Click on the above“Beginner Learning Vision” to add aStar or “Top”
Important content delivered at the first time
With the rapid development of image processing technology, it has driven the emergence and development of image recognition technology, which has gradually become an important component of the field of artificial intelligence, and is widely used in fields such as facial recognition, fingerprint recognition, and medical diagnosis, playing an important role.
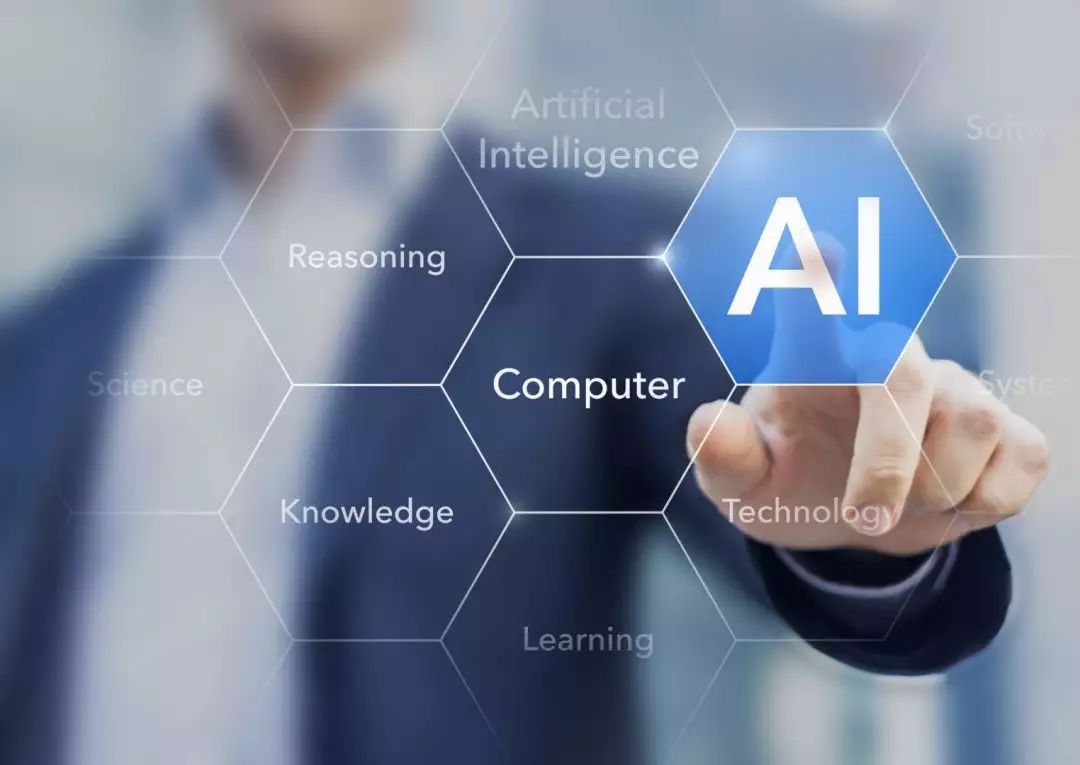
This also gives students more space to think about topics. Today, the editor will briefly discuss the popular topic direction of image recognition technology, hoping to inspire students more!
Overview of Image Recognition Technology
Meaning of Image Recognition Technology
Image recognition is an important field of artificial intelligence, referring to the technology that uses computers to process, analyze, and understand images to identify various different patterns and targets. In general industrial use, industrial cameras are used to capture images, and then software is used to perform further recognition processing based on the grayscale differences in the images.
