
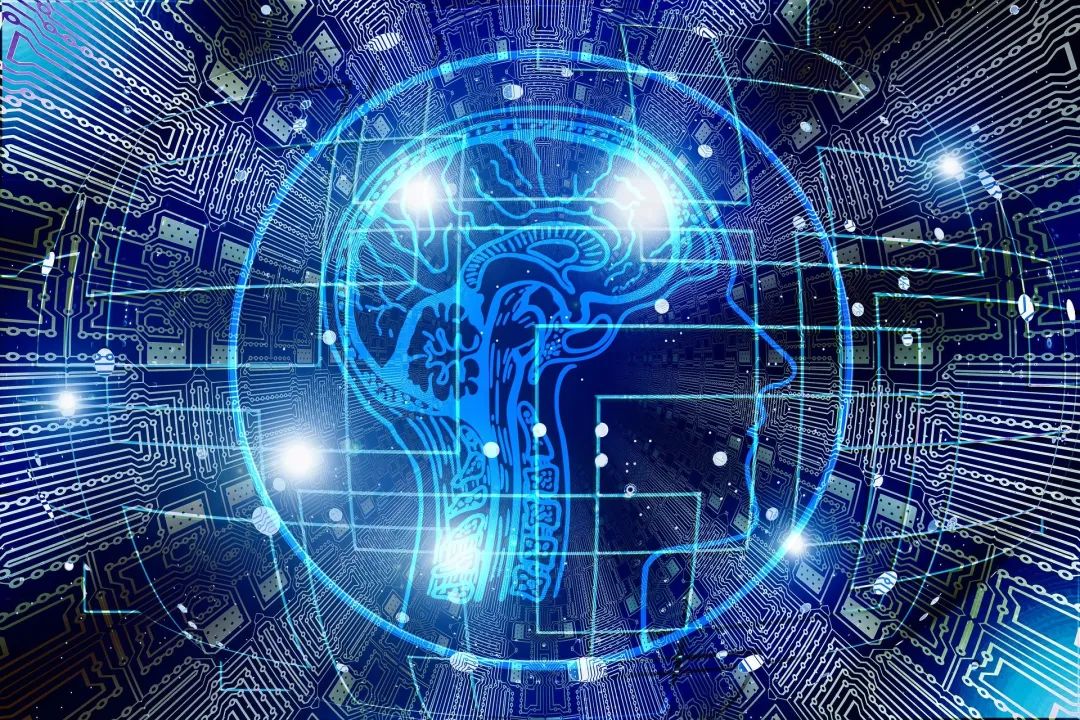

Background of Construction
General Secretary Xi Jinping pointed out, “We should take the new generation of artificial intelligence as a driving force to promote the leapfrog development of science and technology, the optimization and upgrading of industries, and the overall leap in productivity, striving to achieve high-quality development.” “Developing new quality productivity is an inherent requirement and an important focus for promoting high-quality development.” New quality productivity has qualitative characteristics of a new round of technological revolution and industrial transformation, requiring the development of high-tech, high-efficiency, and high-quality new productivity. The 2024 government work report proposed by the State Council emphasized deepening the research and application of big data and artificial intelligence, and carrying out the “Artificial Intelligence +” initiative. To actively implement the deployment of the Party Central Committee and the State Council, the 2024 National Water Resources Work Conference emphasized vigorously promoting the construction of digital twin water resources, pushing for the application of large model algorithms in artificial intelligence, and enhancing the “2+N” intelligent business level.
Advancing the construction of digital twin water resources is a significant mark and important path for promoting high-quality development in water resources at a new stage. Digital twin water resources consist of data baseboards, model platforms, knowledge platforms, business applications, and basic infrastructure for water resource information. With the rapid advancement of digital twin water resources in recent years, a relatively mature system for constructing a digital twin water resource data baseboard and model platform has been established. The data baseboard, as the cornerstone of digital scene construction, covers basic data, real-time monitoring data, business management data, cross-industry shared data, geographical spatial data, and multi-dimensional temporal and spatial scale data models, providing solid support for calculations. The model platform, as the core and key of digital twin water resources, integrates professional water resources models, intelligent models, and digital simulation engines, achieving the assembly and online calculation of mathematical models, providing strong algorithmic support for simulation and precise decision-making in various businesses. However, at the current stage, the construction of the knowledge platform for digital twin water resources mainly focuses on transforming various business rules and experiences into a knowledge base. Under the urgent need for intelligent transformation and upgrading in the water resources sector, how to efficiently utilize these knowledge bases with large models and how to allow knowledge to intelligently drive models for business calculations are current technical challenges that need to be addressed.
