Following the release of large models for language, code, mathematics, etc., DeepSeek has brought another early achievement on the journey towards AGI…
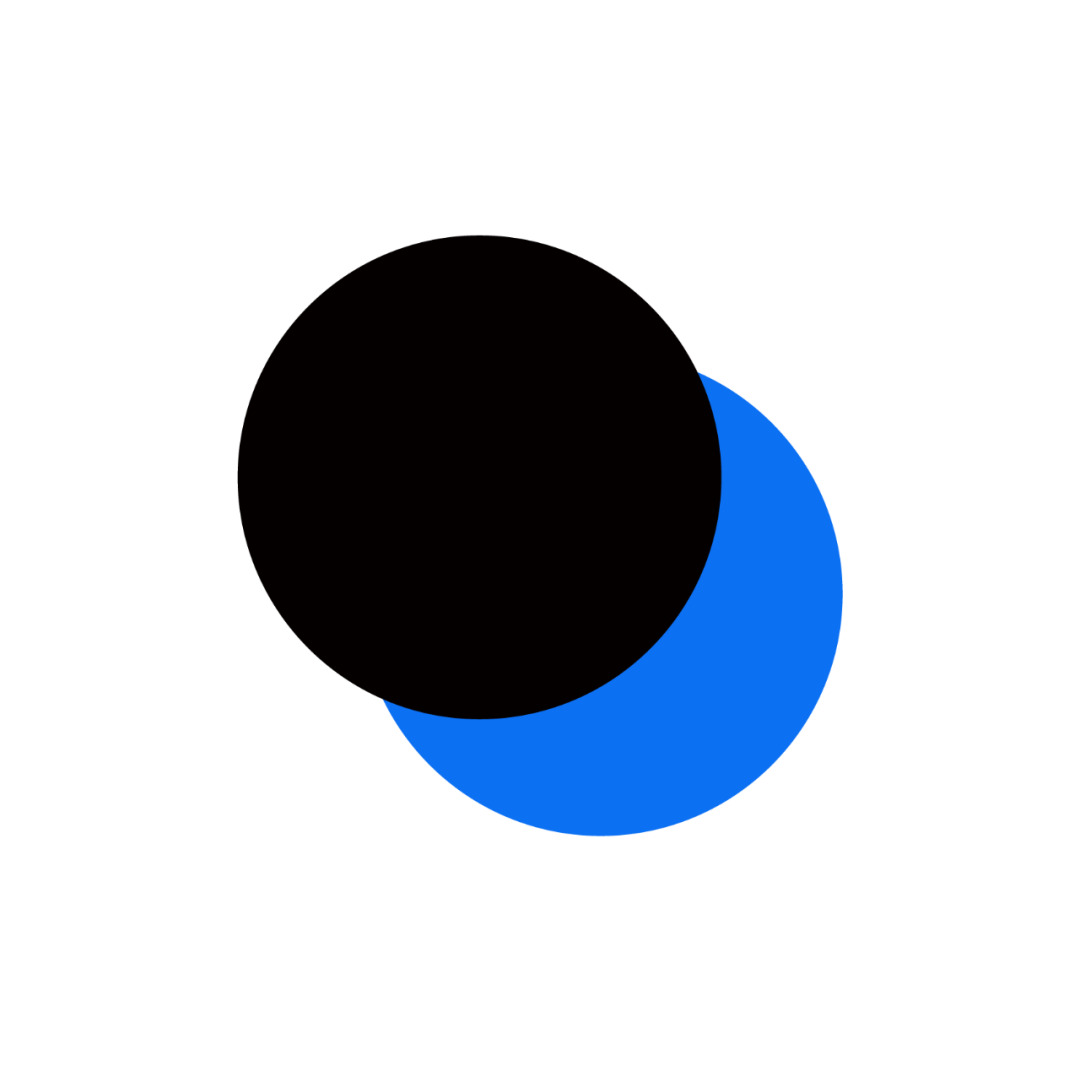
-
Data: Multi-source multimodal data enhances the model’s general cross-modal capabilities, mixing a large proportion of pure text data to maintain the model’s language capabilities without degradation.
