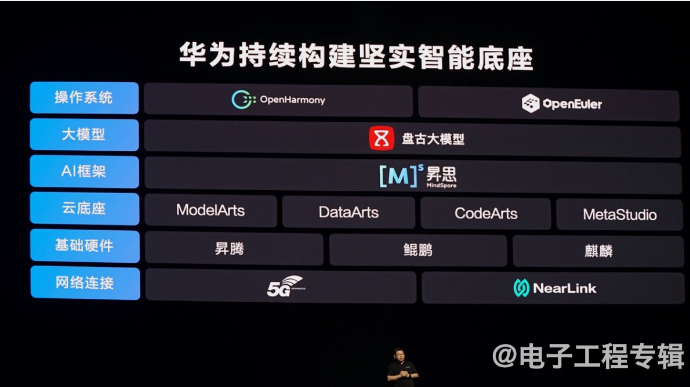
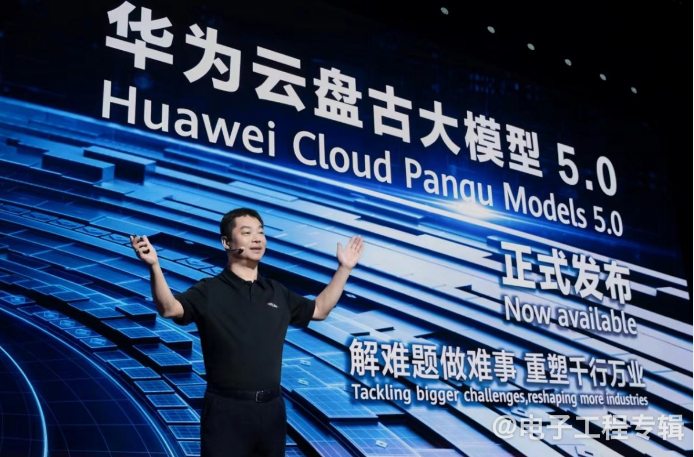
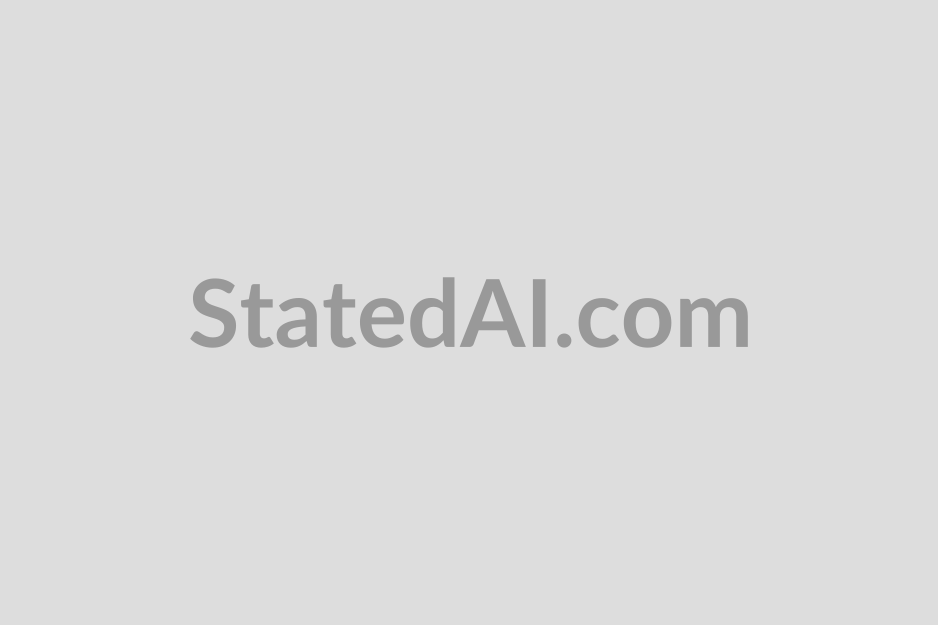
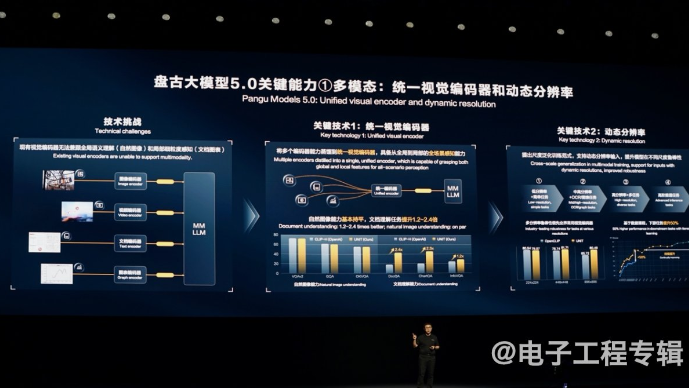
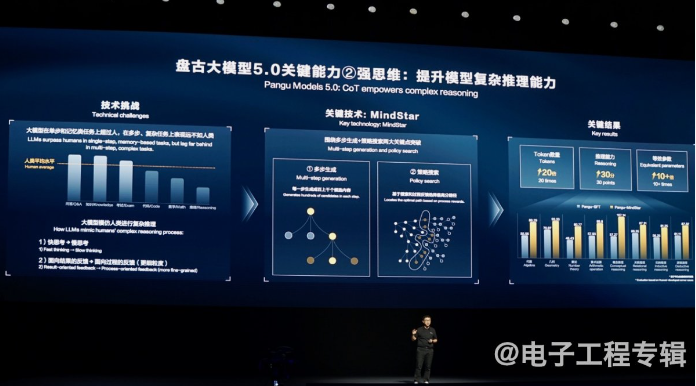