Currently, China is in the midst of a new round of technological revolution and industrial transformation, with new generation information technologies represented by artificial intelligence and quantum information aligning closely with the concept of developing new quality productivity. In recent years, large language models (LLMs) have made significant progress in the field of artificial intelligence, with models such as GPT-3/4, ChatGPT, and Claude expanding model scales and significantly improving performance, achieving generalization in task-solving approaches and being applied in industries such as healthcare and education. Currently available large language models possess vast training datasets and massive model parameters, demonstrating complex decision-making capabilities close to those of humans, and are gradually becoming a core means for building autonomous intelligent agents and empowering business applications across many research fields.
In summary, from the perspectives of policy, industry, and technology, there is an urgent need to conduct research and customized construction of large models in the water resources sector to achieve the goal of empowering water resources business with artificial intelligence large model technology, leading the development of new quality productivity in water resources, and promoting high-quality development in the water resources sector.
Current Issues
The development of large models has undergone several stages, including statistical language models, neural network language models, and pre-trained language models. Currently, with the introduction of scalable training architectures and learning paradigms such as the Transformer architecture, as well as significant improvements in data quality and scale, the parameter scale of large models can expand to tens of billions, hundreds of billions, or even trillions, and pre-training tasks can be unified. With the launch of large model applications such as ChatGPT at the end of 2022, large language model technology has rapidly swept across society, marking an important advancement in artificial intelligence technology applications. In addition to large model products launched by companies such as OpenAI and Baidu, in recent years, the continuous iteration of excellent open-source models such as Llama, GLM, Baichuan, and Qwen, as well as the constant introduction of new model architectures like RWKV and Mamba, have significantly advanced the exploration and practice of large models in industries such as water resources.
Whether large language models can effectively land in the industry is primarily measured by their ability to understand the professional language and logic of the industry, their ability to call professional models and related tools, their ability to present industry demands in an easily understandable way, and their ability to drive the use of proprietary equipment and capabilities in the industry. General large language models often encounter semantic illusions and irrelevant answers when facing the highly specialized field of water resources. The reasons and problems behind this can be summarized into four points.
First, there is a lack of pre-training and fine-tuning in the field of water resources, making it difficult for large language models to deeply understand industry-specific terms, phenomena, and physical mechanisms. For example, when asking a large language model, “Provide a real-time scheduling sand discharge plan for the Xiaolangdi Reservoir based on the conditions of the reservoir’s abnormal flow infiltration point,” it cannot understand the professional meanings and physical mechanisms of terms like “reservoir abnormal flow infiltration point,” “real-time scheduling,” and “sand discharge plan,” making it challenging to provide a professional answer.
Second, there is a lack of integration with business knowledge graph inference capabilities, which leads to the inability of large language models to understand the internal logic of decision-making in the water resources sector and lack the reasoning capabilities of business. For instance, when deploying the instruction to a large language model, “Assess the current rainfall situation and provide a reservoir scheduling plan,” it cannot understand the linkage logic of “rainfall – runoff – confluence – evolution,” making it unable to sequentially invoke the corresponding sub-modules for business analysis.
Third, there is a lack of coupling with professional water resources models, which means that even if large language models possess the logical analysis capabilities described in point two, they still struggle to conduct quantitative analysis for precise decision support. For example, asking a large language model, “Estimate the future water level process of the Yongding River’s Lugou Bridge based on current precipitation between Guanting and Shanqu,” it cannot drive hydrological models and hydraulic models in real-time to conduct forecasting and flood evolution calculations, thereby failing to provide accurate results.
Fourth, there is a lack of interaction with proprietary water resources equipment and capabilities. Currently, large language models cannot operate in familiar work scenarios for water resources workers and cannot effectively drive the operation of water resources equipment and facilities.
Concepts and Functions
The hydraulic large model refers to a model centered on large language models, conducting professional pre-training and fine-tuning with water resources knowledge, reinforcing the logic of large models with water resources knowledge graphs, and enabling quantitative calculations through coupling with professional water resources models, thus constructing a large model for the water resources sector that processes data, understands logic, invokes models, and makes intelligent decisions. In simple terms, the hydraulic large model is an intelligent tool and application that assists in solving issues such as how computers understand human language about water resources, how to decompose water resources workflows, how to apply professional water resources data for reasoning calculations, and how to present according to water resources business logic and elements.
The hydraulic large model possesses five industry application capabilities: “understanding water resources language,” “decomposing water resources tasks,” “invoking professional water resources models,” “displaying simulation results,” and “driving facilities and equipment,” which can lower the threshold for complex water resources businesses, reduce the repetitive workload for business personnel, and provide more comprehensive decision-making information and intelligent decision-making suggestions for decision-makers.
