
Author: Leonie Monigatti
Translation: Zhao Jiankai
Proofreading: zrx
This article is approximately 4800 words long and is recommended for a 7-minute read.
This article introduces you to the LangChain framework.
-
A chatbot tailored to your specific data -
A personal assistant that interacts with the outside world -
Summaries of your documents or code
What is LangChain?
LangChain is a framework designed to help you more easily build LLM-supported applications by providing you with:
-
A universal interface for various foundational models (see Models);
-
A framework to help you manage prompts (see Prompts);
-
And a central interface for long-term memory (see Memory), external data (see Index), other LLMs (see Chains), and other agents that LLMs cannot handle (e.g., computation or search).
This is an open-source project created by Harrison Chase (GitHub repository).
Due to the many features of LangChain, we will discuss the six key modules of LangChain in this article to give you a better understanding of its capabilities.
pip install langchain
import langchain
import os
os.environ["OPENAI_API_KEY"] = ... # insert your API_TOKEN here
-
BLOOM by BigScience -
LLaMA by Meta AI -
Flan-T5 by Google -
GPT-J by Eleuther AI
import os
os.environ["HUGGINGFACEHUB_API_TOKEN"] = ... # insert your API_TOKEN here
Personal Notes: You can try open-source foundational models here. I attempted to make this tutorial work only with open-source models hosted on Hugging Face under regular accounts (google/flan-t5-xl and sentence transformer/all-MiniLM-L6-v2). It works for most examples, but getting some examples to work was also a pain. In the end, I set up a paid account with OpenAI because most examples in LangChain seem to be optimized for the OpenAI API. Overall, running some experiments for this tutorial cost me about $1.
-
Models: Choose from different LLMs and embedding models -
Prompts: Manage LLMs -
Input Chains: Combine LLMs with other components -
Index: Access external data -
Memory: Remember previous conversations -
Agents: Access other tools
-
LLMs take strings as input (prompts) and output strings (completions).
# Proprietary LLM from e.g. OpenAI
# pip install openai
from langchain.llms import OpenAI
llm = OpenAI(model_name="text-davinci-003")
# Alternatively, open-source LLM hosted on Hugging Face
# pip install huggingface_hub
from langchain import HuggingFaceHub
llm = HuggingFaceHub(repo_id = "google/flan-t5-xl")
# The LLM takes a prompt as an input and outputs a completion
prompt = "Alice has a parrot. What animal is Alice's pet?"
completion = llm(prompt)
LLM Models
-
Chat Models are similar to LLMs. They take a list of chat messages as input and return chat messages. -
Text Embedding Models take text input and return a list of floats (embeddings), which are numerical representations of the input text. Embeddings help extract information from text. This information can then be used, for example, to calculate similarity between texts (e.g., movie summaries).
Text Embedding Models
Prompts: Managing LLM Inputs
LLMs have quirky APIs. Although inputting prompts to LLMs in natural language should feel intuitive, a lot of adjustments are needed to the prompts before obtaining the desired output from the LLM. This process is called prompt engineering. Once you have a good prompt, you may want to use it as a template for other purposes. Therefore, LangChain provides you with what is called a prompt template to help you build prompts from multiple components.
from langchain import PromptTemplate
template = "What is a good name for a company that makes {product}?"
prompt = PromptTemplate( input_variables=["product"], template=template,)
prompt.format(product="colorful socks")
from langchain import PromptTemplate, FewShotPromptTemplate
examples = [ {"word": "happy", "antonym": "sad"}, {"word": "tall", "antonym": "short"},]
example_template = """Word: {word}Antonym: {antonym}\n"""
example_prompt = PromptTemplate( input_variables=["word", "antonym"], template=example_template,)
few_shot_prompt = FewShotPromptTemplate( examples=examples, example_prompt=example_prompt, prefix="Give the antonym of every input", suffix="Word: {input}\nAntonym:", input_variables=["input"], example_separator="\n",)
few_shot_prompt.format(input="big")
Give the antonym of every input
Word: happyAntonym: sad
Word: tallAntonym: short
Word: bigAntonym:
from langchain.chains import LLMChain
chain = LLMChain(llm = llm, prompt = prompt)
# Run the chain only specifying the input variable.chain.run("colorful socks")
from langchain.chains import LLMChain, SimpleSequentialChain
# Define the first chain as in the previous code example# ...
# Create a second chain with a prompt template and an LLMsecond_prompt = PromptTemplate( input_variables=["company_name"], template="Write a catchphrase for the following company: {company_name}",)
chain_two = LLMChain(llm=llm, prompt=second_prompt)
# Combine the first and the second chain overall_chain = SimpleSequentialChain(chains=[chain, chain_two], verbose=True)
# Run the chain specifying only the input variable for the first chain.catchphrase = overall_chain.run("colorful socks")
Example Result
# pip install youtube-transcript-api
# pip install pytube
from langchain.document_loaders import YoutubeLoader
loader = YoutubeLoader.from_youtube_url("https://www.youtube.com/watch?v=dQw4w9WgXcQ")
documents = loader.load()
# pip install faiss-cpu
from langchain.vectorstores import FAISS
# create the vectorestore to use as the index
db = FAISS.from_documents(documents, embeddings)
from langchain.chains import RetrievalQA
retriever = db.as_retriever()
qa = RetrievalQA.from_chain_type( llm=llm, chain_type="stuff", retriever=retriever, return_source_documents=True)
query = "What am I never going to do?"