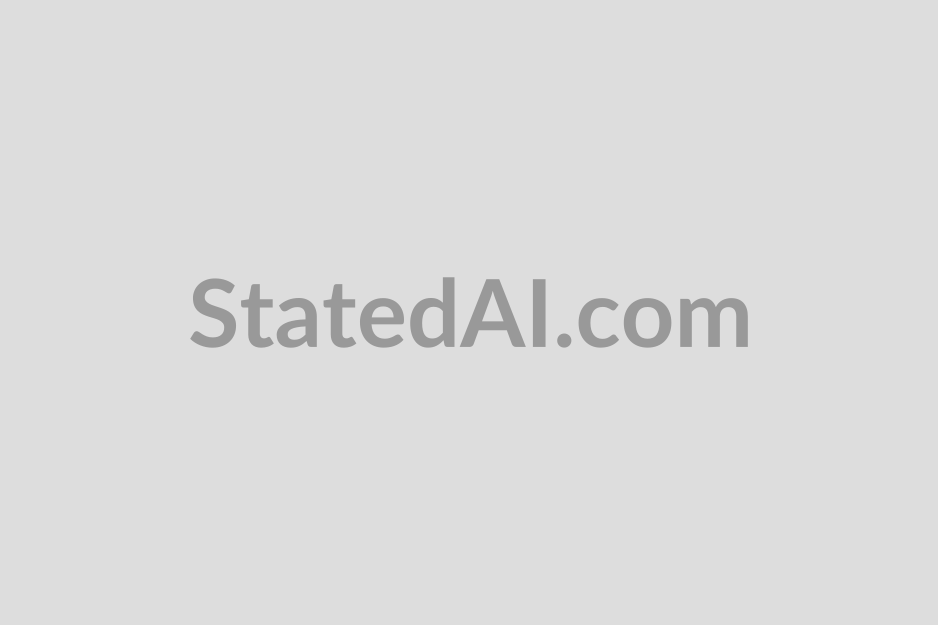
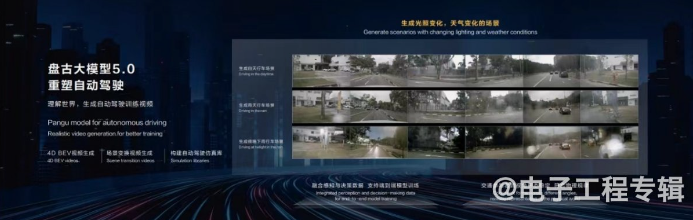
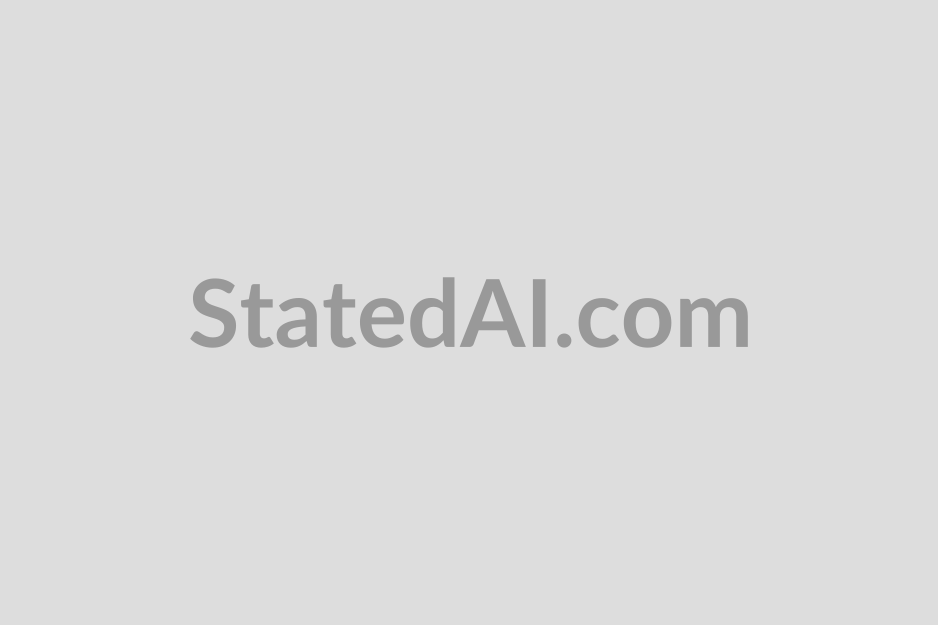
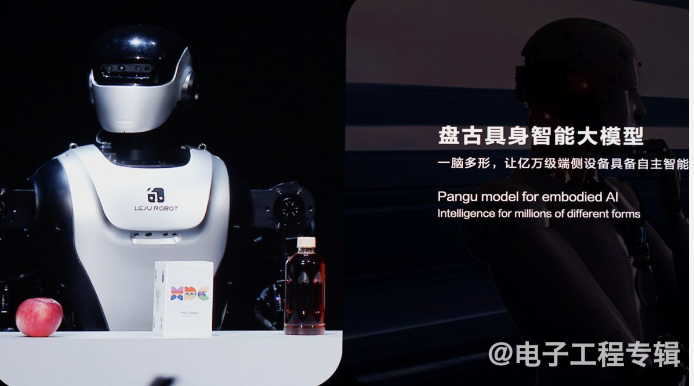
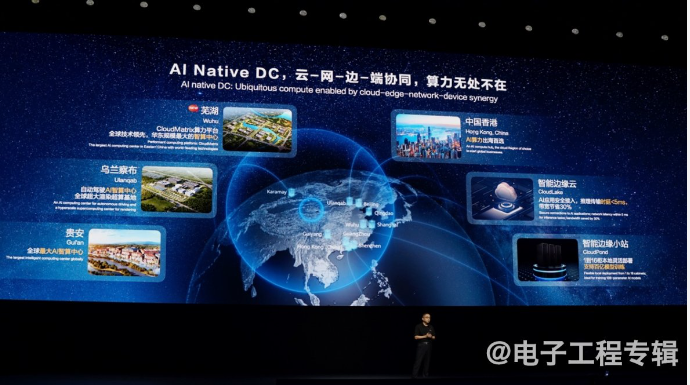