Building a hydraulic large model with the above capabilities is of great significance for enhancing the decision support system capabilities of digital twin water resources. By deeply exploring the potential value of data, the hydraulic large model can drive and utilize water resources knowledge graphs, historical scenario patterns, business rules, and expert experiences, and can also combine and invoke professional water resources models. It can provide strong support for the “four predictions” (forecasting, early warning, rehearsal, and plans) for flood prevention and water resource management and allocation, and provide rich decision-making knowledge support for various businesses such as water resources engineering construction, operation management, and river and lake management, thus helping achieve intelligent management and precise decision-making in multiple business areas of the water resources sector. For example, in response to the autumn flood of the Yellow River Basin in 2021, when asked by a computer to “calculate the scheduling process of the Xiaolangdi hydraulic hub to ensure downstream flood safety and the flow process at the Huayuankou station of the Yellow River main stream,” the hydraulic large model can obtain precipitation runoff inputs from the Sanhua area and above Tongguan, drive hydrological forecasting, hydraulic evolution, and joint scheduling models to obtain the outflow process of Xiaolangdi after joint scheduling and the predicted flow process at the Huayuankou hydrological station, and perform dynamic rolling calculations to provide intelligent support for flood prevention decision-making and refined scheduling of reservoirs.
Feasibility
Currently, the Information Center of the Ministry of Water Resources is coordinating large model R&D teams from industry universities, research institutions, and engineering units, relying on multiple scientific research and production projects to carry out key technology research and preliminary application scenario construction for hydraulic large models, providing feasible solutions for the application of hydraulic large models.
Regarding data foundation, by the end of 2023, information on over 16 million water resource objects such as reservoirs, river channels, and flood retention areas has been dynamically updated nationwide in the “One Map” of water resources, and 2.62 billion business management data points have been aggregated. High-resolution resources, environment, and other series of 23 domestic remote sensing satellite image resources have been obtained through dedicated lines, and over 4600 water resources video resources have been accessed, significantly enhancing new perception capabilities. Flood forecasting plans for 323 important sections of 65 major rivers nationwide, the correlation between river and lake water systems and water resources engineering, water allocation plans for 70 important inter-provincial rivers, and ecological flow control rules for 144 sections of 83 important rivers and lakes have been established, alongside a database of over 140 historical flood data, preliminarily providing the training data and standardized application scenarios needed to construct hydraulic large models. The 94 pilot tasks for building digital twin water resources have provided a data and knowledge base for the construction of hydraulic large models and have tentatively explored relevant business models.
Model linkage aspects, the Ministry of Water Resources is actively organizing the development of 21 water resources professional models in six categories, including basin runoff, soil erosion, groundwater, sediment, and water resource allocation, which have been applied in long-term operations for disaster prevention and water resource management and allocation, accumulating model development experience. The Information Center of the Ministry of Water Resources is leading the promotion of the construction of a digital twin water resources model platform, providing standardized interface tools and a unified platform for the input, output, integration deployment, and data transmission of professional water resources models. The development of professional water resources models and the water resources model platform can compensate for the shortcomings of hydraulic large models in the simulation solving algorithms of professional water resources.
Regarding technical architecture, currently, the domestic IT industry has fully initiated large model R&D applications. In the era of pre-trained language models, the natural language processing field has widely adopted the pre-training + fine-tuning paradigm, forming large-scale pre-trained language models represented by encoder-only architectures like BERT, decoder-only architectures like GPT, and encoder-decoder architectures like T5. Relevant products have been preliminarily applied in industries such as transportation, healthcare, and education, with mature foundational technology for large models.
In terms of talent reserves, with the comprehensive promotion of digital twin water resources construction, various levels of water resources units have accumulated a certain number of technical talents through pilot work, and water resources research institutions and enterprises have also advanced talent team building. These talents possess knowledge of computer science and experience in water resources business applications, providing talent support for the construction of hydraulic large models and the application of business scenarios.

Construction Ideas
Following the requirements of “demand-driven, application-oriented, digital empowerment, and capability enhancement,” based on the digital twin platform of the Ministry of Water Resources, we will conduct the selection, domain training, and fine-tuning of large models suitable for the industry, integrating large models with traditional knowledge in the water resources sector to form an understanding capability of “understanding water resources language”; building a water resources knowledge base and enhancing knowledge graph capabilities for domain large model construction, providing decision support and reasoning constraints through knowledge graph technology to form an analytical capability of “decomposing water resources tasks”; conducting model platform combinations driven by large models and external tool calculations to achieve a computational capability of “invoking professional water resources models”; developing multimodal large model integration based on the national water resources “One Map” to form a display capability of “showing simulation results”; and coupling the hydraulic large model with water-related multi-terminal and chip research and development to create an end capability of “driving facilities and equipment.” Constructing a hydraulic large model platform that is advanced, practical, integrated, open, reliable, and secure, and applying it in “2+N” businesses, gradually forming a co-construction and sharing system for large models in the water resources sector.
▲Construction Ideas for Hydraulic Large Models
Key Technologies that Need In-Depth Research
Building hydraulic large models is not simply about customizing training of large language model algorithms using water resources industry datasets. It is essential to construct a flexible, autonomous, and scalable inference and operation engine for hydraulic large models, forming a training paradigm suitable for the water resources industry, and researching technical challenges like “knowledge graph-based optimization and inference functions for hydraulic large models” and “integration of large models with real-time monitoring and perception technologies in the water resources sector.”
