Yan Hanbing1, Yang Shuting2, Yu Shuzhen2, Chen Yi2
(1. School of Open Learning and Education, East China Normal University, Shanghai 200062;
2. Department of Education Information Technology, East China Normal University, Shanghai 200062)
[Abstract] Immersive learning environments have created a high-quality learning space for cultivating new quality talents, but their applications in the education field remain limited due to factors such as high technological costs, lack of educational theories, and low applicability of learning. Generative artificial intelligence demonstrates significant potential in creative generation, logical expression, and complex interaction understanding. This paper, based on the review of the connotation and components of immersive learning environments, constructs a vertical application mechanism model of generative artificial intelligence in immersive learning environments. Through scenario decomposition, model refinement, and content creation, this model achieves functions such as learning path planning, multimodal resource generation, learning context creation, personalized interaction, and learner profiling. Subsequently, from the practical perspective, this paper proposes an effective learning model based on immersive learning environment, which includes five stages: anchoring objectives, understanding the current situation, course learning, scenario practice, and dynamic monitoring. Finally, the operability of the vertical application mechanism and learning model is demonstrated by the “Zhi Xin Hui Yu” intelligent coaching system, aiming to provide a reference for the vertical application of generative artificial intelligence in the field of education.
[Keywords] Generative Artificial Intelligence; Immersive Learning; Vertical Mechanism; Learning Model; Application Case
In the era of digital intelligence, in response to the call to “accelerate the formation of new productive forces,” the education field should actively innovate learning models to cultivate top innovative talents with both innovative capabilities and practical wisdom[1]. The learning environment is the main place where learning occurs; immersive learning environments, characterized by embodiment, interactivity, and contextualization, are effective technological innovation pathways for enhancing learning motivation[2], supporting proactive knowledge construction, promoting practical skill transfer[3], cultivating problem-solving abilities[4], and developing innovative thinking[5]. However, the application of immersive learning environments in the education field still faces various limitations. For example, in terms of technical implementation, the current development of immersive learning environments often relies on technologies such as XR, digital twins, and affective computing, which have high development costs, poor compatibility among hardware, systems, and content, hindering widespread adoption; in terms of content adaptation, current scenario and interaction designs often depend on preset scripts, with insufficient depth in narrative and immediate feedback, leading to limited capability growth for learners[6]; moreover, the educational application development of immersive technologies typically lacks guidance from learning theories, neglecting the intrinsic mechanisms of technology empowering learning[5]. In the face of the need to expand application scale, enhance learning experience, and promote deep learning, it is imperative to innovate the development path of immersive learning environments, reduce technical costs, enhance content adaptation, and promote learning outcomes.
In recent years, generative artificial intelligence products, with their powerful dialogue context understanding and generative content capabilities, are sparking a wave of innovation in intelligent education globally. Personalized learning resource generation and recommendation, high-frequency interaction and immediate feedback based on generative artificial intelligence, and the resulting innovative learning models provide a foundation for creating immersive learning environments with rich learning experiences, supporting high-quality interactions and adaptive adjustments. Therefore, this study aims to explore the vertical application mechanisms and practical models of generative artificial intelligence in immersive learning environments based on understanding the connotation and components of immersive learning environments, responding to the theoretical and practical issues of “generative artificial intelligence empowering immersive learning.”
1. The Connotation and Components of Immersive Learning Environments
Existing research often defines immersive learning environments from three perspectives: technical applications, psychological changes, and knowledge acquisition. From the perspective of technical applications, immersive learning environments emphasize the use of technologies such as virtual reality, augmented reality, and 3D modeling[4], surrounding learners with images, sounds, or other stimuli to create virtual environments that closely resemble the real world[7]. From the perspective of psychological change, authors such as Csikszentmihalyi interpret “immersion” as “flow”[8], where individuals are fully engaged in an activity, forgetting the surrounding environment and the passage of time, achieving a state of selflessness[9]. Based on this, immersive learning environments not only refer to physical immersion based on technological integration but also imply a deeper immersion that integrates physiological and psychological experiences[10]. From the perspective of knowledge acquisition, the International Immersive Learning Research Network (iLRN) points out that rich knowledge and learner-centered models are key elements of immersive learning experiences[11]. Combining the above viewpoints, immersive learning environments can be defined as a learning environment that utilizes advanced technological means to integrate digital learning resources such as text, images, and sounds, creating realistic learning environments that provide learners with deep, immersive learning experiences, thereby enhancing learning engagement and promoting knowledge construction.
