vLLM
python3 -m vllm.entrypoints.api_server --model ckpt/FlagAlpha/Llama2-Chinese-13b-Chat/
python3 benchmark_serving.py --dataset ShareGPT_V3_unfiltered_cleaned_split.json --tokenizer ckpt/FlagAlpha/Llama2-Chinese-13b-Chat/
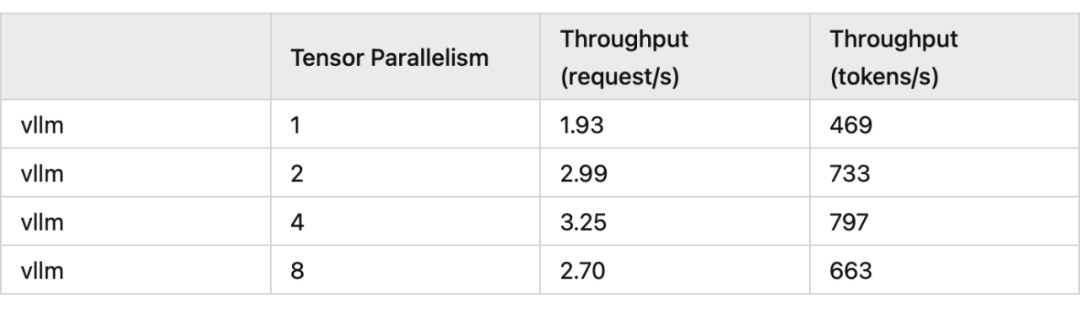
Text Generation Inference
-
Continuous batching similar to vllm -
Support for flash-attention and Paged Attention. -
Support for Safetensors weight loading. -
TGI supports deploying GPTQ model services, allowing us to deploy larger models with continuous batching functionality on a single card. -
Support for deploying multi-GPU services using Tensor Parallelism, model watermarking, and other features.
docker pull ghcr.io/huggingface/text-generation-inference:1.0.0
sudo apt-get update
sudo apt-get install -y nvidia-container-toolkit
sudo nvidia-ctk runtime configure --runtime=docker
sudo systemctl restart docker
-
Dependency Installation
# If there is no network acceleration, it is recommended to add pip Tsinghua source or other domestic pip sources
curl --proto '=https' --tlsv1.2 -sSf https://sh.rustup.rs | sh
apt-get install cargo pkg-config git
-
Download protoc
PROTOC_ZIP=protoc-21.12-linux-x86_64.zip
curl -OL https://github.com/protocolbuffers/protobuf/releases/download/v21.12/$PROTOC_ZIP
sudo unzip -o $PROTOC_ZIP -d /usr/local bin/protoc
sudo unzip -o $PROTOC_ZIP -d /usr/local 'include/*'
rm -f $PROTOC_ZIP
-
If there is no network acceleration, it is recommended to modify the cargo source. Skip if there is network acceleration.
# vim ~/.cargo/config
[source.crates-io]
registry = "https://github.com/rust-lang/crates.io-index"
replace-with = 'tuna'
[source.tuna]
registry = "https://mirrors.tuna.tsinghua.edu.cn/git/crates.io-index.git"
[net]
git-fetch-with-cli=true
-
Execute installation in the TGI root directory:
BUILD_EXTENSIONS=True make install # Install repository and HF/transformer fork with CUDA kernels
-
Installation successful, add environment variables to .bashrc as follows: export PATH=/root/.cargo/bin:$PATH -
Execute text-generation-launcher –help, output indicates successful installation.
docker run --rm \
--gpus all \
-p 5001:5001 \
-v $PWD/tgi_data:/data \
ghcr.io/huggingface/text-generation-inference:1.0.0 \
--model-id /data/Llama2-Chinese-13b-Chat/ \
--hostname 0.0.0.0 \
--port 5001 \
--dtype float16 \
--num-shard 8 \
--sharded true
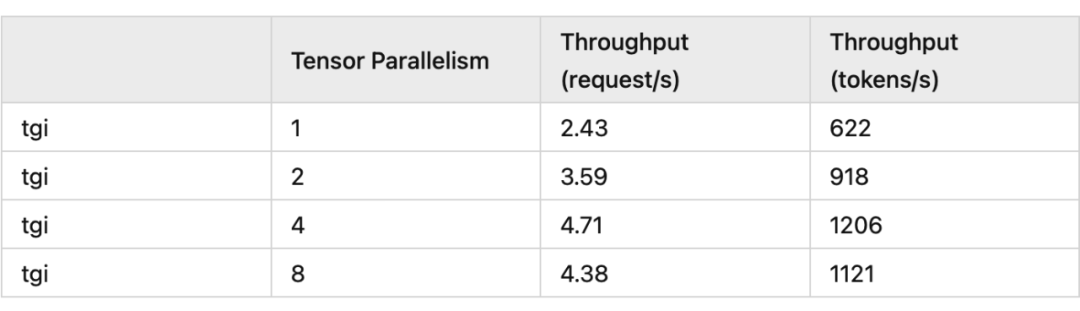
FasterTransformer
sudo docker pull nvcr.io/nvidia/tritonserver:22.05-py3
# Download the model provided by the official
git clone https://github.com/triton-inference-server/server.git
cd ./server/docs/examples
./fetch_models.sh
# Start triton server
docker run --gpus=1 --rm --net=host -v ${PWD}/model_repository:/models nvcr.io/nvidia/tritonserver:22.05-py3 tritonserver --model-repository=/models