Building a Flexible, Autonomous, and Scalable Hydraulic Large Model Engine
Targeting the goal of business reasoning and decision-making driven by water resources knowledge, research a hydraulic large model inference engine with human-machine interaction, reasoning analysis, and scheduling execution capabilities, allowing the large model to support multiple interaction methods, intent recognition, and tool invocation. Research efficient engine and access interface technologies for data conversion and fusion, achieving real-time interaction between hydraulic large models and data baseboards, model platforms, and supporting multi-level user collaboration.
Research on Industry Domain Training Paradigms for Hydraulic Large Models Based on Pre-training and Fine-tuning
Focusing on the application needs of water resources businesses, gather and organize a massive amount of multi-source water resources industry data, classify the data, pre-train the large model, and fine-tune the model based on typical water resources business application scenario processes, using full updates or PEFT (Parameter Efficient Fine-Tuning) methods like LoRA to enhance the model’s understanding and task execution capabilities in the water resources field, forming a training paradigm for fine-tuning large models in the water resources domain, improving the rationality and interpretability of large model inference.
Research on Knowledge Graph-Enhanced Construction and Knowledge Emergence of Hydraulic Large Models
Through research on knowledge graph technology that assists large models in recognition and analysis, enable large models to achieve automated workflow recognition and multi-business data correlation based on semantic understanding, endowing large model algorithms with reasoning capabilities in water resources business processes that they do not possess. Research methods for constructing multi-business integrated knowledge graph ontologies, digitally describing water resources objects and elements in the basin environment from multiple temporal and spatial scales, forming a digital flow of water resources business logic. Apply knowledge mining techniques assisted by large models to establish correlations between objects, models, and data across multiple businesses, completing the construction of a knowledge base based on large models.
Research on Optimization Technology for Hydraulic Professional Model Calculations Driven by Hydraulic Large Models
Research intelligent optimization technologies for model parameters based on remote sensing images and expert experience knowledge, achieving adaptive dynamic optimization of model parameters, enabling hydraulic large models to autonomously drive professional calculations in water resources and iteratively improve knowledge assets, constructing the ability of large model knowledge engines to enhance forecasting workflows. By studying parameter optimization technologies that hold both data and knowledge, achieve intelligent parameter optimization for professional mathematical models in hydrology and engineering scheduling. Develop multi-scale similarity matching algorithms based on situational awareness to match the similarity of basin scenarios based on historical scheduling processes and preset simulation processes, supporting integrated rapid simulation of forecasting and scheduling. Research reinforcement learning decision models guided by scheduling rules and the intervention of schedulers’ experiences, achieving intelligent decision support based on large model interactions and constraints from water resources knowledge, enhancing the efficiency of intelligent algorithm optimization.
Research on Collaborative Monitoring and Perception Technologies Driven by Hydraulic Large Models
In addition to driving calculations for digital twin water resources software, hydraulic large models can also empower the enhancement of water resources’ “sky-ground” monitoring and perception capabilities. Research on the collaborative integration technologies of hydraulic large models with new technologies and equipment such as water resources remote sensing satellites, lidar, rain radar, rain gauges, and hydrological stations, achieving intelligent operation of devices driven by hydraulic large models, intelligent aggregation and processing of monitoring data, and intelligent recognition of monitoring errors, accelerating the enhancement of traditional monitoring and perception methods through artificial intelligence technology, improving all-weather and all-time monitoring and intelligent service capabilities.
Main Architecture
To construct a hydraulic large model with independent intellectual property rights, convenient customization, and broad business applicability, and gradually establish a co-construction and sharing system for large models in the water resources sector, a water resources large model platform architecture based on the business needs of the platform has been designed under the framework of digital twin water resources. It fully draws on physical water resources knowledge and interacts intelligently with digital twin basins, water networks, and engineering constructions, empowering businesses such as flood prevention and water resource management and allocation, while enhancing network security levels through the capabilities of large models. The platform architecture encompasses components, services, and integration methods at all levels, describing from top to bottom the planning of components such as user layer, basic application layer, access interaction layer, native services, inference engine, resource operation platform, system management center, and their relationships with external systems and facilities, as shown in the figure below.
Among them, the resource operation platform fully interacts with the digital twin water resources data baseboard and model platform, primarily responsible for the registration, configuration management, and monitoring evaluation of water resources data, (large) models, water resources knowledge, and skills, providing callable units for business processes driven by water resources knowledge. The inference engine mainly includes services for human-machine interaction, logical reasoning, and scheduling execution, handing the demands and related data and knowledge posed by users over to the large model for inference, and then invoking the functions of the digital twin platform or application systems in sequence according to business logic. The system management center is mainly responsible for user, service, operation, and standard management and can interface with existing systems.