2. Components of Immersive Learning Environments
Cognitive, relational, and contextual characteristics are three typical features of immersive learning environments[12]. Firstly, promoting cognitive development is the ultimate goal of immersive learning; immersive learning environments facilitate learners’ cognitive processing and knowledge construction by providing modular learning content, expressive multimedia learning resources, complex learning tasks, progressively deepening learning activities, and systematically scientific learning methods. Secondly, associating learning behaviors with system feedback is a key channel for immersive learning to occur[13]. By creating interactive experiences to collect learning behavior data and providing immediate feedback based on analysis results, such as goal adjustments, resource recommendations, and task matching, a foundation is laid for the development of personalized, adaptive immersive learning environments. Finally, contexts that match learners’ personalities are important factors influencing the immersive experience[14]. By providing environments, roles, plots, and tasks that align with practical scenarios, immersive learning experiences can evoke resonance and emotional engagement, supporting learners in transforming and recreating objective knowledge, thereby enhancing their practical abilities and socialization levels.
To gain a deeper understanding of the operational mechanisms of immersive learning environments and to optimize design and application, this study categorizes them into three types of components: cognitive, relational, and contextual elements. The cognitive components focus on knowledge acquisition, including learning content, resources, activities, and methods; the relational components focus on the technical means that support learning, including human-computer interaction and learning behavior analysis; the contextual components focus on enhancing the immersive experience, including environmental background, role characteristics, plot design, and task requirements.
3. Innovative Paths of Generative Artificial Intelligence Empowering Immersive Learning Environments
Generative artificial intelligence is a technology that automatically generates text, images, videos, and audio based on prompts, possessing strong capabilities in dialogue context understanding, generative content creation, sequential task execution, and programming language parsing[15]. This study dissects educational technology application cases based on the three types of components of immersive learning environments, exploring the technical points of generative artificial intelligence empowering immersive learning environments.
1. Achieving Adaptive Generation and Pushing of Multimodal Resources
By integrating generative artificial intelligence into digital learning systems, the application model of educational resources shifts from “diagnosis-customization-distribution” to “aggregation-processing-generation,” forming personalized pathways for the production and utilization of learning resources[16]. For example, Wu et al. developed the intelligent tutorial generation system Self-GT, which generates personalized tutorials by capturing learners’ dynamic changes and behavioral characteristics[17]; the University of Michigan constructed a knowledge base called Learning Clues, which includes teaching videos, presentations, and electronic textbooks, providing learners with high-quality knowledge Q&A and resource recommendations[18].
2. Supporting Full Process Optimization of Learning
Generative artificial intelligence supports knowledge understanding and deep learning by providing assistance at all stages of learning. For example, Khan Academy’s chatbot Khanmigo can develop personalized learning plans for learners before class; explain complex concepts in a dialogue format during class, create tasks, and encourage independent problem-solving; and create learning evaluation scales to grade assignments and conduct comprehensive assessments after class[19]. In the future, intelligent companions based on generative artificial intelligence will continue to excel in areas such as information retrieval, knowledge explanation, skill training, and growth companionship[20].
3. Depicting Dynamic and Accurate Learner Profiles
Generative artificial intelligence generates massive real-time learning behavior data through interactive learning services such as human-computer dialogue, providing a normalized data foundation for constructing learner profiles. By integrating multidimensional data such as learning outcomes, evaluations, diagnostics, and preferences, the completeness, accuracy, and timeliness of learner profiles can be enhanced[21]. On this basis, intelligent analysis of learners’ learning progress, interests, and needs can be conducted based on learner profiles to customize personalized development paths[22].
4. Conducting Continuous and In-Depth Dialogical Interaction
Generative artificial intelligence possesses outstanding capabilities for conducting multi-turn dialogues. The interaction form between learners and intelligent learning systems has shifted from traditional menu-based to progressive Q&A. For example, the Socratic Playground helps learners understand data analysis and processing knowledge through frequent dialogue interactions[23]. Additionally, dialogical interaction simplifies interaction forms, reducing the adverse effects of complex operations on learning. Generative artificial intelligence is driving intelligent learning towards a new stage of intelligent inquiry[24].
