In 2022, OpenAI, a high-tech company far away in Silicon Valley, released an intelligent chat program called ChatGPT, marking the beginning of the generative AI revolution. A year later, this world-renowned company faced a “mutiny,” while on the other side of the globe, China’s technological pioneers are actively exploring the application of generative artificial intelligence across various industries. The financial industry, which has “high technology” written in its genes, is gradually attempting to integrate AIGC into its organizations; the future is here, and the outline of AIBank is becoming clearer.
Why Can’t the Financial Industry Directly Use Basic Large Models?
After the release of ChatGPT, Chinese technology companies rushed to join the wave of research on basic large models. Within a year, companies like Baidu, Alibaba, Tencent, and Huawei launched related products, with product capabilities becoming increasingly refined. Generative artificial intelligence has significantly improved efficiency; however, can the financial industry directly use basic large model applications? The answer is clearly no.
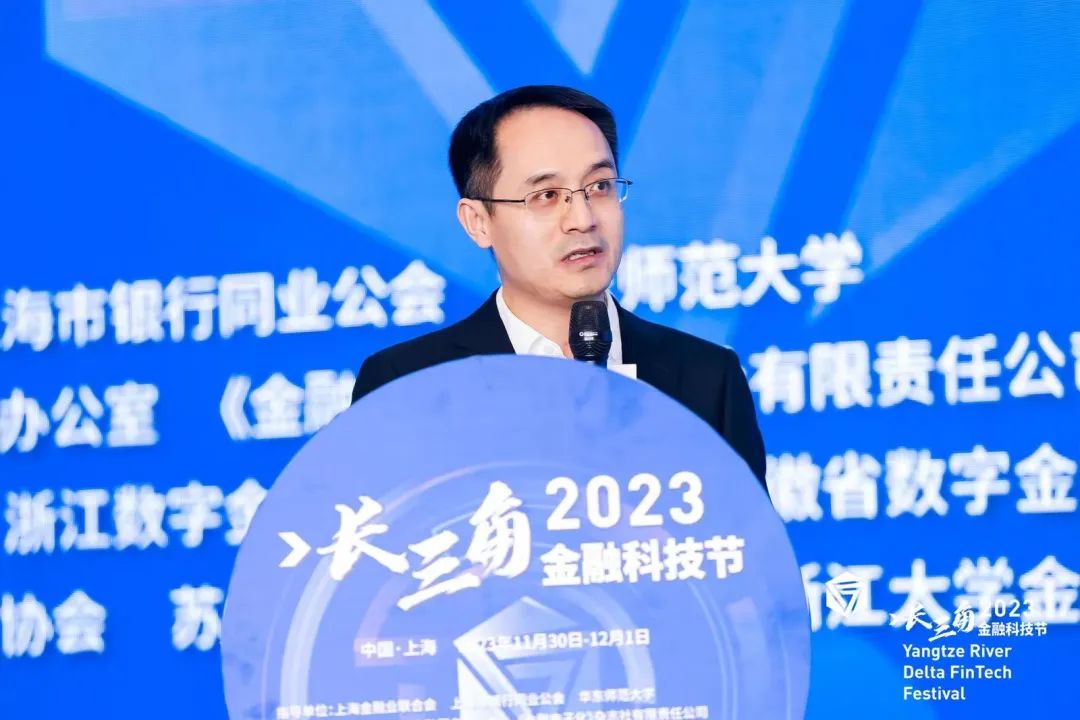
Deputy Director of Shenzhou Information New Dynamics Research Institute, Xue Chunyu
On one hand, “Without data security, there is no economic security.” The financial industry must first consider data security issues when applying large models. For security reasons, no bank can afford the risks associated with inputting financial data into public large models for training; on the other hand, each participant in the financial industry has its own uniqueness and specificity, which determines that universal basic large models cannot be practically used in the financial industry.