The overall platform revolves around demand-side users (decision leaders, frontline business personnel, application system developers, etc.), supply-side users (data, model, knowledge, and tool providers, etc.), and management-side users (industry management, platform management, operation management, etc.), utilizing the resource operation platform and inference engine to offer multi-turn dialogue, knowledge processing, knowledge utilization, intent recognition, tool utilization, and gradually expanding capabilities such as personalized following, continuous learning, multimodal, logical optimization, and tool manufacturing. It supports access to various systems through web (Web), mobile applications (App), co-pilot assistants, mini-programs, and service interfaces (API), supporting applications such as knowledge secretaries, national water resources “One Map” assistants, flood scheduling scheme auxiliary generation, intelligent analysis and early warning for river and lake remote sensing, and intelligent customer service.

Construction Model of Large Models by the Ministry of Water Resources
Through scientific research and preliminary tackling of infrastructure, the Information Center of the Ministry of Water Resources has conducted the construction of large models, establishing a flexible, autonomous, and scalable inference and operation engine framework for hydraulic large models, designing industry domain training based on pre-training and fine-tuning, as well as online updating modes for knowledge parameters, exploring applications in document office, image recognition, professional calculations, and intelligent decision-making. Aiming at different levels of management entities in the water resources industry, the Ministry of Water Resources intends to achieve unified standards, interface specifications, and self-construction of basins, provinces, and projects according to norms through the construction method of “1+7+X,” fully co-constructing and sharing the large models of the Ministry of Water Resources. In supporting the construction of digital twin water resources, it realizes the dynamic updating application of information from the digital twin water resources data baseboard, drives the computational operation of the model platform with hydraulic large models, and gradually empowers flood and drought disaster prevention, water resource management and allocation, river and lake management, and other water resources business applications, forming a typical paradigm of water resources knowledge-driven business applications, enhancing work efficiency and improving the precision of decision-making and the intelligence level of management in the water resources sector. The construction model of hydraulic large models is shown in the figure below.
Document Office Applications
Document office applications primarily address user needs for intelligent, automated, and customized preparation of office materials, integrating functions such as information retrieval, document writing assistance, and summary extraction. They possess the capability to generate meeting plans, draft data table documents, and summarize complex materials, improving efficiency in document preparation and meetings while reducing foundational repetitive operations.
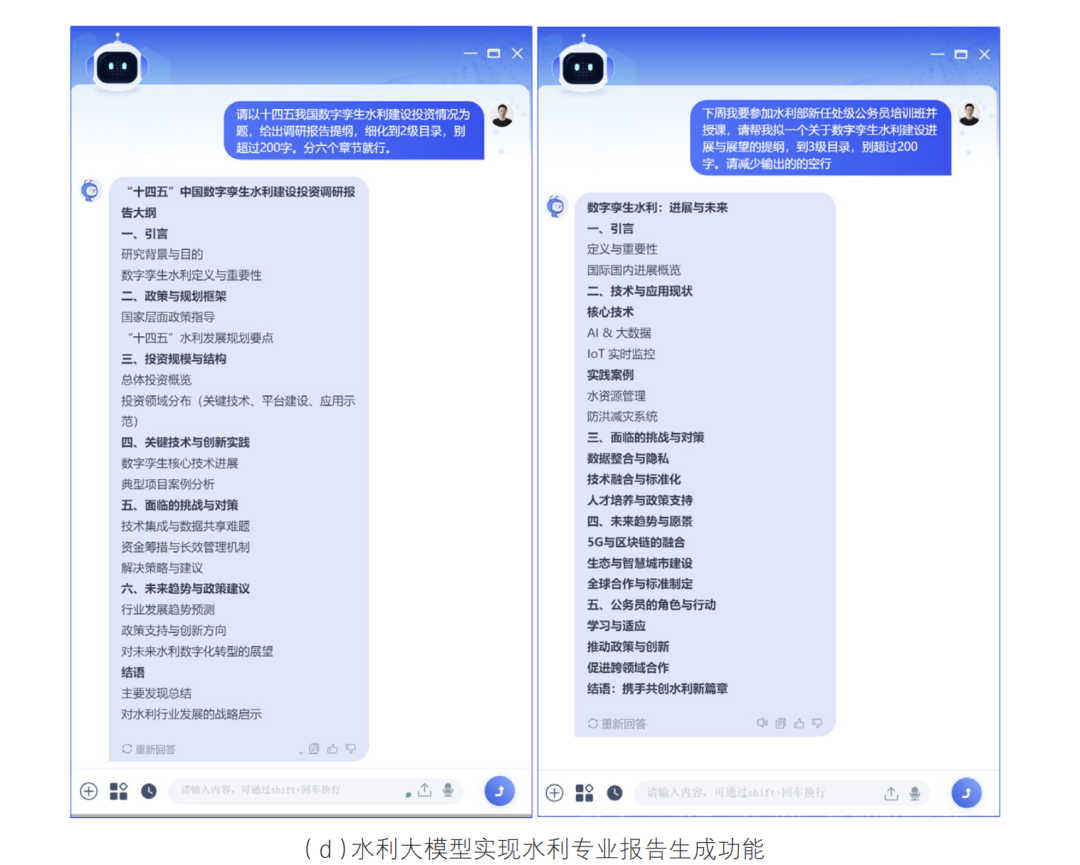
The large model will first generate the framework structure of documents or other materials based on the user’s demands and specified templates (divided into multi-level directories according to word count or user needs), subsequently filling in content based on segmented themes, referencing public knowledge bases such as water resources knowledge, technical standards, and policy regulations, as well as private knowledge bases of users (e.g., retrieval-augmented generation techniques). If real-time data references are required, it will utilize existing data and model query interfaces for summarization. Users can collaborate with the large model to modify and refine materials based on the results generated by the large model through dialogue. Compared to general document version office scenarios, the hydraulic large model platform supports accessing real-time water resources business data from systems such as the national water resources “One Map” for analysis.