result = qa({"query": query})
print(result['result'])
Example Result
Comparison of Chatbots with and without Memory
-
Retain all conversations -
Retain the latest k conversations -
Summarize conversations
from langchain import ConversationChain
conversation = ConversationChain(llm=llm, verbose=True)
conversation.predict(input="Alice has a parrot.")
conversation.predict(input="Bob has two cats.")
conversation.predict(input="How many pets do Alice and Bob have?")
# pip install wikipedia
from langchain.agents import load_tools
from langchain.agents import initialize_agent
from langchain.agents import AgentType
tools = load_tools(["wikipedia", "llm-math"], llm=llm)
agent = initialize_agent(tools, llm, agent=AgentType.ZERO_SHOT_REACT_DESCRIPTION, verbose=True)
agent.run("When was Barack Obama born? How old was he in 2022?")
Translator Introduction
Author Introduction
Zhao Jiankai, a graduate student in Management Science and Engineering at Zhejiang University, focuses on the application of machine learning in social commerce.
Translation Group Recruitment Information
Job Description: Requires a meticulous heart to translate selected foreign articles into fluent Chinese. If you are an overseas student in data science/statistics/computer-related fields, or working abroad in related jobs, or are confident in your foreign language proficiency, you are welcome to join the translation team.
You will get: Regular translation training to improve volunteers’ translation skills, enhance awareness of cutting-edge data science, and overseas friends can stay in touch with domestic technological application development. The THU Datapi industry-university-research background offers good development opportunities for volunteers.
Other Benefits: You will have the opportunity to work with data scientists from renowned companies, students from prestigious universities like Peking University and Tsinghua University, and other overseas institutions.
Click on the end of the article “Read the Original” to join the Datapi team~
Reprint Notice
For reprints, please prominently indicate the author and source at the beginning (transferred from: Datapi ID: DatapiTHU), and place a prominent QR code of Datapi at the end of the article. For articles with original markings, please send [Article Name – Pending Authorized Public Account Name and ID] to the contact email to apply for whitelist authorization and edit according to requirements.
Please feedback the link to the contact email after publication (see below). Unauthorized reprints and adaptations will be pursued legally.
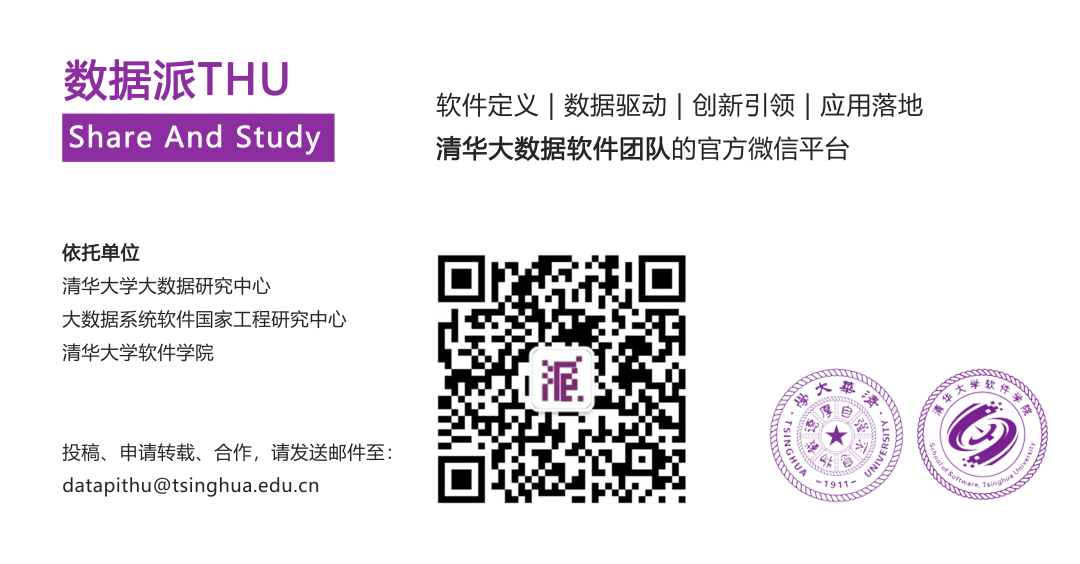
Click “Read the Original” to embrace organization