5. Creating Realistic and Diverse Learning Contexts
Generative artificial intelligence can break the limitations of traditional preset scripts, creating rich and diverse learning contexts, and adjusting context content in real-time to provide learners with personalized learning experiences. For example, Shi et al. integrated legal knowledge and cases into a series of continuous contexts using generative artificial intelligence, conducting legal education through dialogue interactions[25]; Breen used ChatGPT to create a medieval life context, leading students to immerse themselves in historical knowledge[26].
In summary, generative artificial intelligence provides feasible solutions for current immersive learning environments to overcome limitations such as insufficient service adaptability, single context design, and poor learning outcomes through the generation and pushing of multimodal learning resources, optimization of the learning process, construction of learner profiles, dialogical Q&A, and creation of diverse learning contexts, injecting strong technological momentum into immersive learning.
The five innovative paths of generative artificial intelligence empowering immersive learning environments need to be grounded in specific technical implementation mechanisms. Firstly, context is a core feature of immersive learning environments and also an element that promotes meaningful knowledge construction for learners[12]. By decomposing contexts, focusing on specific learning objectives and micro-abilities, the context can be treated as the smallest compositional unit[27], enhancing the relevance and practicality of generated content. Secondly, to ensure that generative artificial intelligence products can understand and process task information related to specific fields while alleviating potential issues with the technology itself, such as hallucination problems[28], the key in vertical applications lies in: based on invoking general large language models, domain experts inject professional knowledge into prompts to deepen the model’s understanding of the vertical domain context, generating specific outputs[29]. Following this technical implementation approach, model refinement serves as a quality assurance mechanism, constructing an expert knowledge base to ensure the accuracy and reliability of generated content. Finally, with content creation as the ultimate goal, a stable and high-quality generation of immersive learning environments can be achieved through multi-agent collaboration[30]. Based on these ideas, this study constructs a vertical application mechanism model of generative artificial intelligence in immersive learning environments, as shown in Figure 1.
1. Context Decomposition: Structuring Courses in a Systematic Manner
Context is the best carrier for generating immersive experiences. Based on the requirements for professional talent cultivation and disciplinary development goals, courses are systematically decomposed into multiple learning modules focused on specific themes or skills, with interconnections and progressions among the modules. On this basis, the modules are further subdivided into several learning contexts, each with specific learning objectives, content, methods, tasks, and assessments, ensuring that the details of learning are implemented and carried out.
This structured course organization method effectively conveys complex learning content, ensures the gradual achievement of course objectives, and provides learners with a coherent learning experience while ensuring the applicability of supporting learning resources for each context. At the same time, it also makes it feasible to customize learning content and plan personalized learning paths based on the needs and levels of learners.
2. Model Refinement: Constructing an Expert Knowledge Base Based on Vertical Data
Due to limitations in data acquisition channels, legal and ethical issues, resources, and costs, mainstream large language models’ training sets often rely on publicly available data from the internet, making it difficult to obtain real-time, non-public, or offline data in vertical fields, thus lacking relevant knowledge. Constructing an expert knowledge base based on vertical data is a key technical means, specifically including data collection and knowledge construction stages.
During the data collection stage, the expert knowledge base should include but not be limited to: subject knowledge datasets, learning activity datasets, methodology datasets, learning context datasets, and learning behavior datasets, referring to the three types of components of immersive learning environments. These datasets can come from various learning resource repositories, authoritative experts, typical practice cases, as well as relevant research results from universities, research institutions, or international organizations. By integrating high-quality data from multiple sources, the professional knowledge system of the large model can be enriched. In the knowledge construction stage, the data is reasonably classified and transformed into knowledge based on the model. For example: constructing subject knowledge graphs with hierarchical and relational features; designing learning activity models based on the constructivist learning theory perspective that encompass teaching, learning, practicing, testing, and evaluating; methodology models that include professional methods, teaching methods, learning methods, and innovative methods; learning context models that extract elements such as environmental background, key roles, plot design, and task requirements based on situational learning theory; and role feature models that extend to include identity background, personality traits, language styles, interaction patterns, and behavioral motivations; as well as learning behavior models that include indicators such as time frequency, learning paths, task submissions, exam performance, interaction participation, and resource usage. Subsequently, the knowledge is organized into a structured expert knowledge base using vectorization for intelligent system invocation and application.
