A public academic platform initiated by overseas scholars
Sharing information, integrating resources
Exchanging academics, occasionally discussing literature
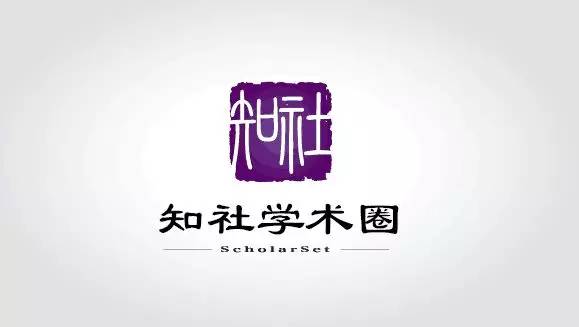
High-performance piezoelectric ceramics are a crucial type of material in modern electronic devices. In pursuit of sustainable development, traditional lead-based piezoelectric materials dominated by PbZr1−xTixO3 (d33 ≈ 200-1500 pC/N) are gradually transitioning to lead-free piezoelectric ceramics based on (K, Na)NbO3 (KNN). After decades of effort, the piezoelectric coefficient (d33) of KNN-based ceramics has continuously improved (d33 ≈ 100-650 pC/N), while a wealth of literature data awaits further exploration. However, the traditional empirical trial-and-error research paradigm is constrained by time-consuming and labor-intensive limitations. In recent years, with