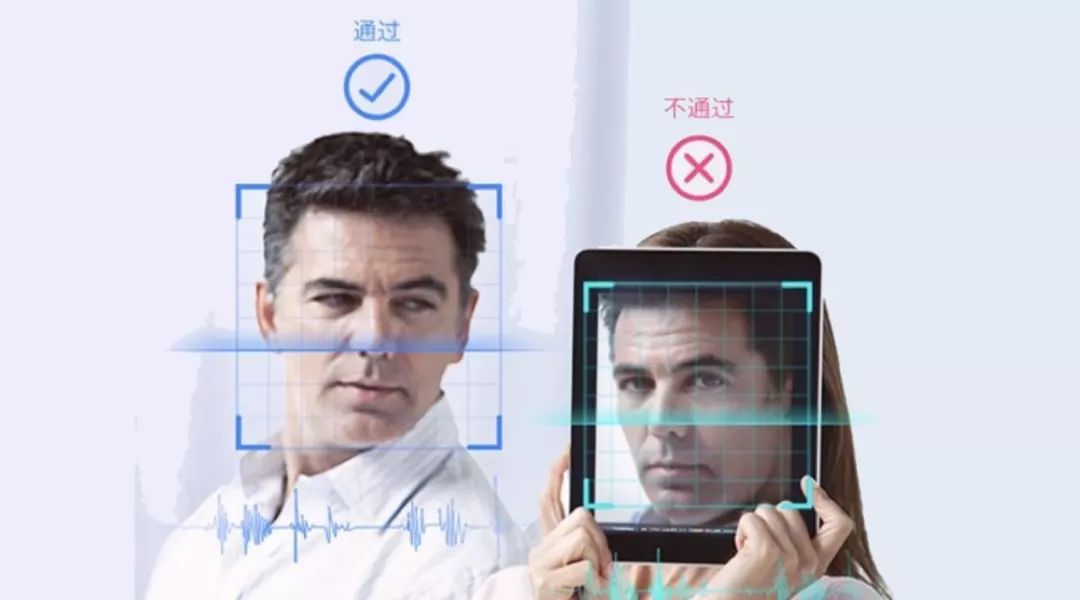
In practical applications, especially for recognition, it is necessary to clarify what kind of object is being recognized, as well as its location and posture. Currently, image recognition has been widely applied in various fields, such as license plate recognition and traffic sign recognition in the transportation field, flying object recognition in the military field, terrain surveying, fingerprint recognition, and facial recognition in the security field.
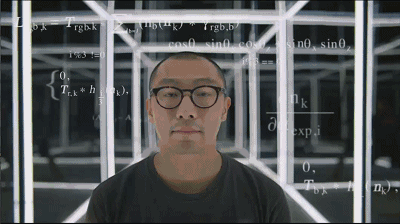
Principle of Image Recognition Technology
The principle of image recognition mainly involves processing information with a certain level of complexity. The processing technology does not randomly appear in computers but is mainly based on the practices of some medical researchers, combined with computer programs to simulate and realize relevant content. The computer implementation of this technology is fundamentally similar to the basic principles of human image recognition, with the only difference being that computers are not influenced by any external factors. Humans do not just recognize based on the image memories stored in their minds but use image features to classify them, and then recognize images based on the features of each category. Computers also adopt the same image recognition principles, classifying and extracting important features of images while effectively eliminating unnecessary excess features, thus enabling image recognition. Sometimes the extraction of the aforementioned features by computers is quite obvious, and sometimes it is rather ordinary, which can significantly affect the efficiency of computer image recognition.