3. Content Creation: Integrating Multi-Agents to Execute Complex Tasks
Immersive learning environments are complex systems with multiple overlapping attributes, including dynamic contextual interactions, effective knowledge transmission, and personalized learning needs. Therefore, relying solely on a single large model and brief prompts cannot achieve truly effective immersive learning. Intelligent agents are systems or programs capable of autonomously executing specific tasks or making decisions, hailed as a milestone creation in human existence and cultural forms[31]. Constructing a complex system integrating multiple intelligent agents based on generative artificial intelligence is a feasible path for achieving automated and intelligent generation of immersive learning environments.
In terms of technical implementation, retrieval-augmented generation (RAG) based on large language models provides a solution for constructing intelligent agents. Semantic retrieval and generation output are the two main steps. Here, semantic retrieval refers to when learners issue specific task instructions, RAG uses these as search terms to retrieve relevant knowledge from the expert knowledge base, injecting it as key background information along with the task instructions into the prompts; generation output refers to RAG applying this prompt to interact with the large language model to generate corresponding content. Additionally, to optimize output, other instructions related to the intelligent agent’s functions can also be appropriately included in the prompts. By encapsulating the instructions for semantic retrieval and generation output, intelligent agents can enhance human-computer interaction effects, making the output content more precise.
The operation of intelligent agents mainly follows four stages: observation, thinking, action, and memory. “Observation” is the perception mechanism, where intelligent agents receive text data, visual data, or audio data from users or other intelligent agents, providing a basis for subsequent actions. “Thinking” is the process of analyzing the results of “observation,” during which the expert knowledge base and large language model are invoked to drive decision-making. In addition to the expert knowledge base, intelligent agents typically also store a topical knowledge base composed of real-time, easily accessible, unstructured vertical datasets, such as scattered course resources on the internet, professional books, and Q&A forums, which can be called upon as needed to enhance the breadth and depth of “thinking.” “Action” is the response to “observation” and “thinking,” manifested in generating contexts, conducting teaching guidance, etc. “Memory” stores past “action” experiences, which are also part of the topical knowledge base, assisting intelligent agents in referencing and adjusting actions during the next “thinking” stage.
Based on generative artificial intelligence, immersive learning environments consist of multiple intelligent agents with different functions, efficiently collaborating to break down the generation process of immersive learning environments into multiple steps, executing them in phases, and autonomously iterating based on learner feedback, providing strong support for the implementation of gradual learning paths, multimodal learning resources, realistic and diverse learning contexts, continuous and in-depth personalized interactions, and dynamic and accurate learner profiles, ultimately stably generating high-quality immersive learning environments.
Research has found that highly immersive learning environments may distract learners from focusing on core content, affecting knowledge acquisition and learning outcomes[32]. Researchers call for the design and development of immersive learning environments to be guided by learning theories[5]. Bransford proposed four principles for designing and implementing learning environments that promote learning: learner-centered, knowledge-centered, assessment-centered, and community-centered[33]. Among them, learner-centered means that new learning should be built upon learners’ prior knowledge, experiences, and beliefs; knowledge-centered means that teaching should focus on core concepts and structures in the field, developing learners’ overall understanding of the discipline; assessment-centered means providing learners with timely and continuous feedback throughout the learning process; community-centered means establishing a culture of mutual learning and collaboration to promote individual and community learning and development. Based on these principles, this study further explores the immersive learning model supported by generative artificial intelligence from the perspective of promoting learning occurrence (as shown in Figure 2), clarifying the application of immersive learning environments.
1. Decomposing Learning Abilities: Anchoring Learning Objectives
First, learners input their basic information and learning needs, and the system uses natural language processing technology to identify the targeted learning objectives and courses, calling the learning design intelligent agent to break down the objectives into several sub-objectives. Secondly, it links knowledge points with the knowledge graph and prioritizes them, automatically generating personalized learning paths that include learning content, activities, and methods based on learners’ preferences. Finally, experts evaluate the scientificity, accuracy, and feasibility of the learning objectives.
2. Learner-Centered: Understanding the Current Learning Situation
Learners conduct learning based on the learning path. The system calls the context creation intelligent agent to initially create the learning context, and the role-playing intelligent agent configures the dialogue module based on the context and knowledge graph to pose a series of guiding questions to the learners, who respond to relevant questions in a dialogical format. Subsequently, the behavior analysis intelligent agent employs natural language processing technology, emotional recognition technology, etc., to diagnose learning situations, clarifying current knowledge retention, learning attitudes, learning difficulties, and dynamically adjusting learning paths.