-
Architecture: Employs a dual-visual encoder structure that is sensitive to both low-level visual signals and high-level semantic information.
-
Training: Utilizes a three-phase training method, first aligning visual and language spaces, then enhancing the model’s general cross-modal understanding through pre-training, and finally aligning with human preferences using specific task data.
-
Experiments: Surpasses models of the same scale (7B parameters) such as EMU2-Chat/Yi-VL, and even exceeds larger scale models (17B parameters) like CogVLM.
Paper link: https://arxiv.org/abs/2403.05525
Model download: https://huggingface.co/deepseek-ai
GitHub homepage: https://github.com/deepseek-ai/DeepSeek-VL
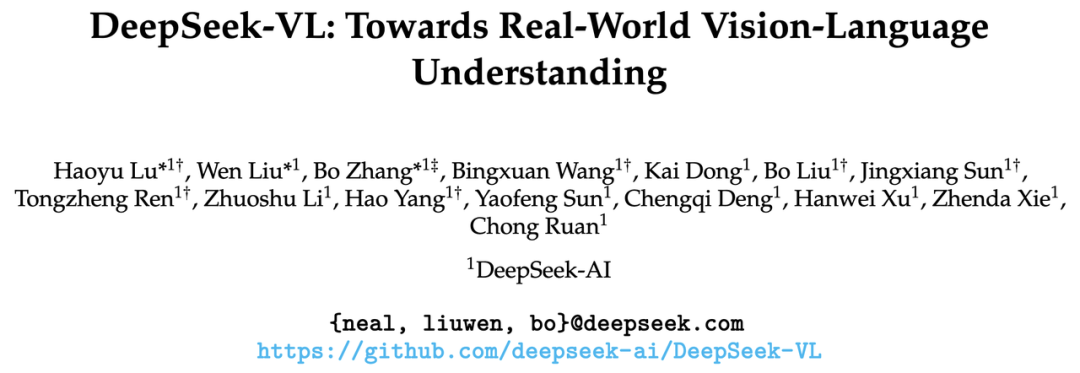
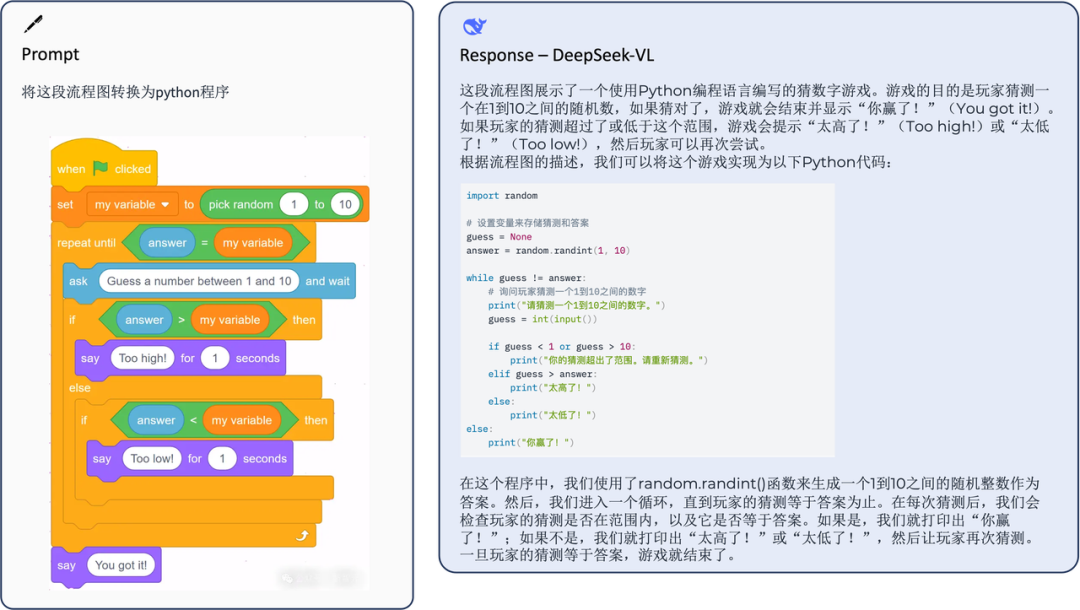
DeepSeek-VL integrates multimodal capabilities without losing language ability, providing detailed and organized responses to most real-world scenario questions. It can accept high-resolution images as input, up to 1024×1024, recognizing small objects within images. It also possesses general multimodal understanding capabilities, handling logic diagrams, web pages, formula recognition, scientific literature, natural images, and demonstrating intelligence in complex scenarios.