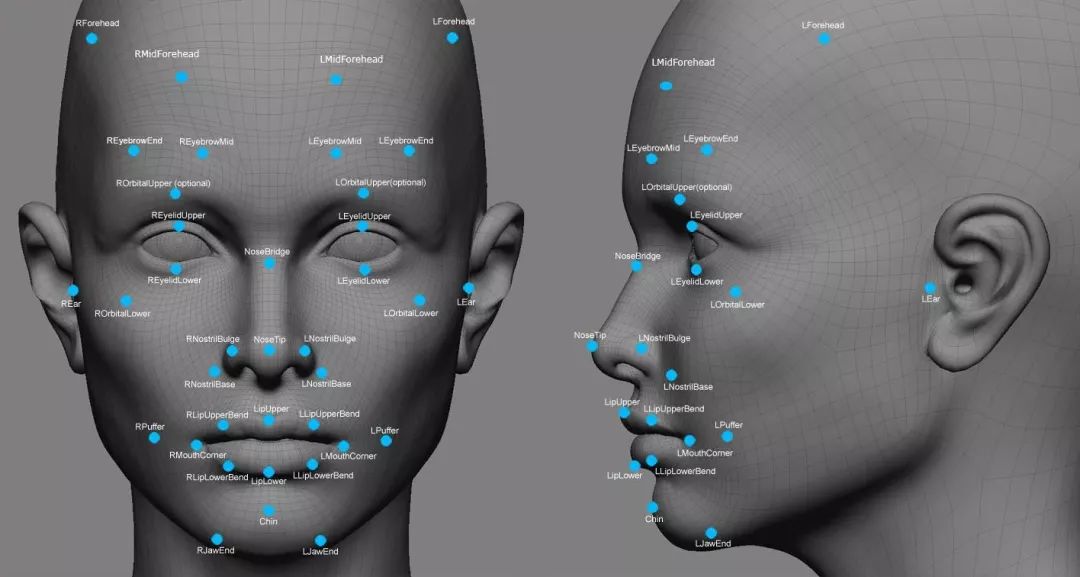
Process of Image Recognition Technology
Since the emergence of image recognition technology is based on artificial intelligence, the process of computer image recognition is generally consistent with the process of human brain image recognition. In summary, this process mainly includes four steps:
1. Information Acquisition, which mainly refers to converting sound and light information into electrical signals through sensors, that is, acquiring basic information about the recognition object and converting it into information that can be recognized by computers;
2. Information Preprocessing, which mainly refers to using operations such as noise reduction, transformation, and smoothing to process images, thereby enhancing the important features of the images;
3. Feature Extraction and Selection, which mainly refers to extracting and selecting image features in pattern recognition. In summary, it involves recognizing the diverse characteristics of images, such as separating features in a certain way, which is known as feature extraction;
4. Designing Classifiers and Classification Decisions, where designing classifiers involves establishing recognition rules based on training, enabling these recognition rules to derive the main types of features, thereby continuously improving the recognition rate of image recognition. After that, by recognizing special features, the final evaluation and confirmation of the image is achieved.
Common Forms of Image Recognition Technology
Firstly, the development of image recognition has gone through three stages: character recognition, digital image processing and recognition, and object recognition.
The research on character recognition started in 1950, generally recognizing letters, numbers, and symbols, with applications ranging from printed text recognition to handwritten text recognition.
The research on digital image processing and recognition began in 1965. Compared to analog images, digital images have significant advantages such as storage convenience, compressibility, and less distortion during transmission, which provide strong momentum for the development of image recognition technology.
Object recognition mainly refers to the perception and understanding of objects and environments in the three-dimensional world, belonging to the category of advanced computer vision. It is a research direction that combines digital image processing and recognition with artificial intelligence and systems science, and its research results are widely applied in various industrial and detection robots.
With the rapid development of computers and information technology, the application of image recognition technology has gradually expanded to many fields, especially playing an increasingly important role in facial and fingerprint recognition, satellite cloud image recognition, and clinical medical diagnosis. Generally, image recognition technology mainly refers to using computers to process captured images according to established targets. In daily life, the application of image recognition technology is also very common, such as license plate capture, product barcode recognition, and handwriting recognition. With the gradual development and continuous improvement of this technology, it will have a broader application field in the future.