the development of artificial intelligence and materials informatics, machine learning has shown great potential in uncovering the structure/composition-performance relationship of materials. At the same time, machine learning methods that combine performance and interpretability, such as symbolic regression, sure independence screening, and sparsifying operator (SISSO), have emerged, providing researchers from non-computer science fields with a more intuitive and understandable new research approach.
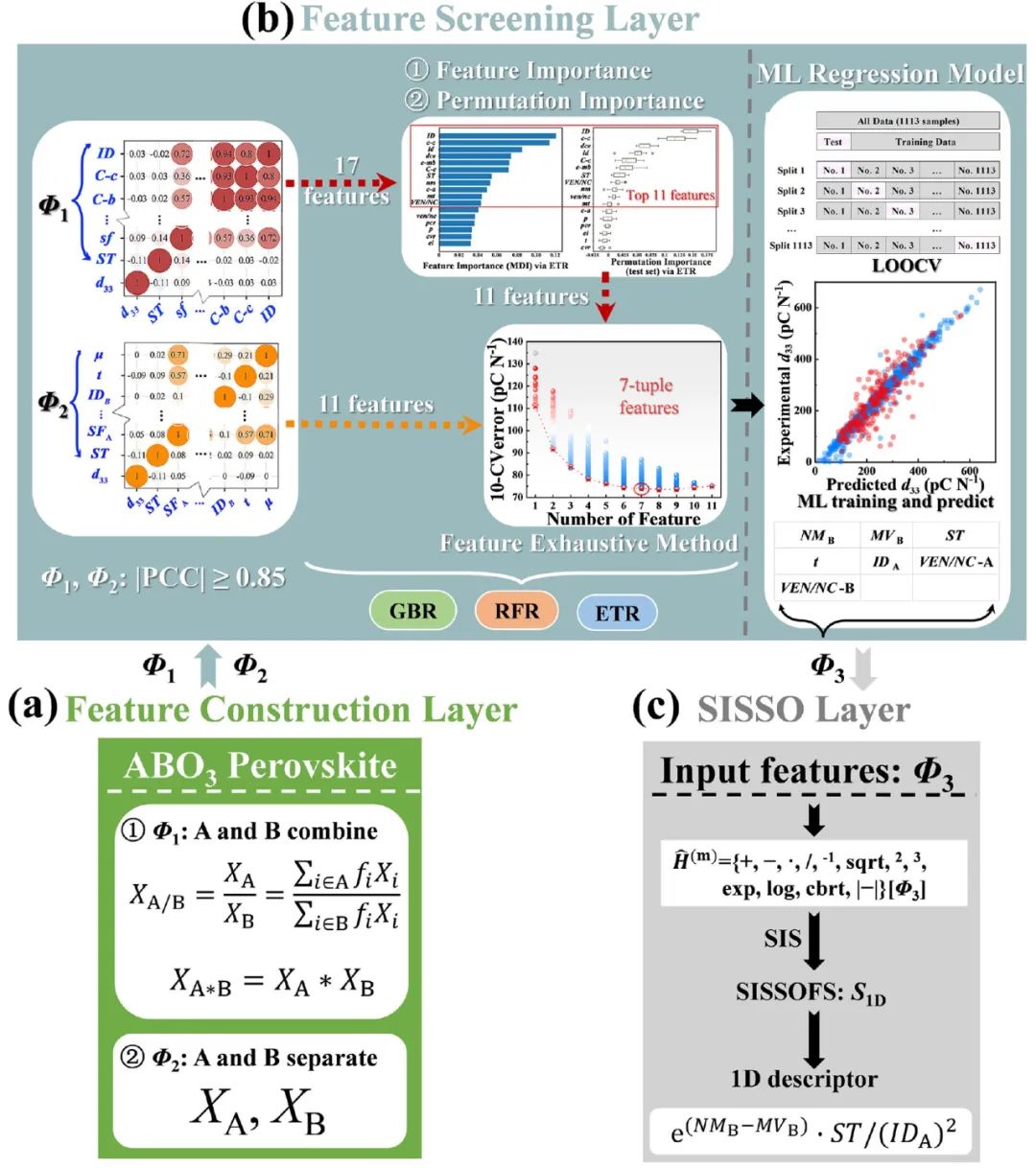
Fig. 1 Feature-assisted SISSO ML framework for d33 prediction and explicit descriptor mining
Associate Professor Wu Xiao from Fuzhou University and Professor Baisheng Sa, along with Professor Sun Zhimei’s team from Beihang University, constructed a d33 descriptor development framework by coupling feature engineering, machine learning regression, and the SISSO algorithm, proposing a descriptor that contains only four easily obtainable parameters:
for predictingKNN-based ceramics’ d33.
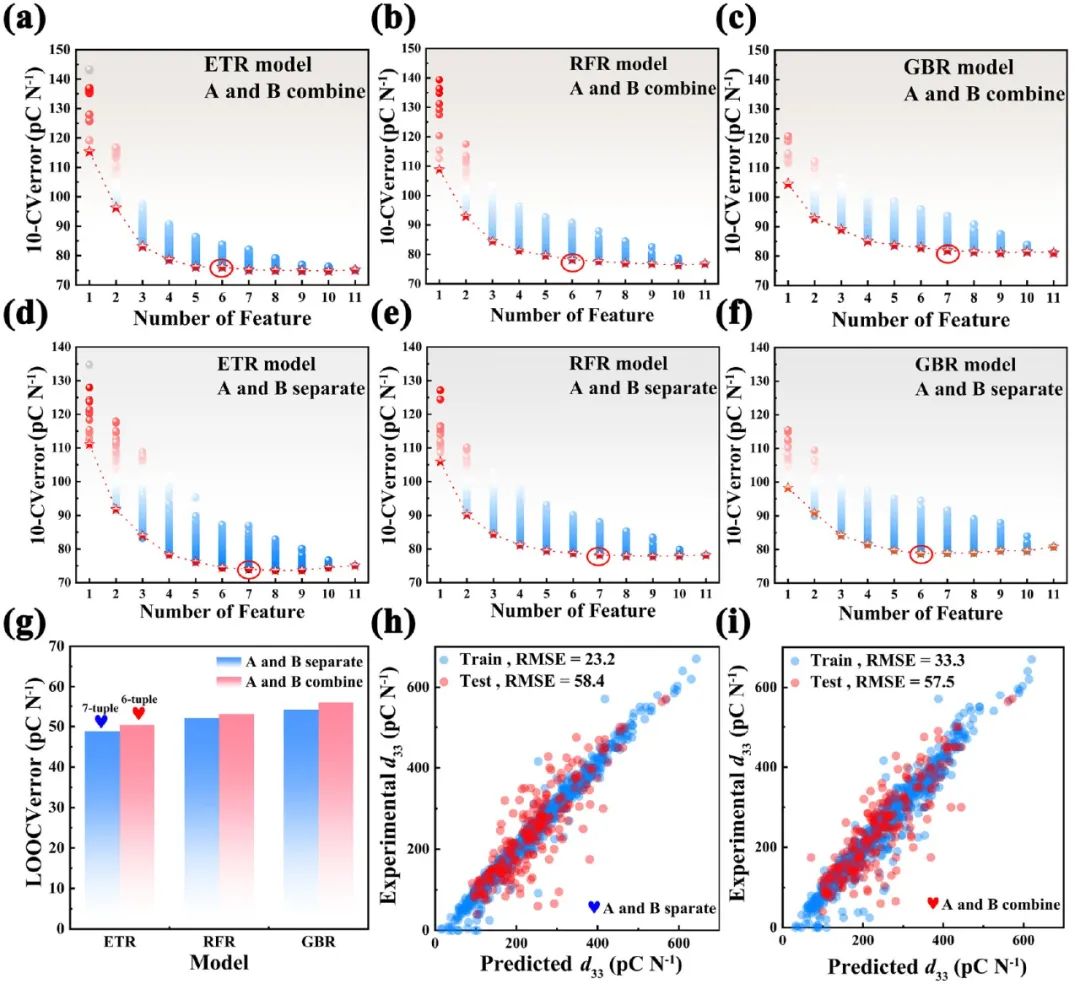
Fig. 2 ML model training.
The study established a regression mapping between the chemical composition of KNN-based ceramics and d33 from 244 published papers containing 1113 data points. The authors constructed features from both global and local perspectives based on the elemental positional configuration of ABO3-type perovskites, then employed Pearson correlation screening, feature importance, and feature exhaustion methods to select key features, achieving the lowest leave-one-out cross-validation error of ±49 pC/N with the optimal extreme random tree regression model. Finally, the optimal feature set was used for SISSO descriptor search, resulting in a descriptor