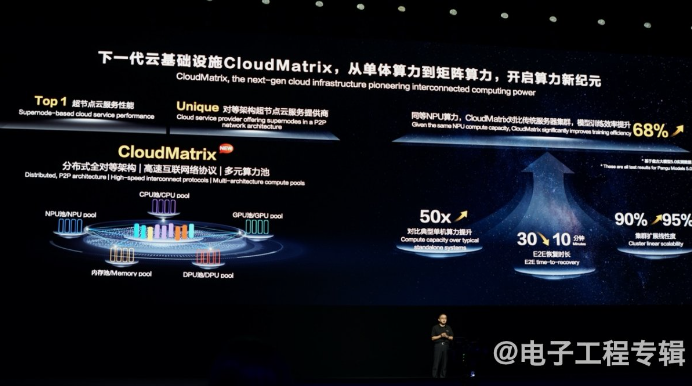
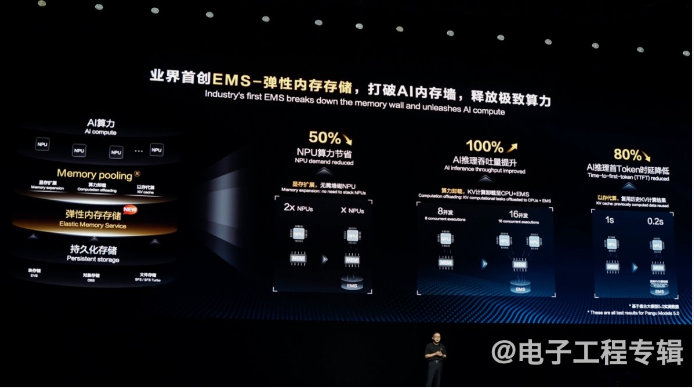
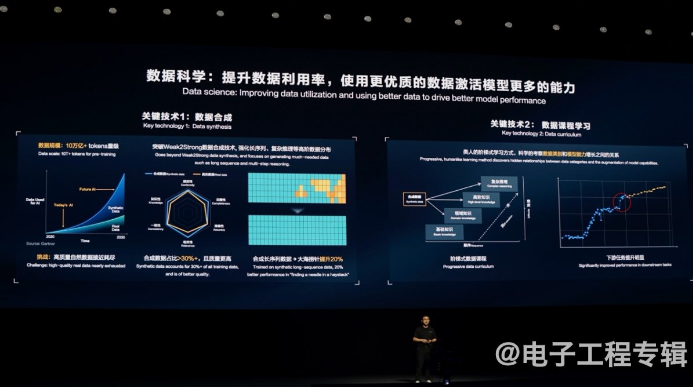
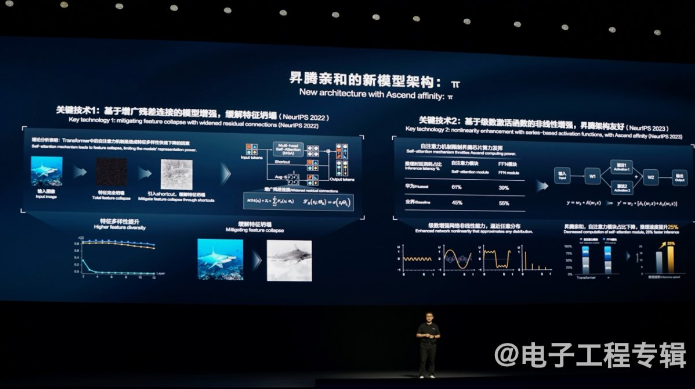
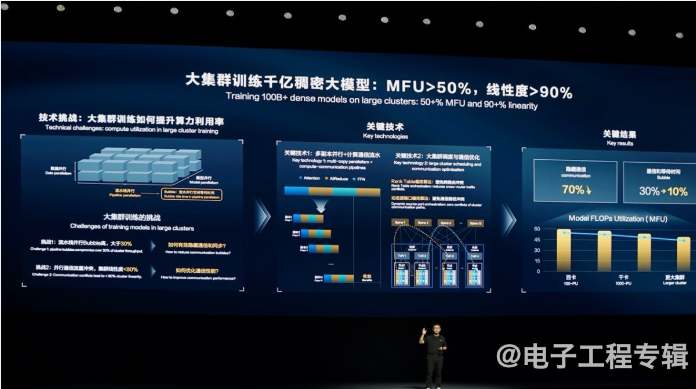
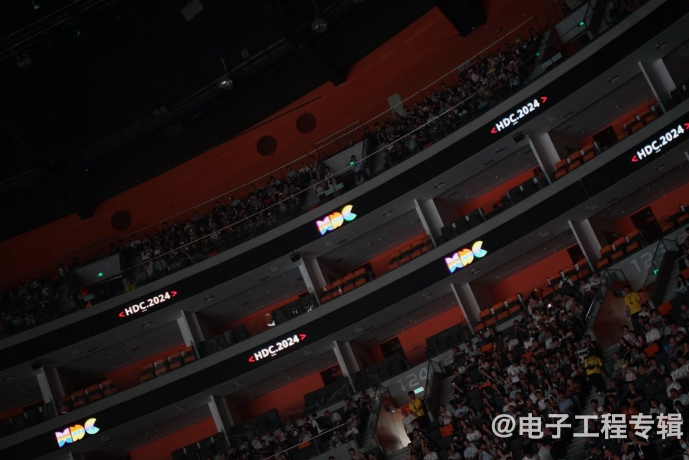
-
Dissecting the Pinduoduo WiFi Signal Booster, Seeing the Board Made Me Cry…
-
Overview of LED Chip Principles
-
The 2024 Silicon 100 List is Out!
