Written by / Vice General Manager of the Information Technology Department, China Merchants Bank, Chen Xi
Information Technology Department, China Merchants Bank, Yang Mian, Ren Ke, Wang Jiu Hua
The rapid development of large models is gradually becoming a key driving force for innovation and transformation across various industries. China Merchants Bank has keenly observed this technological trend and has conducted in-depth exploration of the innovative integration of large models and low-code development. By leveraging the excellent characteristics exhibited by large models in semantic understanding, text generation, and knowledge retrieval, we further lowered the threshold for low-code development and enhanced the developer experience, effectively promoting the democratization of technology at China Merchants Bank.
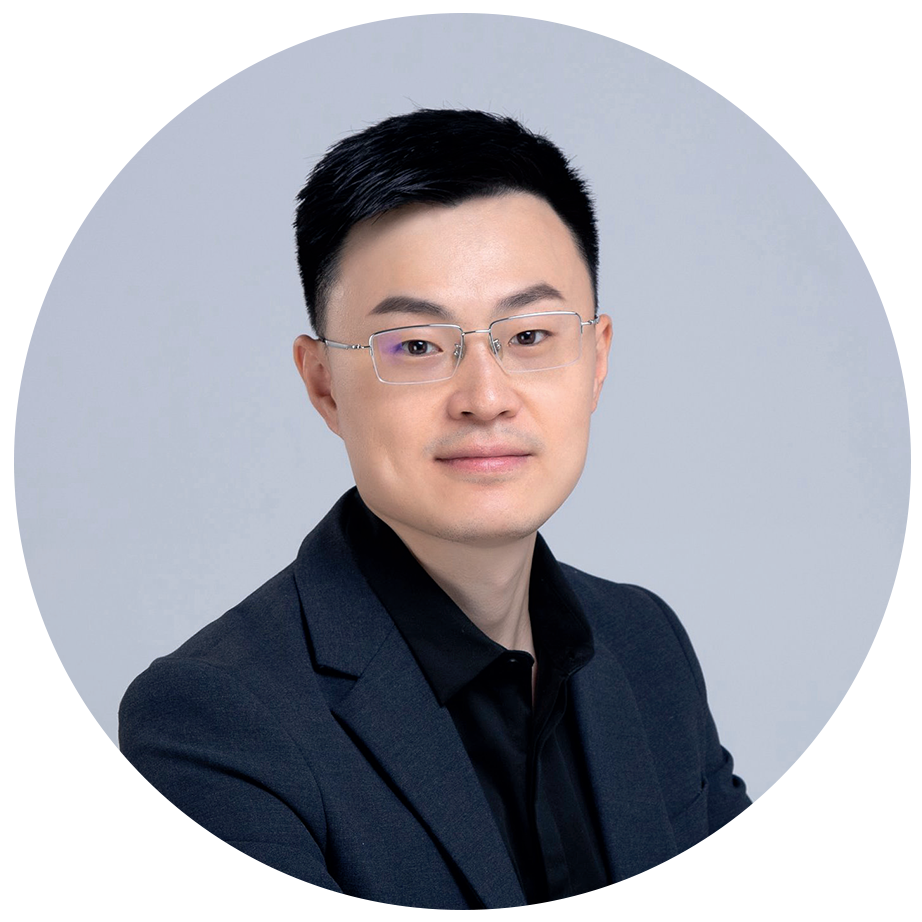
Information Technology Department, China Merchants Bank
Vice General Manager, Chen Xi
The practice and construction of low-code at China Merchants Bank has mainly gone through three stages.
1. Early Exploration
In the early construction of various business systems, China Merchants Bank already reflected design concepts such as “visualization” and “componentization”. Through the accumulation and reuse of components from various fields, we effectively improved the development efficiency of business systems. This practice can be seen as an early endeavor in the low-code field at China Merchants Bank.
2. Construction of an Enterprise-Level Low-Code Development Platform
Since 2019, China Merchants Bank has initiated the construction of an enterprise-level low-code development platform (hereinafter referred to as the low-code platform). After five years of development, the low-code platform has grown into one of the foundational development platforms at the organizational level, with over ten thousand applications launched. The low-code platform supports both zero-code and low-code development modes, meeting the needs of business and IT users at different levels. Currently, business users account for over 50%.
3. Integration of Low-Code and Large Models
In 2023, China Merchants Bank began exploring the integration of low-code and large models, utilizing the new characteristics of large models to significantly enhance the low-code platform in areas such as development assistance, application generation, operational Q&A, and interactive experience.
Thoughts on the Integration of Low-Code and Large Models
In searching for points of integration between low-code and large models, we approached from the following two directions.
Analyzing the entire journey of low-code development: In the phases of requirement analysis, data table design, interface design, process orchestration, and testing and deployment, there are numerous nodes where large models can help lower development thresholds and simplify processes.