that exhibits an intuitive trend of change with d33, and the descriptor value also has a mapping relationship with KNN-based ceramics, indicating that chemical compositions with descriptor values in a smaller range are more likely to achieve high piezoelectric coefficients. This method has been validated in the latest 63 KNN-based ceramic piezoelectric coefficient compositions published.
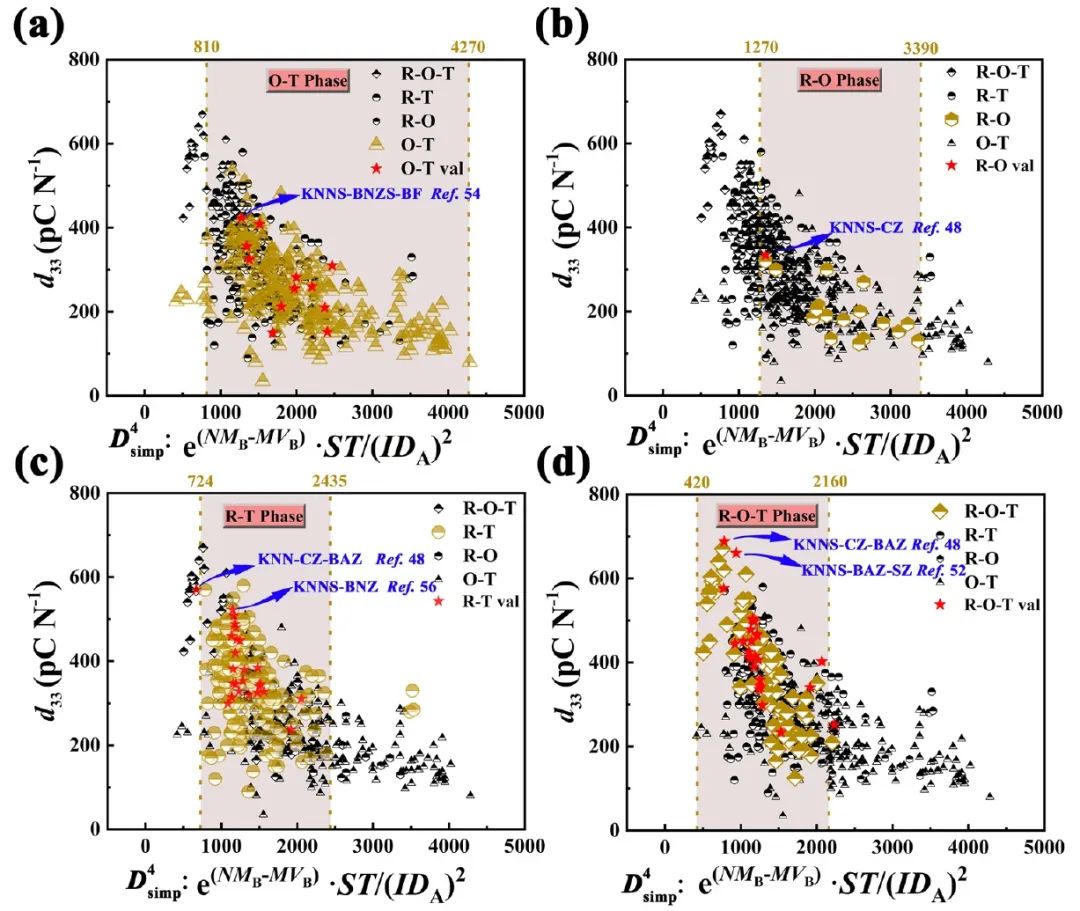
Fig. 3 Descriptor generation and performance.
The study established a mathematical mapping model among d33, composition, and phase boundaries in KNN-based ceramics, providing a highly intuitive and guiding approach to enhance perovskite performance, overcoming the low credibility and interpretability issues of traditional machine learning models.
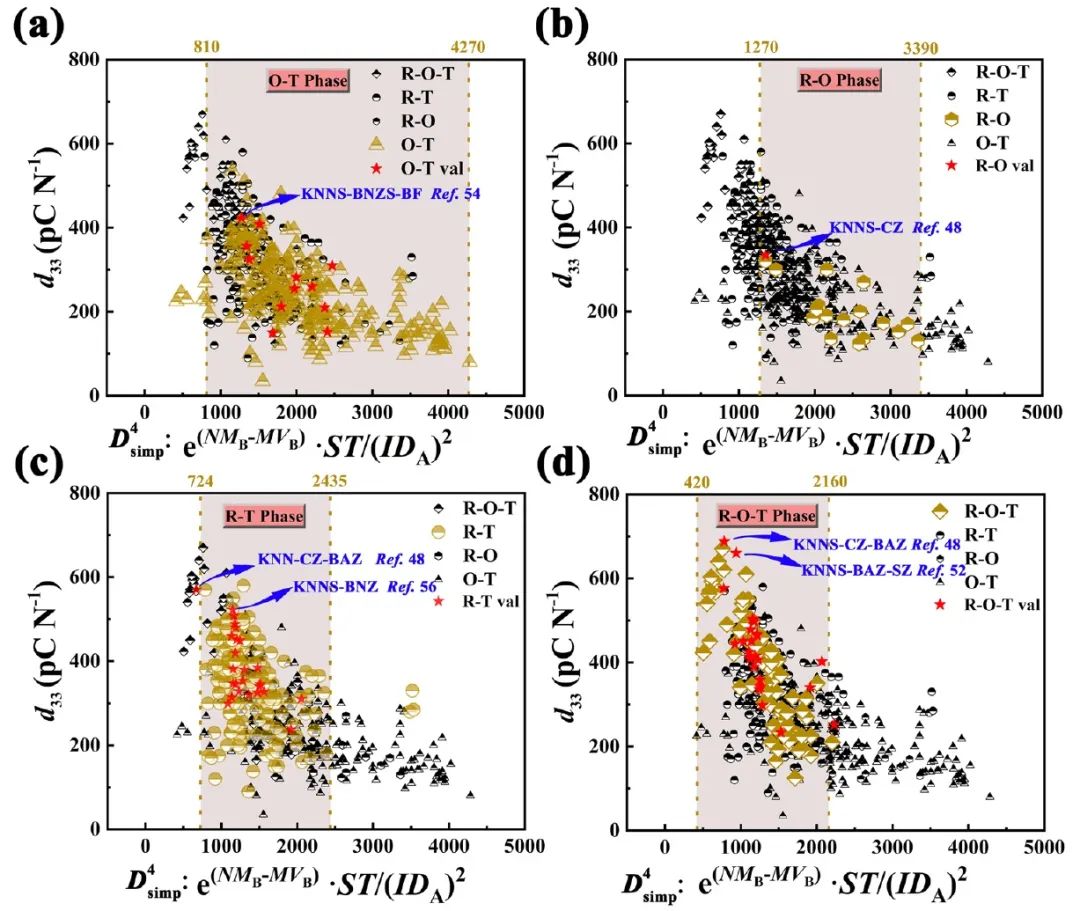
Fig. 4 Mapping analysis of D4simp and phase boundaries.