-
Next-Generation Power Switch Technology: B-TRAN
-
F15 Fighter Jet and Weapons Open Source!! A Total of 250G…
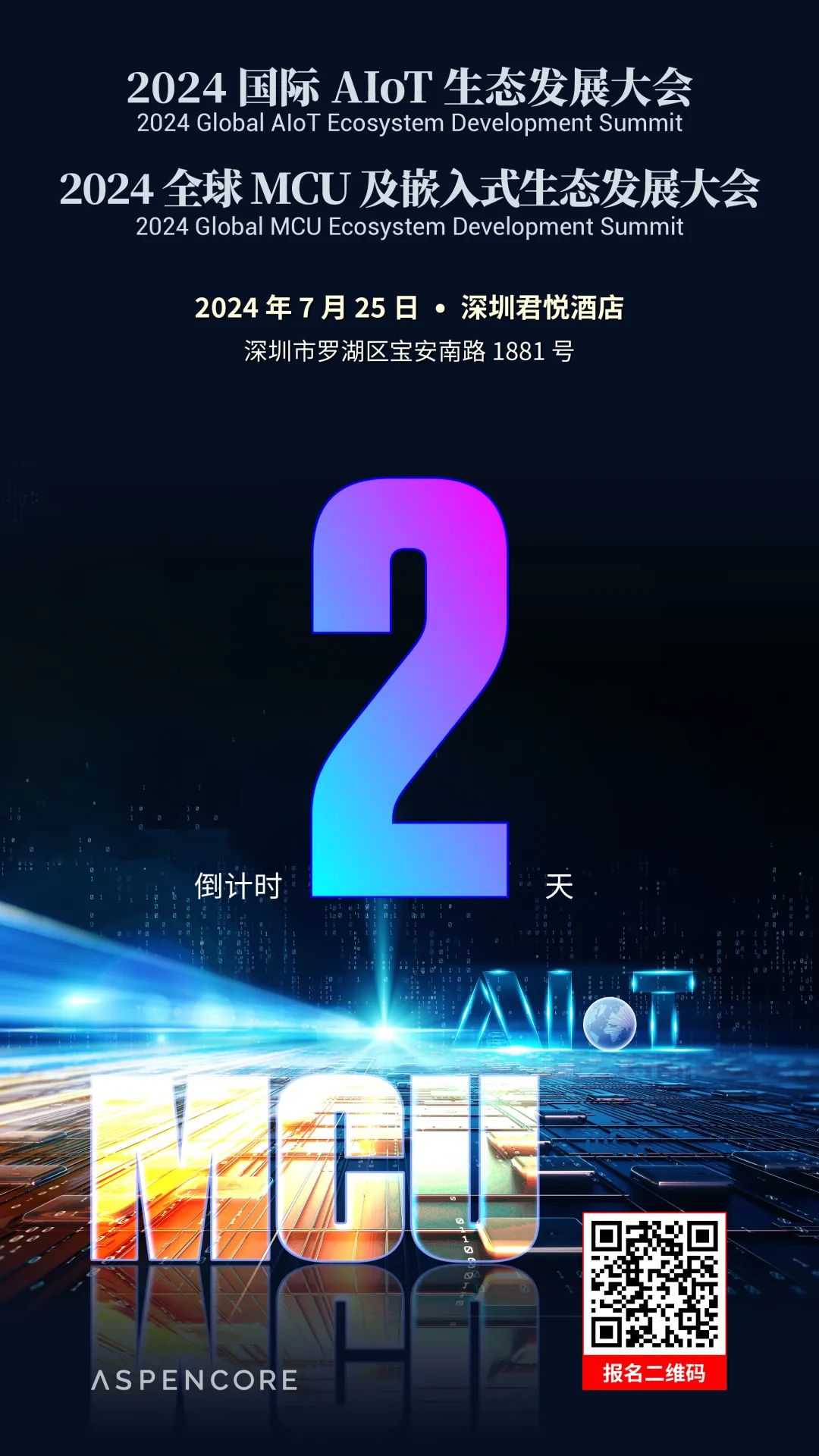