Analyzing typical application patterns of large models: Intelligent Q&A, knowledge retrieval, text generation, and summarization are typical application patterns of large models that can enhance efficiency and quality in development.
By performing a matrix-style cross-analysis of these two paths, a series of exploratory scenarios emerged. By ranking the value and priority of these scenarios, we identified the following key scenarios for practical application.
Practical Scenarios of Large Models under Low-Code
Based on the above thoughts, we focused on three major scenarios: “Low-Code Copilot”, “Low-Code Application Generation”, and “Low-Code Operations” (as shown in Figure 1).
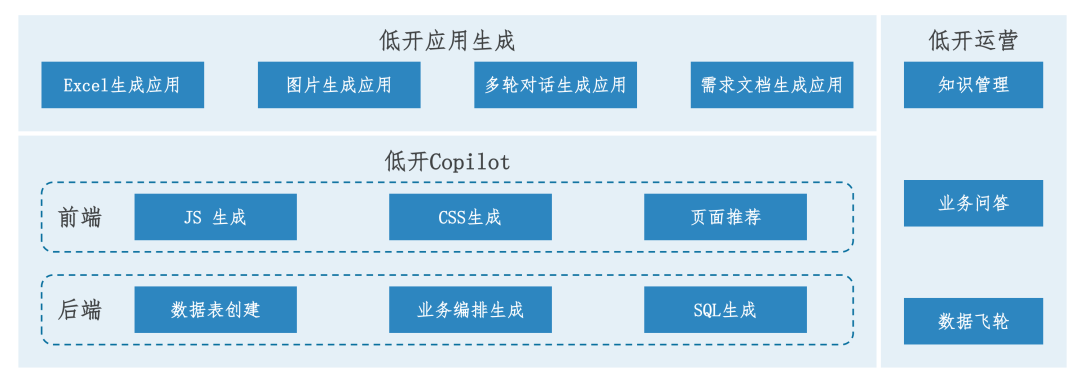
Figure 1: Three Major Scenario Practices
1. Low-Code Copilot
(1) Frontend Development Copilot: JS and CSS Generation. Low-code platforms typically provide default operations and styles; if changes are needed, developers must have a certain level of JS and CSS skills.
In practice, we found that a typical user of the low-code platform is often a backend developer who may not be familiar with JS syntax and may not know how to use CSS.
Therefore, utilizing prompts to generate JS and CSS code in frontend page development can greatly simplify the customization process. This feature quickly rose to become one of the top three in usage within a week of its launch, effectively improving application development efficiency.
(2) Backend Development Copilot: Data Table Creation and Business Orchestration Generation. The core of generating low-code applications on the low-code platform is DSL. DSL is a domain-specific language that abstractly describes business functionality logic and interaction rules. Both data tables and business orchestration are described using DSL.
With the introduction of large models, the process of creating backend data tables has shifted from “interface interaction to generate DSL” to “generate DSL through natural language description”: by describing the fields included in natural language, the large model extracts these fields to generate the DSL for table creation. Business orchestration follows a similar pattern, where the process is described in natural language, and the large model extracts various nodes of the process to generate the business orchestration DSL.
The key to this work lies in generating effective DSL. Since DSL is private domain knowledge of low-code, it is not within the knowledge scope of the foundational model. The user manual accompanying the low-code platform can only describe its rules but cannot exhaustively cover its combinations. Therefore, having the large model generate DSL that varies with the scenario presents certain challenges.
To tackle this difficulty, we incorporated highly relevant knowledge samples recalled from the private domain knowledge base into the prompts, employing a few-shot learning approach to enable the large model to generate content based on the provided knowledge samples. This can generate standard-compliant DSL in certain scenarios.
This approach also has certain limitations, such as the need to include context each time, token length limitations, and the inability to retain memory. In the next stage, we plan to address these issues through fine-tuning to further enhance accuracy in DSL generation.
2. Low-Code Application Generation
Once we established intelligent capabilities at the aforementioned “points”, we then integrated workflows to link these individual points, thereby forming an “end-to-end solution”.
A significant demand for low-code application development arises from the “online and systematic transformation of manual ledger work”. In such scenarios, a substantial amount of manually organized data exists in the form of “Excel sheets” or “paper charts”. To improve development efficiency for such needs, we launched end-to-end application generation, including “Excel Generation Application” and “Image Generation Application” (as shown in Figure 2).