Based on the hydraulic large model platform, users only need to state their document needs in the dialogue box and provide a small amount of private materials (such as document templates or materials organized and collected by the user), and the large model can guide users step-by-step to complete the construction of relevant documents, supporting online viewing or downloading of documents.
Image Recognition Applications
To ensure precision and efficiency in water resources management, image recognition applications are constructed based on the national water resources “One Map,” integrating hydraulic large models with digital twin technology to provide customized services for users. Users can set themes based on their needs, and the system dynamically generates thematic charts covering key hydrological and spatial information such as precipitation distribution, reservoir storage, and river water levels. Specific application scenarios include: using high-resolution remote sensing images, the large model calls remote sensing intelligent recognition models to identify buildings within river management areas; automatically retrieving lists of newly added buildings through the remote sensing platform’s foundational database; automatically generating analysis reports on suspected illegal constructions obstructing floods in river channels, providing optimal route plans for on-site inspections, etc.
Image recognition applications achieve deep integration and intelligent processing of data through advanced data processing technologies and user interface designs. Utilizing an algorithm-driven service selection mechanism, image recognition applications can accurately filter and display relevant water resources services while reducing operational complexity and enhancing user interaction experience. The application of hydraulic large models can significantly improve the efficiency of interactions between business personnel and water resources systems, allowing users to interact with the national water resources “One Map” through clicks or Q&A, such as querying watershed ranges and detailed information on water resources facilities, with query results presented in real-time through charts, text, and base map formats on the front end.
Professional Calculations and Intelligent Decision-Making Applications
Water resources professional mathematical models and calculations are the core and key of digital twin water resources, but professional models such as hydrological forecasting, hydrodynamics, and multi-objective optimization scheduling for water resources engineering require high technical expertise from business personnel. When facing complex decision-making situations, general business personnel often struggle to make scientifically sound professional calculations in water resources, making it difficult to formulate precise decision-making plans. For instance, in the flood prevention “four predictions” workflow, a team composed of multiple business departments and personnel needs to roll through discussions for hours or even days to formulate reasonable flood prevention plans. The application of hydraulic large models can effectively enhance management personnel’s ability to analyze and process massive amounts of water resources business data, and through large model-driven digital twin technology, it can quickly and cost-effectively simulate and optimize various complex decisions, significantly improving decision-making efficiency.
Based on large model-driven multi-source water data knowledge mining technologies, professional calculations and intelligent decision-making applications can achieve the capability of interfacing water resources objects and professional models across multiple business domains. Research methods for constructing multi-business integrated knowledge graph ontologies, allowing large models to interface with business rules, disciplinary knowledge, expert experience, historical scenarios, and other contents of water resources knowledge bases, as well as human natural language understanding capabilities, enabling business application scenarios to drive hydraulic large models to conduct professional calculations and intelligent decision-making in water resources. Through large model-driven professional calculations in water resources, it can simulate the physical processes and expected impacts of different scheduling schemes, achieving intelligent decision support based on large model interactions and constraints from water resources knowledge, thereby enhancing the efficiency, scientificity, and intelligence level of water resources business decision-making.
Hydraulic large models can not only recommend effective forecasting and scheduling schemes based on user-provided information but also drive the digital twin water resources model platform to simulate various extreme scenarios and assess potential risks. It can achieve model engine calls to AI models, enabling minute-level predictions of meteorological typhoon paths and intensities, minute-level simulations of basin rainfall, second-level forecasts of river water level rises, minute-level rapid forecasts of flooding, and minute-level rapid recommendations for scheduling schemes, providing support for the “four predictions” of flood prevention. Taking the example of the “23.7” extraordinary flood in the Haihe River Basin, flood prevention personnel can set real-time simulation scenarios through the large model, driving hydrological forecasting models to conduct rolling forecasts based on real-time precipitation runoff data and model parameter information, obtaining inflow forecasting plans for reservoirs such as Guanting and Zhaitang, and predicting the runoff process of the Yongding River main stream; based on the predicted water conditions, the hydraulic large model drives the reservoir joint scheduling model to intelligently recommend optimized scheduling plans for reservoirs such as Guanting and Zhaitang, as well as the Lugou Bridge hydraulic hub, ensuring rational use of the reservoir group while reducing pressure on the Lugou Bridge hydraulic hub. Subsequently, the hydraulic large model comprehensively considers the forecasts and scheduling plans, driving two-dimensional hydrodynamic models to calculate the flood evolution situation below the Lugou Bridge cross-section, verifying the inundation situation produced by the scheduling plans, assessing the rationality of the forecasts and scheduling plans, and ensuring that the provided plans are scientifically valid and effective in practical applications. Through the full application of hydraulic large models, support can be provided for flood prevention decision-making and precise utilization of flood retention areas in various business scenarios. Other cases of professional calculations and intelligent decision-making applications on the hydraulic large model platform are shown in the figure below.