This article was recently published in npj Computational Materials 9:229(2023), the English title and abstract are as follows. Click the lower left corner “Read Original” to freely access the paper PDF.
An interpretable machine learning strategy for pursuing high piezoelectric coefficients in (K0.5Na0.5)NbO3-based ceramics
Bowen Ma, Xiao Wu, Chunlin Zhao, Cong Lin, Min Gao, Baisheng Sa & Zhimei Sun
Perovskite-type lead-free piezoelectric ceramics allow access to illustrious piezoelectric coefficients (d33) through intricate composition design and experimental modulation. Developing a swift and accurate technology for identifying (K, Na)NbO3 (KNN)-based ceramic compositions with high d33 in exceedingly large “compositional” space will establish an innovative research paradigm surpassing the traditional empirical trial-and-error method. Herein, we demonstrate an interpretable machine learning (ML) framework for quick evaluation of KNN-based ceramics with high d33 based on data from published literature. Specifically, a thorough feature construction was carried out from the global and local dimensions to establish tree regression models with d33 as the target property. Subsequently, the feature-property mapping rules of KNN-based piezoelectric ceramics are further optimized through feature screening. To intuitively understand the correlation mechanisms between ML regression targets and features, the sure independence screening and sparsifying operator (SISSO) method was employed to extract the essential descriptors to explain d33. A straightforward descriptor, “e” ^(“( NM_B – MV_B )” ) “⋅ST/(I” “D_A” )” ^”2″, consisting of only four easily accessible parameters, can accelerate the evaluation of a series of novel KNN-based ceramics with high d33 while exhibiting strong theoretical interpretability. This work not only provides a tool for the rapid discovery of high piezoelectric performance in KNN-based ceramics but also offers a data-driven route for the design of property descriptors in perovskites.
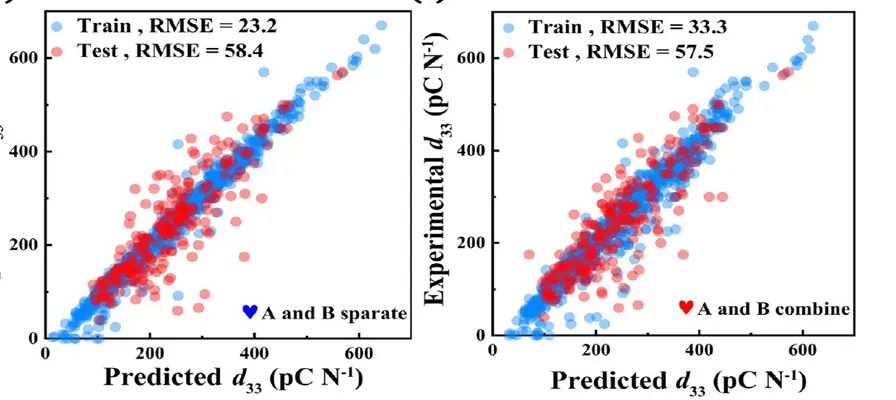
Npj Comput. Mater.: New Material Prediction—Van Hove Singularity Systems in Ternary Borides
Npj Comput. Mater.: Unleashing the Power of Neural Networks: Solving Phonon Transport Problems
Npj Comput. Mater.: Large-Scale, First-Principles Accurate Laser Processes
Npj Comput. Mater.: Coupled Control of Magnetic Anisotropy and Semimetallicity in Multiferroic Van der Waals Heterostructures
For media reprint authorization, please see below