Follow our public account to discover the beauty of CV technology
This article is adapted from Machine Heart, edited by Dan Jiang.
Recently, diffusion models have become a research hotspot in the AI field. Researchers from Google Research and UT-Austin have fully considered the ‘corruption’ process in their latest study, proposing a design framework for diffusion models suitable for more general corruption processes.
We know that score-based models and Denoising Diffusion Probabilistic Models (DDPM) are two powerful generative models that produce samples by reversing the diffusion process. These two types of models have been unified under a single framework in the paper “Score-based generative modeling through stochastic differential equations” by Yang Song et al., and are widely referred to as diffusion models.
Currently, diffusion models have achieved significant success in a range of applications, including image, audio, and video generation, as well as solving inverse problems. In the paper “Elucidating the design space of diffusion-based generative models” by Tero Karras et al., the design space of diffusion models is analyzed, identifying three stages: i) scheduling the noise levels, ii) choosing network parameterization (each parameterization generates a different loss function), iii) designing the sampling algorithm.
Recently, in a collaborative arXiv paper titled “Soft Diffusion: Score Matching for General Corruptions” by researchers from Google Research and UT-Austin, it was noted that there is still an important step in diffusion models: corruption. Generally, corruption is a process of adding noise of varying amplitudes, which also requires rescaling for DDPM. While some attempts have been made to use different distributions for diffusion, a universal framework is still lacking. Therefore, the researchers proposed a diffusion model design framework suitable for more general corruption processes.
Specifically, they introduced a new training objective called Soft Score Matching and a novel sampling method called Momentum Sampler. The theoretical results indicate that for corruption processes that meet regularity conditions, Soft Score Matching can learn their scores (i.e., likelihood gradients), and diffusion must convert any image into any image with non-zero likelihood.
In the experimental section, the researchers trained models on CelebA and CIFAR-10, where the model trained on CelebA achieved a state-of-the-art FID score of 1.85 for linear diffusion models. Moreover, compared to models trained using the original Gaussian denoising diffusion, the models trained by the researchers were significantly faster.
Paper link: https://arxiv.org/pdf/2209.05442.pdf
Overview of the Method
Generally, diffusion models generate images by reversing the corruption process that gradually increases noise. The researchers demonstrated how to learn the reversal of diffusion involving linear deterministic degradation and stochastic additive noise.
Specifically, the researchers demonstrated a framework for training diffusion models using a more general corruption model, which includes three parts: the new training objective Soft Score Matching, the novel sampling method Momentum Sampler, and the scheduling of the corruption mechanism.
First, let’s look at the training objective Soft Score Matching. The name is inspired by soft filtering, a photographic term that refers to filters that remove fine details. It learns the scores of regular linear corruption processes in a provable way and incorporates the filtering process into the network, training the model to predict images that match the diffusion observations after corruption.
As long as diffusion assigns non-zero probabilities to any clean, corrupted image pairs, this training objective can be proven to learn the scores. Additionally, this condition is always satisfied when there is additive noise in the corruption.
Specifically, the researchers explored the corruption process in the following form.
During the process, the researchers found that noise is important for both empirical (i.e., better results) and theoretical (i.e., for learning scores) aspects. This also became a key distinction from the concurrent work Cold Diffusion that reverses deterministic corruption.
Next is the sampling method Momentum Sampling. The researchers demonstrated that the choice of sampler has a significant impact on the quality of generated samples. They proposed Momentum Sampler for reversing general linear corruption processes. This sampler uses a convex combination of corruptions at different diffusion levels and is inspired by momentum methods in optimization.
This sampling method is inspired by the continuous formulation of diffusion models proposed in the aforementioned paper by Yang Song et al. The algorithm for Momentum Sampler is as follows.
The following figure visually demonstrates the impact of different sampling methods on the quality of generated samples. The image on the left, sampled using the Naive Sampler, appears to have repetitions and lacks details, while the image on the right, using the Momentum Sampler, significantly improves sampling quality and FID scores.
Lastly, regarding scheduling. Even when the type of degradation is predefined (e.g., blurring), determining how much to corrupt at each diffusion step is not easy. The researchers proposed a principled tool to guide the design of the corruption process. To find the scheduling, they minimized the Wasserstein distance between distributions along the path. Intuitively, the researchers want to transition smoothly from a fully corrupted distribution to a clean distribution.
Experimental Results
The researchers evaluated the proposed methods on CelebA-64 and CIFAR-10, both standard baselines for image generation. The main purpose of the experiments was to understand the role of corruption types.
The researchers first attempted corruption using blurring and low amplitude noise. The results indicate that their proposed model achieved state-of-the-art results on CelebA, with an FID score of 1.85, surpassing all other methods that only added noise or possibly rescaled images. Additionally, the FID score obtained on CIFAR-10 was 4.64, which, while not state-of-the-art, is still competitive.
Furthermore, on the CIFAR-10 and CelebA datasets, the researchers’ methods also performed better on another metric, sampling time. Another additional benefit is the significant computational advantage. Compared to image generation denoising methods, deblurring (with almost no noise) seems to be a more effective manipulation.
The figure below shows how the FID score changes with the number of function evaluations (NFE). From the results, it can be seen that the researchers’ model can achieve the same or better quality as the standard Gaussian denoising diffusion model with significantly fewer steps on the CIFAR-10 and CelebA datasets.
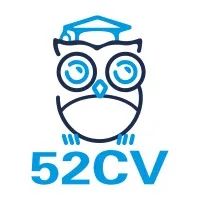
END
Welcome to join the “Computer Vision“ communication group👇 Please note:CV