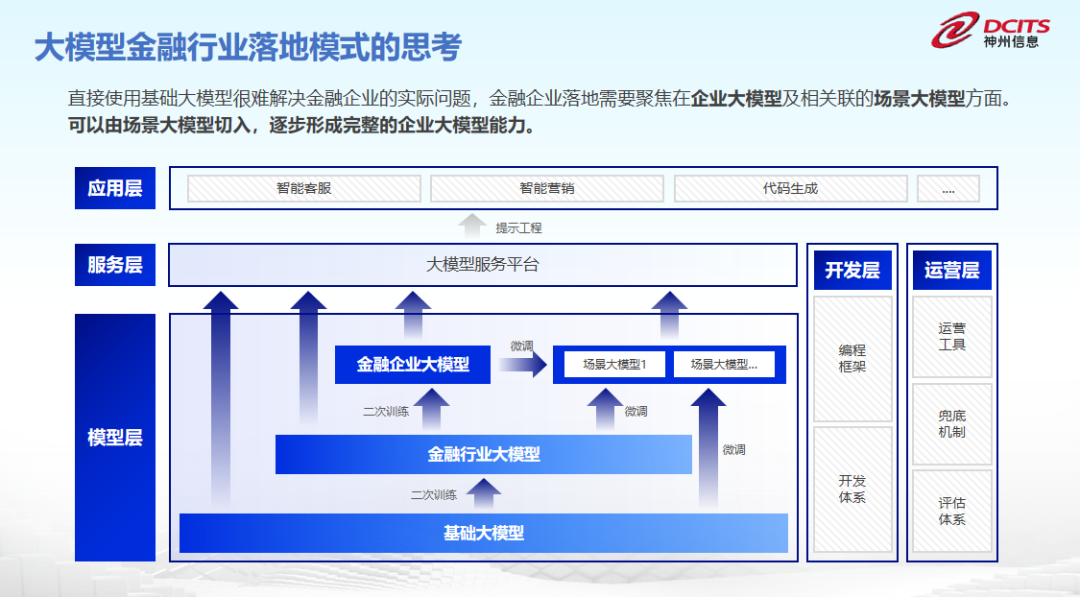
According to the current situation in the financial industry, large models can be divided into four structural layers: basic large models, financial industry large models, financial enterprise large models, and scenario large models. Since directly using basic large models cannot effectively solve the practical problems faced by financial institutions, the financial industry needs to focus on the scenario large models related to enterprise large models. Therefore, we can start from scenario large models and gradually form a complete large model capability for financial enterprises.
Starting with Scenario Large Models: A Five-Step Strategy for Financial Large Models
Without accumulating small steps, one cannot reach a thousand miles. By focusing on a specific practical financial scenario as the entry point for scenario large models, if it can effectively solve the problems faced by financial enterprises in their operations, it will gradually accumulate and solidify into an internal large model for financial enterprises.
