This is the 9th article by Teacher Wang Jue introducing AIGC. Please refer to the previous articles:
Although large models are amazing,
they can help us with general document work
But Teacher Wang Jue has always believed:
For professional research and education, large models are not suitable.
—— Because large models invariably produce “hallucinations”
That is, “serious nonsense”
See: 《There Are No Reliable Educational Applications for GPTs! — 5 Large Models Tested》
it is further processed based on “documents/literature” content
—— The technical term for this is RAG: Retrieval-Augmented Generation
No matter the final effect,
at least all content has sources and will not produce nonsense
We can only use it for teaching and research.
Of course, Kimi’s understanding of natural language instructions,
as well as the quality of the output results are indeed good,
this is why Teacher Wang Jue has always recommended Kimi.
To summarize: from the perspective of content sources
large language models are divided into two categories: pure LLM and RAG
LLM (Large Language Model) is a trained “silicon brain,”
it speaks entirely based on its training capabilities
RAG is based on the trained “silicon brain”
and processes specified document sources
before outputting to humans.
Besides Kimi, Teacher Wang Jue has discovered another RAG model
This RAG model can actually use “academic papers” as a source
which is perfect for academic research!
This RAG model is called “Mita”
Mita and Kimi are used in a similar way
Here we will only discuss their differences.
Focus on two key details in the above interface
which are also the areas where it surpasses Kimi.
Mita currently has two access methods:
Web Version and WeChat Mini Program.
(Mita’s website, just search for it…)
This article will take the web version as an example for introduction.
1. Specified Search Source
For academic research, specifying the “search source” as “academic” is clearly more appropriate:
If you choose “All Networks”, it is the same as Kimi,
searching documents on the internet, nothing new
However, when we switch to “academic,”
Mita will only search for documents in journal papers.
Next, Teacher Wang Jue will conduct an “academic search” on the topic of the impact of generative artificial intelligence on education
Please note: when we switch the search scope to “academic,”
we can further specify whether the journal database is Chinese or English:
The search results return as follows:
The above interface is divided into three parts
text, references, literature structure.
The text, nothing much to say, is similar to Kimi
You can view the details of the references in both the text and the appendix
Moreover, all content in the text is generated based on journal papers
(It is said that it only processes the “abstract” of the papers, not the content of the papers, because the abstracts are publicly available on the internet, but Mita probably cannot access the paper content)
—— It is equivalent to a review of journal papers (abstracts)
which is truly perfect for academic research!
By the way, if needed, you can also “follow up”
Of course, you can also ask follow-up questions
Content Structure Section
displays the outline and structure of the text
in the form of a mind map/text list
—— This is quite considerate, making it easy for us to grasp the content structure and context
The mind map can be zoomed, viewed in full screen
and can also be downloaded as an image to your computer
As for “generate presentation slides”
do not mistake it for PPT
It can be understood as just a way to display an outline.
2. Specify the “level of detail” for the search, as shown in the figure below:
Mita supports three levels of detail: Concise, In-Depth, Research
The returned content and the depth of analysis
each one deeper than the last.
The screenshots earlier in this article are in concise mode,
and you can see that the content is indeed quite brief, good for a general overview
If you want Mita to provide a more in-depth analysis,
you can either directly click “In-depth” or “Research” on the start screen
or click “More In-Depth” at the end of the text
to enter “In-Depth” mode:
In-Depth Mode is based on “Concise” mode,
in addition to the output text being more detailed and diverse
it also adds a list of related events, organizations, and individuals at the end of the text:
has the same structure as “In-Depth Mode”
besides the content being even richer and more diverse than In-Depth Mode
one of the main differences from In-Depth Mode
is the number of references
The number of references in Research Mode
is significantly higher than in Concise and In-Depth Modes!
of course, is better for academic research
Moreover, with Mita’s help,
literature reviews no longer require human brains
More is certainly better
Of course, whether in Kimi or Mita
there are many hidden features,
such as Kimi’s “Writing Cat”, which is activated at certain times
Kimi can directly draw mind maps, flowcharts, and create tables…
Everyone can just try it out while using.
Finally, let Teacher Wang Jue summarize the main similarities and differences between Kimi and Mita from the perspective of the “human brain”:
-
-
Both are LLM+RAG (Retrieval-Augmented Generation)
-
Kimi and Mita’s “All Networks” sources are basically the same
-
Both do not support multimodal (like images, music, video, etc.)
-
Both support multi-turn dialogue
-
Both can analyze article links, summarize, and extract outlines
-
-
Kimi can only search all network articles while Mita can specify “academic” search, winning
-
Kimi’s document source is generally 10-12 articles, while Mita’s concise and in-depth modes have about 30 articles, and research mode has nearly 100 articles, winning
-
Kimi supports uploading local documents, winning Mita only supports internet search
-
Kimi is a standard large language model with a high understanding of human instructions (prompts) and often executes well, winning Mita does not understand the “roles,” “skills,” etc. specified in the prompts, so it can be understood as a powerful literature review tool “searcher.”
—— This will be the focus of the next article, demonstrating the amazing understanding of prompts by large models and a possible use scenario of a large model in student learning.
Finally, let’s see how the two “silicon brains” view the “similarities and differences” issue!
In comparison, Kimi’s summary is much stronger,
not only is it more comprehensive and reliable,
but it also presents the results in a table format:
Additionally, both Kimi and Mita support “sharing conversations”, here is the answer link generated by Kimi (converted to a QR code), everyone can scan to view:
As for where to find the “share” conversation button, I believe it won’t be difficult for you!
To facilitate everyone’s discussion on the application of AI technology in education, Teacher Wang Jue has created a WeChat group, welcome to join:
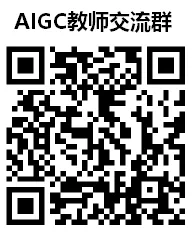
This article is part of Teacher Wang Jue’s training course “Essential Skills for Teachers in the AI Era”. To introduce the above training course to your unit, please enter “AI Training” at the beginning of this public account to learn about the contact information.
This public account provides comprehensive and in-depth articles on learning science research, micro-course and PPT research, as well as other practical technology research! Enter the code on the public account homepage to see more research:
-
wk: Micro-course Super Collection, covering design, production, and application
-
ppt: PPT Skills Collection
-
xxkx: Learning Science Research Articles