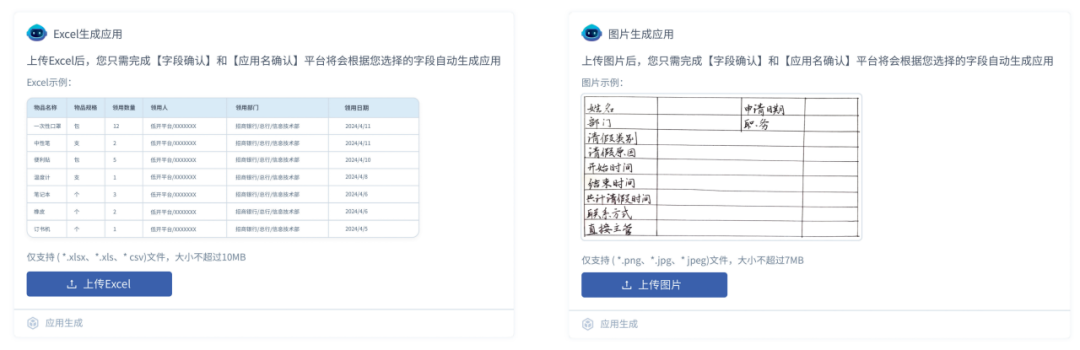
Figure 2: Intelligent Application Generation
In the “Excel Generation Application”, users only need to upload a local Excel data table to construct an application with a complete set of CRUD functionalities, generating both form and table interaction views. The “Image Generation Application” takes it a step further by first recognizing images on paper, extracting key table elements, and subsequently generating applications.
End-to-end application generation must address two challenges: the first challenge is effectively recognizing entities in Excel or images and determining the corresponding field types. If only the large model is used, the accuracy does not meet engineering requirements; in practice, we combined the large model with traditional engineering data dictionaries, raising the accuracy to over 95%; the second challenge is achieving the correct binding of entities to data tables, page components, and methods. To enhance binding accuracy, we established a series of application templates and utilized a template engine to select appropriate templates for application generation. By solidifying resource binding through templates, accurate rendering of applications is ultimately achieved.
Once the functionality for constructing end-to-end applications went live, the time required to develop a complete application was compressed from hours to minutes. We will continue to roll out solutions such as “Multi-Turn Dialogue Application Creation” and “Requirement Document Creation Application” as end-to-end solutions.
3. Low-Code Operations
The primary knowledge base of the low-code platform mainly consists of product documentation and help documents. As operations progressed, a wealth of Q&A, solutions, and reporting materials have been accumulated. However, operational personnel often encounter knowledge blind spots, and the quality of solutions provided can sometimes be inadequate when addressing more complex issues.
The large model’s RAG technology can effectively address the above issues. For example, when a user consults an operational colleague about how to resolve SQL exceptions in the application, the operational colleague, not being an SQL expert, may find it challenging to quickly and accurately identify the cause of the SQL exception. After deploying the RAG-based low-code operational assistant, it can not only point out issues in the SQL but also directly provide optimized SQL for users to copy and use.
Currently, the response accuracy of operations utilizing the large model has reached approximately 75%.
Technical Highlights of the Integration of Low-Code and Large Models
1. Data Flywheel
After several years of development, the low-code platform at China Merchants Bank currently has over ten thousand applications online, with more than a million DSL fragments accumulated, creating a substantial amount of low-code assets. The project team continuously fine-tunes the large model using this vast amount of private domain data to enhance its performance.
To improve the efficiency and quality of private domain data cleaning and annotation, we adopted a solution of “AI automatic annotation + manual re-inspection”. Using a rule engine tool, the low-code platform built an AI automatic annotation bot based on the large model, which annotates the corpus through scheduled tasks and batch runs. These annotated corpuses are then issued to data annotators in task form, who correct the automatically annotated corpus through a “work-as-annotation” approach. The corrected data is used as corpus for fine-tuning the large model to further enhance the model’s performance, achieving an automated data closed loop.
2. Constraining the Hallucinations of Large Models
During the application of large models, the generated DSL fragments can range from a few hundred to tens of thousands of characters, making it easy to produce “serious nonsense”. Ensuring the stability and quality of the output content from the large model and constraining its hallucinations is a technical challenge faced by the low-code platform.
After analyzing the data of DSL fragments generated under similar scenarios, the project team found that the main content of DSL generated in similar scenarios varies little. For example, 80% of the DSL fragments corresponding to the most common “query forms” and “tables with CRUD” remain unchanged. Based on this, we categorized the varying parts of the DSL into scenarios and constructed a scenario-based generation logic to limit the range of content variations produced by the large model. Additionally, we employed reflection and chain-of-thought patterns during generation to improve accuracy. Through these technical modifications, the probability of hallucinations generated by the large model has been significantly reduced, allowing the generated DSL fragments to be utilized by the platform.
Summary and Outlook
Currently, China Merchants Bank has achieved initial results in the practice of low-code and large models. How to continuously upgrade the low-code platform based on AI capabilities and assist in the construction of the digital China Merchants Bank still remains a challenging task. In the future, we will further expand the boundaries of large model applications in the low-code field, constructing more precise DSL through continuous fine-tuning of the model, and uncovering more end-to-end scenarios. Simultaneously, we will explore in-depth in the multimodal aspect, leveraging various interaction methods such as text, images, and voice to create a superior user experience.
(This article was published in the September 2024 issue of “Financial Electronics”)