What is the actual experience like? Let’s look at some examples.
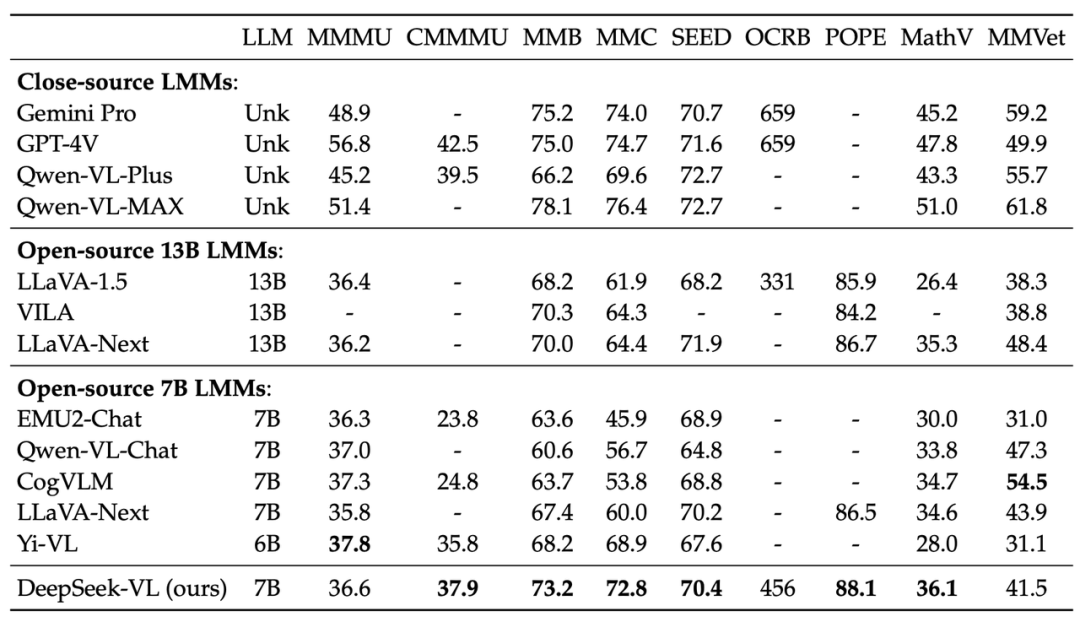
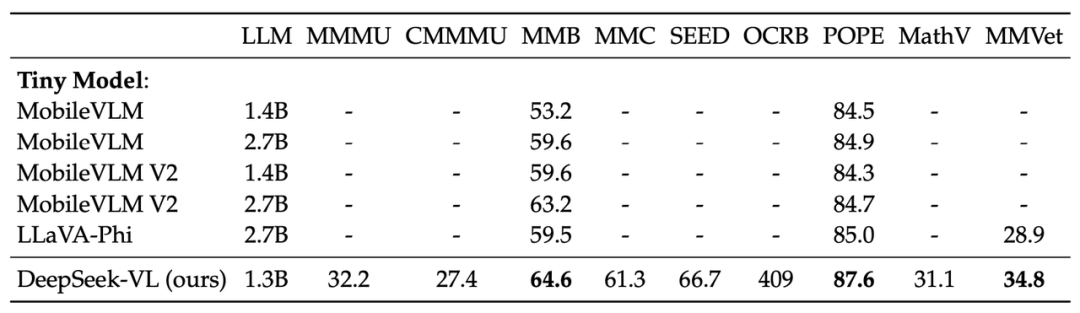
Data – Diverse and Scalable
We are committed to ensuring our data is diverse while also being scalable. The data comes from resources such as Common Crawl, web code, e-books, educational materials, and arXiv articles. Our dataset broadly covers real-world scenarios including web page screenshots, PDF files, OCR datasets, charts, and knowledge-based content (expert knowledge, textbooks), aiming to include actual real-world scenarios as much as possible.