3. Knowledge-Centered: Conducting Course Learning
Based on learning objectives and basic learning situations, the system calls the resource creation intelligent agent to generate multimodal course learning resources. On one hand, resources can take various forms, such as explanatory videos, online quizzes, and simulation experiments; on the other hand, they can generate multiple modal resources based on learners’ preferences and cognitive styles, such as visual types focusing on text, images, and videos, auditory types focusing on audio, and dynamic types focusing on interactive simulations. Additionally, resources are dynamically updated based on learners’ progress and feedback to ensure demand matching.
4. Community-Centered: Experiencing Contextual Practice
To deepen learners’ understanding of knowledge and mastery of skills, the system again calls the context creation intelligent agent to generate realistic practice contexts and publish contextual tasks, such as completing projects. Learners and teaching guidance intelligent agents form a human-machine community, collaboratively discussing solutions through dialogue interactions. During this process, intelligent agents provide necessary learning support, suggestions, and feedback, dynamically adjusting guidance strategies based on learners’ performance and task progress.
5. Assessment-Centered: Implementing Dynamic Monitoring
Dynamic monitoring runs through the entire immersive learning process. The system calls the behavior analysis intelligent agent to continuously track learning behaviors, such as activity participation, task completion rates, and the frequency and quality of interactions with intelligent agents, and transmits the results in real-time to other intelligent agents. In addition, intelligent agents will conduct comprehensive assessments after the course and tasks, providing a basis for improving future learning plans. Furthermore, the massive data generated during the process will also supplement learners’ basic information, supporting real-time optimization of immersive learning environments.
1. Case Introduction
“Zhi Xin Hui Yu” is an intelligent coaching system characterized by supporting scenario-based training, as shown in Figure 3. This system focuses on key issues and special rules in family education, aiming to provide parents with high-quality, customized, full-cycle family education services by creating an immersive learning environment supported by generative artificial intelligence, promoting the scientific and professional development of family education[34].
2. Case Analysis
1. System Design Mechanism
The development framework of the system is mainly achieved through three key steps: “context decomposition-model refinement-content creation.”
Context Decomposition: Constructing a Parent-Child Capability Map. Aiming at enhancing parents’ educational literacy, common family education issues are identified, extracting core skills such as setting reasonable educational expectations and cultivating children’s self-awareness and self-esteem. These core skills can be further subdivided into several micro-abilities and matched with specific contexts. For example, setting reasonable educational expectations can be divided into: correctly understanding children’s abilities, setting reasonable goals, and dynamically adjusting educational expectations, thus constructing a parent-child capability map to support the planning of learning paths and the development of learning resources.
Model Refinement: Constructing a Family Education Expert Knowledge Base. A large number of theoretical models are introduced to enrich the expert knowledge base, including various micro-ability models composed of emotional regulation process models, multiple intelligences theory, self-determination theory, etc., and question models centered on the Socratic teaching method, as well as role feature models based on the five-factor theory, to enable the system to call upon them as needed.
Content Creation: Supporting Practical Training in Family Education Scenarios. Multiple intelligent agents are created, and efficient collaboration is achieved based on workflows, with the context creation intelligent agent creating learning contexts based on parent profiles; the role-playing intelligent agent playing the child and configuring the dialogue module based on the context; and the behavior analysis intelligent agent collecting parents’ dialogue behaviors and analyzing learning behaviors, transmitting the results to the role-playing intelligent agent while updating the parent profile, ensuring the effectiveness and adaptability of the generated family education learning context.
2. System Application Model
The system has constructed an immersive learning model comprising four stages: “context diagnosis-course learning-cognitive assessment-intelligent coaching.”
Context Diagnosis: Planning Learning Paths Based on Parents’ Capability Status. Parents independently select micro-ability points, and the system generates realistic contexts by combining parent profiles, simulating dialogues with the child. After submitting the diagnosis, parents’ behaviors are extracted and analyzed to present their capability performance levels. By providing precise and personalized learning situation diagnostics, it ensures that learning content and training contexts can highly match each parent’s actual needs.
Course Learning: Supporting Theoretical Learning with High-Quality Digital Courses. Based on the context diagnosis results, the system recommends courses that match key skills and core knowledge from the “School-Family-Society Collaborative Education Theme Digital Course Group” developed by the school, supplemented with actionable and referable learning resources such as behavior guidelines and typical cases, supporting parents’ understanding and internalization of theoretical knowledge.