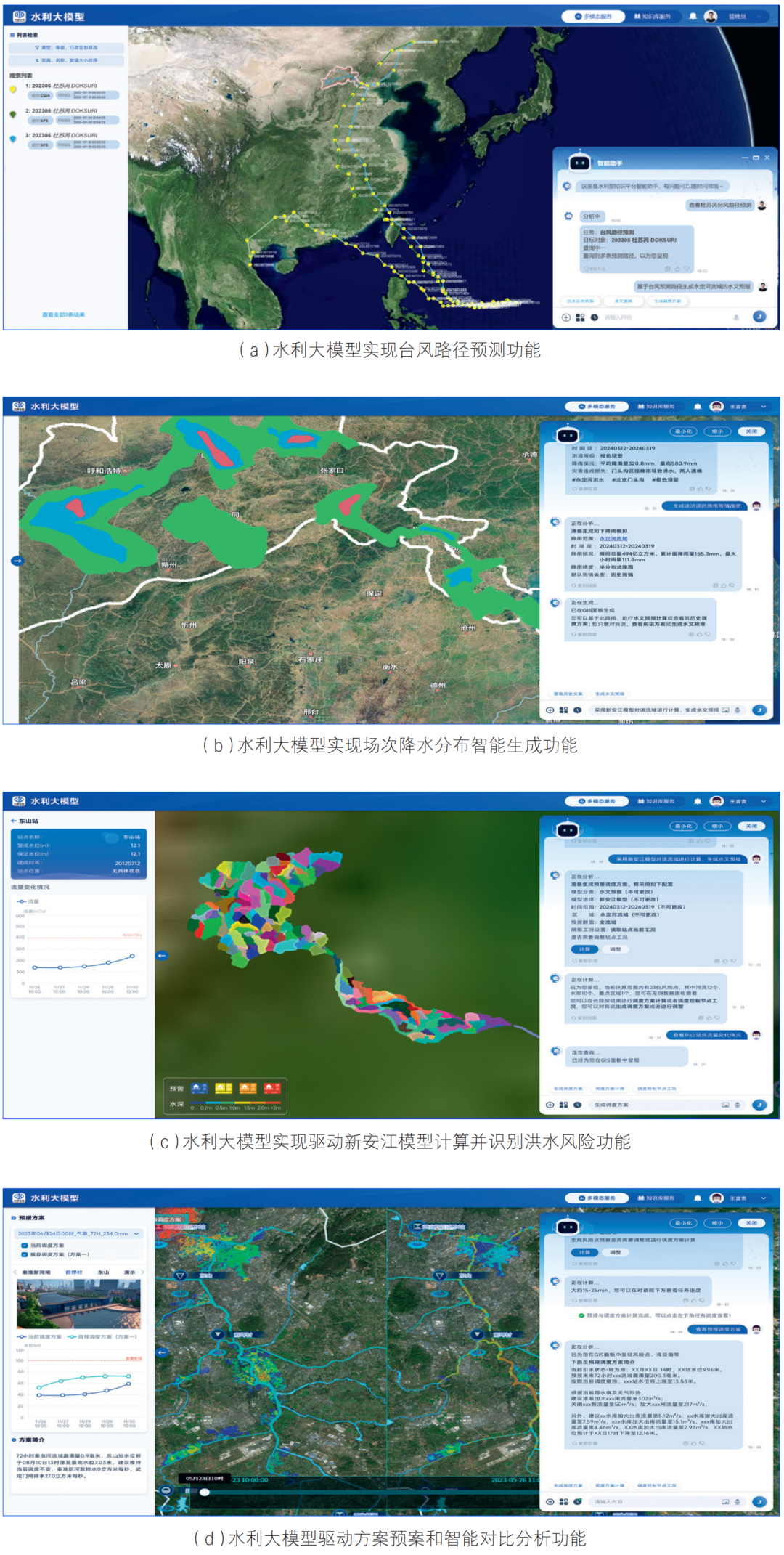

This article outlines the background of constructing hydraulic large models, proposes the concept of hydraulic large models, deeply analyzes the importance and necessity of constructing hydraulic large models, systematically introduces the construction ideas and architecture, proposes key technologies that need breakthroughs, and elaborates on the exploration of the Ministry of Water Resources in constructing large models and practical application scenarios in the industry. It introduces the hydraulic large model built on the basis of the national water resources “One Map,” digital twin water resources data baseboard, and model platform, which possesses the capabilities of “understanding water resources language,” “decomposing water resources tasks,” “invoking professional water resources models,” “showing simulation results,” and “driving facilities and equipment.” In the future, it will comprehensively promote hydraulic large models to different levels of management entities in the water resources sector, gradually forming a co-construction, sharing, and common use system for large models in the water resources field through the construction model of “1+7+X.” The hydraulic large model plays an important role and significance in improving business efficiency, reducing operational complexity, promoting scientific decision-making, popularizing water resources business knowledge, and advancing digital twin water resources development. It is recommended to enhance the construction of the standard system for hydraulic large models in the water resources technical standard system and strengthen the trial use and promotion of large models by the Ministry of Water Resources. Through the construction of hydraulic large models, fully leverage the leading role of technological innovation to drive the transformation and upgrading of the water resources industry, promote the development of new quality productivity in water resources, and provide an important engine for leading high-quality development in digital twin water resources construction.
