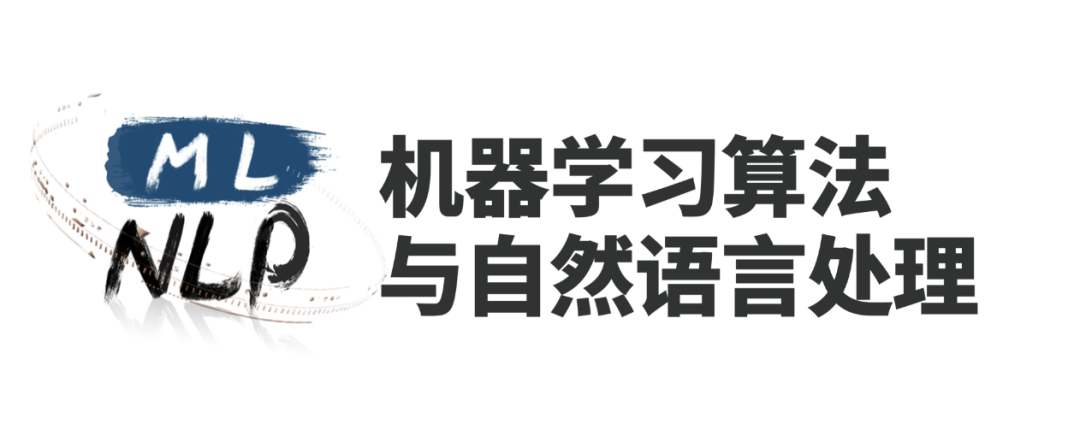
MLNLP community is a well-known machine learning and natural language processing community in China and abroad, covering NLP master’s and doctoral students, university teachers, and corporate researchers.
The community’s vision is to promote communication and progress between the academic and industrial circles of natural language processing and machine learning at home and abroad, especially for the progress of beginners.
Reprinted from | AI Technology Review
Authors | Li Mei, Chen Fangyuan
Editor | Chen Caixian
As a powerful, open, and sufficiently simple model, the recently popular Stable Diffusion provides unlimited creative possibilities beyond text-to-image generation.
Recently, Justin Pinkney, a machine learning researcher from Lambda Labs, fine-tuned this model to build a Pokémon generator!
Let’s take a look at some interesting examples~
The following images are some Pokémon generated after inputting names: a girl with a pearl earring, Obama, Trump, Boris Johnson, Totoro, Hello Kitty.
Lady Gaga, Boris Johnson, Putin, Merkel, Trump, Plato:
In addition to existing characters and public figures, you can also input a description to generate your imagined Pokémon: a skeleton priest.
You can also input your name or nickname to generate your own Pokémon image. This is so cool, as Twitter users have been creating based on their names to see what they would look like as Pokémon.
Caption: Pokémon image of Twitter user Jo Barf Creepy
Caption: Pokémon image of Twitter user Elizabeth Holmes
Caption: Pokémon image of Twitter user Upbeatblue
Caption: Pokémon image of Twitter user Onion-sama
Inputting names of cartoon characters can also yield corresponding Pokémon:
And those Pokémon that accompanied people in their childhood also have new appearances in this generator: Pikachu, Bulbasaur, Charizard, Treecko, Lucario, Mew.
How the Pokémon Generator Works
Pinkney showcased the training process of this Pokémon generator on Twitter.
Link: https://github.com/LambdaLabsML/examples/tree/main/stable-diffusion-finetuning
He stated that Stable Diffusion is a great general model, but obtaining outputs in a specific style is not easy, which usually requires doing a lot of tedious work to create complex text prompt libraries, or you can take the shortcut of fine-tuning the image generation model.
Pinkney fine-tuned the initial Stable Diffusion on a dataset of Pokémon images.
First, he built a dataset. The dataset contains Pokémon images and corresponding text descriptions, such as Bulbasaur being described as “an image of a green Pokémon with red eyes,” while Caterpie is described as “a green-yellow toy with a red nose.”
Of course, these descriptions were not done manually, but were generated using a neural network, specifically the image description model BLIP. Although these descriptions are not perfect, they are sufficient.
Then, he spent only a few hours training the AI model on an A6000, allowing the model to learn to generate images in the style of Pokémon, while retaining previous knowledge for a while, ultimately leading to overfitting on the dataset.
Initially, the samples looked like normal images, then gradually acquired the Pokémon style, and as training continued, eventually presented a Pokémon image that differed from the original prompt:
This is a very simple fine-tuning, but it runs extremely well. With this fine-tuned model, no matter what prompt you give it, it will generate Pokémon. So there’s no need to rack your brains for prompts anymore.