Cognitive Assessment: Providing Professional Feedback to Parents. Referring to learning objectives and course content, appropriate scales are selected to accurately assess the effectiveness of course learning, providing personalized and professional learning feedback and suggestions to parents, dynamically adjusting learning paths.
Intelligent Coaching: Strengthening Practical Application in the Form of a Human-Machine Community. Parents and the system form a learning community. Supported by the thinking model, the system simulates children’s real emotional reactions and behavioral patterns in different contexts, providing parents with real-time nonlinear feedback. This supports parents in repeatedly practicing and accumulating experience through multiple rounds of dialogue interactions, effectively enhancing their parent-child skill levels.
It can be observed that the design mechanism and application model of the “Zhi Xin Hui Yu” intelligent coaching system closely align with the vertical application mechanism of generative artificial intelligence in immersive learning environments and the immersive learning model proposed in this study. While providing sufficient evidence, the system still has room for optimization and iteration in areas such as adaptive learning path design and multimodal learning resource generation.
With the development of generative artificial intelligence, immersive learning environments are ushering in new development opportunities through embedded demand-driven dialogical interaction models, constructing realistic and diverse learning contexts, creating personalized multimodal learning resources, and achieving stable and flexible knowledge output, giving rise to new forms of immersive learning aimed at mastery learning. The application of new technologies needs to be supported by new underlying architectures. The vertical application mechanisms and learning models of generative artificial intelligence in immersive learning environments proposed in this study are reflections called forth by practice. By segmenting learning contexts, constructing expert knowledge bases, and creating multi-agents, efforts are made to avoid the negative impacts of “hallucinations,” improve the quality of generated content, and promote high-quality knowledge transfer through sequential learning stages. Although this study demonstrates the operability of the mechanisms and models through the “Zhi Xin Hui Yu” intelligent coaching system, its application effects still require further verification. Furthermore, education is not only about knowledge transmission but also about shaping the soul. Focusing on the application potential and space of generative artificial intelligence in enhancing learners’ emotions is an inevitable path to promote immersive learning toward deeper levels and higher quality, which will also be a topic for future exploration.
This article was published in the second issue of the Journal of Educational Technology Research in 2025. For reprints, please contact the editorial office of the Journal of Educational Technology Research (official email: [email protected]).
Please cite as follows: Yan Hanbing, Yang Shuting, Yu Shuzhen, Chen Yi. Generative Artificial Intelligence Empowering Immersive Learning: Mechanism, Model, and Application[J]. Journal of Educational Technology Research, 2025, 46(2):64-71.
Editor: Yuan Mengxia
Proofreader: Guo Wanrong
Reviewer: Guo Jiong
[References]
[1] Zhu Zhitian, Dai Ling, Zhao Xiaowei, et al. Cultivation of New Quality Talents: The New Mission of Education in the Era of Digital Intelligence[J]. Journal of Educational Technology Research, 2024, 45(1): 52-60.
[2] SOLANES J E, MONTAVA-JORD S, GOLF-LAVILLE E, et al. Enhancing STEM education through interactive metaverses: a case study and methodological framework[J]. Applied sciences, 2023, 13(19): 10785.
[3] VATS S, JOSHI R. The impact of virtual reality in education: a comprehensive research study [C]// Transfer, Diffusion and Adoption of Next-Generation Digital Technologies. TDIT 2023. IFIP Advances in Information and Communication Technology. Cham: Springer, 2023: 126-136.
[4] Gong Xin, Xu Jie, Qiao Ailing. An Assessment of the Hidden Nature Based on Immersive Learning Environments: Mechanism, Framework, and Application[J]. Journal of Educational Technology Research, 2023, 44(12): 64-72.
[5] RADIANTI J, MAJCHRZAK T A, FROMM J, et al. A systematic review of immersive virtual reality applications for higher education: design elements, lessons learned, and research agenda[J]. Computers & education, 2020, 147: 103778.
[6] MEYER O A, OMDAHL M K, MAKRANSKY G. Investigating the effect of pre-training when learning through immersive virtual reality and video: a media and methods experiment[J]. Computers & education, 2019, 140: 103603.
[7] Liu Ruixue, Wan Kun, Wang Mei. Design and Empirical Study of Immersive Learning Environments Aimed at Spatial Reasoning Skills Development[J]. China Educational Technology, 2021(12): 40-47.
[8] Csikszentmihalyi, M., & Csikszentmihalyi, I. (2010). The Design of Experience. New York, NY: Harper & Row.