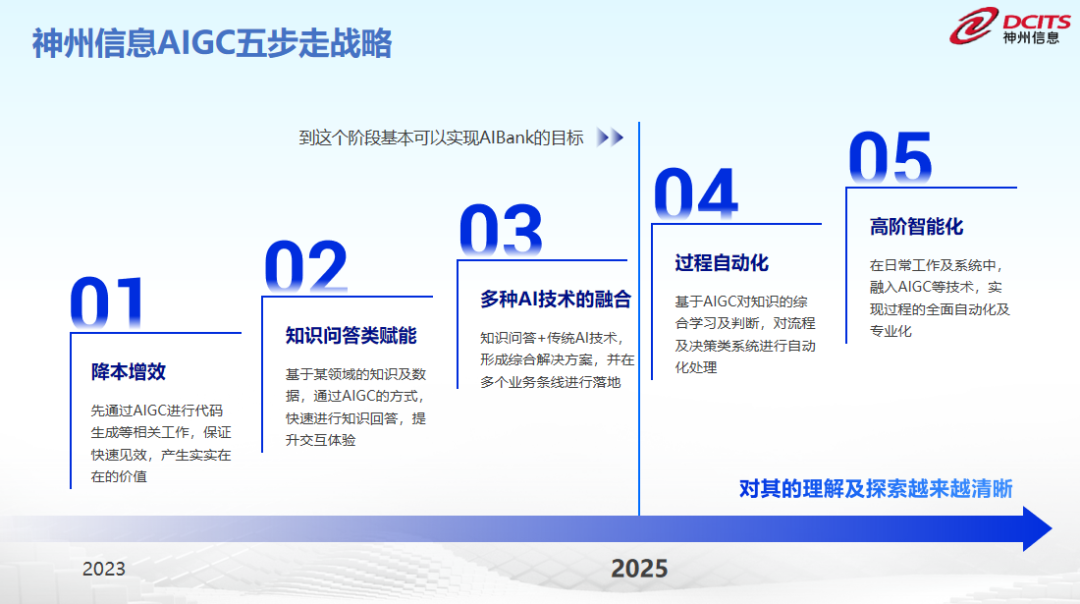
To this end, Shenzhou Information has formulated a five-step strategy for AIGC, objectively breaking down the large model for financial enterprises into five stages: cost reduction and efficiency enhancement – knowledge Q&A empowerment – integration of various AI technologies – process automation – advanced intelligence.
First, as the starting point of this five-step strategy, the initial focus is on reducing costs and enhancing efficiency within financial institutions: empowering code generation work through AIGC to lower system construction costs;
Second, in the area of knowledge Q&A, financial institutions can first complete the internal knowledge Q&A capability reserve and gradually expand to related business scenarios, assisting employees/cashiers in handling complex business scenarios, improving interaction experience and work efficiency;
Third, products like intelligent investment advisors and digital humans not only require AIGC capabilities but also need the cross-integration of many specialized knowledge areas. It is necessary to integrate AIGC with traditional AI algorithms or knowledge graphs. Currently, this capability still needs further development; once completed, the goal of AIBank can be achieved;
Fourth, based on AIGC’s understanding of various knowledge, achieve complete process automation—customized for each individual;
Fifth, at the highest stage of development, further implement AIGC and other technologies in daily work systems to achieve comprehensive process automation and specialization.
Scenario Large Models Move Towards Practice, Further Enhancing Development Efficiency
As is well known, there are many products for code generation in open-source large models. However, due to the data security and specificity of financial enterprises, the code generated by open-source models is often inadequate for the internal development systems and standards of financial institutions. To complete the layout of AIBank, it is necessary to achieve breakthroughs in the first stage. Shenzhou Information’s first product, CodeMaster, has been launched.
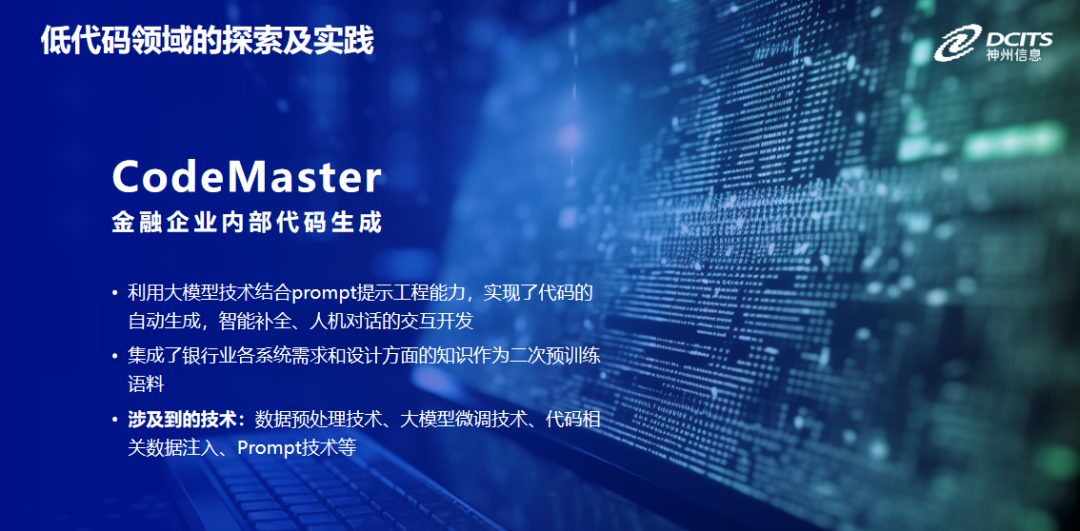
This product consists of three parts: generating code from design documents, generating unit test code from code, and generating/completing design documents from code. These three parts can form a complete closed loop, and combined with human-computer interaction during the process, corrections, and data labeling, gradually form a positive cycle that continuously enhances overall generation quality.