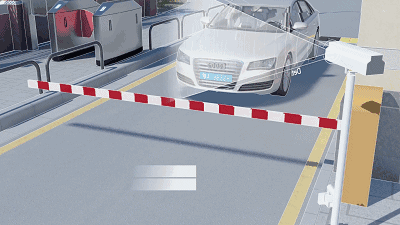
Image Recognition Technology Based on Neural Networks
Currently, image recognition based on neural networks is a relatively new technology, effectively integrating neural network algorithms based on traditional image recognition methods. Here, the neural network mainly refers to artificial neural networks; in other words, the neural networks discussed in this article are not the neural networks of animal bodies but refer to a type of neural network that simulates animal neural networks artificially. Regarding image recognition technology based on neural networks, the genetic algorithm effectively combined with the BP neural network is the most classic model, which can be applied in many fields. For example, in intelligent vehicle monitoring, photo recognition technology is used. When a vehicle passes a certain location, the detection device will generate a corresponding response, activating the image capture device to obtain feature images of the vehicle from both the front and back, and during the process of recognizing the license plate characters, two algorithms based on neural networks and fuzzy matching are used.
Image Recognition Technology Based on Nonlinear Dimensionality Reduction
Using computers to recognize images is a recognition technology based on high-dimensional forms. Regardless of the resolution of the original image, the data generated by that image usually has multi-dimensional characteristics, which increases the difficulty of computer recognition to some extent. To make the performance of computer image recognition more efficient, employing methods for dimensionality reduction is one of the most direct and effective methods. Generally, dimensionality reduction can be divided into nonlinear dimensionality reduction and linear dimensionality reduction. For example, the most common linear dimensionality reduction methods include principal component analysis and linear singular analysis, which are characterized by simplicity and ease of understanding. The projection images obtained by applying linear dimensionality reduction to the data set yield the low-dimensional optimal representation of that data set.
As a newly emerging image recognition technology in information technology, it has been widely applied in many application fields. With the rapid development of information technology, image recognition technology has also developed very rapidly. In many social fields, effectively applying image recognition technology will fully realize the value of social and economic benefits.
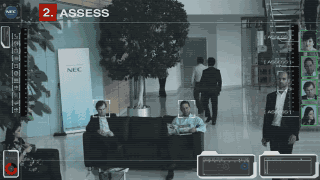
The editor believes that through this popular science article, many students have gained a deeper understanding of image recognition, hoping to broaden students’ thinking and use artificial intelligence image recognition technology to solve more problems and benefit society and the world!
Download 1: Chinese Version Tutorial for OpenCV-Contrib Extension Modules
Reply "Chinese Tutorial for Extension Modules" in the backend of the "Beginner Learning Vision" public account to download the first Chinese version of the OpenCV extension module tutorial on the internet, covering over twenty chapters on extension module installation, SFM algorithm, stereo vision, target tracking, biological vision, super-resolution processing, etc.
Download 2: 52 Lectures on Python Vision Practical Projects
Reply "Python Vision Practical Projects" in the backend of the "Beginner Learning Vision" public account to download 31 visual practical projects including image segmentation, mask detection, lane line detection, vehicle counting, eyeliner addition, license plate recognition, character recognition, emotion detection, text content extraction, facial recognition, etc., to help quickly learn computer vision.
Download 3: 20 Lectures on OpenCV Practical Projects
Reply "20 Lectures on OpenCV Practical Projects" in the backend of the "Beginner Learning Vision" public account to download twenty practical projects based on OpenCV for advanced learning of OpenCV.
Group Chat
Welcome to join the public account reader group to communicate with peers. Currently, there are WeChat groups for SLAM, 3D vision, sensors, autonomous driving, computational photography, detection, segmentation, recognition, medical imaging, GAN, algorithm competitions, etc. (which will gradually be subdivided). Please scan the WeChat ID below to join the group, and note: "Nickname + School/Company + Research Direction", for example: "Zhang San + Shanghai Jiao Tong University + Visual SLAM". Please follow the format, otherwise, you will not be approved. After successful addition, you will be invited to join related WeChat groups based on your research direction. Please do not send advertisements in the group, or you will be removed. Thank you for your understanding~