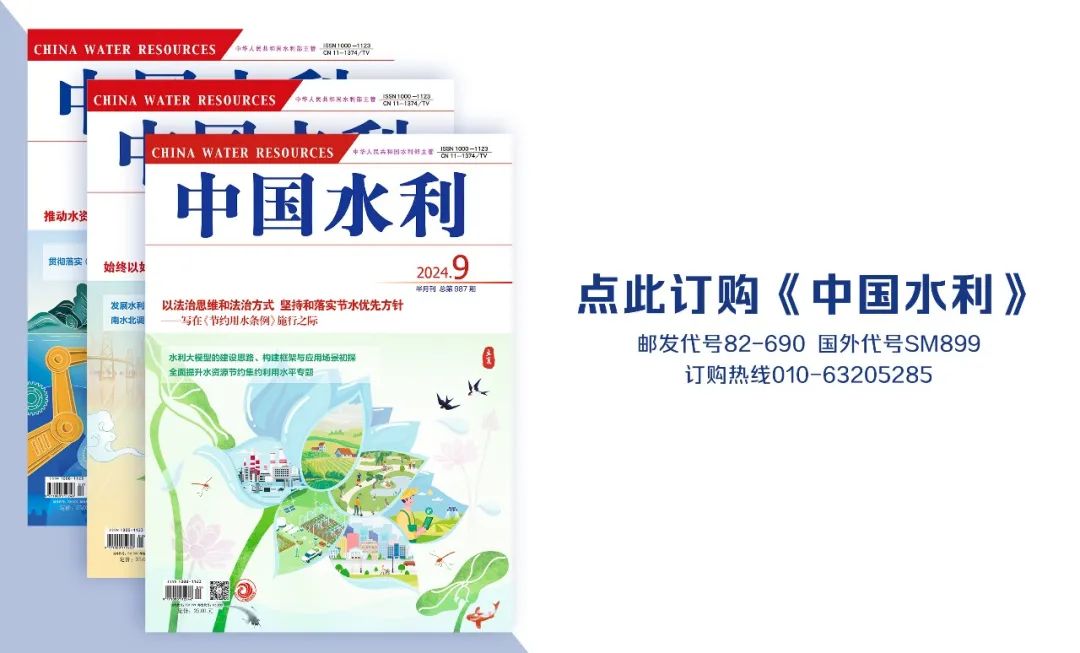
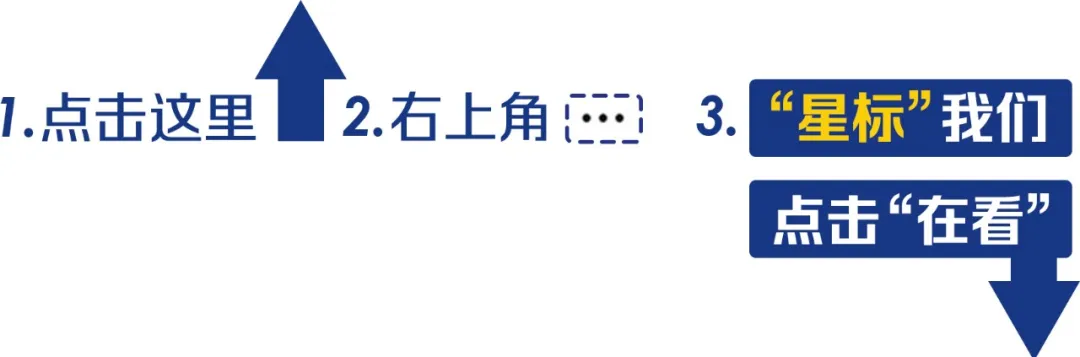