[9] Xu Ruyi, Chen Weidong, Zheng Sisi, et al. Embodied Integration: The Connotation Construction, Realization Mechanism, and Educational Application of Immersive Experience – Discussing the New Field of AI + Immersive Learning[J]. Journal of Distance Education, 2021, 39(1): 28-40.
[10] Qin Qin, Shao Biliu. Immersive Reading in Integrated Communication: Concept, Value, and Path[J]. Journal of Nanchang University (Humanities and Social Sciences Edition), 2021, 52(1): 92-98.
[11] Chen Kaiquan, Wu Zhichao, Liu Hong, et al. Technical Paths and Application Models of Extended Reality (XR) Supporting Immersive Learning – Analysis of the International Conference on Immersive Learning Research Network (iLRN 2020)[J]. Journal of Distance Education, 2020, 38(5): 3-13.
[12] DE FREITAS S, NEUMANN T. The use of ‘exploratory learning’ for supporting immersive learning in virtual environments[J]. Computers & education, 2009, 52(2): 343-352.
[13] Wang Zi, Yang Dong, Zhou Jun, et al. Analyzing Learner Interaction Behavior Based on Data Mining Technology in Desktop VR Education Applications – A Case Study of the Teaching of “Electricity and Magnetism” in Junior High School[J]. Modern Educational Technology, 2020, 30(12): 98-104.
[14] SORRENTINO R M, WALKER A M, HODSON G, et al. Trends and prospects in motivation research [M]. Hingham, MA: Kluwer Academic Publishers, 2001.
[15] Lu Yu, Yu Jinglei, Chen Penghe, et al. The Educational Applications and Prospects of Generative Artificial Intelligence – A Case Study of ChatGPT System[J]. China Distance Education, 2023(4): 24-31, 51.
[16] Wu Di, Li Huan, Chen Xu. Analyzing the Impact of the General Large Model of Artificial Intelligence on Education[J]. Open Educational Research, 2023, 29(2): 19-25, 45.
[17] WU X, WANG H H, ZHANG Y T, et al. A tutorial-generating method for autonomous online learning[J]. IEEE Transactions on Learning Technologies, 2024, 17: 1558-1567.
[18] Learning Clues. Create AI-powered virtual teaching assistants[EB/OL]. [2023-04-22]. https://learningclues.com/.
[19] KHAN S. Harnessing GPT-4 so that all students benefit. A nonprofit approach for equal access [EB/OL]. (2023-03-13) [2024-05-15]. https:/blog.khanacademy.org/harnessing-ai-so-that-all-students-benefit-a-nonprofit-approach-for-equal-access/.
[20] Yang Zongkai, Wang Jun, Wu Di, et al. Exploring the Impact of ChatGPT/Generative Artificial Intelligence on Education and Response Strategies[J]. Journal of East China Normal University (Educational Science Edition), 2023, 41(7): 26-35.
[21] Qi Yuanyi, Wang Lamei. The Opportunities, Challenges, and Application Scenarios of Generative Artificial Intelligence in Open Education[J]. Adult Education, 2024, 44(6): 56-61.
[22] Sun Lihui, Zhou Liang. The Practical Logic of Integrating Generative Artificial Intelligence into the National Smart Education Platform for Primary and Secondary Schools[J]. China Educational Technology, 2024(8): 71-79.
[23] Socratic PlayGround For Learning. Socratic playground for learning[EB/OL]. (2024-05-15). https://chat2023.skoonline.org/wizard/index.html.
[24] Dai Ling, Zhao Xiaowei, Zhu Zhitian. Intelligent Inquiry Learning: A New Model of Dialogical Learning Based on ChatGPT[J]. Open Educational Research, 2023, 29(6): 42-51, 111.
[25] SHI S J, LI J W, ZHANG R. A study on the impact of generative artificial intelligence supported situational interactive teaching on students’ ‘flow’ experience and learning effectiveness—a case study of legal education in China[J]. Asia Pacific Journal of Education, 2024, 44(1): 112-138.
[26] BREEN B. Simulating Ancient Ur with ChatGPT[EB/OL]. (2023-10-18)[2024-04-22]. https://resobscura.substack.com/p/simulating-ur-with-chatgpt.
[27] Wei Fei, Zhu Zhitian. Strategies for Teacher Information Technology Capability Building Aimed at Educational Digital Transformation[J]. Chinese Journal of Education, 2022(9): 13-20.