curl -v localhost:8000/v2/health/ready
# Use docker pull to get the client libraries and examples image from NGC.
sudo docker pull nvcr.io/nvidia/tritonserver:22.05-py3-sdk
# Run the client image
sudo docker run --gpus all -it --rm --net=host nvcr.io/nvidia/tritonserver:22.05-py3-sdk
# run the inference example
/workspace/install/bin/image_client -m densenet_onnx -c 3 -s INCEPTION /workspace/images/mug.jpg
export BUILD_DICTIONARY="/data/build"
export TRITON_VERSION=22.05
cd $BUILD_DICTIONARY
git clone https://github.com/Rayrtfr/fastertransformer_backend.git
cd $BUILD_DICTIONARY/fastertransformer_backend
docker build --build-arg TRITON_VERSION=${TRITON_VERSION} -t triton_ft_backend:${TRITON_VERSION}-v-1 -f docker/Dockerfile .
docker run -idt --gpus=all --net=host --shm-size=4G --name triton_ft_backend_pure \
-v $PWD:/data \
-p18888:8888 -p18000:8000 -p18001:8001 -p18002:8002 triton_ft_backend:${TRITON_VERSION}-v-1 bash
git clone https://github.com/Rayrtfr/FasterTransformer.git
cd FasterTransformer
mkdir models && sudo chmod -R 777 ./*
python3 ./examples/cpp/llama/huggingface_llama_convert.py \
-saved_dir=./models/llama \
-in_file=../Llama2-Chinese-13b-Chat \
-infer_gpu_num=1 \
-weight_data_type=fp16 \
-model_name=llama
mkdir triton-model-store
cd triton-model-store/
cp -r fastertransformer_backend/all_models/llama triton-model-store/
# Modify triton-model-store/llama/fastertransformer/config.pbtxt
parameters {
key: "tensor_para_size"
value: {
string_value: "1"
}
}
## Modify model_checkpoint_path to the above converted path
parameters {
key: "model_checkpoint_path"
value: {
string_value: "/data/FasterTransformer/models/llama/1-gpu/"
}
}
## Modify FasterTransformer/examples/cpp/llama/llama_config.ini
model_name=llama_13b
model_dir=/data/FasterTransformer/models/llama/1-gpu/
# Modify these two files triton-model-store/llama/preprocess/1/model.py triton-model-store/llama/postprocess/1/model.py
# Check if this path corresponds to the tokenizer path
self.tokenizer = LlamaTokenizer.from_pretrained("/data/Llama2-Chinese-13b-Chat")
cd FasterTransformer
mkdir build && cd build
git submodule init && git submodule update
pip3 install fire jax jaxlib transformers
cmake -DSM=86 -DCMAKE_BUILD_TYPE=Release -DBUILD_PYT=ON -DBUILD_MULTI_GPU=ON -D PYTHON_PATH=/usr/bin/python3 ..
make -j12
make install
CUDA_VISIBLE_DEVICES=0 /opt/tritonserver/bin/tritonserver --model-repository=triton-model-store/llama/
I0730 13:59:40.521892 33116 grpc_server.cc:4589] Started GRPCInferenceService at 0.0.0.0:8001
I0730 13:59:40.523018 33116 http_server.cc:3303] Started HTTPService at 0.0.0.0:8000
I0730 13:59:40.564427 33116 http_server.cc:178] Started Metrics Service at 0.0.0.0:8002
python3 fastertransformer_backend/inference_example/llama/llama_grpc_stream_client.py
seq_len:148 token_text:<s><s><unk> : What does Beijing have?
</s><s>Assistant: Beijing is the capital of China and is a city with a long history and ancient civilization. It has a rich historical heritage and cultural treasures, including the Summer Palace, the Forbidden City, the Fragrant Hills, the Grand View Garden, and the Longshun Temple, etc. In addition, Beijing also has a rich variety of food, drinks, and sights.
Scan the QR code to add the assistant on WeChat