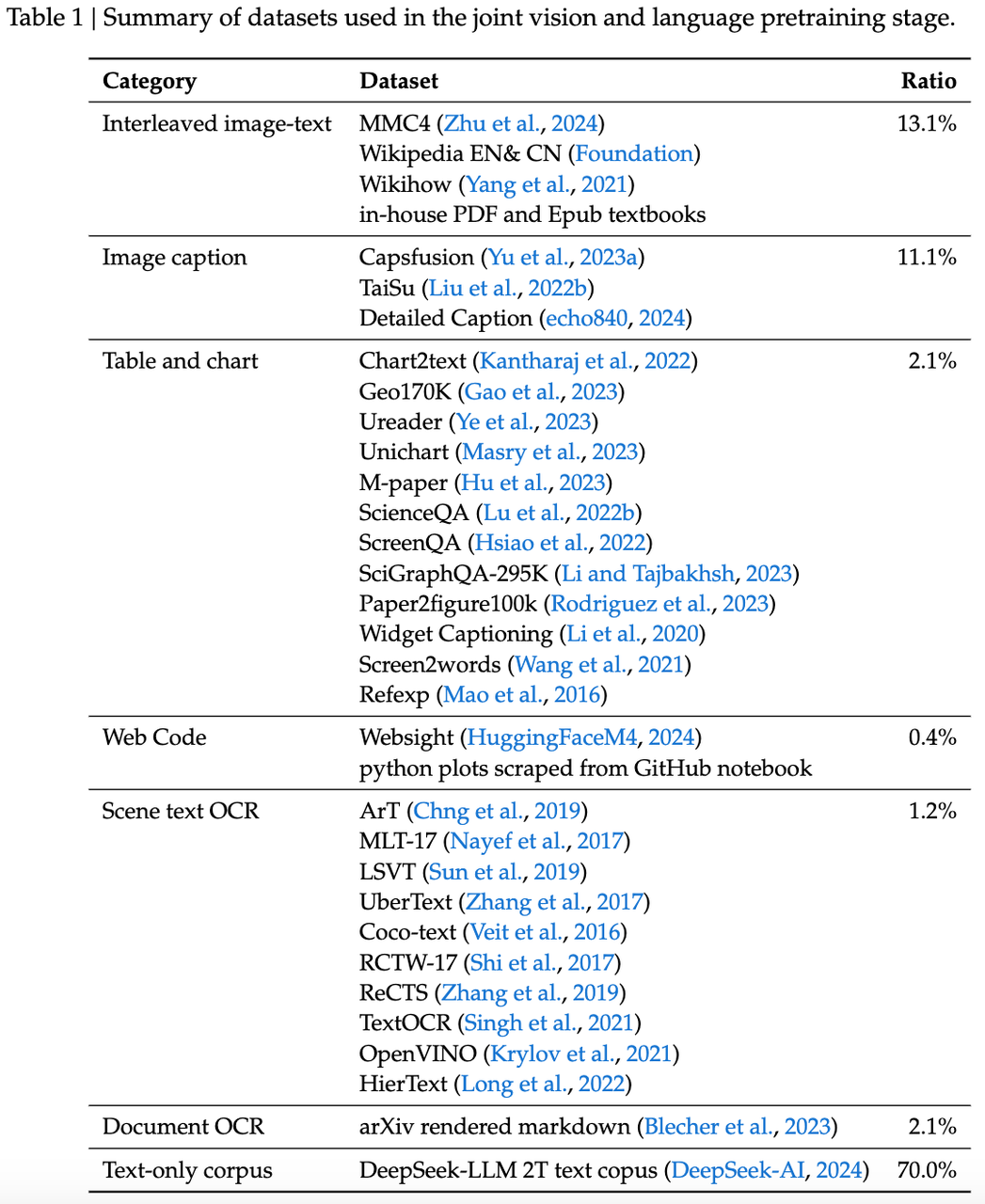
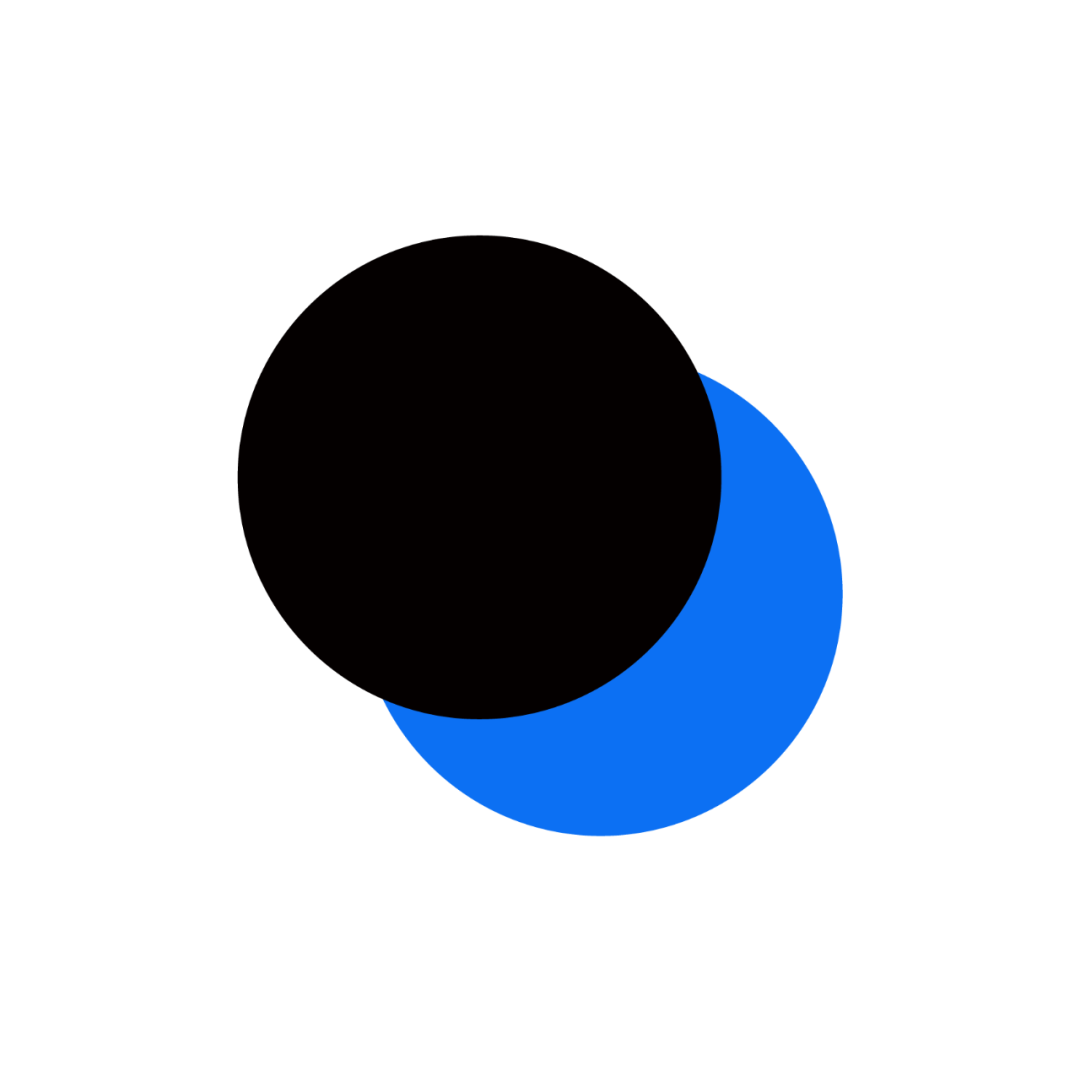
Considering efficiency and the needs of most real-world scenarios, DeepSeek VL integrates a hybrid visual encoder that effectively handles high-resolution images (1024 x 1024) while maintaining relatively low computational overhead. This design choice ensures the model captures key semantics and details of various visual tasks.