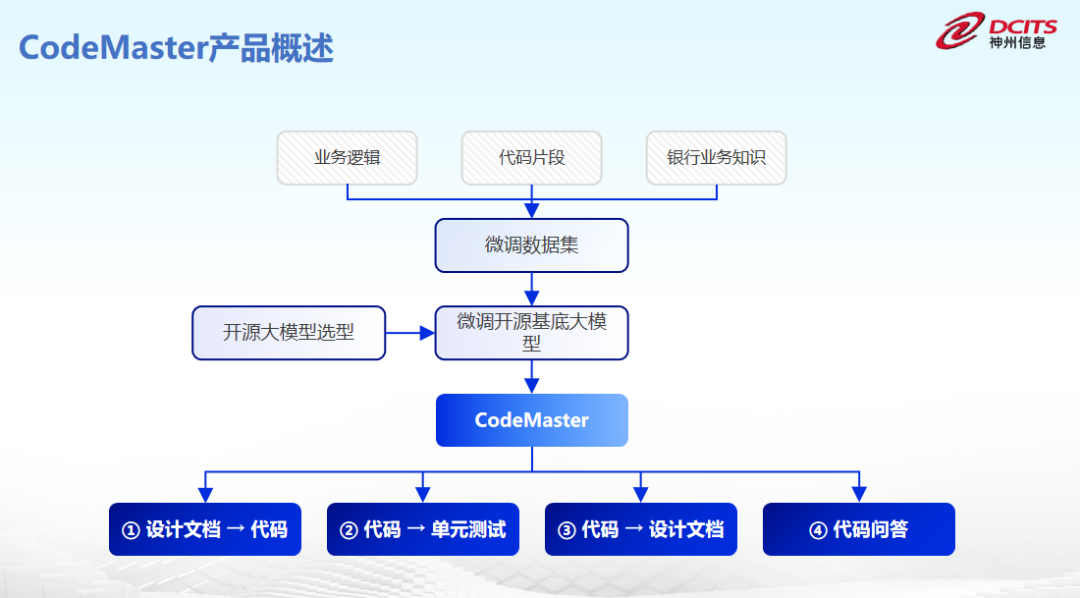
Taking a certain bank’s client as an example, primarily focused on query-type transactions, gradually expanding to the development of query interfaces for complex financial transaction scenarios. By constructing a basic version of a large model with low-code generation, and integrating various fine-tuning algorithms like LoRA along with internally constructed datasets and Prompt techniques, the development work that originally took 2 hours can be compressed to 5-10 minutes, forming a unified code logic encapsulation style that enhances readability, facilitates management and maintenance, and avoids bugs, vulnerabilities, and defects caused by manual coding, thus optimizing the capabilities of large model low-code generation and usability.
In the unit testing scenario, traditional unit testing work is cumbersome and labor-intensive, and it is easy for the code to be inconsistent with the unit tests. Therefore, CodeMaster can generate corresponding unit test code with one click, and after continuous fine-tuning and optimization of the model, it has reached a level close to real business data, with overall code coverage being quite high and time consumption reduced by 60% compared to traditional development. Considering that the ratio of code development to unit test case writing is approximately 1:1 or even higher (in cases of high coverage), the contribution of code development plus unit testing to the entire development process is nearly 30%.
