (PGC), user-generated content (UGC), AI-assisted content production, and AI-generated content (AIGC). Currently, it is in a situation where stages one and two are dominant, with stage three as a supplement.
AIGC overcomes the shortcomings of PGC and UGC, which cannot balance quality and quantity, and it is expected to become the mainstream content production model in the future.
The theoretical evolution of the AIGC content production model will undergo four development stages
AIGC Generation Technologies Can Be Classified by Modality
AIGC can be divided into text, video, image, audio, and cross-modal generation based on its content modality
AIGC Different Modalities Correspond to Various Generation Technologies and Application Scenarios
AIGC different modalities correspond to various generation technologies and application scenarios, each with its own subcategories.
The characteristics and subcategories corresponding to various AIGC technology application scenarios are illustrated in the diagram below
AIGC Text Generation Technology Scenarios Can Be Divided into Interactive and Non-Interactive
In non-interactive text generation technologies, structured writing has a relatively fixed form, generating relatively low difficulty, and is widely applied commercially; while creative writing has greater openness, posing higher difficulty in long text generation, requiring further technological development.
With the development of communication and internet technologies, online social demands are rapidly increasing, leading to a quick development of interactive text products like chatbots.
The relevant sub-characteristics of the text content production field are described in the diagram below
AIGC Text Generation Technology Commercialization is Expected to Have First-Mover Advantages
The pre-training large model technology in the text field is mature, with a wide variety of subcategories in the text field, leading in the number of products, and the number of models has surpassed that of other modality technologies. In digital content, text modality data far exceeds that of images/videos/audios, with relatively larger development prospects.
Text generation functionalities based on GPT-3 have been embedded into software such as Writesonic, Conversion.ai, and Copysmith, with relatively clear commercialization prospects.
Text generation technology commercialization has comparative advantages
AIGC text modality technology (including text and code) is leading in commercialization over video/image modality technologies
AIGC Image Generation Technology Has Significantly Improved with Model Structure Optimization
The continuous evolution of model structures has improved the diversity of images produced by AIGC, but the implementation of higher demanding functionalities still awaits further technological enhancement.
Image editing is easier than image generation and 2D-3D conversion, and many products currently support “image editing.” However, for “image generation” tasks, due to the greater number of elements in images, the generation effects still show instability, and higher-demand functional image generation still requires technological improvements.
AIGC Audio Generation Technology Is Evolving Toward More Emotion and Human Features
Text-to-speech tasks are relatively mature, with speech quality reaching natural standards, and future developments will focus on more emotional and rhythmic speech synthesis and small-sample speech learning.
In music generation tasks, the issue of difficult music data annotation still needs to be resolved. The granularity of data annotation affects the controllability of music generation tasks. If controllability can be resolved, music generation tasks specifying style, emotion, and other factors are expected to see widespread applications in scenarios like film and games.
Video Generation is a High-Potential Scenario in the AIGC Application Ecosystem
Video generation is essentially similar to image generation, involving frame-level segmentation of videos to process each segment.
The video generation process includes three stages: data extraction, training, and conversion. Current technology focuses on improving accuracy and real-time performance for video modifications. Given the comprehensive attributes of text, images, and audio in videos, video generation is also an important application scenario in the cross-modal generation field.
Cross-Modal Generation Technology is a Turning Point for Truly Achieving Cognitive and Decision-Making Intelligence
Information in the real world is a comprehensive system of text, audio, visuals, sensors, and various human sensations. To simulate the real world more accurately, it is necessary to bridge the capabilities of various modalities, such as text-image and text-video cross-modal generation capabilities.
The development of large pre-trained models has gradually matured cross-modal capabilities, with