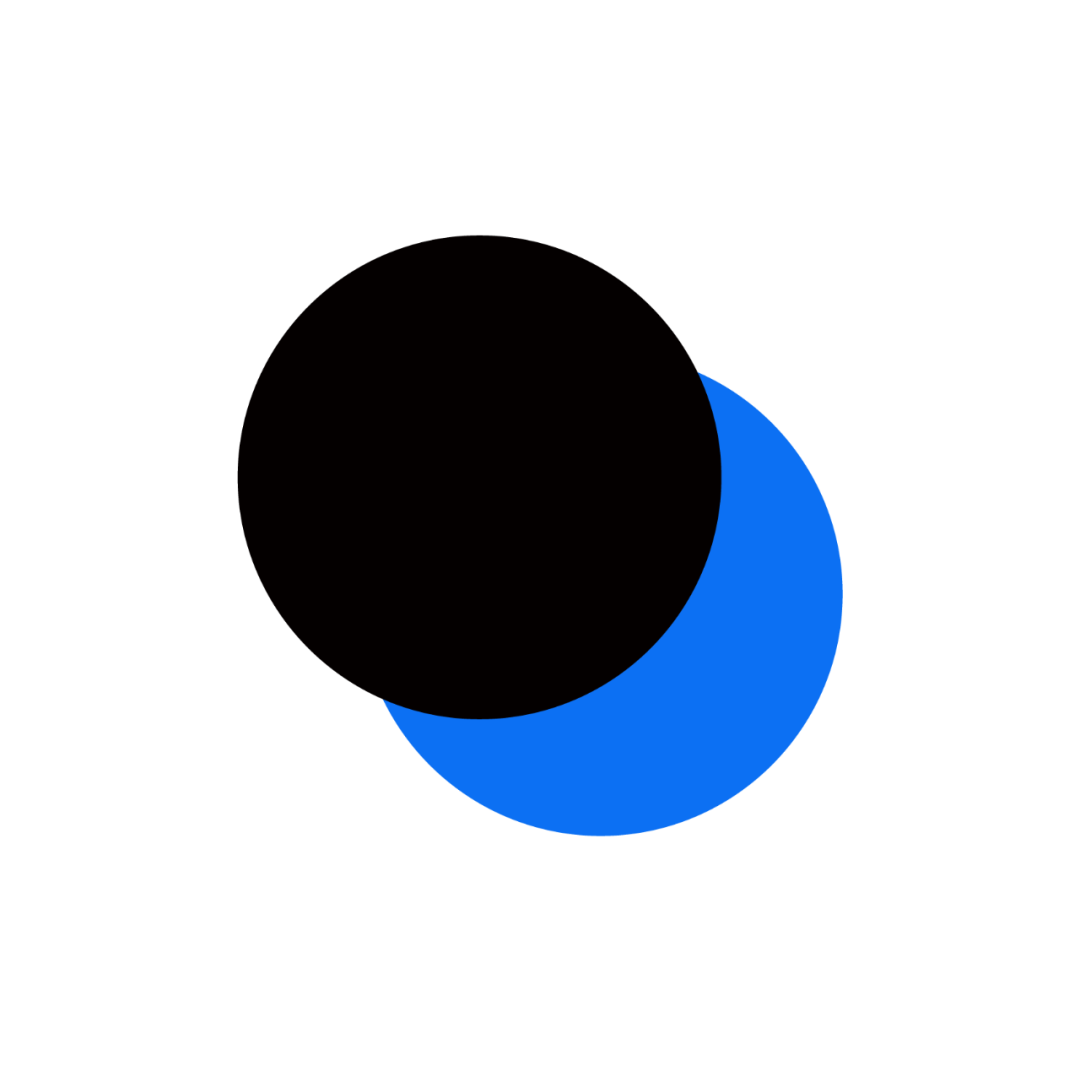
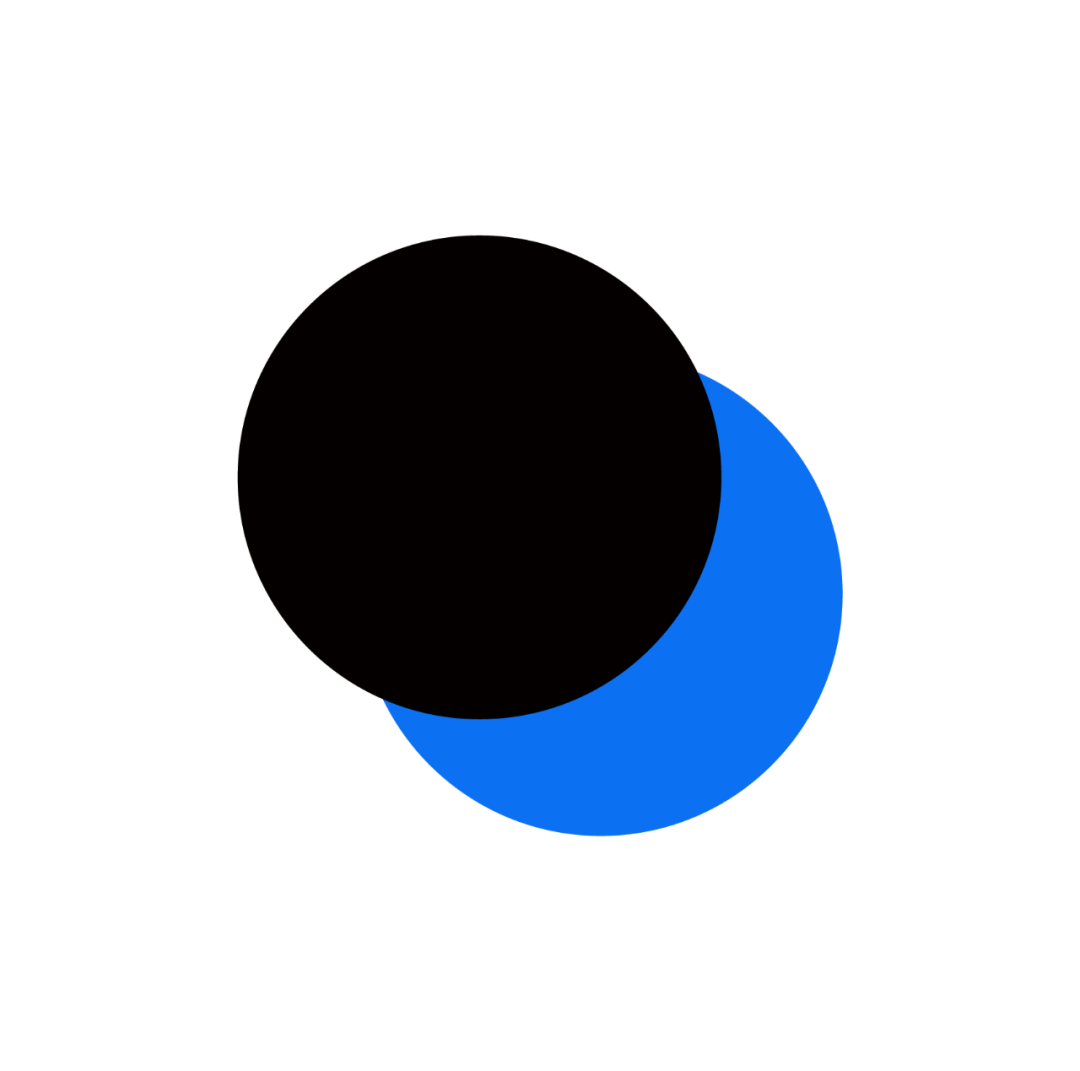
DeepSeek (深度求索) is dedicated to exploring the essence of AGI, gathering more creativity and productivity through open source.
In the future, we will continue to release larger scale, innovative frameworks, and models with better complex reasoning capabilities!
—end—