The overall effect is remarkable: the time spent on design documents – code/unit testing is reduced by about 40% compared to traditional development models, and the time spent on code – design document parts is reduced by about 75%. Additionally, CodeMaster is also enhancing its capabilities in “code Q&A,” which can further improve the work efficiency of developers.
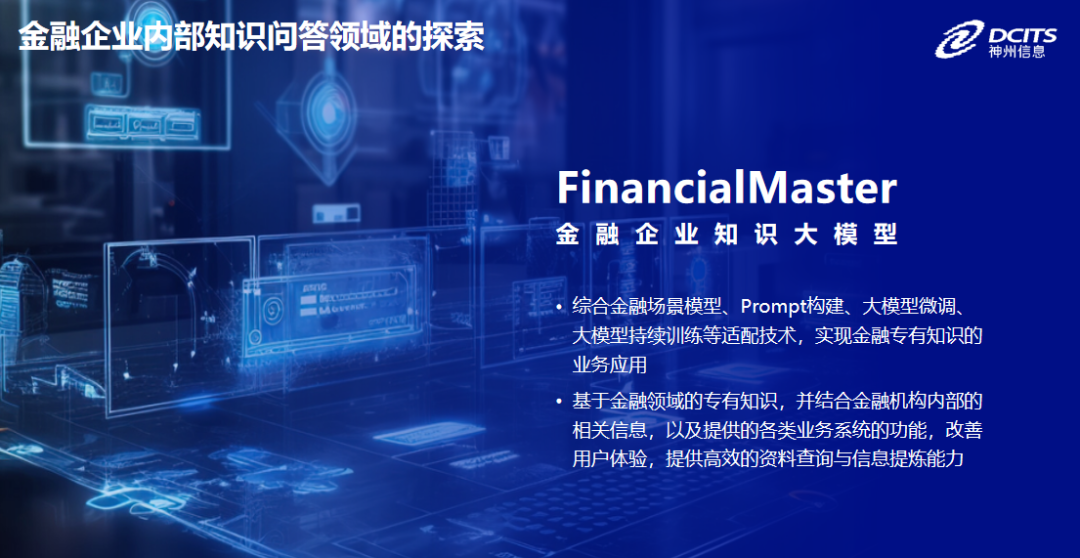
Knowledge Q&A, as another main battlefield in the large model field, is represented by another large model product developed by Shenzhou Information, FinancialMaster, which integrates financial scenario models, Prompt construction, large model fine-tuning, and continuous training technologies to achieve the business application of proprietary financial knowledge. It combines relevant information from financial institutions and the functionalities of various business systems to improve user experience and provide efficient information retrieval and extraction capabilities.
Shenzhou Information continuously deepens its practice of large models, stimulating new momentum for customer success. Starting from scenario large models and guided by the five-step strategy, it demonstrates tremendous value in terms of industry relevance, efficiency enhancement, and cost reduction, continuously optimizing financial technology services and injecting new technological momentum into supporting the real economy.
(Source: Shenzhou Information)

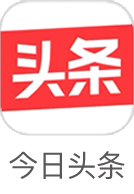
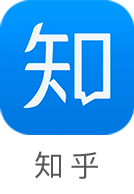
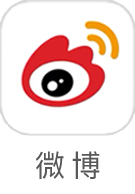
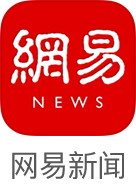
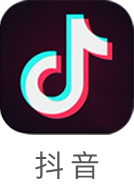
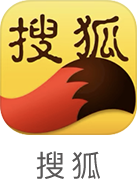
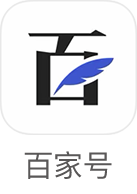
New Media Center
Director / Kuang Yuan
Editors / Yao Liangyu Fu Tiantian Zhang Jun Tai Siqi