When creating Pokémon, you can choose to output multiple:
Caption: Mechanical cat with wings
Pinkney stated that everyone is welcome to use this model in more complex ways in new fields. Such small tools exemplify the benefits of open-source AI models like Stable Diffusion.
After this model sparked a creative frenzy online, Pinkney published a blog with some additional work details.
He found it surprising that this model managed to retain some general knowledge from the initial Stable Diffusion, even though it was trained on a limited dataset for only a few thousand steps. However, when fine-tuning for Pokémon, the model quickly began to overfit; if sampled in a simple way, the model would generate nonsensical Pokémon for new prompts, meaning it had catastrophically forgotten the original data it was trained on. However, Stable Diffusion retains an exponentially moving average (EMA) version of the model during training, which is usually used for inference.
Thus, when using EMA weights, we are actually using an average of the original model and the fine-tuned model. It turns out that this is essential for generating Pokémon. Additionally, you can fine-tune the model’s effects by averaging the new model’s weights with those of the initial model to control the number of generated Pokémon. Fine-tuning and averaging the model can effectively mix the original content with the fine-tuned style.
Caption: The left is a fully fine-tuned model, and the right is a model that only fine-tuned the attention layer.
Moreover, you can freeze different parts of the model for fine-tuning; for example, the image above shows the generation effects of two fine-tuning methods, where the model that only fine-tuned the attention layer can generate a more normal Yoda but is not very good at making Pokémon.
Reference link: https://www.justinpinkney.com/pokemon-generator/
Technical Group Invitation
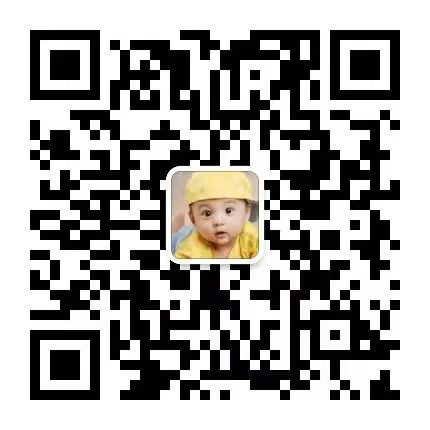
△ Long press to add assistant
Scan the QR code to add the assistant WeChat
Please note: Name-School/Company-Research Direction
(e.g., Xiao Zhang-Harbin Institute of Technology-Dialogue Systems)
to apply for joining Natural Language Processing/Pytorch and other technical groups
About Us
MLNLP community is a grassroots academic community jointly built by scholars in machine learning and natural language processing from home and abroad. It has now developed into a well-known machine learning and natural language processing community at home and abroad, aimed at promoting progress among the academic and industrial circles of machine learning and natural language processing and a wide range of enthusiasts.
The community can provide an open exchange platform for the further education, employment, and research of related practitioners. Everyone is welcome to follow and join us.
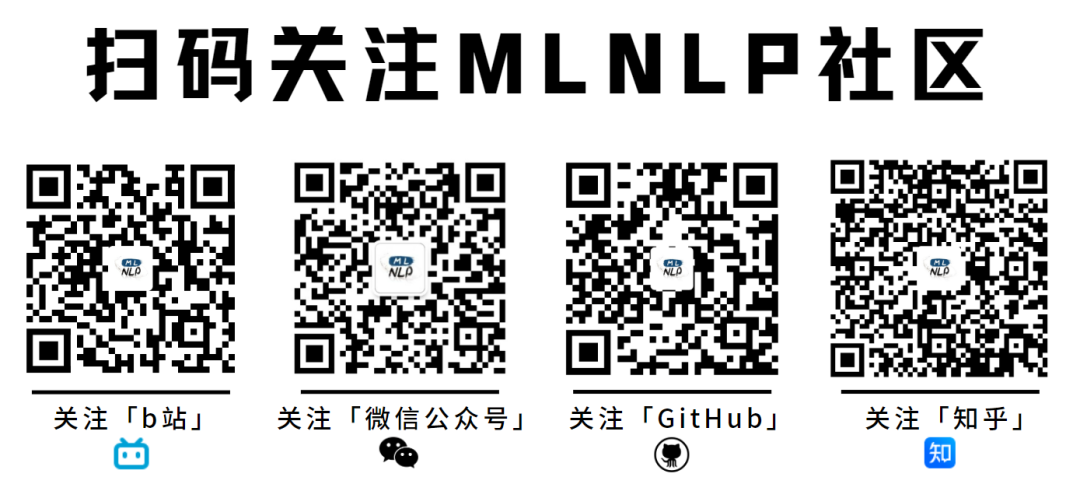