[28] Liu Sannuyaya, Hao Xiaohan. Challenges and Approaches of Generative Artificial Intelligence in Promoting Educational Innovation[J]. Tsinghua University Education Research, 2024, 45(3): 1-12.
[29] NORI H, LEE Y T, ZHANG S, et al. Can generalist foundation models outcompete special-purpose tuning? Case study in medicine [EB/OL]. (2023-11-28)[2024-09-19]. https://arxiv.org/abs/2311.16452.
[30] Wu Yonghe, Jiang Yuanhao, Chen Yuanyuan, et al. Multi-Agent Supported by Large Language Models: Technical Paths, Educational Applications, and Future Prospects[J]. Open Educational Research, 2024, 30(5): 63-75.
[31] Chen Changfeng. The Rise of Intelligent Platforms and the Emergence of Intelligent Agents: Large Models Will Transform Society and Civilization[J]. Journalism, 2024(2): 15-24, 48.
[32] RICHARDS D, TAYLOR M. A comparison of learning gains when using a 2D simulation tool versus a 3D virtual world: an experiment to find the right representation involving the marginal value theorem[J]. Computers & education, 2015, 86: 157-171.
[33] Bransford, J. D., Brown, A. L., & Cocking, R. R. (2013). How People Learn: Brain, Mind, Experience, and School. Cheng Kela, et al. Translated. Shanghai: East China Normal University Press.
[34] East China Normal University. East China Normal University Establishes Family Education Research Institute, Intelligent Coaching System Released[EB/OL]. (2024-05-16)[2024-09-19]. https://www.ecnu.edu.cn/info/1426/66381.htm.
Generative Artificial Intelligence Empowering Immersive Learning:
Mechanism, Model and Application
YAN Hanbing1, YANG Shuting2, YU Shuzhen2, CHEN Yi2
(1.School of Open learning and Education, East China Normal University, Shanghai 200062;
2.Department of Education Information Technology, East China Normal University, Shanghai 200062)
[Abstract] Immersive learning environments have created a high-quality learning space for cultivating new quality talents, but their applications in the education field remains limited due to the factors such as high technological costs, lack of educational theories, and low applicability of learning. Generative artificial intelligence demonstrates significant potential in creative generation, logical expression, and complex interaction understanding.This paper, based on the review of the connotation and components of immersive learning environments, constructs a vertical application mechanism model of generative artificial intelligence in immersive learning environments.Through scenario decomposition, model refinement and content creation, this model achieves the functions such as learning path planning, multimodal resource generation, learning context creation, personalized interaction, and learner profiling. Subsequently, from the practical perspective, this paper proposes an effective learning model based on immersive learning environment, which includes five stages: anchoring objectives, understanding the current situation, course learning, scenario practice, and dynamic monitoring. Finally, the operability of the vertical application mechanism and learning model is demonstrated by the “Zhi Xin Hui Yu” intelligent coaching system, aiming to provide a reference for the vertical application of generative artificial intelligence in the field of education.
[Keywords] Generative Artificial Intelligence; Immersive Learning; Vertical Mechanism; Learning Model; Application Case
Funding Project: 2023 National Social Science Fund Key Project in Education “Research on Innovative Teaching Paradigms and Practical Exploration under the Background of Digital Education” (Project No.: ACA230019)
[Author’s Introduction]
YAN Hanbing (1971—), female, from Acheng, Heilongjiang Province. Professor, PhD, mainly engaged in teaching design, teacher training design and management, and educational digital governance research in the context of digital intelligence. E-mail: [email protected].
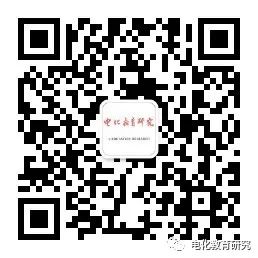
The Journal of Educational Technology Research, founded in 1980, is an academic theoretical field and authoritative publication in the education and educational technology sectors in China, known as the “Research Base for Theoretical Research on Chinese Educational Technology.” It mainly studies cutting-edge issues in modern education, serving national education and teaching reform; focusing on innovations in domestic and international information technology education theories.
The columns established by the Journal of Educational Technology Research mainly include theoretical discussions, online education, learning environments and resources, curriculum and teaching, subject construction and teacher development, educational technology for primary and secondary schools, history, and international comparison.
The Journal of Educational Technology Research